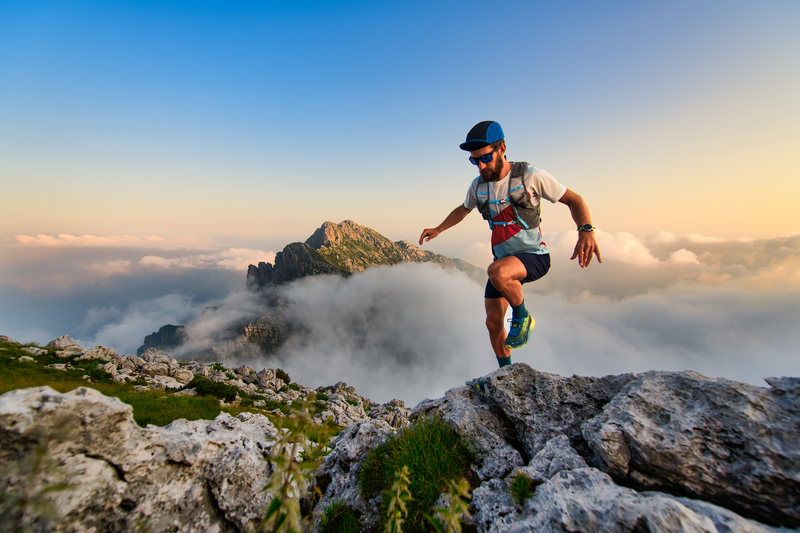
94% of researchers rate our articles as excellent or good
Learn more about the work of our research integrity team to safeguard the quality of each article we publish.
Find out more
ORIGINAL RESEARCH article
Front. Pharmacol. , 29 June 2023
Sec. Ethnopharmacology
Volume 14 - 2023 | https://doi.org/10.3389/fphar.2023.1210667
This article is part of the Research Topic Real-World Evidence of Natural Products, Herbal Medicines, and Traditional Chinese Medicine Treatments View all 15 articles
Introduction: Type 2 diabetes (T2D) is a multifactorial complex chronic disease with a high prevalence worldwide, and Type 2 diabetes patients with different comorbidities often present multiple phenotypes in the clinic. Thus, there is a pressing need to improve understanding of the complexity of the clinical Type 2 diabetes population to help identify more accurate disease subtypes for personalized treatment.
Methods: Here, utilizing the traditional Chinese medicine (TCM) clinical electronic medical records (EMRs) of 2137 Type 2 diabetes inpatients, we followed a heterogeneous medical record network (HEMnet) framework to construct heterogeneous medical record networks by integrating the clinical features from the electronic medical records, molecular interaction networks and domain knowledge.
Results: Of the 2137 Type 2 diabetes patients, 1347 were male (63.03%), and 790 were female (36.97%). Using the HEMnet method, we obtained eight non-overlapping patient subgroups. For example, in H3, Poria, Astragali Radix, Glycyrrhizae Radix et Rhizoma, Cinnamomi Ramulus, and Liriopes Radix were identified as significant botanical drugs. Cardiovascular diseases (CVDs) were found to be significant comorbidities. Furthermore, enrichment analysis showed that there were six overlapping pathways and eight overlapping Gene Ontology terms among the herbs, comorbidities, and Type 2 diabetes in H3.
Discussion: Our results demonstrate that identification of the Type 2 diabetes subgroup based on the HEMnet method can provide important guidance for the clinical use of herbal prescriptions and that this method can be used for other complex diseases.
Type 2 diabetes (T2D) is the most common type of diabetes and accounts for approximately 90% of all diabetes cases worldwide; T2D is a complex, serious and multifactorial chronic disease that has become an increasingly prevalent health issue and imposes a tremendous economic burden worldwide (Li et al., 2015; International Diabetes Federation IDF, 2019). People with T2D have an approximately 15% higher overall excess mortality risk than people who do not have T2D (Tancredi et al., 2015). Although T2D is defined by a single metabolite, glucose, it is increasingly recognized as a highly heterogeneous disease with varying clinical manifestations (Gregg et al., 2014; World Health Organization, 2019a; Ahlqvist et al., 2021). Therefore, identifying the precise subtypes of T2D patients would be important for preventing serious complications, predicting individualized drug responses and improving health outcomes for patients with diabetes in the early stage and help predict the drug responses of patients with diabetes (Pigeyre et al., 2022; Williams et al., 2022).
