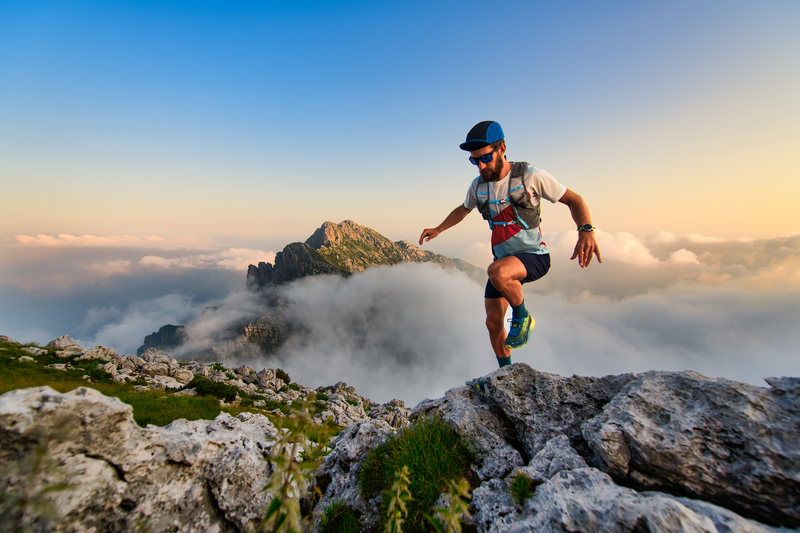
95% of researchers rate our articles as excellent or good
Learn more about the work of our research integrity team to safeguard the quality of each article we publish.
Find out more
SYSTEMATIC REVIEW article
Front. Pharmacol. , 08 August 2023
Sec. Drugs Outcomes Research and Policies
Volume 14 - 2023 | https://doi.org/10.3389/fphar.2023.1220950
This article is part of the Research Topic Novel methods and technologies for the evaluation of drug outcomes and policies View all 15 articles
Objectives: Health economic evaluations (HEEs) help healthcare decision makers understand the value of new technologies. Artificial intelligence (AI) is increasingly being used in healthcare interventions. We sought to review the conduct and reporting of published HEEs for AI-based health interventions.
Methods: We conducted a systematic literature review with a 15-month search window (April 2021 to June 2022) on 17th June 2022 to identify HEEs of AI health interventions and update a previous review. Records were identified from 3 databases (Medline, Embase, and Cochrane Central). Two reviewers screened papers against predefined study selection criteria. Data were extracted from included studies using prespecified data extraction tables. Included studies were quality assessed using the National Institute for Health and Care Excellence (NICE) checklist. Results were synthesized narratively.
Results: A total of 21 studies were included. The most common type of AI intervention was automated image analysis (9/21, 43%) mainly used for screening or diagnosis in general medicine and oncology. Nearly all were cost-utility (10/21, 48%) or cost-effectiveness analyses (8/21, 38%) that took a healthcare system or payer perspective. Decision-analytic models were used in 16/21 (76%) studies, mostly Markov models and decision trees. Three (3/16, 19%) used a short-term decision tree followed by a longer-term Markov component. Thirteen studies (13/21, 62%) reported the AI intervention to be cost effective or dominant. Limitations tended to result from the input data, authorship conflicts of interest, and a lack of transparent reporting, especially regarding the AI nature of the intervention.
Conclusion: Published HEEs of AI-based health interventions are rapidly increasing in number. Despite the potentially innovative nature of AI, most have used traditional methods like Markov models or decision trees. Most attempted to assess the impact on quality of life to present the cost per QALY gained. However, studies have not been comprehensively reported. Specific reporting standards for the economic evaluation of AI interventions would help improve transparency and promote their usefulness for decision making. This is fundamental for reimbursement decisions, which in turn will generate the necessary data to develop flexible models better suited to capturing the potentially dynamic nature of AI interventions.
The use of artificial intelligence (AI) has significantly grown in the healthcare sector. Exploiting its ability to streamline tasks, provide real-time analytics, and process larger quantities of data has contributed to its increased prominence (Panch et al., 2018). Additionally, it may have the potential to deliver quality care at lower costs. AI is being used to address challenges ranging from staff shortages to ageing populations and rising costs (Dall et al., 2013). The number of AI technologies approved by the US Food and Drink Administration (FDA) was nearly 350 between 2016 and mid-2021, compared to less than 30 in the preceding 19 years (Miller, 2021).
Several systematic reviews have been published that examine health economic evaluations (HEEs) for AI in healthcare. The most recent is Voets et al. (1 April 2021) (Voets et al., 2022), who searched for publications from 5 years prior and included 20 full texts, discussing the methods, reporting quality and challenges. They found that automated medical image analysis was the most common type of AI technology, just under half of studies reported a model-based HEE, and the reporting quality was moderate. Overall, Voets et al. concluded that HEEs of AI in healthcare often focus on costs rather than health impact, and insight into benefits is lagging behind the technological developments of AI.
