- 1School of Traditional Chinese Medicine, Beijing University of Chinese Medicine, Beijing, China
- 2Guangzhou Key Laboratory of Formula-Pattern of Traditional Chinese Medicine, School of Traditional Chinese Medicine, Jinan University, Guangzhou, China
- 3School of Life Sciences, Beijing University of Chinese Medicine, Beijing, China
Background: Chronic fatigue syndrome (CFS) is characterized by significant and persistent fatigue. Ginseng is a traditional anti-fatigue Chinese medicine with a long history in Asia, as demonstrated by clinical and experimental studies. Ginsenoside Rg1 is mainly derived from ginseng, and its anti-fatigue metabolic mechanism has not been thoroughly explored.
Methods: We performed non-targeted metabolomics of rat serum using LC-MS and multivariate data analysis to identify potential biomarkers and metabolic pathways. In addition, we implemented network pharmacological analysis to reveal the potential target of ginsenoside Rg1 in CFS rats. The expression levels of target proteins were measured by PCR and Western blotting.
Results: Metabolomics analysis confirmed metabolic disorders in the serum of CFS rats. Ginsenoside Rg1 can regulate metabolic pathways to reverse metabolic biases in CFS rats. We found a total of 34 biomarkers, including key markers Taurine and Mannose 6-phosphate. AKT1, VEGFA and EGFR were identified as anti-fatigue targets of ginsenoside Rg1 using network pharmacological analysis. Finally, biological analysis showed that ginsenoside Rg1 was able to down-regulate the expression of EGFR.
Conclusion: Our results suggest ginsenoside Rg1 has an anti-fatigue effect, impacting the metabolism of Taurine and Mannose 6-phosphate through EGFR regulation. This demonstrates ginsenoside Rg1 is a promising alternative treatment for patients presenting with chronic fatigue syndrome.
1 Introduction
Chronic fatigue syndrome (CFS) is a common (0.006%–3%), severely disabling disorder characterized by long-term extreme fatigue that persists even after resting (Nguyen et al., 2019; Moore et al., 2021). CFS is accompanied by depression, concentration difficulty and memory loss (Cvejic et al., 2016; Chaves-Filho et al., 2019). An estimated 1–5 million people present with CFS in Europe every year, with an estimated annual cost of approximately €40 billion in health expenses (Nacul et al., 2021); while in the United States CFS affects 1–2.5 million people yearly and costs between $1.7∼$24 billion (Bested and Marshall, 2015), compared to 44.71 ± 6.10 cases/100,000 people in South Korea (Lim et al., 2021). In China, a previous cross-sectional study found CFS is prevalent among adolescents (Shi et al., 2018).
At present, CFS’s etiology remains unknown, and there are no effective treatments available (Sandler and Lloyd, 2020), with deficient and unspecific diagnostic criteria further preventing appropriate approaches. This makes it imperative to increase the efforts for discovering biomarkers to aid diagnosis and include Chinese medicine has as a potential area for therapy.
Spleen deficiency represents the core pathogenesis of CFS (Geng and Wang, 2012) and can be treated with ginseng, a classic herbal prescription (Ma et al., 2021) that contains ginsenosides as main active components, of which Rg1, a triterpenoid saponin, is the most abundant (Mohanan et al., 2018; Yousuf et al., 2022). In addition, Rg1 presents anti-fatigue effects, as demonstrated by an increase in swimming, fight, and rest times in CFS model rats, which are associated with an increase in serum IgA, IgG, IgM, IFN-β, IFN-γ, T-AOC and Ache (He et al., 2020). Li et al. (2022) found that Panax notoginseng saponin R1 can be efficiently transformed into ginsenoside Rg1 to enhance anti-fatigue effects. CFS is also associated with several metabolic disorders, including energy, amino acids, nucleotides, nitrogen, hormones, lipids and neurotransmitter-related pathways (Armstrong et al., 2014; Nagy-Szakal et al., 2018). However, it remains unclear whether ginsenoside Rg1 can regulate these metabolic disorders in CFS rats.
Traditional Chinese medicine has played an important role in health protection and disease treatment for thousands of years, and is becoming gradually recognized by the international community, as shown by the development of metabolomics, an emerging systems biology technology whose core idea is similar to the holistic approach of traditional Chinese medicine (Wang et al., 2015). Moreover, network analysis explores the associations between drugs, targets, and diseases through network information, whereby combining it with metabolomics can provide insights into the complex interrelationships between biomarkers and disease.
