Commentary: The pharmacogenomic landscape of an Indigenous Australian population
- 1Centre for Genomics and Personalised Health, Queensland University of Technology, Brisbane, QLD, Australia
- 2Faculty of Medicine, University of Queensland, Brisbane, QLD, Australia
- 3John Curtin School of Medical Research, College of Health and Medicine, Australian National University, Canberra, ACT, Australia
- 4Department of Clinical Pharmacy and Toxicology, Leiden University Medical Center, Leiden, Netherlands
- 5Translational Research Institute, Queensland University of Technology, Brisbane, QLD, Australia
Background: Population genomic studies of individuals of Indigenous ancestry have been extremely limited comprising <0.5% of participants in international genetic databases and genome-wide association studies, contributing to a “genomic gap” that limits their access to personalised medicine. While Indigenous Australians face a high burden of chronic disease and associated medication exposure, corresponding genomic and drug safety datasets are sorely lacking.
Methods: To address this, we conducted a pharmacogenomic study of almost 500 individuals from a founder Indigenous Tiwi population. Whole genome sequencing was performed using short-read Illumina Novaseq6000 technology. We characterised the pharmacogenomics (PGx) landscape of this population by analysing sequencing results and associated pharmacological treatment data.
Results: We observed that every individual in the cohort carry at least one actionable genotype and 77% of them carry at least three clinically actionable genotypes across 19 pharmacogenes. Overall, 41% of the Tiwi cohort were predicted to exhibit impaired CYP2D6 metabolism, with this frequency being much higher than that for other global populations. Over half of the population predicted an impaired CYP2C9, CYP2C19, and CYP2B6 metabolism with implications for the processing of commonly used analgesics, statins, anticoagulants, antiretrovirals, antidepressants, and antipsychotics. Moreover, we identified 31 potentially actionable novel variants within Very Important Pharmacogenes (VIPs), five of which were common among the Tiwi. We further detected important clinical implications for the drugs involved with cancer pharmacogenomics such as thiopurines and tamoxifen, immunosuppressants like tacrolimus and certain antivirals used in the hepatitis C treatment due to potential differences in their metabolic processing.
Conclusion: The pharmacogenomic profiles generated in our study demonstrate the utility of pre-emptive PGx testing and have the potential to help guide the development and application of precision therapeutic strategies tailored to Tiwi Indigenous patients. Our research provides valuable insights on pre-emptive PGx testing and the feasibility of its use in ancestrally diverse populations, emphasizing the need for increased diversity and inclusivity in PGx investigations.
Introduction
Global estimates suggest that less than 0.5% of all participants within international genetic databases and genome-wide association studies (GWAS) are of Indigenous ancestry. As of 2018, 22% of genetic data in GWAS were derived from persons of non-European ancestry, while less than 4% were contributed by Africans, Latinos, and Indigenous individuals (Sirugo et al., 2019). In 2019, the overall Indigenous participation in GWAS cohorts was just ∼0.02%. A recent study by Hitchman et al. have reported a rare uncertain function allele CYP2D6*71, with a high prevalence of 8.9% among New Zealand Māori and Pacific peoples demonstrating the need for more genetic studies in distinct ethnic groups (Hitchman et al., 2022). The lack of reference variant data for these minority groups has hampered clinical variant analysis efforts focused on these populations, resulting in the imprecise interpretation of clinical results and thereby directly impacting the access of these populations to equitable health outcomes (Garrison et al., 2019).
Indigenous Australians represent the world’s longest continuously surviving cultural group, having inhabited the Australian continent for over 60,000 years. Their culturally and ancestrally diverse communities have descended from a single founding population roughly 10,000–32,000 years ago. There are about 600 distinct Indigenous Australian groups, each with their own culture, customs, language, and laws (Malaspinas et al., 2016). However, these Indigenous groups face disproportionately high burdens of disease and mortality compared to other Australians across all age groups. Non-communicable diseases such as chronic lung disease, cardiovascular disease, type-2 diabetes, chronic renal failure, cancer, and mental disorders are the major contributors to the high rates of mortality among adult members of the Indigenous population. The burden of chronic diseases in this population imposes extensive suffering, social and familial dislocation, and burdensome healthcare costs on these Indigenous patients and their communities (Thynne and Gabb, 2016). Tiwi people are an Indigenous group living on Bathurst and Melville Islands, close to Darwin in Australia. There are 2348 individuals live in Tiwi Islands in comparative isolation distinct from mainland Aboriginal people (Australian Bureau of Statistics, 2021) and represents a highly homogeneous population. In this study, we focus on the Pharmacogenomics (PGx) in this distinct Indigenous group.
Adverse drug reactions (ADRs), inadequate efficacy, and treatment failure are common, yet serious, consequences of trial-and-error prescription efforts globally. The likelihood of an unsuitable prescription may be >80% in some instances, with an up to 40% risk of an ADR (Cacabelos et al., 2019). ADRs have been attributed to increased morbidity, mortality, and additional costs that can affect overall patient wellbeing in addition to placing a significant burden on healthcare systems and the pharmaceutical industry. A prevalence of 6.5% has been reported for ADR-related hospital admissions, with a median bed-stay of up to 8 days and an estimated annual cost of USD 847 million for the National Health System in England (Pirmohamed et al., 2004). ADRs have also been reported as the fourth leading cause of death in the United States and Canada (Hacker, 2009). Furthermore, 462 drugs have been withdrawn from the market between 1953-2013 due to associated severe ADRs (Onakpoya et al., 2016). In Australia, nearly 1.7 million patients were prescribed a medication listed by the Clinical Pharmacogenetics Implementation Consortium (CPIC, 2022) in 2017, 40% of which were predicted to carry one or more PGx variants associated with the prescribed medication (Polasek et al., 2019). Moreover, 250,000 annual hospital admissions in Australia were medication related with an estimated cost of AUD 1.4 billion to the healthcare system, of which two-thirds were potentially preventable (Lim et al., 2022). The genetic profile of an individual is estimated to account for 30%–40% of their response to medications (Kozyra et al., 2017). Much like this inter-individual variability, significant differences in the distribution of variations and associated drug response outcomes across racially and ethnically diverse groups are well established (Zhou and Lauschke, 2021).
Genetic and clinical evidence to support inter-individual and inter-ethnic diversity in drug responses, and PGx has evolved as an effective tool to manage medication risk. Evidence-based guidelines for a total of 147 drugs are published by the CPIC, the Dutch Pharmacogenetics Working Group (DPWG) (KNMP Pharmacist organization Guidelines, 2022), and other professional societies. A total of 411 FDA approved drug label annotations are available for 367 unique drugs to assist in clinical decision-making (U. S. Food and Drug Administration, 2022). Myriad PGx information is easily accessible from resources including PharmGKB (PharmGKB, 2022) and PharmVar (PharmVar, 2022). However, PGx research and clinical trials are biased in their representation, focusing largely on populations of European ancestry and exhibiting inappropriate inclusion of other racial/ethnic groups. Therefore, generalizing the PGx findings of these studies in genetically distinct minority groups can be problematic (Thynne and Gabb, 2016). The Caucasian ethnicity is the most prevalent among the general Australian population and vary from Indigenous Australians. The AFs of CYP2D6, CYP2C9, CYP2C19, and VKORC1 in a cohort of Australian patients were consistent with the previously reported frequencies in Caucasians (Mostafa et al., 2019). Despite numerous attempts to characterize the unique PGx profiles of genetically distinct populations (Zhou and Lauschke, 2018; Gonzalez-Covarrubias et al., 2019; Sahana et al., 2022) their representation in PGx research remains limited. Indigenous Australians harbor genetic diversity seen in few other population groups. Although the drug safety information among these people are highly limited, previous evidence show that they carry a high ADR risk for certain medications. In one clinical case report, a 30-year-old Australian indigenous woman carrying a CYP2C9*3 poor metabolizer genotype and an HLA-B*56-02 positive genotype experienced a phenytoin-induced drug rash with eosinophilia and other systemic symptoms (Somogyi et al., 2019). Also, serious statin-associated toxicities have been reported among Indigenous Australians (Gabb et al., 2013), indicating the greater importance of pre-emptive testing for potential ADR risk in this patient population. However, Tiwi Indigenous people, like many other ethnicities within Australia and globally, are severely underrepresented in population genomic studies conducted to date, resulting in a significant “genomic gap” that limits their access to personalised medicine and equitable healthcare.
We previously conducted one of the earliest Indigenous Australian PGx studies evaluating pharmacological variability in this population (Jaya Shankar et al., 2022). Building on this recent report, we herein carried out a comprehensive PGx analysis of a founder Indigenous population in Australia, harboring a unique genetic architecture. Specifically, we analysed whole genome sequencing (WGS) data and associated pharmacological treatment data from 473 Tiwi individuals, including the previously analyzed 187 participants to identify key variants of pharmacological relevance enriched within the population that could potentially interfere with medication use in the context of chronic disease. This study provides a better understanding of the underlying genetic factors in the Tiwi population and highlights the critical need for robust strategies aimed at implementing pharmacovigilance to improve the health outcomes for members of this Indigenous community.
Materials and methods
Study population and datasets
The WGS data for this study were acquired from blood samples collected from 492 Tiwi individuals between 2013 and 2014 in a previous study conducted to investigate the prevalence of chronic kidney disease (CKD) in this population (Thomson et al., 2019). The associated meta-data included: lifestyle information, CKD status, comorbidities, medication, blood glucose, blood pressure, blood albumin, and creatinine. The cohort was sequenced in four batches using PCR-free sample preparation and Illumina Novaseq6000 paired-end sequencing with an average coverage 30x. After quality control (QC) and filtering, 459 samples were retained for further downstream analysis. The GRCh38 version of multi-sample VCFs from the 1000 Genome phase three project (1KGP) (n = 2504) (1000 Genomes Project Consortium et al., 2015) was used in the PCA analysis.