Precision medicine has been recognized as a new medical approach for refining the disease taxonomy and improving the healthcare capability (National Research Council US, 2011; Zhou et al., 2018). Recently, several studies have identified new subtypes of T2D through data-driven analysis of a clinical population, which has improved the understanding of T2D with the goal of improving patient care in clinical settings (Li et al., 2015; Ahlqvist et al., 2018). These studies suggested that there are opportunities to further refine the current definition of T2D in real-world clinical settings into additional subtypes (American Diabetes Association, 2010). Traditional Chinese medicine (TCM) is a typical kind of personalized medicine (Jiang et al., 2012; Zhou et al., 2014) that classifies disease conditions into different subtypes (i.e., syndromes) through the comprehensive analysis of symptom phenotypes identified by the four main diagnostic TCM procedures (observation, listening, questioning, and pulse analyses). Furthermore, individualized treatment (in most cases, with herbal prescriptions) would be ordered for patients according to the diagnosis of syndromes. This clinical framework presents a novel view of disease conditions from symptom profiles and herbal prescriptions for patients.
In this study, we collected large-scale real-world TCM clinical data on T2D and used an established heterogeneous medical record network (HEMnet) (Edward et al., 2017) method to identify the clinical subgroups of T2D. Four types of clinical features, namely, symptom phenotypes, syndrome diagnoses, herbal prescriptions and comorbid disease conditions, together with phenotype–genotype associations and botanical drug -efficacy relationships, were incorporated into the HEMnet approach to help identify clinical groups with both clinical meaningfulness and biological insights. Enrichment analysis was used to identify the significant features of the clinical characteristics and molecular pathways of the T2D patient groups. Our findings are expected to help refine the understanding of T2D by both improving personalized treatment and identifying the underlying mechanisms.
The data of 2137 inpatients diagnosed with T2D were collected from the EMR database of the Second Affiliated Hospital of Shandong University of TCM from 2016 to 2021, which included all inpatient information obtained during hospitalization, such as demographic information, symptoms, laboratory or physical tests, diagnoses and treatment. Because most data were in free text that cannot be used directly for analysis, we used a clinical information extraction tool (Shu et al., 2019) to efficiently extract the biomedical entities (e.g., symptoms, diseases) from these records. Then, to normalize the various clinical term descriptions, we manually checked and standardized the terms “disease”, “botanical drug” and “drug” by referring to the 10th Revision of International Classification of Diseases (ICD-10) (World Health Organization, 2019b), the Pharmacopoeia of the People’s Republic of China 2020 Revision (ChP 2020) (Chinese Pharmacopoeia Commission, 2020), and DrugBank Online (Wishart et al., 2018), respectively. In addition, diseases with detailed ICD-10 codes were further aggregated into higher level codes. For example, the ICD-10 codes I50.903 and I50.905 were aggregated into ICD-10 code I50.9.
In this study, several external data sources were used to support this research. The efficacy of botanical drugs was extracted from ChP 2020, and human protein‒protein interactions (PPIs) were obtained from the STRING database (Szklarczyk et al., 2019). The phenotype–genotype and botanical drug–target associations were extracted from the SymMap database (Wu et al., 2019). The disease–gene associations were extracted from the MalaCards database (Rappaport et al., 2017).
Missing data and semantic mismatch were the two main challenges of EMR analysis. Therefore, we used HEMnet to address the challenges of EMR analysis by leveraging information from several external sources to supplement clinical data (Edward et al., 2017). In our study, we utilized three distinct categories of edges to create the HEMnet (Figure 1). The first two categories PPI and phenotype–genotype were drawn from the external database, while the last category was drawn directly from the EMRs.
1) PPI. This network was based on HumanNet, an external network of protein-encoding genes (Lee et al., 2011). The nodes are proteins, and the undirected edges are the interactions between proteins.
2) Phenotype–genotype associations. This network was obtained from SymMap. The nodes were phenotype or genotype, and the undirected edges were the association of the phenotype and genotype.
3) Co-occurrence of clinical entities from the EMR. We directly added the clinical cooccurrence edges of botanical drugs from each medical record. The missing data was one of the main challenges of electronic medical records (EMR) analysis, especially the lack of symptom information. Botanical drugs can represent symptom precision to address missing symptom information in EMR. We repeated this for all clinical features in each patient’s medical record.
Then, HEMnet uses an embedding method, ProSNet (Wang et al., 2017), to infer relationships among its constituent nodes. ProSNet takes a heterogeneous network as input, on which it performs a novel dimensionality reduction algorithm to optimize a low-dimensional vector representation for each node. The vectors of two nodes are colocalized in the low-dimensional space if the nodes are close to each other in the heterogeneous network. After generating low-dimensional vector representations of nodes in the HEMnet, a similarity matrix was constructed according to the similarity between every two embedding vector features, which was calculated by cosine similarity. Finally, the similarity matrix was used to fill in missing features of the original patient characteristics and form the phenotypes of patients (Edward et al., 2017).
The K-means clustering (MacQueen, 1967) was used for the patient phenotype. According to the outcomes, the patients were divided into eight non-overlapping subgroups. The t-distributed stochastic neighbour embedding (t-SNE) algorithm (Cieslak et al., 2020) was used to visualize the outcomes.