An up-to-date representation of the economic evidence base may be insightful. Clearly, AI is a rapidly developing area in healthcare, demonstrated by the National Institute for Health and Care Excellence (NICE) recently incorporating AI technologies into its Evidence Standards Framework (Unsworth et al., 2021; National Institute for Health and Care Excellence, 2022). While some of this rise may be attributable to changes in legislation, it indicates the importance of AI in the current healthcare climate and the need to have a contemporary understanding of its economic value. Additionally, the COVID-19 pandemic has led to a rapid increase in the digitalization of data and health services including teleconsultations, online prescriptions and remote monitoring (Gunasekeran et al., 2021). Therefore, we sought to update the Voets et al. systematic review. We report updated results consistent with the original review, by disaggregating the HEEs into costs, clinical effectiveness, modelling characteristics and methodologies to understand common techniques, limitations, assumptions, and uncertainties. This update allows us to advance the discussion around whether existing modelling methods and reporting standards are suitable to appropriately assess the cost effectiveness of AI technologies compared to non-AI technologies in healthcare.
This review was undertaken to inform ongoing work within the HTx project. HTx is a Horizon 2020 project supported by the European Union lasting for 5 years from January 2019. The main aim of HTx is to create a framework for the Next-Generation Health Technology Assessment (HTA) to support patient-centred, societally oriented, real-time decision-making on access to and reimbursement for health technologies throughout Europe.
The search strategy included the period from 1 April 2021 to 17 June 2022, in order to update the original search conducted by Voets et al. (Voets et al., 2022). The original search used the PubMed and Scopus databases. For the present update, the original search strategy was translated for use in MEDLINE, EMBASE, via the Ovid platform, and Cochrane Central, via Wiley. These databases were preferred due to their accessibility, and searching all 3 was considered to provide comparable coverage to PubMed and Scopus (Ramlal et al., 2021).
The search strategy was simplified into 2 concept pathways: 1. “Artificial intelligence” and 2. “Health economic evaluations”. The search queries in Supplementary Appendix SA show the strategies divided into their respective databases. Subsequent terms in the AI pathway included, “artificial intelligence”, “machine learning”, and “data driven”. The second pathway included terms such as, “cost effectiveness”, “health outcomes”, “cost”, “budget”. An English language query was applied to the search strategy. The initial database selection and search strategies were guided by NICE information specialists. The review and search protocol were not registered.
Studies were included if they were a HEE of an AI intervention and a comparator, such as current standard of care or a non-AI intervention. This included trial-based economic evaluations and model-based studies. There were no exclusion criteria on types of economic evaluation, such that cost-effectiveness analyses (CEAs), cost-utility analyses (CUAs), cost-minimization analyses (CMA) and budget impact analyses (BIAs) were included. We term all of these as HEEs, which are defined as the “comparative analysis of alternative courses of action in terms of both their costs and consequences” (Rudmik and Drummond, 2013). CEAs evaluate whether an intervention provides relative value, in terms of cost and health outcomes, to a respective comparator. CUAs are a subset of CEAs where the health outcome includes a preference-based measure such as the Quality Adjusted Life Year (QALY). BIA studies evaluate the affordability of an intervention for payers to allocate resources. Included studies reported a quantitative health economic outcome such as costs, or costs in relation to effectiveness. For the exclusion criteria in the initial screening of titles and abstracts, studies that were not original research or systematic reviews such as commentaries, letters, and editorials were excluded. Overall, the inclusion and exclusion criteria were consistent with Voets et al. (Voets et al., 2022).
After duplicates were removed, 2 reviewers independently screened titles, and abstracts. The reviewers discussed any discrepancies, and where agreement could not be reached, an independent third reviewer was consulted. The same process was followed for subsequent full-text screening.
The data extraction was initially completed by 1 reviewer, and then validated by a second reviewer who independently extracted and compared data from the included studies. The extraction strategy was divided into three components, the first and second components included the characteristics and the methodological details of the studies. The former included aspects such as the purpose of the AI technology, medical field, funding, care pathway phase (prevention, diagnostics, monitoring, treatment) and the type of AI (i.e., pattern recognition, risk prediction, etc.). The second table of methodological details included aspects such as the type of HEE, the comparator, and the outcome measure. The third component was relevant only for model-based HEEs, extracting parameters such as model states, time horizon, and details of sensitivity analyses.
The extracted data were synthesised using a narrative approach as heterogeneity between studies inhibited the utility of a quantitative synthesis. Descriptive statistics were used to summarize the characteristics of the retrieved studies, where appropriate.
The quality assessment of all included studies was conducted using the NICE quality appraisal checklist for economic evaluations (National Institute for Health and Care Excellence, 2012). This checklist has been adopted in the literature of economic evaluation reviews (Elvidge et al., 2022) and is used by NICE when assessing HEE evidence for all public health guidelines. Included studies with a decision-analytic model were quality assessed independently by 2 reviewers using the methodological checklist section of the quality appraisal checklist. The checklist has 11 individual questions to create an overall assessment of whether there are minor-, potentially serious-, or very serious limitations that affects the robustness of the results. Quality assessment was not used as part of the exclusion criteria, as one of the research aims was to explore the reporting standards.
Although it is not possible to fully remove the potential of bias due to the subjective nature of the assessment, pre-set criteria were created to minimize its effects. The criteria are as follows: studies with very serious limitations included studies that had significant modelling discrepancies that could materially change the cost-effectiveness conclusion (e.g., the intervention changing from dominant to dominated). Also, very serious limitations are derived from a financial conflict of interest, where the developer of the AI technology also funded the HEE. Potentially serious limitations refer to methodological uncertainties which may change the quantitative result (e.g., an increase in the cost-effectiveness ratio), however the outcome could stay the same (e.g., the increase is not meaningful). All other limitations were considered to be minor limitations. The reviewers discussed any discrepancies in their quality assessments, and if major disagreements emerged, an independent third reviewer was consulted.