Indigenous community consultations
This project has been carried out in consultation and ongoing engagement with community experts, Indigenous Elders and lead Indigenous research experts. Guidelines developed to guide the ethical conduct of research with Indigenous peoples have been adhered to in all aspects (e.g., NHMRC). The core values of Spirit and Integrity, Cultural Continuity, Equity, Reciprocity, Respect and Responsibility have been embedded throughout the project. Participants provided consent for their DNA samples to be used to investigate the causes of CKD (Thomson et al., 2019). The current study subsequently received the support of the Tiwi Island Land Council.
Ethical approval
Approval was formally obtained through several consultations over 30 years with the Tiwi Land Council, Tiwi Elders, and traditional owners of the Tiwi Islands. The Tiwi Islands are privately owned and follow a permission system that recognises the importance and value of Aboriginal responsibility towards the country and is consistent with land titles held under Australian Law. The Northern Territory Department of Health also provided ethical approval for this study (2012–1767) followed by approvals by the QUT HREC (No. 2022-6199-10439- HE26) and ANU HREC (No. 2014-663). Ethics for human participants were reviewed by the human research ethics committees of The Northern Territory Department of Health (2012–1767), The Australian National University (2014-663), The University of Queensland (2012001146), and The University of Tasmania (H0012832).
Sequence and variant pre-processing and quality control
Sequenced reads from 473 samples were mapped to GRCh38 reference genome and variant calling was carried out according to GATK best practices using the DRAGEN-GATK pipeline v3.8.4 (Miller et al., 2015). Multi-sample VCF files for 473 Tiwi individuals were pre-processed using BCFtools v1.9 (Danecek et al., 2021) to normalize and left-align the indels, add unique variant IDs, and remove exact duplicate variants. Genotype-level filtering was performed using BCFtools (DP < 10 and/or GQ < 20 genotypes were set to missing). Filtered per-chromosome VCFs were converted to the plink format using plink v1.9 (Chang et al., 2015). Variants with >10% missingness were excluded and Principal Component Analysis (PCA) was performed with stringently filtered high-quality autosomal single nucleotide polymorphisms (SNPs) to identify outlier samples. Duplicate samples and monozygotic twins were removed using plink–king-cutoff 0.354. Samples with extreme heterozygosity (±3 standard deviations from the mean heterozygosity rate) and missingness >10% were also excluded. Variants with Hardy-Weinberg Equilibrium (HWE) p-value < 1 × 10−6 were removed to obtain a high-quality variant set for downstream analyses.
Principal Component Analysis
Autosomal SNPs were selected from the VCF file. Variants were filtered for >1% missingness, MAF <1%, and HWE P < 1 × 10−6. Samples with >10% missingness were excluded. Filtered variants were LD-pruned using plink–indep-pairwise 50 5 0.2 and duplicate/monozygotic twins were removed using plink–king-cutoff 0.354. The 1KGP multi-sample VCF file containing 2504 unrelated individuals (Americans: 347, Europeans: 503, Africans: 661, South Asians: 489, East Asians: 504) was also filtered using the above criteria, and common variants in both the Tiwi and 1KGP datasets were merged. PCA was run using plink–pca and the eigenvec file was plotted using R statistical software v.4.0.3 (R: A language and environment for statistical computing, 2021). PCA outlier samples were identified using PC1 and PC2 thresholds in PCA plots.
Identification of star alleles
Haplotypes consisting of a combination of variants [SNPs, indels, or structural variants (SVs)] that are inherited together are called star alleles (*) in pharmacogenomics. We selected 19 genes with pharmacological relevance for this analysis and a set of bioinformatics tools to identify star alleles present in these genes. The star allele calling tools included Cyrius v1.1.1 (Chen et al., 2021), PyPGx v0.17.0, StellarPGx v1.2.5 (Twesigomwe et al., 2021), Stargazer v.1.0.8 (Seung-been Lee et al., 2019; Lee et al., 2019), Aldy v3.3 (Numanagic et al., 2018) and PGxPOP v1.0 (McInnes et al., 2021). A consensus set of star alleles, called in agreement with at least two tools per each gene, were identified through an ensemble genotyping approach for further downstream analysis (Twesigomwe et al., 2020), except ABCG2, RYR1 and CACNA1S which are only supported by PyPGx tool (Lee et al., 2022). Genes with known SVs such as large deletions, insertions, or hybrids (e.g., CYP2D6, CYP2B6, CYP4F2, and G6PD), were evaluated using Cyrius, PyPGx, and StellarPGx which have better capability to detect complex variants. The GRCh38-aligned BAM files were used as input to the tools and consensus genotypes were manually evaluated.
Metabolic phenotypes [normal metabolizer (NM), intermediate metabolizer (IM), poor metabolizer (PM), rapid metabolizer (RM) or ultra-rapid metabolizer (UM)] and phenotypes associated with altered function in drug targets or drug transporters were assigned for diplotypes according to PharmGKB, PharmVar and CPIC definitions. Population specific frequencies for star alleles and phenotypes were assigned by PharmGKB.
Prevalence of high-evidence variants
PharmGKB clinical annotations for 265 unique variants (118 SNPs and 147 haplotypes) with PharmGKB level 1A and 1B were considered as high-evidence PGx variants (accessed 10th July 2022), and were screened for their frequency and distribution in the Tiwi and compared to other global populations from gnomAD, 1KGP and UK Biobank (UKB) 200k exome data (Szustakowski et al., 2021).
Characterization of potentially actionable variants in VIPs
We screened 67 VIPs (MT-RNR1 mitochondrial gene was excluded), defined by the PharmGKB as significantly involved in the metabolism or response of one or more drugs, to identify known and novel deleterious variants enriched in Tiwi that could potentially alter drug responses that have yet to be included in official star allele definitions (Figure 1). The variants in 67 genes were selected from the filtered Tiwi VCF and annotated with ANNOVAR (Wang et al., 2010) to add global population (1KGP, gnomAD) AFs, dbSNP rsIDs, and ClinVar annotations. Additionally, clinical annotations from the Human Gene Mutation Database (HGMD) (Stenson et al., 2003) and AFs from the UKB 200k exome data were also added. AF comparisons between Tiwi and gnomAD_All population were carried out using Fisher’s exact test with Benjamini–Hochberg correction for multiple testing error to select statistically significant variants. Variants with functional annotations in ClinVar or HGMD were screened to identify known pathogenic variants in the Tiwi population (Figure 1). Variants without any functional information were assessed using in silico computational algorithms (SIFT “D”, PolyPhen2 “D” or “P”, LRT “D”, MutationTaster “D” or “A”, MutationAssessor “H” or “M”, FATHMM “D”, MetaSVM “D”, MetaLR “D” or CADD >20). Variants predicted to be deleterious by at least two tools or having a CADD phred score >20 were defined as potentially deleterious that could alter the protein function. Of these, commonly enriched variants (AF > 1%) with >2-fold AF in Tiwi Vs. rare in gnomAD_All (AF < 6% in gnomAD_All) or absent in all other global populations were identified. The AF comparisons between Tiwi and gnomAD_All population were carried out using Fisher’s exact test with Benjamini–Hochberg multiple testing error correction. Variants having AF differences with adjusted p-value <0.05 vs. gnomAD_All were selected as statically significant variants for further downstream analysis. High-evidence variants and variants detected in the star allele analysis were excluded to identify potentially actionable novel PGx variants in VIPs.
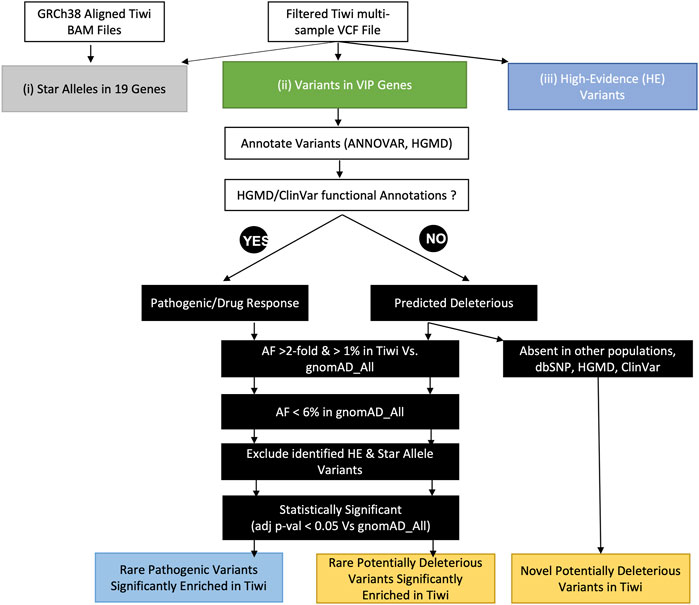
FIGURE 1. Schematic diagram of downstream analyses to identify pharmacologically important variants in the study population. This analysis was performed through three approaches: 1) the identification of star alleles in 19 pharmacogenes using an ensemble of star allele calling tools, 2) the identification of pharmacologically important variants in VIP genes, and 3) the identification of high-evidence variants in the study cohort.
Results
Population structure of Tiwi people
We have previously shown that Tiwi people are genetically distinct using a separately collected cohort of 249 individuals (Thomson et al., 2019) and a subset of the cohort used in this study consisting of 187 individuals (Jaya Shankar et al., 2022). The findings were recapitulated in the current study through PCA analysis of a larger cohort consisting of 469 individuals after QC (Figure 2). A total of ten individuals clustered with the European and East Asian populations likely due to admixture in the Tiwi population. These individuals were excluded from our analyses to remove bias in allele frequency calculations. Majority of the Tiwi people clustered separately from other global populations, revealing that they are genetically distinct.