The chi-square test and relative risk (RR) (Pirhaji et al., 2008; Ouimet et al., 2010) were used to assess the significance of clinical features, including symptom phenotypes, syndrome diagnoses, botanical drugs and comorbidities in eight subgroups. In this study, patients with a certain clinical feature, such as a symptom phenotype, in a particular subgroup as an exposed group, and the remaining patients with this certain clinical feature as the non-exposed group. So RR is defined as RR = (Cij/Ci)/((Cj-Cij)/(N-Ci)), where Ci is the number of patients in subgroup i, Cj is the number of patients with a clinical feature j, Cij represents the number of patients in subgroup i and with a clinical feature j and N is the total number of patients in the study. A p-value <0.05, which was obtained from the chi-square test, and an RR > 1 indicated that a clinical feature was truly significant.
The GO and KEGG pathway enrichment analysis are useful to trackle the DNA-related and protein-related problems. And they offers considerable power for discovering the biological functions of genes and proteins (Chen et al., 2017). The Gene Ontology (GO) project serves as a comprehensive source for functional genomics. The project creates evidence-supported annotations to describe the biological roles of individual genome products (e.g., genes, proteins, ncRNAs, complexes) (Gene Ontology Consortium, 2015). The KEGG pathway database is the main database in Kyoto Encyclopedia of Genes and Genomes (KEGG), and it consists of manually drawn reference pathway maps together with organism-specific pathway maps (Kanehisa et al., 2017). We obtained enriched GO and KEGG pathways using the Database for Annotation, Visualization, and Integrated Discovery (DAVID), which is a web-based online bioinformatics resource that aims to provide tools for the functional interpretation of large lists of genes/proteins (Sherman et al., 2022).
As shown in the table below (Table 1), of the 2137 T2D patients, 1347 (63.03%) were male, and 790 (36.97%) were female. The ages of most T2D patients (60.60%) were between 60 and 79 years old. The average length of stay (LOS) was 14.08 ± 9.20, and for most patients (41.83%), LOS was between 8 and 14 days. We counted the distinct number of comorbidities of each patient and found that most patients had 6–10 diagnoses (56.43%).
Then, we analysed the distribution of the top five clinical features including symptom phenotypes, syndrome diagnoses, botanical drugs, and comorbidities (Table 2).
With the method introduced in the Materials and Methods, we utilized three distinct categories of edges to create the HEMnet, which contained 5,846 nodes and 125,426 connected edges. There were 3,000 symptom nodes and 2,846 gene nodes. Furthermore, there were 16,641 PPI edges, 8,749 phenotype–genotype edges, and 100,036 symptom edges.
Then, the embedding method ProSNet was used to generate low-dimensional vector representations of nodes in the HEMnet. A similarity matrix was constructed according to the similarity between every two embedding vector features, which was calculated by cosine similarity, and used to fill in missing features of the original patient characteristics to form the patient phenotypes. Finally, using the K-means clustering algorithm, eight non-overlapping patient subgroups were obtained. The t-SNE algorithm was used to visualize the clustering results (Figure 2). The numbers of patients in the eight subgroups were as follows (Table 3): H1 (n = 547, 25.60%), H2 (n = 501, 23.44%), H3 (n = 432, 20.22%), H4 (n = 298, 13.94%), H5 (n = 197, 9.22%), H6 (n = 132, 6.18%), H7 (n = 18, 0.84%), and H8 (n = 12, 0.56%).
FIGURE 2. The visualized clustering result of HEMnet. The correspondence between the C_0-C_7 clusters in the figure and the H1-H8 subgroups in this paper is as follows: C_0 = H5, C_1 = H2, C_2 = H1, C_3 = H6, C_4 = H4, C_5 = H8, C_6 = H7, C_7 = H3. This picture was to reduce the dimensionality of the patient’s characterization vector to a two-dimensional vector for display. So the x-axis and y-axis represent the patient’s characterization vector, and the closer the two points are, the closer the patient’s characteristics are.
We then selected the top 10 clinical features in these modules according to their frequency in each subgroup. Then, the RR and chi-square test (RR > 1 and p < 0.05, see Materials and methods) were used to screen the significant clinical features.
Because of fewer patients in H7 and H8 subgroups, it was less meaningful to analyse them. And since this study focused on the precision treatment of comorbidities, the H1, H2, and H4 subgroups with no significant botanical drugs and the H5 subgroup with a lower frequency of botanical drug use were excluded according to the screening results. Finally, H3 and H6 were included for further analysis.