The searches across the 3 databases yielded 4,475 records, resulting in 3,033 unique records following deduplication (Table 1). After screening titles and abstracts against the study selection criteria 2,993 were excluded due to not relating to a human health intervention, not reporting a HEE, not relating to an AI-based intervention, or being a excludable study type (e.g., commentary). Therefore, 40 studies proceeded to full-text screening. Of those, 16 were excluded based on the selection criteria, and 2 were excluded as duplicates that had already been included in the Voets et al. review (Voets et al., 2022). We excluded a further study due to unclear reporting about whether it was a primary analysis or a review of other economic models. Therefore, 21 studies remained which were suitable for data extraction. See Figure 1 for the PRISMA flowchart showing the inclusion and exclusion stages.
FIGURE 1. PRISMA flowchart describing study selection and reasons for exclusion during full-text screening.
The general characteristics of the 21 included studies are presented in Table 2. The majority were published in 2022. There was a wide variation of AI interventions in different medical fields. The most frequent were general medicine and oncology (each 4/21, 19%), followed by ophthalmology and respiratory medicine (each 3/21, 14%), cardiology (2/21, 10%), and dermatology, mental health, radiology, sleep and analgesics (each 1/21, 5%). The interventions spanned the screening (9/21, 43%), diagnosis (8/21, 38%), treatment (1/21, 5%) and monitoring (3/21, 14%) stages of the clinical pathway. The most common type of AI evaluated was automated image analysis (9/21, 43%). Others were risk prediction (6/21, 29%), pattern recognition (2/21, 10%), personalized treatment recommendation (1/21, 5%), clinical decision support (1/21, 5%) and combined risk prediction and clinical decision support (2/21, 10%). Most studies were funded by governments and industry (each 5/21, 24%), followed by academia (3/21, 14%). Two (2/21, 10%) were jointly funded by industry and academia and one (1/21, 5%) was funded by the European Commission.
The 21 HEEs contained 10 (10/21, 48%) CUAs, 8 (8/21, 38%) CEAs and 2 (2/21, 10%) BIAs. One (1/21, 5%) HEE reported results as both a CEA and a CUA. Among the CEAs the outcomes ranged from cost saved per patient screened, cost per death averted, cost per DALY averted, cost per case prevented and cost saving per additional tooth retention year. The healthcare system perspective was the most common. Of the 21, 10 (10/21, 48%) took a healthcare system perspective, 6 (6/21, 29%) payer, 4 (4/21, 19%) societal and 1 study (1/21, 5%) took both a societal and health system perspective. In some studies, the payer perspective represented insurers, both public and private.
The time horizon for the 21 studies ranged from 8 weeks to lifetime, with lifetime being the most common (5/21, 24%). One year was the second most common time horizon (3/21, 14%), followed by 6 months and 5 years with two each (2/21, 10%). Time horizons of 8 weeks, 16 months, 3 years, 15 years, 20 years, 30 years, and 35 years were all present in one study each (1/21, 5%). In two studies the time horizon was not reported (2/21, 10%). Most HEEs with a time horizon longer than 1 year used a 3% annual discount rate (7/13, 54%). Six studies discounted costs and health outcomes differentially. Of these, 2 studies (2/13, 15%) discounted costs at 4% and health outcomes at 1.5%, 2 (2/13, 15%) discounted the costs but did not report discount rates for health outcomes, 1 (1/13, 8%) used undiscounted costs but did not report discounting of health outcomes, and 1 (1/13, 8%) did not report discount rates for the costs but discounted health outcomes at 3%. Table 3 reports all the methodological details of the included HEEs.
Of the 21 HEEs, 16 (16/21, 76%) included a decision analytic model. The modelling characteristics of these are summarized in Table 4. The most common model types were Markov models (6/16, 38%) and decision trees (4/16, 25%) with 3 (3/16, 19%) using a short-term decision tree followed by a longer-term Markov component. Of the remaining 3 studies, there was 1 cost simulation, 1 Markov chain Monte Carlo simulation, and 1 hybrid decision tree and microsimulation model. Authors typically justified their chosen model type by linking the decision to the type of AI intervention, the outcome measure, and the time horizon. Most Markov models used a cycle length of 1 year, and the rest used 1 month or 1 day. Studies that used decision tree models stated their primary reason for doing so was for their simplicity.
In terms of results, 7 (7/21, 33%) HEEs reported the AI intervention was cost effective versus the comparator relative to an appropriate threshold value, 5 (5/21, 24%) demonstrated that the AI intervention was dominant, and 2 (2/21, 10%) demonstrated equivalence. In 1 (1/21, 5%) study the AI intervention was cost effective versus one comparator and dominant versus the other. In 2 (2/21, 10%) studies the AI interventions produced savings. Three (3/21, 14%) studies did not state a preferred cost-effectiveness threshold to determine if the result was cost effective. The AI intervention was found to be cost ineffective in 1 (1/21, 5%) study.
Of the studies that reported sensitivity analysis (18/21, 86%), 17 reported one-way sensitivity analyses, though the remaining study did conduct probabilistic sensitivity analysis. Seven (7/21, 33%) studies reported both one-way and probabilistic analyses, while 4 (4/21, 19%) reported both one-way and scenario analyses. Three studies (3/21, 14%) reported one-way, probabilistic and scenario analyses.
A summary of the results from the quality appraisal checklist is shown in Table 5. The assessment resulted in 6 (6/21, 29%) studies with very serious limitations, 11 (11/21, 52%) with potentially serious limitations, and 4 (4/21, 19%) with minor limitations. Initially the two reviewers disagreed on the assessment for two of the studies (Ericson et al., 2022; Mital and Nguyen, 2022). Both were upgraded for the reasons given below.