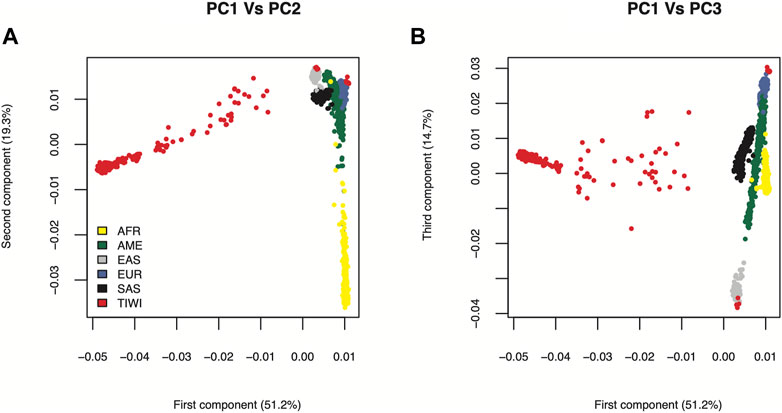
FIGURE 2. PCA plot of Tiwi and 1KGP global populations. The principal components were generated using stringently filtered 54,710 common genome-wide SNPs from 469 Tiwi and 2504 1KGP unrelated individuals. (A) Principal components 1 and 2 explained 70.5% of the observed variance (B) Principal components 1 and 3 explained 65.9% of the observed variance. African (AFR), American (AME), East Asian (EAS), European (EUR), South Asian (SAS).
Distribution of clinically actionable haplotypes, genotypes and phenotypes in Tiwi people
The prevalence of no function alleles and their clinical implications
No function alleles in certain pharmacogenes resulting in an impaired metabolic phenotype were found to be more common in the Tiwi cohort compared to other populations (Figure 3A, Supplementary Table S1). For instance, the CYP3A5*3 was the most prevalent allele with a frequency of 0.94. A similar frequency has been reported in Europeans (0.92) whereas the frequency tends to be lower in others (South Asians: 0.67 and Africans: 0.24). The CYP3A5 IMs/expressers (*1/*3) and PMs/nonexpressers (*3/*3) were 12.2% and 87.8% in the cohort, respectively. These prevalences closely represent frequencies in Europeans (IMs: 14%; PMs: 86%) but the presence of PMs in other populations were comparatively lower (<77%) while IMs were higher than in Tiwi (>21%) (Figure 3B, Supplementary Table S1). Unlike other CYP enzymes, CYP3A5 expressers (NMs or IMs) have been recommended to use a higher starting dose of tacrolimus, while a standard starting dose has been recommended for nonexpressers (PMs) (Birdwell et al., 2015) (Table 1). Tacrolimus concentration should be high enough to prevent organ rejection while not reaching toxic levels. The presence of expressers in the Tiwi was low compared to other populations. However, due to substantial evidence of kidney-related complications which could lead to kidney transplant events, genotyping could be beneficial to increase tacrolimus effectiveness and prevent ADRs in this population.
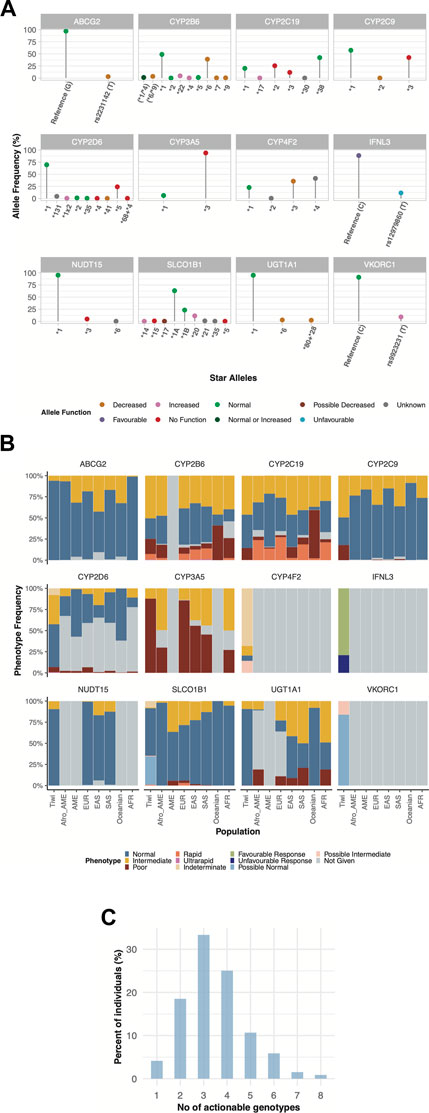
FIGURE 3. Pharmacogenetic variability of haplotypes (star alleles) and predicted phenotypes across twelve pharmacogenes in Tiwi. (A) Allele frequencies of star alleles identified across 12 genes in the study cohort. Colours on dots in star alleles represent the allele function. (B) Frequency and distribution of predicted phenotypes associated with star alleles compared to global populations across 12 pharmacogenes. (C) Distribution of clinically actionable genotypes in Tiwi. African American (Afro_AME), American (AME), European (EUR), East Asian (EAS), South Asian (SAS), African (AFR). In (A): (*6/*9)—could not accurately assigned between *6 and *9. (*1/*4) - could not accurately assigned between *1 and *4.
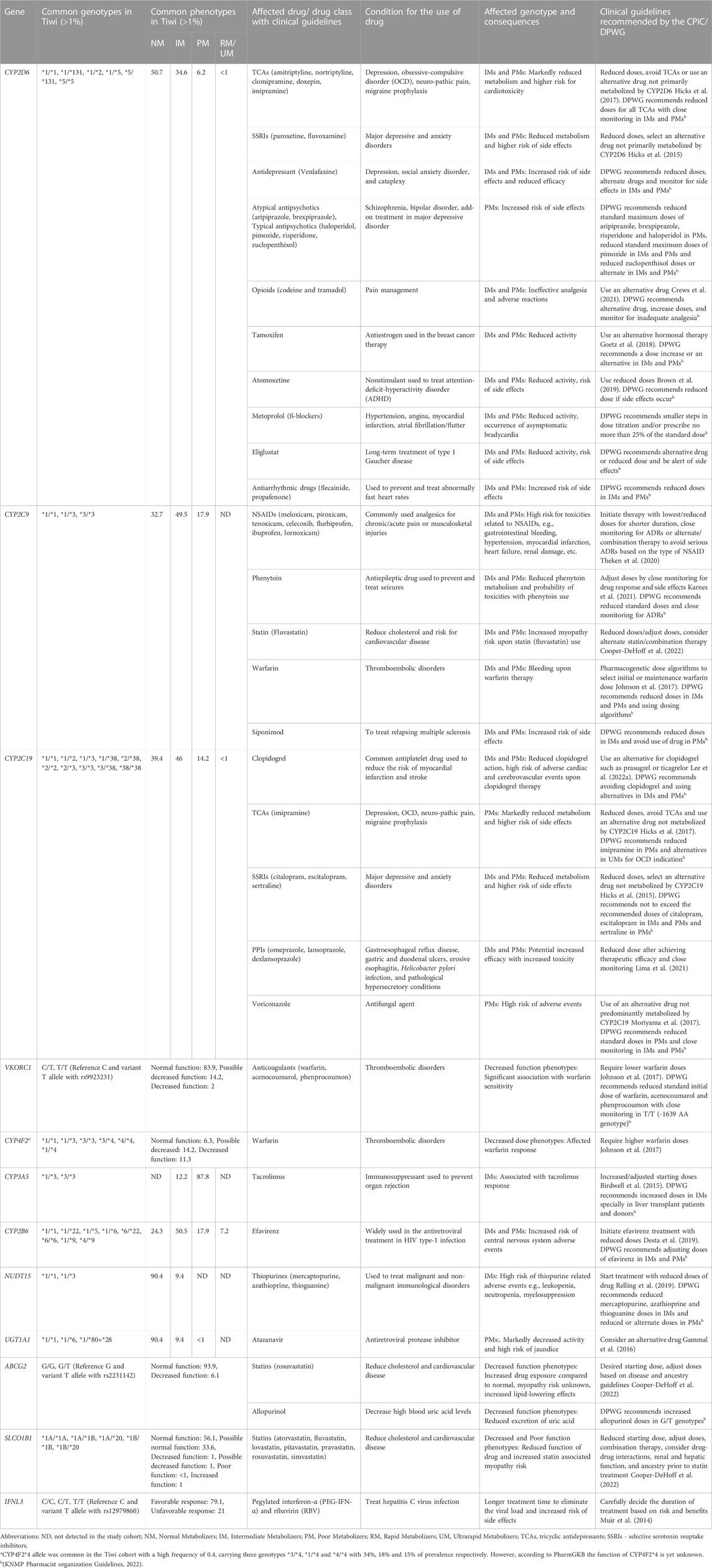
TABLE 1. Clinically actionable genotypes and phenotypes commonly detected in the Tiwi population and their clinical implications.
Also, the CYP2C9*3 No function allele had a frequency of 0.42 in this cohort, but a lower frequency has been reported in other populations (Europeans: 0.08, South Asians: 0.1, Africans: 0.01, Oceanians: 0.02). The AF of CYP2C19*2 in Tiwi was 0.25, which was higher as compared to Europeans (0.15) and Africans (0.16) but lower than, that is, reported in Asians (0.3) and Oceanians (0.61). On the other hand, CYP2C19*3 had an AF of 0.12 in the Tiwi cohort but was much lower in other populations (<0.07) except in Oceanians (0.15). More than half of the Tiwi cohort carried alleles associated with impaired CYP2C19 function, with IMs contributing 46% and PMs 14.2%. The prevalence of IMs and PMs were similar in East Asians (IMs: 46% and PMs: 13%) but lower in other groups (IMs<41% and PMs<8%), except for PMs reported in Oceanians (57%) (Figure 3B, Supplementary Table S1). CYP2C19 is a predominant determinant of patient responses to clopidogrel, a widely used antiplatelet drug in individuals at a higher risk of myocardial infarction or stroke. The CYP2C19 IMs and PMs have been reported to exhibit reduced clopidogrel effectiveness, leading to treatment failures and a high risk of adverse cardiac and cerebrovascular events (Lee et al., 2022). The CYP2C19 PMs have shown greatly reduced tricyclic antidepressants (TCA) efficacy and decreased responses to selective serotonin reuptake inhibitors (SSRIs), proton pump inhibitors (PPIs), and the antifungal agent voriconazole, warranting dose adjustments or an alternative drug to avoid treatment failures and side effects (Hicks et al., 2015; Hicks et al., 2017; Moriyama et al., 2017; Lima et al., 2021) (Table 1).