We present the statistically significant botanical drugs, comorbidities, syndromes, and symptoms in H3 and H6 (Table 4, Table 5, Table 6, and Table 7), Poria, Astragali Radix, Glycyrrhizae Radix et Rhizoma, Cinnamomi Ramulus, and Ophiopogonis radix were the significant botanical drugs. Essential (primary) hypertension, atherosclerotic heart disease, heart failure, unstable angina, etc., were the significant comorbidities. Qi-Yin deficiency was the main significant syndrome. And chest tightness, fever, coarse lung breathing, vomiting, expectoration, etc., were the significant symptoms. In H6, Chuanxiong Rhizoma, Gastrodiae Rhizoma, and Baked Ziziphi Spinosae Semen were the significant botanical drugs. Cerebral infarction, sequelae of cerebral infarction and sequelae of intracerebral haemorrhage were the significant comorbidities. Deficient qi and blood stasis was the main significant syndrome. And poor physical activity, fever, slurring of speech, vomiting, etc., were the significant symptoms.
In this part, we explored the shared molecular associations between the significant botanical drugs and comorbidities of T2D in H3 and H6. First, we identified the distinct genes associated with each significant botanical drug and comorbidity in H3 and H6 from an external database (see Materials and methods). Then, we obtained the pathways and GO terms for the botanical drugs, comorbidities and T2D in H3 and H6 by the DAVID program (2021, see Materials and methods). Finally, we screened out pathways and GO terms with p < 0.05 from botanical drugs, comorbidities and T2D. We identified the overlapping pathways and GO terms among the botanical drugs, comorbidities, and T2D in H3 and H6 (Table 8 and Table 9). In H3, there were six overlapping pathways and eight overlapping GO terms among the botanical drugs, comorbidities, and T2D. In H6, there were no overlapping pathways among the botanical drugs, comorbidities, and T2D. Therefore, we reported on the pathways that overlapped between the two of them. There was only one overlapping GO term among the botanical drugs, comorbidities, and T2D. For example, most of the pathways and GO functions in H3 were associated with T2D, such as type II diabetes mellitus, insulin resistance, glucose metabolic process, and response to glucose. The significant botanical drugs in H3 had some overlapping pathways and GO terms with comorbidities and T2D.
In recent years, the continual growth of EMR databases has facilitated clinical research, paved the way for data mining applications, and supported population health. However, missing data is the biggest barrier to using EMRs (Kruse et al., 2018). In our study, the problem of missing data and semantic mismatch in EMRs posed a considerable challenge. For example, if T2D was not the primary diagnosis, the patient’s T2D-related symptoms would not be recorded in the medical record, which results in incomplete information in the patient’s medical record. Furthermore, the overabundant expression of symptoms, diagnoses, botanical drugs, and syndromes in clinical TCM data leads to mismatched records containing semantically similar but lexically distinct terms. Therefore, the problem of missing data and semantic mismatch were solved by standardizing the data and creating the HEMnet to ensure the reliability of the research results (Edward et al., 2017).
Analysing disease comorbidities with EMR data has become popular in real-world clinical settings for chronic disease conditions such as T2D and chronic liver diseases (Li et al., 2015; Ahlqvist et al., 2018; Shu et al., 2019; Mansour Aly et al., 2021). In this manuscript, the HEMnet method was used to identify the eight non-overlapping patient subgroups. Then, H3 and H6 were screened according to a specific screening strategy for subgroups to further analyse the clinical features. For example, cardiovascular disease (CVD), such as atherosclerotic heart disease, heart failure, unstable angina, cardiac arrhythmia, atrial fibrillation and flutter, was a significant comorbidity of T2D in H3. In large prospective trials, T2D has been identified as a significant risk factor for CVD, including stroke, angina, heart failure, myocardial infarction, and atherosclerosis (Emerging Risk Factors Collaboration Sarwar et al., 2010; Peters et al., 2014; Shah et al., 2015; Einarson et al., 2018). Regarding treatment, Poria, Astragali radix, Glycyrrhizae radix et rhizoma, Cinnamomi ramulus, and Ophiopogonis radix were the significant botanical drugs in H3. And studies have shown that these botanical drugs used alone or in combination with other botanical drugs are often used to treat diabetes as well as other disorders (Jia et al., 2003; Li et al., 2004; Lindequist et al., 2005).
Furthermore, to explore the shared molecular associations among the significant botanical drugs, comorbidities and T2D in H3 and H6, we explored the overlapping pathways and GO terms between the significant botanical drugs and comorbidities of T2D in H3 and H6. The significant botanical drugs in H3 had six pathways and eight GO terms that overlapped between comorbidities and T2D. This result indicated that these botanical drugs may have therapeutic effects on comorbidities and T2D via the pathways and GO terms identified in the analysis. For example, the overlapping pathways in H3 inculded insulin resistance which is one shared defect in T2D and Essential (primary) Hypertension. Although the mechanisms by which defective insulin action per se contributes to high blood pressure are still somewhat uncertain (Ferrannini and Cushman, 2012). But previous studies have demonstrated that within the physiological concentration range of insulin, it causes slight increases in limb blood flow by enhancing the release of nitric oxide (via stimulation of nitric oxide synthase activity in endothelial cells) and by potentiating acetylcholine-induced vasodilation. In people with insulin resistance, vasodilation in response to supraphysiological insulin concentrations is reduced (Taddei et al., 1995; Yki-Järvinen and Utriainen, 1998; Steinberg and Baron, 2002; Giacco and Brownlee, 2010). Astragaloside Ⅳ (AST Ⅳ, chemical formula: C41H68O14, molecular weight:785), as the primary active ingredient of Astragali radix, has the pharmacological effects of regulating lipid and carbohydrate metabolism and improving insulin resistance. Previous studies have shown that AST Ⅳ improvement of insulin resistance may be related to activation of the IRS1/protein kinase B (AKT) insulin signaling pathway to increase the glucose transporter type 4 (GLUT4) activity, thus increasing glucose uptake and insulin sensitivity (Zhou et al., 2021). So the main findings of the GO and KEGG pathway enrichment analysis require further experimental verification.