Studies deemed to have very serious limitations were those where an issue in 1 or more quality criteria were highly likely to materially change the cost-effectiveness conclusion for the AI intervention. There were several key reasons which led to this assessment for 5 of the included studies. In one there was an acknowledged overestimation of cost data, representation issues between the dataset and target population, and a short 6-month horizon rather than the 12-month time horizon deemed best practice by the American College of Radiology (Rosenthal and Dudley, 2007). In another, adverse health effects were not captured, which the authors suggested would increase the cost-effectiveness estimate (Fusfeld et al., 2022). This study also had a financial conflict of interest where research was funded by the company which developed the AI intervention. This was true for another 2 studies (Ericson et al., 2022; Szymanski et al., 2022). In another study, the result changed from intervention dominant to cost ineffective when input data, arising from multiple sources and assumption, were varied during the sensitivity analyses (Ziegelmayer et al., 2022).
Studies with potentially serious limitations tended to have a paucity of appropriate input data. Instead, alternative sources, or multiple sources were used with resulting generalizability issues. It was common for studies to have assumptions for the cost and effectiveness of the AI intervention, compliance, and the impact of the AI intervention on the subsequent treatment pathway. Examples of this are 1 study that assumed all patients would consent to a test (Mallow and Belk, 2021); 1 study that used a primary outcome that was patient reported (Delgadillo et al., 2022) and 1 study that assumed the effectiveness of the AI intervention last for 10 years, despite having data for only 5 years (Mital and Nguyen, 2022). These studies did account for the key uncertainties in sensitivity analyses and the effect was either minor or the initial assumptions were shown to be robust. Some studies were assessed as having potentially serious limitations due to unclear reporting, which reduced transparency around key information such as whether a cost had been applied for the AI intervention, how it would integrate with clinical care, and who the anticipated user of the AI intervention was.
This paper systematically reviewed 21 HEEs of AI interventions. The studies mainly evaluated AI-based automated image analysis interventions for diagnosis and screening in general medicine, oncology and ophthalmology. Nearly all were CUAs and CEAs that took a healthcare system or payer perspective, and most had lifetime time horizons. Some of the HEEs were trial-based analyses, but the large majority were model-based which mostly used Markov models. In terms of the HEE results, the AI interventions were cost effective or dominant in just over half and all the studies performed sensitivity analyses.
This study reports an updated search to the review conducted by Voets et al. (Voets et al., 2022), providing a contemporary snapshot of the HEE evidence base for AI health technologies Our update captures an additional 15-month period in a time where AI health based technologies are on the exponential rise, evidenced by the near quadruple number of initial unique search results since April 2021 (Voets et al., 2022). It appears there has been no change in the most commonly evaluated purpose of AI being used as a healthcare intervention, as Voets et al. also found the most common to be automated image analysis (Voets et al., 2022). Ophthalmology and screening were the dominant specialty and phase of the care pathway at which the AI intervention was used, and these were also prevalent in this updated review. The prevailing type of HEE in the original review was cost minimization with the preferred outcome measure of cost saved per case identified. This was common among our included studies, although we termed it CEA, but CUA was the most common study type in this update. There was a difference between the two reviews in how many of the technologies were found to be cost saving. Voets et al. found the majority were whilst this was true for only 2 studies in this review. This could be due to differences in applying the terms ‘cost-saving’ and ‘cost-effective’ as a large proportion of studies in this updated review were cost-effective.
Another difference was the fact that the large majority of HEEs in our review were model-based, compared to 45% of those in Voets (Voets et al., 2022). This could suggest a shift towards using models to estimate future costs and benefits of AI technologies, permitting longer time horizons than trial-based evaluations (the most common time horizon is our review was lifetime, compared to 1 year in Voets). Furthermore, the increasing use of model-based evaluations may suggest AI interventions are moving towards traditional value assessment frameworks that are commonplace in the health technology assessment of medicines. This increase in model-based technologies may also explain the differences in results regarding cost saving versus cost effective. Perhaps it is easier or more expected to generate cost-effectiveness estimates when using a model compared to non-model HEEs where it may be more common to focus on costs.
Voets et al. (2022) found that the evidence supporting the chosen analytical methods, assessment of uncertainty, and model structures was underreported. Our quality assessment determined that most studies had potentially serious limitations tending to arise from the sources and assumptions regarding the input data. These findings are consistent, which suggests that despite an increase in the use of more sophisticated economic evaluation techniques, the evidence supporting them remains limited. In some cases, the uncertainty and lack of clarity for the reader were due to the reporting of the HEE rather than the data quality. In numerous studies it was hard to determine fundamentals such as whether a cost had been applied for the AI intervention, how it would integrate with clinical care and who the anticipated user of the AI intervention was. As mentioned, not all of the studies we identified clearly stated how the AI intervention would integrate with clinical care. Studies did not typically thoroughly or transparently estimate subsequent care and downstream health outcomes resulting from the use of an AI intervention. Our findings from this literature review suggest this is an area that needs to be better considered and reported.