Similarly, the prevalence of homozygous CYP2C9*3 in PMs was 17.9% in the Tiwi, which was comparatively higher than in other global populations (0.02%–1.2%). The CYP2C9*3 was found in heterozygous IMs (49.2%) and was highly prevalent in the Tiwi as compared to all other populations (3%–18% across global populations) (Figure 3B, Supplementary Table S1). The CYP2C9 IMs and PMs renders them vulnerable to toxicities related to nonsteroidal anti-inflammatory drugs (e.g., meloxicam, piroxicam), phenytoin, statins, siponimod, and warfarin therapy that might lead to serious bleeding. CPIC recommends dose adjustments and careful monitoring for ADRs or the use of an alternate drug not primarily metabolized by CYP2C9 in these groups (Johnson et al., 2017; Theken et al., 2020; Karnes et al., 2021; Cooper-DeHoff et al., 2022; KNMP Pharmacist organization Guidelines, 2022) (Table 1).
The CYP2D6*5 whole allele deletion was remarkably higher in Tiwi (0.24). A frequency of 0.17 and 0.16 have been previously reported among South Africans (Gaedigk and Coetsee, 2008) and north Indians (Taylor et al., 2020) respectively. The prevalence of *5 was comparatively lower among others, with 0.03 frequency in Europeans and 0.05 in Asians and Africans. The CYP2D6 enzyme is a key metabolizer for >25% of clinically used drugs (Zhou, 2009). We found that the CYP2D6*5 whole allele deletion was the major contributor in CYP2D6 PMs and IMs of the Tiwi cohort with 6.2% and 34.6% prevalence respectively. The presence of PMs and IMs were remarkably higher in the Tiwi as compared to other ethnicities except PMs reported in Europeans (PMs: 6.5% in Europeans and <3% in others; IMs: 20% in East Asians and <11% in others) (Figure 3B, Supplementary Table S1). Impaired CYP2D6 function has a high risk of ineffective analgesia and ADRs when conducting opioid therapy using codeine and tramadol in CYP2D6 IMs and PMs has been well established (Crews et al., 2021). In CYP2D6 IMs and particularly in PMs, reduced metabolism of the widely used classes of antidepressants including TCAs (Hicks et al., 2017) and SSRIs (e.g., fluvoxamine and paroxetine) (Hicks et al., 2015) has been frequently reported. CYP2D6 IMs and PMs have a higher risk of breast cancer recurrence when treated with tamoxifen (Goetz et al., 2018) and an impaired metabolism has been reported during atomoxetine treatment (Brown et al., 2019). Hence, genotype-guided treatment strategies in CYP2D6 phenotypes with an impaired function is recommended (Table 1). With 41% of the cohort being CYP2D6 IMs or PMs with a potentially impaired CYP2D6 metabolism, PGx studies of the Tiwi population thus have important implications for genotype-guided therapy related to several medications.
Another No function allele, NUDT15*3, was detected in Tiwi with an AF of 0.05, which was higher than Europeans (0.002) but lower than Asians (0.06). The NUDT15 IMs carrying the NUDT15*3 in *1/*3 genotype (9.4%) was lower than the prevalence reported in Asians (13%–17%) but much higher than previously reported in Europeans (0.76%) (Figure 3B, Supplementary Table S1). The NUDT15 plays an important role in the thiopurine response used in the treatment of inflammatory diseases and cancer. Reduced starting doses are recommended for IMs to prevent increased risk of thiopurine-related toxicities (Relling et al., 2019) (Table 1).
The prevalence of decreased function alleles and their clinical implications
Other functionally important alleles that predicted higher AF in the Tiwi population included the decreased function alleles in CYP4F2 and CYP2B6 associated with drug metabolism. The CYP2B6*6 had an AF of 0.39 in Tiwi, where lower frequencies were reported in other populations (<0.2) except in Africans (0.4) and Oceanians (0.6). Overall, 75.6% of the cohort predicted to have impaired CYP2B6 metabolism with the majority being IMs (50.5%). Other populations have reported a comparatively lower prevalence of 33%–47% for IMs. CYP2B6 PMs comprised 18%, which was higher than all others except in Oceanians (41%) and Africans (23.7%) (Figure 3B, Supplementary Table S1). Additionally, 7.2% of the Tiwi cohort showed rapid or ultrarapid metabolism of CYP2B6. CYP2B6 IMs and PMs face a greater risk of central nervous system toxicities upon administration of efavirenz, a commonly used drug in antiretroviral therapy, and CPIC recommends initiating treatments with lower doses to minimize complications (Desta et al., 2019) (Table 1).
Similarly, the CYP4F2*3 allele was found with an AF of 0.36 in this cohort. The prevalence of CYP4F2*3 in Tiwi was higher compared to Europeans (0.27), East Asians (0.23) and Africans (0.1) but closer to, that is, reported among Americans and South Asians (0.4). In contrast, decreased function alleles, VKORC1 rs9923231 variant T allele and ABCG2 rs2231142 variant T allele were reported with 0.1 and 0.03 frequency in the Tiwi cohort, associated with drug target and transport respectively. Global populations have reported higher frequencies of rs9923231(T) (AF ≥ 0.4) except for populations of South Asian and African ancestry (<0.2). Similarly, higher frequencies have been reported for rs2231142 (T) among East Asians (0.31) and Oceanians (0.2), while South Asians and Africans have reported lower frequencies of 0.09 and 0.006 respectively. Warfarin response is strongly associated with the rs9923231 variant in VKORC1, and lower doses are recommended for patients with affected genotypes (Johnson et al., 2017). This variant had a prevalence of 2% in homozygotes (rs9923231/rs9923231) and 14.2% in heterozygotes (*1/rs9923231) with a possible decreased function (Figure 3B, Supplementary Table S1). In addition to CYP2C9 and VKORC1 alleles, the CYP4F2*3 has also been linked to increased warfarin dose requirements in Europeans and Asians but not among Africans. The CYP4F2*3 impaired phenotype, predicted a prevalence of 11.33% in homozygotes and 14.16% in *1/*3 heterozygotes leading to possible decreased function phenotypes (Johnson et al., 2017) (Figure 3B, Supplementary Table S1). Also, the rs2231142 variant in ABCG2, which reduces the activity of the encoded transporter protein, was found to be heterozygous in 6.1% of the Tiwi cohort. Although not affected as rs2231142 homozygotes, dose adjustments based on disease and ancestry have been recommended for heterozygotes. The impaired ABCG2 has clinical implications with allopurinol which is used to reduce high blood uric acid levels requiring increased doses of the drug (Saito et al., 2016) (Table 1).
Additionally, we found two other decreased function alleles *6 and *80+*28 in UGT1A1 gene, involved with drug metabolism which showed AF of 0.03 and 0.02 in the cohort respectively, with implications for atazanavir used in antiretroviral therapy (Gammal et al., 2016). The UGT1A1*6 allele has been reported with high frequency among East Asians (0.15) but much lower in Europeans (0.008).
Other functionally relevant alleles detected in Tiwi people
Some other functional pharmacogene variants found in Tiwi included the increased function allele SLCO1B1*20 associated with drug absorption which showed an AF of 0.11 (Europeans: 0.04). Also, the IFNL3 variant T allele (rs12979860) involved with an unfavourable response had an 0.11 frequency in this cohort. A total of 20.9% of the Tiwi cohort were either homozygous or heterozygous for the rs12980275 variant allele in the IFNL3 gene leading to an unfavourable response to pegylated interferon-α (PEG-IFN-α) and ribavirin (RBV) therapy in the treatment of hepatitis C infection, requiring careful consideration of the possibility of side effects before initiating therapy (Muir et al., 2014) (Table1). Also, several other variant alleles of interest were reported with high frequency among the Tiwi cohort. These included the CYP2D6*131 unknown function allele defined by the rs17002853 which was the second abundant CYP2D6 allele detected in the cohort having an AF of 0.043. The CYP4F2*4 allele with an unknown function was also observed with 0.4 frequency in Tiwi which is defined by the two SNPs, rs3093105 and rs2108622.
For seven actionable pharmacogenes, CFTR, RYR1, CACNA1S, DPYD, TPMT, G6PD, and CYP2C8, over 99% of the individuals in the study cohort were predicted to have normal metabolism (Supplementary Table S2). For genes, CFTR, TPMT, CACNA1S and RYR1 variant alleles were not detected within the Tiwi cohort. In DPYD gene, HapB3 (c.1236G>A) deficient allele was detected with 0.0012 frequency, which was 0.02 among Europeans and South Asians. Similarly, the CYP2C8*3 which had an AF of 0.001 was the only CYP2C8 variant allele detected in Tiwi. The prevalence of CYP2C8*3 was 10%–23% among Caucasians while rare or absent in Asians and Africans (Ingelman-Sundberg et al., 2007). The G6PD deficient allele frequency within the cohort was low (0.011) with a high prevalence reported in malaria endemic regions, e.g., 33% of prevalence across Africa has been reported previously (Howes et al., 2012).
Overall, we identified that every Tiwi individual in the cohort carries at least one actionable genotype and 77% of them have at least three actionable genotypes with important implications for drug-gene interventions in the Tiwi population (Figure 3C).
Prevalence of high-evidence PGx variants
Among the unique 265 variants in the PharmGKB with the highest level of evidence for their pharmacological relevance, we identified 20 high-evidence SNPs and 27 high-evidence variant haplotypes in the Tiwi cohort (Figure 4, Supplementary Table S3).