Our study has several potential limitations. Our sample included only 2137 hospitalized patients, resulting in an insufficient number of patients with some subtypes of T2D for identification of additional significant TCM phenotypes. In future studies, more patients should be included to ensure the abundance of the results. Another limitation is that Western medicine and laboratory tests were not included in our study. Therefore, the resulting disease subtypes would incorporate little information on these features. In addition, some patients were not given herbal prescriptions. This might affect the results of data mining. Finally, we used EMRs from only one hospital, and the resulting patient subgroups that were identified may not be representative. And further experiments should be performed to verify the results of this paper (Sheng et al., 2021).
Our results demonstrate that Cardiovascular disease (CVD) and Qi-Yin deficiency syndrome were significant comorbidity and TCM syndrome of T2D in subgroup H3, respectively. Regarding treatment, Poria, Astragali radix, Glycyrrhizae radix et rhizoma, Cinnamomi ramulus, and Ophiopogonis radix were the significant botanical drugs in subgroup H3. In subgroup H6, cerebral infarction and its sequelae, Qi deficiency and blood stasis syndrome were significant comorbidities and TCM syndrome, respectively. Regarding treatment, Chuanxiong rhizoma, Gastrodiae rhizoma, and Baked ziziphi spinosae semen were the significant botanical drugs. So identification of the T2D subgroup based on the HEMnet method can provide important guidance for the clinical use of herbal prescriptions and that this method can be used for other complex diseases.
The raw dataset obtained from the electronic medical record of the hospital presented in this article is not available because of local legislation and institutional requirements. Requests to access the datasets should be directed to the corresponding author. The external datasets, such as human protein-protein interactions, phenotype-genotype, and botanical drug-target associations supporting the conclusions of this article will be made available by the authors, without undue reservation.
Ethical review and approval was not required for the study on human participants in accordance with the local legislation and institutional requirements. Written informed consent for participation was not required for this study in accordance with the national legislation and the institutional requirements.
SZ was in charge of writing and revising the paper. HL was responsible for the data analysis and data mining of the paper. XJ was in charge of polishing the paper. XZ extracted the external data sources, such as the efficacy of herbs, human protein-protein interactions, and phenotype-genotype. RL, YL, CL, JC, and GL structured the text and extracted biomedical entities from electronic medical records of traditional Chinese medicine. WZ, QL, LW, XW, and YS standardized the data of symptoms, herbs, syndromes, and diseases. SW and YX were responsible for the design of the paper. All authors contributed to the article and approved the submitted version.
This work is partially supported by the National Key Research and Development Program of China (Nos 2018YFC1704100, 2018YFC1704103).
Thanks to the staff of the hospital information department of the Second Affiliated Hospital of Shandong University of TCM for their data and technical support.
The authors declare that the research was conducted in the absence of any commercial or financial relationships that could be construed as a potential conflict of interest.
The reviewer TW declared a shared parent affiliation with the authors XZ, SW to the handling editor at the time of review.
All claims expressed in this article are solely those of the authors and do not necessarily represent those of their affiliated organizations, or those of the publisher, the editors and the reviewers. Any product that may be evaluated in this article, or claim that may be made by its manufacturer, is not guaranteed or endorsed by the publisher.
The Supplementary Material for this article can be found online at: https://www.frontiersin.org/articles/10.3389/fphar.2023.1210667/full#supplementary-material
Ahlqvist, E., Prasad, R. B., and Groop, L. (2021). 100 years of insulin: Towards improved precision and a new classification of diabetes mellitus. J. Endocrinol. 252 (3), R59–R70. doi:10.1530/JOE-20-0596
Ahlqvist, E., Storm, P., Käräjämäki, A., Martinell, M., Dorkhan, M., Carlsson, A., et al. (2018). Novel subgroups of adult-onset diabetes and their association with outcomes: A data-driven cluster analysis of six variables. Lancet Diabetes Endocrinol. 6 (5), 361–369. doi:10.1016/S2213-8587(18)30051-2
American Diabetes Association (2010). Diagnosis and classification of diabetes mellitus. Diabetes Care 33 (1), S62–S69. doi:10.2337/dc10-S062
Chen, L., Zhang, Y. H., Wang, S., Huang, T., and Cai, Y. D. (2017). Prediction and analysis of essential genes using the enrichments of gene ontology and KEGG pathways. PLoS One 12 (9), 0184129. doi:10.1371/journal.pone.0184129
Chinese Pharmacopoeia Commission (2020). Pharmacopoeia of the People’s Republic of China 2020 revision. Beijing: China Medical Science and Technology Press.