AI-based interventions have the potential to be distinct from traditional medical interventions if they can learn (from data) over time. Theoretically, this means the relationship between the intervention and outcome may not be fixed; an AI intervention could get more effective over time, unlike the typical effect waning assumption associated with medicines. This has implications when considering future benefits and how to extrapolate this over the time horizon of the HEE. The prevailing model structures used in HEEs of AI interventions to date—Markov models, decision trees, and hybrids of the 2—may limit the extent to which studies have been able to capture and examine the dynamic nature of AI interventions. Therefore, there is the possibility that the existing HEE evidence base has not captured the true potential value of many AI interventions due to limitations imposed by their model structures, and only a third of our included studies explored the impact of structural uncertainty in sensitivity analysis. Furthermore, traditional, ‘simple’ models may not facilitate easy modelling of downstream costs and benefits, by quickly becoming slow or unwieldy. This, potentially, fails to show the full benefit of the AI intervention, inhibiting implementation. Guo et al. (Guo et al., 2020) acknowledge this through a paradox of “no evidence, no implementation—no implementation, no evidence”. More sophisticated types of model, that are less restricted by the structural limitations that affect simple decision tree and Markov models may be better placed to capture full pathway effects in addition to potential time-dependent effectiveness of AI-based interventions.
Simulation-based modelling presents the opportunity to build flexible, sophisticated models that can overcome several limitations of Markov models and decision trees. They can easily incorporate the history of past events, model factors that can vary between patients and have a non-linear relationship with outcomes, and do not use discrete time intervals (Davis et al., 2014). They can also track the path of each person over time and estimate individual-level effects or mean group-level effects for a population (Davis et al., 2014). These possibilities may lead to models capable of addressing the potential dynamic nature of AI interventions learning over time and the impact on linked decision points and subsequent care in a clinical pathway. As data on AI-based interventions continues to be collected and reported, the ability to develop these models should improve. One thing to note, however, is that for these models to underpin reimbursement decisions HTA agencies would need to be able to critique and utilize them. This may require new skills, knowledge and experience and present other challenges. Utilizing these sorts of models also leads to the debate of whether HTA should be more ‘living’. This refers to regular and scheduled updates of recommendations instead of the more traditional ‘one-off’ decisions. Living HTA presents opportunities as well as challenges (Thokala et al., 2023) and is not yet common practice.
The usefulness of a published HEE for decision making depends on how well it is conducted and reported. Reporting guidelines play an important role in improving transparency and completeness and as new technologies emerge, can help drive best practice. A prominent reporting standard within the field of HEEs is the Consolidated Health Economic Evaluation Reporting Standards (CHEERS) (Husereau et al., 2022). This outlines minimum reporting standards and was recently updated in 2022. It includes a 28-item checklist covering methodological approach, data identification, model inputs, assumptions, uncertainty analysis, and conflicts of interest. It does not include any reporting items that are specific to any AI components of the intervention, but the authors did recognize that CHEERS could be more specific for certain situations and welcomed opportunities to create additional reporting guidance. An extension to CHEERS covering AI specific items could improve the reporting, transparency and ultimately decision making for AI interventions. This could also help mitigate the paradox of poor reporting inhibiting adoption of AI interventions.
The system-wide need and motivation for improving best practice around data collection and transparency for AI health interventions is evident. Extensions for AI technologies have already been developed for other checklists. CONSORT-AI (Liu et al., 2020) contains AI-specific items for the reporting of RCTs, and it was done in collaboration with the SPIRIT-AI extension for trial protocols (Rivera et al., 2020). Including AI-specific items in the reporting of HEEs may be a logical step to contribute to this standard setting and help to ensure that all relevant information is available to decision makers.
This study has some limitations. We updated the Voets et al. systematic literature review, but searched different databases. It is possible there may have been relevant studies within our search window that we missed by not searching the same databases; however, we believe the databases we searched should give at least equivalent, and probably superior, sensitivity to the original review. Indeed, the sensitivity of our search strategy is evidenced by the large number of studies excluded at primary screening (2,993) relative to the total number of unique records (3,033). The sensitivity of HEE search filters is well known (Hubbard et al., 2022). While this means our review is highly likely to have identified all relevant published studies, it does mean further updates may be labor intensive with lots of records to screen to identify a relatively small number of relevant studies.
Our review specifically focused on economic evaluations and whilst out of scope, some studies, such as those only reporting patient reported outcome measures, may have been of interest to readers. Additionally, a potential limitation is that our search only covered the period from 1 April 2021 to 17 June 2022. This relatively short search period remains informative due to the rapid advent of AI in healthcare, but it also means that it is likely that relevant economic evaluations have been published since our review.
Another limitation relates to the subjective nature of the NICE quality appraisal checklist. Although the checklist allowed for a further level of analysis regarding the quality of the economic evaluation, it should be used as a broad interpretation rather than a critique of any given study. Despite negating any potential bias by having 2 reviewers, it is possible that different reviewers may have implemented the checklist differently and produced different results. Additionally, other, similar checklists exist (Philips et al., 2004; Drummond, 2015; Adarkwah et al., 2016), and although they broadly serve a similar purpose of understanding the methodological limitations of HEEs, they may have resulted in different or more nuanced quality assessments.
This updated review, while covering just a 15-month window, found more economic evaluations of AI health interventions since the last comprehensive systematic literature review which covered the preceding 5 years. Many of the included studies were model-based evaluations and the most common AI intervention was automated image analysis used for screening or diagnosis in the areas of general medicine and oncology. Most evaluations reported the cost per QALY gained.