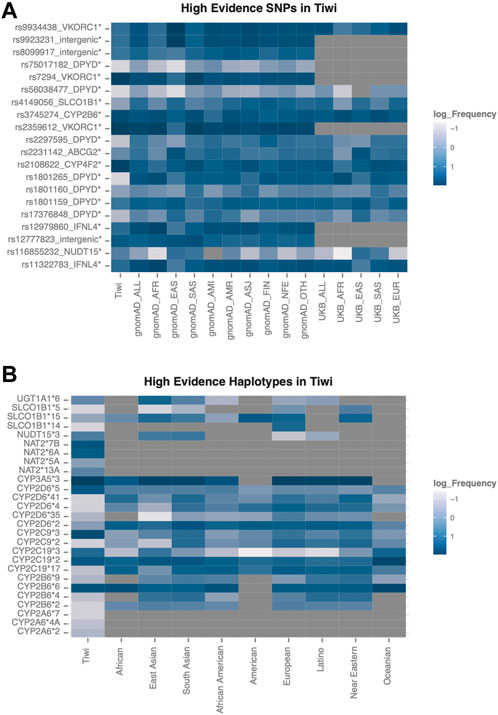
FIGURE 4. Variants with highest evidence for their pharmacological relevance identified in Tiwi. (A) Frequency distribution across global populations for the 20 high-evidence SNPs. Variants with statistically significant allele frequencies are marked with * (adj p-value <0.05 Vs. gnomAD_All) (B) Allele frequencies across global populations for the 27 high-evidence haplotypes in Tiwi.
The frequency differences of the 20 high-evidence SNPs were significant (adj p-value <0.05 Vs. gnomAD_All) (Figure 4A). Of these, seven variants were common in Tiwi with an AF > 0.1 and two of them (rs7294, AF 0.9 and rs2108622, AF 0.78) had AFs two-fold higher compared to gnomAD_All population. Prevalences of these two variants were lower in all other populations (0.1–0.5), except rs7294, which was enriched in South Asians with an AF of 0.7. The intronic rs7294 T allele has been associated with increased warfarin doses compared to the reference C allele in different populations (Chen et al., 2014; Krishna Kumar et al., 2014). Overall, 347 and 65 Tiwi individuals carried the TT and CT genotype for this variant respectively, with potential implications for high warfarin dose requirements. Moreover, the association of the nonsynonymous variant rs2108622, the core variant in CYP4F2*3, with warfarin is well known (Johnson et al., 2017). The rs12777823 near CYP2C18 was also common in Tiwi with an AF of 0.25 and the prevalence was higher in Africans and Asians (0.25–0.36) than other populations (0.1–0.2). The rs12777823 variant A allele has shown a significant interference with warfarin clearance and reduced doses are recommended in African Americans (Johnson et al., 2017). The rs2359612 in the VKORC1 intronic region was prevalent in Tiwi with an AF > 0.9 compared to other populations. Also, the rs2359612 variant G allele has been reported with increased dose of warfarin in populations (Limdi et al., 2008; Schwarz et al., 2008). Non-synonymous core variant rs3745274 in CYP2B6 showed an AF of 0.445 in Tiwi and usually reported high in Africans and South Asians (0.37–0.4), but lower in other global populations. As previously discussed, CYP2B6 is involved with efavirenz response. Intronic variant rs12979860 and splicing variant rs11322783 in IFNL3/IFNL4 were common in Tiwi with an AF > 0.1, but lower compared to other populations. Both variants were associated with unfavourable response to PEG-IFN-α and RBV used in the treatment of hepatitis C infection (Muir et al., 2014).
Among the 27 high-evidence haplotypes identified in Tiwi, eight were common with prevalence >10%. As shown in Figure 4B, CYP2B6*6, CTP2D6*5, CYP2C19*2, CYP2C19*3, CYP2C9*3, CYP3A5*3, NAT2*6A, and NAT2*7B haplotypes showed the highest prevalence within the cohort, most of which have important drug interventions as detailed in Table 1.
Spectrum of potentially actionable variants in VIPs
We analysed 67 VIPs, defined by the PharmGKB to be significantly associated with drug metabolism with variants in these genes being associated with a higher risk of altered drug responses and serious side effects. In total, we identified 26,895 variants in the 67 VIPs, of which 300 were pathogenic or associated with drug response according to HGMD or ClinVar annotations. Among these, 14 rare variants were significantly enriched in Tiwi (Tiwi: >10%, gnomAD_All and UKB_All: <6%, adj p-value <0.05 Vs. gnomAD_All) (Figure 5A, Supplementary Table S3). The CYP2C9 intronic variant, rs9332127 which is associated with warfarin sensitivity, was observed with 0.12 frequency in Tiwi, 0.16 average in Africans and less than 0.06 in all other populations. The prevalence of SLCO1B1 upstream region variant, rs4149015 involved with increased pravastatin clearance was 0.13 in Tiwi and lower in all other populations except in East Asians and Finnish (East Asians: 0.13, Finnish: 0.11, others: <0.07). The rs4149015 variant was also inferred by star allele calling tools indicating the presence of a novel SLCO1B1 allele in this population. Additionally, two nonsynonymous variants (rs1799931 and rs1131341) and intergenic rs9332096 variant were associated with reduced activity, while two upstream variants (rs6413420 and rs12364283) were associated with increased activity.
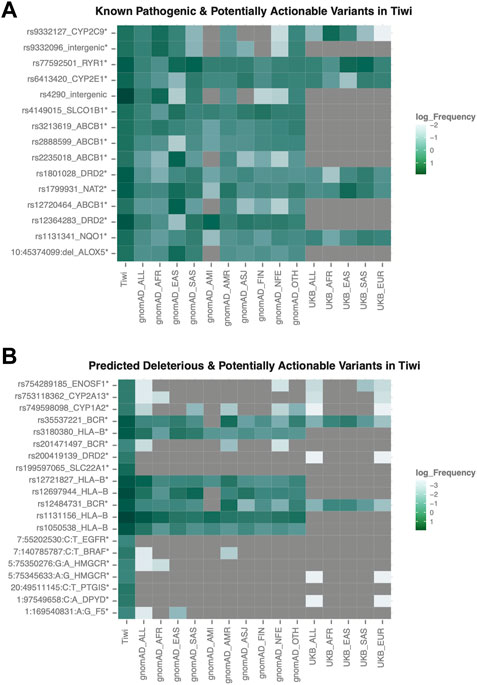
FIGURE 5. Frequency distribution of potentially actionable variants in VIP genes. (A) Rare pathogenic variants commonly enriched in Tiwi (B) Rare variants predicted to be deleterious and commonly enriched in Tiwi. Variants with statistically significant allele frequencies are marked with * (adj p-value <0.05 Vs. gnomAD_All). Variant rs12721827 was common in gnomAD_All (AF > 0.06).
For 25,367 variants with no functional annotations in HGMD or ClinVar databases, their potential functional relevance was predicted using in silico functionality prediction algorithms. Interestingly, among the predicted 86 potentially deleterious variants, 31 exonic variants (30 nonsynonymous and one stop-gain) in 21 VIP genes were not reported in any major populations (gnomAD, 1KGP, UKB) or in public databases (dbSNP, HGMD, ClinVar) (Table 2, Supplementary Table S3). Of these 31 variants, five were common in Tiwi with AF > 1%. We observed another 16 nonsynonymous potentially deleterious rare variants (AF < 6% in gnomAD_All), which have been previously reported in either dbSNP or in one/more major populations, which were significantly enriched in Tiwi with AF > 20-fold compared to gnomAD_All or UKB_All populations (adj p-value <0.05 Vs. gnomAD_All) (Figure 5B, Supplementary Table S3).
Discussion
The complexity of the human genome is strikingly highlighted by the existence of ethnically distinct pharmacogenomic variants that guides personalized medicine, with such diversity being particularly pronounced in geographically isolated groups (Rosenberg et al., 2005). Such population clusters are likely to possess a unique PGx profile distinct from major global populations. Non-Europeans carry the highest burden of functionally deleterious variants, many of which are not represented in current star allele definitions (McInnes et al., 2021). Moreover, rare genetic variants have been attributed greater functional significance, and identifying population-specific rare variants with pharmacological relevance is crucial to understanding the broader PGx profile of a population.
Most genetic and clinical PGx studies are based on populations of European ancestry, and the findings of these studies cannot be extrapolated to unique minority groups. Indigenous Australians are culturally and ancestrally diverse, comprising one of the largest Indigenous communities in the world. Despite many recent efforts to characterize their underlying genetic background, there have been insufficient pharmacogenetic studies focused on these Indigenous communities. Our study focuses on characterizing the PGx profile of the founder Indigenous population. We and others have characterized the blood group profiles (Jadhao et al., 2022), genetic susceptibility to chronic kidney disease (Thomson et al., 2019) and a variant affecting platelet function (Ningtyas et al., 2020) in this population. This study is the first to comprehensively investigate clinically actionable pharmacogenes in the Tiwi people, contributing novel, important findings and further validating the results of a previous attempt to characterise Tiwi PGx profiles (Jaya Shankar et al., 2022).
Our analysis of 459 Tiwi community members revealed that every individual in the study cohort carries at least one clinically actionable genotype associated with one or more of the 138 drugs linked to clinically actionable haplotypes across 19 pharmacogenes. Similar frequencies have been reported in other study groups across different gene panels. According to the PG4KDS study, nearly 98.5% Caucasian and 99.1% African people in the United States carry at least one high-risk genotype across 12 pharmacogenes (Dunnenberger et al., 2015). The eMERGE-PGx study across 82 pharmacogenes reported that 96.2% of the participants carry at least one actionable genotype respectively (Bush et al., 2016). These findings show the importance of selecting an appropriate gene panel for a specific population for pre-emptive testing, to ensure transferring the benefits to a majority.
Of 265 high-evidence variants in PharmGKB, 20 high-evidence SNPs and 27 high-evidence haplotypes were identified in the Tiwi cohort (Figure 4, Supplementary Table S3). Our findings also expand the current knowledge base, as we report an additional four pharmacologically important high-evidence variants, rs7294, rs2359612, rs11322783, and rs12777823 commonly enriched in Tiwi that were not observed among the known star alleles identified for this cohort. Furthermore, we identified another 14 functionally relevant known pathogenic variants (e.g., rs9332127), 16 potentially deleterious rare variants (e.g., rs3180380) (Figure 5, Supplementary Table S3), and 31 population-specific novel variants (Table 2) in VIP genes that could be potentially actionable in this population.