Cieslak, M. C., Castelfranco, A. M., Roncalli, V., Lenz, P. H., and Hartline, D. K. (2020). t-Distributed Stochastic Neighbor Embedding (t-SNE): A tool for eco-physiological transcriptomic analysis. Mar. Genomics 51, 100723. doi:10.1016/j.margen.2019.100723
Edward, W. H., Sheng, W., and Bingxue, L. (2017). “HEMnet: Integration of electronic medical records with molecular interaction networks and domain knowledge for survival analysis,” in Proceedings of the 8th ACM international conference on bioinformatics, computational biology, and health informatics (ACM-BCB '17) (New York, NY, USA: Association for Computing Machinery), 378–387.
Einarson, T. R., Acs, A., Ludwig, C., and Panton, U. H. (2018). Economic burden of cardiovascular disease in type 2 diabetes: A systematic review. Value Health 21 (7), 881–890. doi:10.1016/j.jval.2017.12.019
Ferrannini, E., and Cushman, W. C. (2012). Diabetes and hypertension: The bad companions. Lancet 380 (9841), 601–610. doi:10.1016/S0140-6736(12)60987-8
Gene Ontology Consortium (2015). Gene ontology Consortium: Going forward. Nucleic Acids Res. 43, D1049–D1056. doi:10.1093/nar/gku1179
Giacco, F., and Brownlee, M. (2010). Oxidative stress and diabetic complications. Circ. Res. 107 (9), 1058–1070. doi:10.1161/CIRCRESAHA.110.223545
Gregg, E. W., Li, Y., Wang, J., Burrows, N. R., Ali, M. K., Rolka, D., et al. (2014). Changes in diabetes-related complications in the United States, 1990-2010. N. Engl. J. Med. 370 (16), 1514–1523. doi:10.1056/NEJMoa1310799
International Diabetes Federation (IDF) (2019). IDF diabetes atlas. 9th edn. Brussels, Belgium: International Diabetes Federation. Available at: https://www.diabetesatlas.org.
Jia, W., Gao, W., and Tang, L. (2003). Antidiabetic herbal drugs officially approved in China. Phytother. Res. 17 (10), 1127–1134. doi:10.1002/ptr.1398
Jiang, M., Lu, C., Zhang, C., Yang, J., Tan, Y., Lu, A., et al. (2012). Syndrome differentiation in modern research of traditional Chinese medicine. J. Ethnopharmacol. 140 (3), 634–642. doi:10.1016/j.jep.2012.01.033
Kanehisa, M., Furumichi, M., Tanabe, M., Sato, Y., and Morishima, K. (2017). Kegg: New perspectives on genomes, pathways, diseases and drugs. Nucleic Acids Res. 45 (D1), D353–D361. doi:10.1093/nar/gkw1092
Kruse, C. S., Stein, A., Thomas, H., and Kaur, H. (2018). The use of electronic health records to support population health: A systematic review of the literature. J. Med. Syst. 42 (11), 214. doi:10.1007/s10916-018-1075-6
Lee, I., Blom, U. M., Wang, P. I., Shim, J. E., and Marcotte, E. M. (2011). Prioritizing candidate disease genes by network-based boosting of genome-wide association data. Genome Res. 21 (7), 1109–1121. doi:10.1101/gr.118992.110
Li, L., Cheng, W. Y., Glicksberg, B. S., Gottesman, O., Tamler, R., Chen, R., et al. (2015). Identification of type 2 diabetes subgroups through topological analysis of patient similarity. Sci. Transl. Med. 7 (311), 311ra174. doi:10.1126/scitranslmed.aaa9364
Li, W. L., Zheng, H. C., Bukuru, J., and De Kimpe, N. (2004). Natural medicines used in the traditional Chinese medical system for therapy of diabetes mellitus. J. Ethnopharmacol. 92 (1), 1–21. doi:10.1016/j.jep.2003.12.031
Lindequist, U., Niedermeyer, T. H., and Jülich, W. D. (2005). The pharmacological potential of mushrooms. Evid. Based Complement. Altern. Med. 2 (3), 285–299. doi:10.1093/ecam/neh107
MacQueen, J. (1967). “Some methods for classification and analysis of multivariate observations,” in Proceedings of the fifth Berkeley symposium on mathematical statistics and probability (Berkeley: University of California Press).