Overall, the reporting of the studies exhibited limitations. Only a small number of studies were judged to have just minor limitations, according to application of the NICE quality assessment checklist. The majority had potentially serious or very serious limitations resulting from conflicts between research funding and authorship, uncertainty in input data changing the outcome of the evaluation, and lack of transparent reporting of key elements, such as the cost of the technology and how it will be implemented into clinical practice. Specific reporting standards for the economic evaluation of AI interventions would help to improve transparency, reproducibility and trust, and promote their usefulness for decision making. This is fundamental for implementation and coverage decisions which in turn will generate the necessary data to develop flexible models better suited to capture the potentially dynamic nature of the AI intervention.
The raw data supporting the conclusion of this article will be made available by the authors, without undue reservation.
JV designed and conducted the systematic literature review with support from LA for the database search and management of results. JV drafted the initial manuscript, extracted data and conducted quality assessment. CH extracted data as a second reviewer, conducted quality assessment, and developed the manuscript. JE provided comments and feedback throughout JV’s project and on the manuscript development. DD oversaw the work. All authors contributed to the article and approved the submitted version.
CH, JE, and DD are funded through the HTx project. The HTx project has received funding from the European Union’s Horizon 2020 research and innovation programme under grant agreement no. 825162. This dissemination reflects only the authors’ view and the Commission is not responsible for any use that may be made of the information it contains.
The authors would like to thank Sarosh Nagar for his participation as an independent screener.
The authors declare that the research was conducted in the absence of any commercial or financial relationships that could be construed as a potential conflict of interest.
All claims expressed in this article are solely those of the authors and do not necessarily represent those of their affiliated organizations, or those of the publisher, the editors and the reviewers. Any product that may be evaluated in this article, or claim that may be made by its manufacturer, is not guaranteed or endorsed by the publisher.
The Supplementary Material for this article can be found online at: https://www.frontiersin.org/articles/10.3389/fphar.2023.1220950/full#supplementary-material
Adams, S. J., Mondal, P., Penz, E., Tyan, C. C., Lim, H., and Babyn, P. (2021). Development and cost analysis of a lung nodule management strategy combining artificial intelligence and lung-RADS for baseline lung cancer screening. J. Am. Coll. Radiology 18 (5), 741–751. doi:10.1016/j.jacr.2020.11.014
Adarkwah, C. C., van Gils, P. F., Hiligsmann, M., and Evers, S. M. A. A. (2016). Risk of bias in model-based economic evaluations: The ECOBIAS checklist. Expert Rev. Pharmacoeconomics Outcomes Res. 16 (4), 513–523. doi:10.1586/14737167.2015.1103185
Areia, M., Mori, Y., Correale, L., Repici, A., Bretthauer, M., Sharma, P., et al. (2022). Cost-effectiveness of artificial intelligence for screening colonoscopy: A modelling study. Lancet Digital Health 4 (6), 436–444. doi:10.1016/S2589-7500(22)00042-5
Dall, T. M., Gallo, P. D., Chakrabarti, R., West, T., Semilla, A. P., and Storm, M. V. (2013). An aging population and growing disease burden will require ALarge and specialized health care workforce by 2025. Health Aff. 32 (11), 2013–2020. doi:10.1377/hlthaff.2013.0714
Davis, S., Stevenson, M., Tappenden, P., and Wailoo, A. (2014) Nice dsu technical support document 15: Cost-effectiveness modelling using patient-level simulation.
de Vos, J., Visser, L. A., de Beer, A. A., Fornasa, M., Thoral, P. J., Elbers, P. W. G., et al. (2022). The potential cost-effectiveness of a machine learning tool that can prevent untimely intensive care unit discharge. Value Health 25 (3), 359–367. doi:10.1016/j.jval.2021.06.018
Delgadillo, J., Ali, S., Fleck, K., Agnew, C., Southgate, A., Parkhouse, L., et al. (2022). Stratified care vs stepped care for depression. A cluster randomized clinical trial. JAMA Psychiatry 79 (2), 101–108. doi:10.1001/jamapsychiatry.2021.3539
Drummond, M. (2015). Methods for the economic evaluation of health care programmes. Fourth: Oxford University Press.