The results of our study have important implications for recommending suitable drugs and adjusting doses for this population based on the pharmacokinetics or pharmacodynamics of commonly prescribed drugs. We observed that nearly ten widely used drugs such as, clopidogrel, warfarin, atorvastatin, metoprolol, omeprazole, phenytoin, escitalopram, risperidone have clinically relavent drug-gene interactions reported in this study (Table 1). For example, we found that CYP2D6*5 whole allele deletion rates were nearly four-fold higher among the Tiwi people (24%) as compared to all other global populations. The CYP2D6*5 variant has been found at comparatively lower frequencies in other ethnic populations (Supplementary Table S1). Overall, 41% of the Tiwi people are either CYP2D6 IMs or PMs, with this rate being remarkably higher than all other reported global frequencies. Other CYP variants were also present at a relatively high frequency in the Tiwi cohort, much as in other populations. For example, the CYP2C9 no-function alleles were detected at a higher prevalence in the Tiwi (42.5%) than in other global populations, with reported prevalence rates of 11% in South Asians, 7.6% in Europeans, and 1% in Africans. Furthermore, CYP2B6 variants were present in 76% of the Tiwi cohort as compared to 20%–40% of African minority populations (Supplementary Table S1).
Variants in CYP2C19, VKORC1, and CYP4F2 were significantly associated with warfarin response. Additionally, high-evidence variants such as rs7294, rs2359612, and rs12777823 and potentially actionable variants such as rs9332127 that were commonly identified in the Tiwi have important implications for warfarin responses, warranting the use of warfarin dosing algorithms to calculate the appropriate dose before treatment. Statin-induced myopathy risk was also predicted to be increased in more than half of the cohort due to variants in CYP2C9, SLCO1B1, and ABCG2. Moreover, we identified a commonly enriched potentially actionable variant, rs4149015, that is, related to pravastatin clearance. Also, responses to antidepressants such as TCAs and SSRIs were likely to be affected due to the presence of CYP2D6 and CYP2C19 variants in more than 50% of Tiwi people. In cases where a drug is affected due to variants in multiple genes, CPIC highlights the benefits of adjusting doses based on population-specific combined genotype information to improve the efficacy and safety of treatments. Affected Tiwi individuals also carry a higher risk of drug toxicities and markedly diminished efficacy in treatments related to a wide range of drugs or drug classes including analgesics (NSAIDs), antiplatelets (clopidogrel), antiepileptics (phenytoin), antiretrovirals (efavirenz), antifungals (voriconazole), PPIs (omeprazole, lansoprazole, dexlansoprazole), tacrolimus, and statins (atorvastatin, simvastatin, rosuvastatin, and fluvastatin), most of which are commonly used for the treatment of chronic kidney disease and associated complications prevalent among the Tiwi people. Overall, our results indicate that Tiwi people carry a unique PGx profile, providing a sound basis underscoring the importance of implementing a panel-based cost-effective PGx testing approach in this population prior to prescribing drugs whose metabolism might be affected. Ultimately, these findings are expected to enhance the quality of health for the Tiwi people while reducing the burden on healthcare systems due to ADRs, treatment failures and extended hospital stays. Our approach is adaptable and equally beneficial to people in other populations and healthcare systems globally.
There are a few limitations of this study. First, this study population is highly homogeneous. This is expected due to the isolation of the Tiwi people for more than 10,000 years. Although we eliminated three highly similar individuals (plink -king-cutoff 0.354) from downstream analysis, we are reluctant to remove a greater proportion due to the limited availability of genomic data and the social and ethical barriers to its collection. Therefore, some degree of relatedness bias is still represented in the population allele frequencies in our study. In addition, the availability of meta-information pertaining to diseases and the use of medications per individual is limited and we were thus not able to assess the actual proportion of the population that could benefit from our analysis, though such an analysis could have added an additional level of evidence regarding the importance of PGx testing in this population. Moreover, we employed an ensemble genotyping approach to identify star alleles. Ensemble genotyping approaches have previously reported high haplotype concordance (Twesigomwe et al., 2020) and have shown better capability to detect star alleles using WGS data with sufficient coverage ( ≥ 30x) (Lee et al., 2022). Due to the highly polymorphic nature of certain genes, presence of complex structural variants, and paralogs within close proximity have significantly challenged accurate calling of star alleles in genes such as CYP2D6 and CYP2B6. Despite these factors, our findings show that pre-emptive testing can provide valuable guidance for clinical decision-making aimed at avoiding unfavourable responses following treatment and enhancing therapeutic efficacy.
Data availability statement
The Tiwi genomic data presented in this article cannot be readily accessed due to ethical approval restrictions on sharing the data publicly. Requests to access the datasets should be directed to SN (shiv.nagaraj@qut.edu.au).
Ethics statement
The studies involving human participants were reviewed and approved by QUT HREC, NT Health.
Author contributions
SN led the project and oversaw the analysis. SS designed and performed the research. SN and SS prepared the first draft of the manuscript. SJ assisted in data curation and reviewed the manuscript. WH, BM and H-JG refined the manuscript providing critical comments. All authors contributed to manuscript revision, read and approved the submitted version.
Funding
Funding for this research was supported by grants from the National Health and Medical Research Council (GNT1024207) and MRFF Genomics Health Futures Mission (76757). The Centre for Chronic Disease, The University of Queensland, is supported in-part by the NHMRC, Chronic Kidney Disease Centre of Research Excellence, 2016–2020 (APP1079502). The National Centre for Indigenous Genomics’ genome sequencing programme is supported by grants from the Australian Genomics Health Alliance, the Australian Research Data Commons (ARDC), the Canberra Medical Society, the National Computational Infrastructure (NCI) through their ANU and National Merit Allocation Schemes, and the NHMRC (GNT1143734).
Acknowledgments
The authors would like to acknowledge the following people: Barry Ullungurra for his help as the key contact person with the Tiwi Islanders; Bev Mcleod and Ceri Flowers for their project management and sample and data collection; Maria Scarlett for her considerable advice and guidance on the ethics of this project; and Beverley Hayhurst for the original sample collection and most notably the study participants and the Tiwi Land Council for their time and ongoing support for this project. We thank our Indigenous Advisory Committee comprising Carl Nixon, Pirrawayingi Puruntatameri and Therese Bourke for their guidance and advice on cultural practices.
Conflict of interest
The authors declare that the research was conducted in the absence of any commercial or financial relationships that could be construed as a potential conflict of interest.
Publisher’s note
All claims expressed in this article are solely those of the authors and do not necessarily represent those of their affiliated organizations, or those of the publisher, the editors and the reviewers. Any product that may be evaluated in this article, or claim that may be made by its manufacturer, is not guaranteed or endorsed by the publisher.
Supplementary material
The Supplementary Material for this article can be found online at: https://www.frontiersin.org/articles/10.3389/fphar.2023.1180640/full#supplementary-material
References
Australian Bureau of Statistics (2021). Tiwi Islands 2021 census all persons QuickStats. Available: https://www.abs.gov.au/census/find-census-data/quickstats/2021/LGA74050 (Accessed February 24, 2023).
Birdwell, K. A., Decker, B., Barbarino, J. M., Peterson, J. F., Stein, C. M., Sadee, W., et al. (2015). Clinical pharmacogenetics implementation consortium (CPIC) guidelines for CYP3A5 genotype and tacrolimus dosing. Clin. Pharmacol. Ther. 98 (1), 19–24. doi:10.1002/cpt.113
Brown, J. T., Bishop, J. R., Sangkuhl, K., Nurmi, E. L., Mueller, D. J., Dinh, J. C., et al. (2019). Clinical pharmacogenetics implementation consortium guideline for cytochrome P450 (CYP)2D6 genotype and atomoxetine therapy. Clin. Pharmacol. Ther. 106 (1), 94–102. doi:10.1002/cpt.1409
Bush, W. S., Crosslin, D. R., Owusu-Obeng, A., Wallace, J., Almoguera, B., Basford, M. A., et al. (2016). Genetic variation among 82 pharmacogenes: The PGRNseq data from the eMERGE network. Clin. Pharmacol. Ther. 100 (2), 160–169. doi:10.1002/cpt.350
Cacabelos, R., Cacabelos, N., and Carril, J. C. (2019). The role of pharmacogenomics in adverse drug reactions. Expert Rev. Clin. Pharmacol. 12 (5), 407–442. doi:10.1080/17512433.2019.1597706
Chang, C. C., Chow, C. C., Tellier, L. C., Vattikuti, S., Purcell, S. M., and Lee, J. J. (2015). Second-generation PLINK: Rising to the challenge of larger and richer datasets. Gigascience 4, 7. doi:10.1186/s13742-015-0047-8
Chen, J., Shao, L., Gong, L., Luo, F., Wang, J., Shi, Y., et al. (2014). A pharmacogenetics-based warfarin maintenance dosing algorithm from Northern Chinese patients. PLoS One 9 (8), e105250. doi:10.1371/journal.pone.0105250
Chen, X., Shen, F., Gonzaludo, N., Malhotra, A., Rogert, C., Taft, R. J., et al. (2021). Cyrius: Accurate CYP2D6 genotyping using whole-genome sequencing data. Pharmacogenomics J. 21 (2), 251–261. doi:10.1038/s41397-020-00205-5
Cooper-DeHoff, R. M., Niemi, M., Ramsey, L. B., Luzum, J. A., Tarkiainen, E. K., Straka, R. J., et al. (2022). The clinical pharmacogenetics implementation consortium guideline for SLCO1B1, ABCG2, and CYP2C9 genotypes and statin-associated musculoskeletal symptoms. Clin. Pharmacol. Ther. 111 (5), 1007–1021. doi:10.1002/cpt.2557
CPIC (2022). Clinical pharmacogenetics implementation consortium. Available: https://cpicpgx.org/(Accessed July 10, 2022).