Mansour Aly, D., Dwivedi, O. P., Prasad, R. B., Käräjämäki, A., Hjort, R., Thangam, M., et al. (2021). Genome-wide association analyses highlight etiological differences underlying newly defined subtypes of diabetes. Nat. Genet. 53 (11), 1534–1542. doi:10.1038/s41588-021-00948-2
National Research Council (US) (2011). Toward precision medicine: Building a knowledge network for biomedical research and a new taxonomy of disease. Washington (DC): National Academies Press US. doi:10.17226/13284Committee on A Framework for developing a new taxonomy of disease
Ouimet, M. C., Simons-Morton, B. G., Zador, P. L., Lerner, N. D., Freedman, M., Duncan, G. D., et al. (2010). Using the U.S. National Household Travel Survey to estimate the impact of passenger characteristics on young drivers' relative risk of fatal crash involvement. Accid. Anal. Prev. 42 (2), 689–694. doi:10.1016/j.aap.2009.10.017
Peters, S. A., Huxley, R. R., and Woodward, M. (2014). Diabetes as risk factor for incident coronary heart disease in women compared with men: A systematic review and meta-analysis of 64 cohorts including 858,507 individuals and 28,203 coronary events. Diabetologia 57 (8), 1542–1551. doi:10.1007/s00125-014-3260-6
Pigeyre, M., Hess, S., Gomez, M. F., Asplund, O., Groop, L., Paré, G., et al. (2022). Validation of the classification for type 2 diabetes into five subgroups: A report from the ORIGIN trial. Diabetologia 65 (1), 206–215. doi:10.1007/s00125-021-05567-4
Pirhaji, L., Kargar, M., Sheari, A., Poormohammadi, H., Sadeghi, M., Pezeshk, H., et al. (2008). The performances of the chi-square test and complexity measures for signal recognition in biological sequences. J. Theor. Biol. 251 (2), 380–387. doi:10.1016/j.jtbi.2007.11.021
Rappaport, N., Twik, M., Plaschkes, I., Nudel, R., Iny Stein, T., Levitt, J., et al. (2017). MalaCards: An amalgamated human disease compendium with diverse clinical and genetic annotation and structured search. Nucleic Acids Res. 45 (D1), D877–D887. doi:10.1093/nar/gkw1012
Emerging Risk Factors Collaboration Sarwar, N., Gao, P., Seshasai, S. R. K., Gobin, R., and Kaptoge, S. (2010). Diabetes mellitus, fasting blood glucose concentration, and risk of vascular disease: A collaborative meta-analysis of 102 prospective studies. Lancet 375 (9733), 2215–2222. doi:10.1016/S0140-6736(10)60484-9
Shah, A. D., Langenberg, C., Rapsomaniki, E., Denaxas, S., Pujades-Rodriguez, M., Gale, C. P., et al. (2015). Type 2 diabetes and incidence of cardiovascular diseases: A cohort study in 1·9 million people. Lancet Diabetes Endocrinol. 3 (2), 105–113. doi:10.1016/S2213-8587(14)70219-0
Sheng, S., Yang, Q. N., Zhu, H. N., and Xian, Y. Y. (2021). Network pharmacology-based exploration of the mechanism of Guanxinning tablet for the treatment of stable coronary artery disease. World J. Tradit. Chin. Med. 7, 456–466. doi:10.4103/wjtcm.wjtcm_25_21
Sherman, B. T., Hao, M., Qiu, J., Jiao, X., Baseler, M. W., Lane, H. C., et al. (2022). David: A web server for functional enrichment analysis and functional annotation of gene lists (2021 update). Nucleic Acids Res. 50 (1), W216–W221. doi:10.1093/nar/gkac194
Shu, Z., Liu, W., Wu, H., Xiao, M., Wu, D., Cao, T., et al. (2019). Symptom-based network classification identifies distinct clinical subgroups of liver diseases with common molecular pathways. Comput. Methods Programs Biomed. 174, 41–50. doi:10.1016/j.cmpb.2018.02.014
Steinberg, H. O., and Baron, A. D. (2002). Vascular function, insulin resistance and fatty acids. Diabetologia 45 (5), 623–634. doi:10.1007/s00125-002-0800-2
Szklarczyk, D., Gable, A. L., Lyon, D., Junge, A., Wyder, S., Huerta-Cepas, J., et al. (2019). STRING v11: Protein-protein association networks with increased coverage, supporting functional discovery in genome-wide experimental datasets. Nucleic Acids Res. 47 (D1), D607–D613. doi:10.1093/nar/gky1131
Taddei, S., Virdis, A., Mattei, P., Natali, A., Ferrannini, E., and Salvetti, A. (1995). Effect of insulin on acetylcholine-induced vasodilation in normotensive subjects and patients with essential hypertension. Circulation 92 (10), 2911–2918. doi:10.1161/01.cir.92.10.2911
Tancredi, M., Rosengren, A., Svensson, A. M., Kosiborod, M., Pivodic, A., Gudbjörnsdottir, S., et al. (2015). Excess mortality among persons with type 2 diabetes. N. Engl. J. Med. 373, 1720–1732. doi:10.1056/NEJMoa1504347
Wang, S., Qu, M., and Peng, J. (2017). ProSNet: Integrating homology with molecular networks for protein function prediction. Pac Symp. Biocomput 22, 27–38. doi:10.1142/9789813207813_0004
Williams, D. M., Jones, H., and Stephens, J. W. (2022). Personalized type 2 diabetes management: An update on recent advances and recommendations. Diabetes Metab. Syndr. Obes. 15, 281–295. doi:10.2147/DMSO.S331654
Wishart, D. S., Feunang, Y. D., Guo, A. C., Lo, E. J., Marcu, A., Grant, J. R., et al. (2018). DrugBank 5.0: A major update to the DrugBank database for 2018. Nucleic Acids Res. 46 (D1), D1074–D1082. doi:10.1093/nar/gkx1037
World Health Organization (2019a). Classification of diabetes mellitus. Geneva: World Health Organization. Available at: https://apps.who.int/iris/handle/10665/325182.