Elvidge, J., Summerfield, A., Nicholls, D., and Dawoud, D. (2022). Diagnostics and treatments of COVID-19: A living systematic review of economic evaluations. Value Health 25 (5), 773–784. doi:10.1016/j.jval.2022.01.001
Ericson, O., Hjelmgren, J., Sjövall, F., Söderberg, J., and Persson, I. (2022). The potential cost and cost-effectiveness impact of using a machine learning algorithm for early detection of sepsis in intensive care units in Sweden. J. Health Econ. Outcomes Res. 9 (1), 101–110. doi:10.36469/jheor.2022.33951
Fusfeld, L., Menon, S., Gupta, G., Lawrence, C., Masud, S. F., and Goss, T. F. (2022). US payer budget impact of a microarray assay with machine learning to evaluate kidney transplant rejection in for-cause biopsies. J. Med. Econ. 25 (1), 515–523. doi:10.1080/13696998.2022.2059221
Gunasekeran, D. V., Tseng, R. M. W. W., Tham, Y. C., and Wong, T. Y. (2021). Applications of digital health for public health responses to COVID-19: A systematic scoping review of artificial intelligence, telehealth and related technologies. NPJ Digit. Med. 4 (1), 40–41. doi:10.1038/s41746-021-00412-9
Guo, C., Ashrafian, H., Ghafur, S., Fontana, G., Gardner, C., and Prime, M. (2020). Challenges for the evaluation of digital health solutions—a call for innovative evidence generation approaches. NPJ Digit. Med. 3 (1), 110–114. doi:10.1038/s41746-020-00314-2
Huang, X.-M., Yang, B. F., Zheng, W. L., Liu, Q., Xiao, F., Ouyang, P. W., et al. (2022). Cost-effectiveness of artificial intelligence screening for diabetic retinopathy in rural China. BMC Health Serv. Res. 22 (260), 260. doi:10.1186/s12913-022-07655-6
Hubbard, W., Walsh, N., Hudson, T., Heath, A., Dietz, J., and Rogers, G. (2022). Development and validation of paired MEDLINE and Embase search filters for cost-utility studies. BMC Med. Res. Methodol. 22 (1), 310–319. doi:10.1186/s12874-022-01796-2
Husereau, D., Drummond, M., Augustovski, F., de Bekker-Grob, E., Briggs, A. H., Carswell, C., et al. (2022). Consolidated health economic evaluation reporting standards 2022 (CHEERS 2022) statement: Updated reporting guidance for health economic evaluations. BMJ 376 (Cheers), e067975–e067977. doi:10.1136/bmj-2021-067975
Kessler, S., Desai, M., McConnell, W., Jai, E. M., Mebine, P., Nguyen, J., et al. (2021). Economic and utilization outcomes of medication management at a large medicaid plan with disease management pharmacists using a novel artificial intelligence platform from 2018 to 2019: A retrospective observational study using regression methods. J. Manag. Care Specialty Pharm. 27 (9), 1186–1196. doi:10.18553/jmcp.2021.21036
Liu, X., Cruz Rivera, S., Moher, D., Calvert, M. J., and Denniston, A. K.SPIRIT-AI and CONSORT-AI Working Group (2020). Reporting guidelines for clinical trial reports for interventions involving artificial intelligence: The CONSORT-AI extension. Nat. Med. 26 (9), e537–e548. doi:10.1016/S2589-7500(20)30218-1
MacPherson, P., Webb, E. L., Kamchedzera, W., Joekes, E., Mjoli, G., Lalloo, D. G., et al. (2021). Computer-aided X-ray screening for tuberculosis and HIV testing among adults with cough in Malawi (the prospect study): A randomised trial and cost-effectiveness analysis. PLoS Med. 18 (9), 10037522–e1003817. doi:10.1371/journal.pmed.1003752
Mallow, P. J., and Belk, K. W. (2021). Cost-utility analysis of single nucleotide polymorphism panel-based machine learning algorithm to predict risk of opioid use disorder. J. Comp. Eff. Res. 10 (18), 1349–1361. doi:10.2217/cer-2021-0115
Miller, M. (2021). FDA publishes approved list of AI/ML-enabled medical devices, IQVIA blog. Available at: https://www.iqvia.com/locations/united-states/blogs/2021/10/fda-publishes-approved-list-of-ai-ml-enabled-medical-devices (Accessed: May 9, 2023).
Mital, S., and Nguyen, H. V ( (2022). Cost-effectiveness of using artificial intelligence versus polygenic risk score to guide breast cancer screening. BMC Cancer 22 (1), 501. doi:10.1186/s12885-022-09613-1
Morrison, S. L., Dukhovny, D., Chan, R. V. P., Chiang, M. F., and Campbell, J. P. (2022). Cost-effectiveness of artificial intelligence-based retinopathy of prematurity screening. JAMA Ophthalmol. 140 (4), 401–409. doi:10.1001/jamaophthalmol.2022.0223
National Institute for Health and Care Excellence (2012). Methods for the development of NICE public health guidance.Appendix I quality appraisal checklist- economic evaluations
National Institute for Health and Care Excellence (2022). Evidence standards framework (ESF) for digital health technologies. National Institute for Health and Care Excellence. Available at: https://www.nice.org.uk/corporate/ecd7 (Accessed: May 9, 2023).
Nsengiyumva, N. P., Hussain, H., Oxlade, O., Majidulla, A., Nazish, A., Khan, A. J., et al. (2021). Triage of persons with tuberculosis symptoms using artificial intelligence-based chest radiograph interpretation: A cost-effectiveness analysis. Open Forum Infect. Dis. 8 (12), 567. doi:10.1093/ofid/ofab567
Panch, T., Szolovits, P., and Atun, R. (2018). Artificial intelligence, machine learning and health systems. J. Glob. Health 8 (2), 020303–020308. doi:10.7189/jogh.08.020303
Philips, Z., Ginnelly, L., Sculpher, M., Claxton, K., Golder, S., Riemsma, R., et al. (2004). Review of guidelines for good practice in decision-analytic modelling in health technology assessment. Health Technol. Assess. 8 (36)–iv, ix-xi, 1-158. doi:10.3310/hta8360
Ramlal, A., Ahmad, S., Kumar, L., Khan, F., and Chongtham, R. (2021). “From molecules to patients: The clinicalapplications of biological databases andelectronic health records,” in Translational bioinformatics in healthcare and medicine. Editors K. Raza, and N. Dey (First Edit. Academic Press), 107–125. doi:10.1016/B978-0-323-89824-9.00009-4