Crews, K. R., Monte, A. A., Huddart, R., Caudle, K. E., Kharasch, E. D., Gaedigk, A., et al. (2021). Clinical pharmacogenetics implementation consortium guideline for CYP2D6, OPRM1, and COMT genotypes and select opioid therapy. Clin. Pharmacol. Ther. 110 (4), 888–896. doi:10.1002/cpt.2149
Danecek, P., Bonfield, J. K., Liddle, J., Marshall, J., Ohan, V., Pollard, M. O., et al. (2021). Twelve years of SAMtools and BCFtools. Gigascience 10 (2), giab008. doi:10.1093/gigascience/giab008
Desta, Z., Gammal, R. S., Gong, L., Whirl-Carrillo, M., Gaur, A. H., Sukasem, C., et al. (2019). Clinical pharmacogenetics implementation consortium (CPIC) guideline for CYP2B6 and efavirenz-containing antiretroviral therapy. Clin. Pharmacol. Ther. 106 (4), 726–733. doi:10.1002/cpt.1477
Dunnenberger, H. M., Crews, K. R., Hoffman, J. M., Caudle, K. E., Broeckel, U., Howard, S. C., et al. (2015). Preemptive clinical pharmacogenetics implementation: Current programs in five US medical centers. Annu. Rev. Pharmacol. Toxicol. 55, 89–106. doi:10.1146/annurev-pharmtox-010814-124835
Gabb, G. M., Vitry, A., Condon, J., Limaye, V., and Alhami, G. (2013). Serious statin-associated myotoxicity and rhabdomyolysis in aboriginal and torres strait Islanders: A case series. Intern Med. J. 43 (9), 987–992. doi:10.1111/imj.12196
Gaedigk, A., and Coetsee, C. (2008). The CYP2D6 gene locus in South African coloureds: Unique allele distributions, novel alleles and gene arrangements. Eur. J. Clin. Pharmacol. 64 (5), 465–475. doi:10.1007/s00228-007-0445-7
Gammal, R. S., Court, M. H., Haidar, C. E., Iwuchukwu, O. F., Gaur, A. H., Alvarellos, M., et al. (2016). Clinical pharmacogenetics implementation consortium (CPIC) guideline for UGT1A1 and atazanavir prescribing. Clin. Pharmacol. Ther. 99 (4), 363–369. doi:10.1002/cpt.269
Garrison, N. A., Hudson, M., Ballantyne, L. L., Garba, I., Martinez, A., Taualii, M., et al. (2019). Genomic research through an indigenous lens: Understanding the expectations. Annu. Rev. Genomics Hum. Genet. 20, 495–517. doi:10.1146/annurev-genom-083118-015434
Genomes Project Consortium Auton, A., Brooks, L. D., Durbin, R. M., Garrison, E. P., and Kang, H. M. (2015). A global reference for human genetic variation. Nature 526 (7571), 68–74. doi:10.1038/nature15393
Goetz, M. P., Sangkuhl, K., Guchelaar, H. J., Schwab, M., Province, M., Whirl-Carrillo, M., et al. (2018). Clinical pharmacogenetics implementation consortium (CPIC) guideline for CYP2D6 and tamoxifen therapy. Clin. Pharmacol. Ther. 103 (5), 770–777. doi:10.1002/cpt.1007
Gonzalez-Covarrubias, V., Morales-Franco, M., Cruz-Correa, O. F., Martínez-Hernández, A., García-Ortíz, H., Barajas-Olmos, F., et al. (2019). Variation in actionable pharmacogenetic markers in natives and mestizos from Mexico. Front. Pharmacol. 10, 1169. doi:10.3389/fphar.2019.01169
Hicks, J. K., Bishop, J. R., Sangkuhl, K., Muller, D. J., Ji, Y., Leckband, S. G., et al. (2015). Clinical pharmacogenetics implementation consortium (CPIC) guideline for CYP2D6 and CYP2C19 genotypes and dosing of selective serotonin reuptake inhibitors. Clin. Pharmacol. Ther. 98 (2), 127–134. doi:10.1002/cpt.147
Hicks, J. K., Sangkuhl, K., Swen, J. J., Ellingrod, V. L., Muller, D. J., Shimoda, K., et al. (2017). Clinical pharmacogenetics implementation consortium guideline (CPIC) for CYP2D6 and CYP2C19 genotypes and dosing of tricyclic antidepressants: 2016 update. Clin. Pharmacol. Ther. 102 (1), 37–44. doi:10.1002/cpt.597
Hitchman, L. M., Faatoese, A., Merriman, T. R., Miller, A. L., Liau, Y., Graham, O. E. E., et al. (2022). Allelic diversity of the pharmacogene CYP2D6 in New Zealand Māori and Pacific peoples. Front. Genet. 13, 1016416. doi:10.3389/fgene.2022.1016416
Howes, R. E., Piel, F. B., Patil, A. P., Nyangiri, O. A., Gething, P. W., Dewi, M., et al. (2012). G6PD deficiency prevalence and estimates of affected populations in malaria endemic countries: A geostatistical model-based map. PLoS Med. 9 (11), e1001339. doi:10.1371/journal.pmed.1001339
Ingelman-Sundberg, M., Sim, S. C., Gomez, A., and Rodriguez-Antona, C. (2007). Influence of cytochrome P450 polymorphisms on drug therapies: Pharmacogenetic, pharmacoepigenetic and clinical aspects. Pharmacol. Ther. 116 (3), 496–526. doi:10.1016/j.pharmthera.2007.09.004
Jadhao, S., Hoy, W., Lee, S., Patel, H. R., McMorran, B. J., Flower, R. L., et al. (2022). The genomic landscape of blood groups in Indigenous Australians in remote communities. Transfusion 62 (5), 1110–1120. doi:10.1111/trf.16873
Jaya Shankar, A., Jadhao, S., Hoy, W., Foote, S. J., Patel, H. R., Scaria, V., et al. (2022). Pharmacogenomic analysis of a genetically distinct Indigenous population. Pharmacogenomics J. 22, 100–108. doi:10.1038/s41397-021-00262-4
Johnson, J. A., Caudle, K. E., Gong, L., Whirl-Carrillo, M., Stein, C. M., Scott, S. A., et al. (2017). Clinical pharmacogenetics implementation consortium (CPIC) guideline for pharmacogenetics-guided warfarin dosing: 2017 update. Clin. Pharmacol. Ther. 102 (3), 397–404. doi:10.1002/cpt.668
Karnes, J. H., Rettie, A. E., Somogyi, A. A., Huddart, R., Fohner, A. E., Formea, C. M., et al. (2021). Clinical pharmacogenetics implementation consortium (CPIC) guideline for CYP2C9 and HLA-B genotypes and phenytoin dosing: 2020 update. Clin. Pharmacol. Ther. 109 (2), 302–309. doi:10.1002/cpt.2008
KNMP Pharmacist organization Guidelines (2022). Royal Dutch pharmacist's association. Available: https://www.knmp.nl/dossiers/farmacogenetica (Accessed December 21, 2022).
Kozyra, M., Ingelman-Sundberg, M., and Lauschke, V. M. (2017). Rare genetic variants in cellular transporters, metabolic enzymes, and nuclear receptors can be important determinants of interindividual differences in drug response. Genet. Med. 19 (1), 20–29. doi:10.1038/gim.2016.33
Krishna Kumar, D., Shewade, D. G., Loriot, M. A., Beaune, P., Balachander, J., Sai Chandran, B. V., et al. (2014). Effect of CYP2C9, VKORC1, CYP4F2 and GGCX genetic variants on warfarin maintenance dose and explicating a new pharmacogenetic algorithm in South Indian population. Eur. J. Clin. Pharmacol. 70 (1), 47–56. doi:10.1007/s00228-013-1581-x
Lee, C. R., Luzum, J. A., Sangkuhl, K., Gammal, R. S., Sabatine, M. S., Stein, C. M., et al. (2022a). Clinical pharmacogenetics implementation consortium guideline for CYP2C19 genotype and clopidogrel therapy: 2022 update. Clin. Pharmacol. Ther. 112 (5), 959–967. doi:10.1002/cpt.2526
Lee, S., Wheeler, M. M., Patterson, K., McGee, S., Dalton, R., Woodahl, E. L., et al. (2019b). Stargazer: A software tool for calling star alleles from next-generation sequencing data using CYP2D6 as a model. Genet. Med. 21, 361–372. doi:10.1038/s41436-018-0054-0
Lee, S. B., Shin, J. Y., Kwon, N. J., Kim, C., and Seo, J. S. (2022b). ClinPharmSeq: A targeted sequencing panel for clinical pharmacogenetics implementation. PLoS One 17 (7), e0272129. doi:10.1371/journal.pone.0272129
Lee, S. B., Wheeler, M. M., Thummel, K. E., and Nickerson, D. A. (2019a). Calling star alleles with stargazer in 28 pharmacogenes with whole genome sequences. Clin. Pharmacol. Ther. 106 (6), 1328–1337. doi:10.1002/cpt.1552
Lim, R., Ellett, L. M. K., Semple, S., and Roughead, E. E. (2022). The extent of medication-related hospital admissions in Australia: A review from 1988 to 2021. Drug Saf. 45 (3), 249–257. doi:10.1007/s40264-021-01144-1
Lima, J. J., Thomas, C. D., Barbarino, J., Desta, Z., Van Driest, S. L., El Rouby, N., et al. (2021). Clinical pharmacogenetics implementation consortium (CPIC) guideline for CYP2C19 and proton pump inhibitor dosing. Clin. Pharmacol. Ther. 109 (6), 1417–1423. doi:10.1002/cpt.2015
Limdi, N. A., Karnett, D., Goldstein, J. A., Beasley, T. M., McGwin, G., Adler, B. K., et al. (2008). Influence of CYP2C9 and VKORC1 on warfarin dose, anticoagulation attainment and maintenance among European-Americans and African-Americans. Pharmacogenomics 9 (5), 511–526. doi:10.2217/14622416.9.5.511
Malaspinas, A. S., Westaway, M. C., Muller, C., Sousa, V. C., Lao, O., Alves, I., et al. (2016). A genomic history of Aboriginal Australia. Nature 538 (7624), 207–214. doi:10.1038/nature18299
McInnes, G., Lavertu, A., Sangkuhl, K., Klein, T. E., Whirl-Carrillo, M., and Altman, R. B. (2021). Pharmacogenetics at scale: An analysis of the UK Biobank. Clin. Pharmacol. Ther. 109 (6), 1528–1537. doi:10.1002/cpt.2122
Miller, N. A., Farrow, E. G., Gibson, M., Willig, L. K., Twist, G., Yoo, B., et al. (2015). A 26-hour system of highly sensitive whole genome sequencing for emergency management of genetic diseases. Genome Med. 7, 100. doi:10.1186/s13073-015-0221-8
Moriyama, B., Obeng, A. O., Barbarino, J., Penzak, S. R., Henning, S. A., Scott, S. A., et al. (2017). Clinical pharmacogenetics implementation consortium (CPIC) guidelines for CYP2C19 and voriconazole therapy. Clin. Pharmacol. Ther. 102 (1), 45–51. doi:10.1002/cpt.583
Mostafa, S., Kirkpatrick, C. M. J., Byron, K., and Sheffield, L. (2019). An analysis of allele, genotype and phenotype frequencies, actionable pharmacogenomic (PGx) variants and phenoconversion in 5408 Australian patients genotyped for CYP2D6, CYP2C19, CYP2C9 and VKORC1 genes. J. Neural Transm. (Vienna) 126 (1), 5–18. doi:10.1007/s00702-018-1922-0
Muir, A. J., Gong, L., Johnson, S. G., Lee, M. T., Williams, M. S., Klein, T. E., et al. (2014). Clinical Pharmacogenetics Implementation Consortium (CPIC) guidelines for IFNL3 (IL28B) genotype and PEG interferon-α-based regimens. Clin. Pharmacol. Ther. 95 (2), 141–146. doi:10.1038/clpt.2013.203
Ningtyas, D., Thomson, R. J., Tarlac, V., Nagaraj, S. H., Hoy, W., Mathews, J. D., et al. (2020). Analysis of the F2LR3 (PAR4) single nucleotide polymorphism (rs773902) in an indigenous Australian population. Front. Genet. 11, 432. doi:10.3389/fgene.2020.00432
Numanagic, I., Malikic, S., Ford, M., Qin, X., Toji, L., Radovich, M., et al. (2018). Allelic decomposition and exact genotyping of highly polymorphic and structurally variant genes. Nat. Commun. 9 (1), 828. doi:10.1038/s41467-018-03273-1
Onakpoya, I. J., Heneghan, C. J., and Aronson, J. K. (2016). Post-marketing withdrawal of 462 medicinal products because of adverse drug reactions: A systematic review of the world literature. Bmc Med. 14, 10. doi:10.1186/s12916-016-0553-2
PharmGKB (2022). PharmGKB [online]. Available: https://www.pharmgkb.org/(Accessed July 10, 2022).