World Health Organization (2019b). Tenth revision of international classification of diseases (ICD-10). Geneva: World Health Organization. Avaliable At: https://icd.who.int/browse10/2019/en.
Wu, Y., Zhang, F., Yang, K., Fang, S., Bu, D., Li, H., et al. (2019). SymMap: An integrative database of traditional Chinese medicine enhanced by symptom mapping. Nucleic Acids Res. 47 (D1), D1110–D1117. doi:10.1093/nar/gky1021
Yki-Järvinen, H., and Utriainen, T. (1998). Insulin-induced vasodilatation: Physiology or pharmacology? Diabetologia 41 (4), 369–379. doi:10.1007/s001250050919
Zhou, X., Lei, L., Liu, J., Halu, A., Zhang, Y., Li, B., et al. (2018). A systems approach to refine disease taxonomy by integrating phenotypic and molecular networks. EBioMedicine 31, 79–91. doi:10.1016/j.ebiom.2018.04.002
Zhou, X., Li, Y., Peng, Y., Hu, J., and Zhang, R. (2014). Clinical phenotype network: The underlying mechanism for personalized diagnosis and treatment of traditional Chinese medicine. Front. Med. 8 (3), 337–346. doi:10.1007/s11684-014-0349-8
Zhou, X., Wang, L. L., Tang, W. J., and Tang, B. (2021). Astragaloside IV inhibits protein tyrosine phosphatase 1B and improves insulin resistance in insulin-resistant HepG2 cells and triglyceride accumulation in oleic acid (OA)-treated HepG2 cells. J. Ethnopharmacol. 268, 113556. doi:10.1016/j.jep.2020.113556
Keywords: type 2 dabetes, real-world clinical data, heterogeneous medical record network method, traditional chinese medcine, enrichment analysis
Citation: Zhao S, Li H, Jing X, Zhang X, Li R, Li Y, Liu C, Chen J, Li G, Zheng W, Li Q, Wang X, Wang L, Sun Y, Xu Y and Wang S (2023) Identifying subgroups of patients with type 2 diabetes based on real-world traditional chinese medicine electronic medical records. Front. Pharmacol. 14:1210667. doi: 10.3389/fphar.2023.1210667
Received: 23 April 2023; Accepted: 15 June 2023;
Published: 29 June 2023.
Edited by:
Xuezhong Zhou, Beijing Jiaotong University, ChinaReviewed by:
Tiancai Wen, China Academy of Chinese Medical Sciences, ChinaCopyright © 2023 Zhao, Li, Jing, Zhang, Li, Li, Liu, Chen, Li, Zheng, Li, Wang, Wang, Sun, Xu and Wang. This is an open-access article distributed under the terms of the Creative Commons Attribution License (CC BY). The use, distribution or reproduction in other forums is permitted, provided the original author(s) and the copyright owner(s) are credited and that the original publication in this journal is cited, in accordance with accepted academic practice. No use, distribution or reproduction is permitted which does not comply with these terms.
*Correspondence: Yunsheng Xu, eHlzNjVAMTI2LmNvbQ==; Shihua Wang, NDkyNTY0MTE2QHFxLmNvbQ==
†These authors have contributed equally to this work
Disclaimer: All claims expressed in this article are solely those of the authors and do not necessarily represent those of their affiliated organizations, or those of the publisher, the editors and the reviewers. Any product that may be evaluated in this article or claim that may be made by its manufacturer is not guaranteed or endorsed by the publisher.
Research integrity at Frontiers
Learn more about the work of our research integrity team to safeguard the quality of each article we publish.