Rivera, S. C., Liu, X., Chan, A. W., Denniston, A. K., and Calvert, M. J.SPIRIT-AI and CONSORT-AI Working Group (2020). Guidelines for clinical trial protocols for interventions involving artificial intelligence: The SPIRIT-AI Extension. BMJ 370, m3210–m3214. doi:10.1136/bmj.m3210
Rosenthal, M. B., and Dudley, R. A. (2007). Pay-for-Performance. JAMA 297 (7), 740–744. doi:10.1001/jama.297.7.740
Rudmik, L., and Drummond, M. (2013). Health economic evaluation: Important principles and methodology. Laryngoscope 123 (6), 1341–1347. doi:10.1002/lary.23943
Salcedo, J., Rosales, M., Kim, J. S., Nuno, D., Suen, S. C., and Chang, A. H. (2021). Cost-effectiveness of artificial intelligence monitoring for active tuberculosis treatment: A modeling study. PloS one 16 (7), e0254950. doi:10.1371/journal.pone.0254950
Schwendicke, F., Mertens, S., Cantu, A. G., Chaurasia, A., Meyer-Lueckel, H., and Krois, J. (2022). Cost-effectiveness of AI for caries detection: Randomized trial. J. Dent. 119, 104080. doi:10.1016/j.jdent.2022.104080
Szymanski, T., Ashton, R., Sekelj, S., Petrungaro, B., Pollock, K. G., Sandler, B., et al. (2022). Budget impact analysis of a machine learning algorithm to predict high risk of atrial fibrillation among primary care patients. Eur. Eur. pacing, Arrhythm. cardiac Electrophysiol. J. Work. groups cardiac pacing, Arrhythm. cardiac Cell. Electrophysiol. Eur. Soc. Cardiol. 24 (8), 1240–1247. doi:10.1093/europace/euac016
Thokala, P., Srivastava, T., Smith, R., Ren, S., Whittington, M. D., Elvidge, J., et al. (2023). Living health technology assessment: Issues, challenges and opportunities. PharmacoEconomics 41 (3), 227–237. doi:10.1007/s40273-022-01229-4
Tseng, A. S., Thao, V., Borah, B. J., Attia, I. Z., Medina Inojosa, J., Kapa, S., et al. (2021). Cost effectiveness of an electrocardiographic deep learning algorithm to detect asymptomatic Left ventricular dysfunction. Mayo Clin. Proc. 96 (7), 1835–1844. doi:10.1016/j.mayocp.2020.11.032
Turino, C., Benítez, I. D., Rafael-Palou, X., Mayoral, A., Lopera, A., Pascual, L., et al. (2021). Management and treatment of patients with obstructive sleep apnea using an intelligent monitoring system based on machine learning aiming to improve continuous positive airway pressure treatment compliance: Randomized controlled trial. J. Med. Internet Res. 23 (10), 240722–e24112. doi:10.2196/24072
Unsworth, H., Dillon, B., Collinson, L., Powell, H., Salmon, M., Oladapo, T., et al. (2021). The NICE Evidence Standards Framework for digital health and care technologies – developing and maintaining an innovative evidence framework with global impact. Digit. Health 7, 20552076211018617–20552076211018620. doi:10.1177/20552076211018617
van Leeuwen, K. G., Meijer, F. J. A., Schalekamp, S., Rutten, M. J. C. M., van Dijk, E. J., van Ginneken, B., et al. (2021). Cost-effectiveness of artificial intelligence aided vessel occlusion detection in acute stroke: An early health technology assessment. Insights into Imaging 12 (133), 133. doi:10.1186/s13244-021-01077-4
Voets, M. M., Veltman, J., Slump, C. H., Siesling, S., and Koffijberg, H. (2022). Systematic review of health economic evaluations focused on artificial intelligence in healthcare: The tortoise and the cheetah. Value Health 25 (3), 340–349. doi:10.1016/j.jval.2021.11.1362
Xiao, X., Xue, L., Ye, L., Li, H., and He, Y. (2021). Health care cost and benefits of artificial intelligence-assisted population-based glaucoma screening for the elderly in remote areas of China: A cost-offset analysis. BMC Public Health 21 (1), 1065–1112. doi:10.1186/s12889-021-11097-w
Keywords: artificial intelligence, cost effectiveness, cost utility, simulation models, health economic evaluation, mixed-methods, systematic review
Citation: Vithlani J, Hawksworth C, Elvidge J, Ayiku L and Dawoud D (2023) Economic evaluations of artificial intelligence-based healthcare interventions: a systematic literature review of best practices in their conduct and reporting. Front. Pharmacol. 14:1220950. doi: 10.3389/fphar.2023.1220950
Received: 11 May 2023; Accepted: 25 July 2023;
Published: 08 August 2023.
Edited by:
Mauro Tettamanti, Mario Negri Institute for Pharmacological Research (IRCCS), ItalyReviewed by:
Tanja Mueller, University of Strathclyde, United KingdomCopyright © 2023 Vithlani, Hawksworth, Elvidge, Ayiku and Dawoud. This is an open-access article distributed under the terms of the Creative Commons Attribution License (CC BY). The use, distribution or reproduction in other forums is permitted, provided the original author(s) and the copyright owner(s) are credited and that the original publication in this journal is cited, in accordance with accepted academic practice. No use, distribution or reproduction is permitted which does not comply with these terms.
*Correspondence: Claire Hawksworth, Y2xhaXJlLmhhd2tzd29ydGhAbmljZS5vcmcudWs=
†These authors have contributed equally to this work
Disclaimer: All claims expressed in this article are solely those of the authors and do not necessarily represent those of their affiliated organizations, or those of the publisher, the editors and the reviewers. Any product that may be evaluated in this article or claim that may be made by its manufacturer is not guaranteed or endorsed by the publisher.
Research integrity at Frontiers
Learn more about the work of our research integrity team to safeguard the quality of each article we publish.