PharmVar (2022). Pharmacogene variation consortium [online]. Available: https://www.pharmvar.org/(Accessed July 10, 2022).
Pirmohamed, M., James, S., Meakin, S., Green, C., Scott, A. K., Walley, T. J., et al. (2004). Adverse drug reactions as cause of admission to hospital: Prospective analysis of 18,820 patients. Bmj-British Med. J. 329 (7456), 15–19. doi:10.1136/bmj.329.7456.15
Polasek, T. M., Mina, K., and Suthers, G. (2019). Pharmacogenomics in general practice the time has come. Aust. J. General Pract. 48 (3), 100–105. doi:10.31128/AJGP-10-18-4733
R: A language and environment for statistical computing (2021). Vienna, Austria: R Foundation for Statistical Computing. https://www.R-project.org/.
Relling, M. V., Schwab, M., Whirl-Carrillo, M., Suarez-Kurtz, G., Pui, C. H., Stein, C. M., et al. (2019). Clinical pharmacogenetics implementation consortium guideline for thiopurine dosing based on TPMT and NUDT15 genotypes: 2018 update. Clin. Pharmacol. Ther. 105 (5), 1095–1105. doi:10.1002/cpt.1304
Rosenberg, N. A., Mahajan, S., Ramachandran, S., Zhao, C., Pritchard, J. K., and Feldman, M. W. (2005). Clines, clusters, and the effect of study design on the inference of human population structure. PLoS Genet. 1 (6), e70. doi:10.1371/journal.pgen.0010070
Sahana, S., Bhoyar, R. C., Sivadas, A., Jain, A., Imran, M., Rophina, M., et al. (2022). Pharmacogenomic landscape of Indian population using whole genomes. Cts-Clinical Transl. Sci. 15, 866–877. doi:10.1111/cts.13153
Saito, Y., Stamp, L. K., Caudle, K. E., Hershfield, M. S., McDonagh, E. M., Callaghan, J. T., et al. (2016). Clinical pharmacogenetics implementation consortium (CPIC) guidelines for human leukocyte antigen B (HLA-B) genotype and allopurinol dosing: 2015 update. Clin. Pharmacol. Ther. 99 (1), 36–37. doi:10.1002/cpt.161
Schwarz, U. I., Ritchie, M. D., Bradford, Y., Li, C., Dudek, S. M., Frye-Anderson, A., et al. (2008). Genetic determinants of response to warfarin during initial anticoagulation. N. Engl. J. Med. 358 (10), 999–1008. doi:10.1056/NEJMoa0708078
Sirugo, G., Williams, S. M., and Tishkoff, S. A. (2019). The missing diversity in human genetic studies. Cell. 177 (1), 26–31. doi:10.1016/j.cell.2019.02.048
Somogyi, A. A., Barratt, D. T., Phillips, E. J., Moore, K., Ilyas, F., and Gabb, G. M. (2019). High and variable population prevalence of HLA-B*56:02 in indigenous Australians and relation to phenytoin-associated drug reaction with eosinophilia and systemic symptoms. Br. J. Clin. Pharmacol. 85 (9), 2163–2169. doi:10.1111/bcp.14025
Stenson, P. D., Ball, E. V., Mort, M., Phillips, A. D., Shiel, J. A., Thomas, N. S., et al. (2003). Human Gene Mutation Database (HGMD): 2003 update. Hum Mutat 21 (6), 577–581. doi:10.1002/humu.10212
Szustakowski, J. D., Balasubramanian, S., Kvikstad, E., Khalid, S., Bronson, P. G., Sasson, A., et al. (2021). Advancing human genetics research and drug discovery through exome sequencing of the UK Biobank. Nat Genet 53 (7), 942–948. doi:10.1038/s41588-021-00885-0
Taylor, C., Crosby, I., Yip, V., Maguire, P., Pirmohamed, M., and Turner, R. M. (2020). A review of the important role of CYP2D6 in pharmacogenomics. Genes. (Basel) 11 (11), 1295. doi:10.3390/genes11111295
Theken, K. N., Lee, C. R., Gong, L., Caudle, K. E., Formea, C. M., Gaedigk, A., et al. (2020). Clinical pharmacogenetics implementation consortium guideline (CPIC) for CYP2C9 and nonsteroidal anti-inflammatory drugs. Clin. Pharmacol. Ther. 108 (2), 191–200. doi:10.1002/cpt.1830
Thomson, R. J., McMorran, B., Hoy, W., Jose, M., Whittock, L., Thornton, T., et al. (2019). New genetic loci associated with chronic kidney disease in an indigenous Australian population. Front. Genet. 10, 330. doi:10.3389/fgene.2019.00330
Thynne, T., and Gabb, G. M. (2016). Therapeutic drug safety for indigenous Australians: How do we close the gap? Med. J. Aust. 204 (1), 16–17. doi:10.5694/mja15.00937
Twesigomwe, D., Drogemoller, B. I., Wright, G. E. B., Siddiqui, A., da Rocha, J., Lombard, Z., et al. (2021). StellarPGx: A nextflow pipeline for calling star alleles in cytochrome P450 genes. Clin. Pharmacol. Ther. 110 (3), 741–749. doi:10.1002/cpt.2173
Twesigomwe, D., Wright, G. E. B., Drogemoller, B. I., da Rocha, J., Lombard, Z., and Hazelhurst, S. (2020). A systematic comparison of pharmacogene star allele calling bioinformatics algorithms: A focus on CYP2D6 genotyping. NPJ Genom Med. 5, 30. doi:10.1038/s41525-020-0135-2
U. S. Food and Drug Administration (2022). U. S. Food and drug administration. USA: FDA. Available: https://www.fda.gov/(Accessed July 10, 2022).
Wang, K., Li, M., and Hakonarson, H. (2010). Annovar: Functional annotation of genetic variants from high-throughput sequencing data. Nucleic Acids Res. 38 (16), e164. doi:10.1093/nar/gkq603
Zhou, S. F. (2009). Polymorphism of human cytochrome P450 2D6 and its clinical significance: Part II. Clin. Pharmacokinet. 48 (12), 761–804. doi:10.2165/11318070-000000000-00000
Zhou, Y., and Lauschke, V. M. (2018). Comprehensive overview of the pharmacogenetic diversity in Ashkenazi Jews. J. Med. Genet. 55 (9), 617–627. doi:10.1136/jmedgenet-2018-105429
Keywords: pharmacogenomics, pharmacogenetics, adverse drug reactions, population genomics, Indigenous Australians, variable drug response
Citation: Samarasinghe SR, Hoy W, Jadhao S, McMorran BJ, Guchelaar H-J and Nagaraj SH (2023) The pharmacogenomic landscape of an Indigenous Australian population. Front. Pharmacol. 14:1180640. doi: 10.3389/fphar.2023.1180640
Received: 06 March 2023; Accepted: 07 April 2023;
Published: 22 May 2023.
Edited by:
Marcelo Rizzatti Luizon, Federal University of Minas Gerais, BrazilReviewed by:
Simran D. S. Maggo, Children’s Hospital of Los Angeles, United StatesIngrid Fricke-Galindo, Instituto Nacional de Enfermedades Respiratorias-México (INER), Mexico
Copyright © 2023 Samarasinghe, Hoy, Jadhao, McMorran, Guchelaar and Nagaraj. This is an open-access article distributed under the terms of the Creative Commons Attribution License (CC BY). The use, distribution or reproduction in other forums is permitted, provided the original author(s) and the copyright owner(s) are credited and that the original publication in this journal is cited, in accordance with accepted academic practice. No use, distribution or reproduction is permitted which does not comply with these terms.
*Correspondence: Shivashankar H. Nagaraj, shiv.nagaraj@qut.edu.au