2 Materials and methods
2.1 Animals, drug administration and sample collection
We purchased 32 male Sprague-Dawley rats from the Beijing Vital River Laboratory Animal Technology Co., Ltd. [animal license No. SCXK (Beijing) 2016–0006]. The rats were kept under controlled environmental conditions (room temperature 22°C ± 2°C, 12-h light/dark cycle) with free access to standard food and water. All experiments were performed according to the EU (Directive 2010/63/EU) ethical guidelines and were approved by the Animal Care and Therapy Ethics Committee of the Beijing University of Chinese Medicine (BUCM-4-2019030402–1036).
After 1 week of adaptive feeding, all rats were randomly divided into four groups (n = 8 per group), including the normal Control group (Control), the model group (CFS), the model + positive control group (CFS+ Oryzanol&VB1), and the model + ginsenoside Rg1 group (CFS + Rg1). Starting from week 3, all groups were given intragastric administration 30 min before modeling every day.
The rats in each group were given continuous gavage for 2 weeks, once a day, and received the following treatments: 1) Control: intragastric administration of double distilled water (10 ml/kg body weight); 2) CFS: intragastric administration of double distilled water (10 ml/kg body weight); 3) CFS+ Oryzanol&VB1 group: gavage glutamine and vitamin B1, (3.15 mg/kg/d, 10 ml/kg body weight) (Xu et al., 2019); 4) CFS + Rg1 group: intragastric administration of ginsenoside Rg1 (50 mg/kg/d, 10 ml/kg body weight) (Feng et al., 2010; Heinrich et al., 2020).
After performing behavioral tests, we anesthetized the animals using isoflurane. The hippocampus and prefrontal cortex were stripped and quickly preserved in liquid nitrogen. Blood was collected through the abdominal aorta and serum was separated by centrifugation (3500 rpm, 10 min, 4°C). Serum and brain tissue were stored at -80°C until analyzed.
2.2 Chemicals and reagents
Ginsenoside Rg1 was purchased from Chengdu DeSiTe Bio-Technology Co., Ltd. with the following specifications: 5 g/bottle (HPLC ≥ 98%, CAS No.22427-39-0). Guweisu tablets were purchased from Beijing Zhongxin Pharmaceutical Co., Ltd. with the following specifications: 10mg/tablet (No.H13020683). Vitamin B1 tablets were purchased from Tianjin Feiying Yuchuan Pharmaceutical Co., Ltd. with the following specifications: 10 mg/tablet (No.12020592). D-xylose was purchased from BioRuler.
UPLC Methanol, Acetonitrile, and ultra-pure water were purchased from Fisher Chemical (Fair Lawn, United States). Uplc-grade FormicAcid was purchased from CNW (Shanghai, China). UPLC Grade 2-Propanol was purchased from Merck (Darmstadt, Germany). 2-Chloro-L-Phenylalanine (≥98%) was obtained from Adamas-beta (Shanghai, China).
Bicinchoninic acid (BCA) protein quantitative detection Kit, SDS-PAGE Gel Preparation Kit, Radio-Immunoprecipitation Assay (RIPA) Lysate, and enhanced chemiluminescence (ECL) were purchased from Servicebio (Wuhan, China). GAPDH (GB15002) was purchased from Servicebio (Wuhan, China). AKT1 (ab81283), VEGFA (ab214424), and EGFR (ab52894) were purchased from Abcam (Shanghai, China).
2.3 Chronic fatigue syndrome model establishment
A multi-factor modeling method was used to simulate CFS pathogenesis (Shao et al., 2017): 1) Load-weighted forced swimming: Rats in the modeling group swam in a transparent toughened plastic bucket (diameter 20 × height 50 cm) for 10 min every day for 28 days. The water temperature of the swimming pool was controlled at 23°C ± 2°C. A small artery clip was attached to the lead wire about 5% of the body weight of the rat, and then the weight was placed on the back hair of each rat. 2) Restriction: The rats in the model group were bound to the wooden restriction frame (22 × 10 × 2 cm in length and 10 × 2 cm in thickness) for 3 h every day, respectively, and subjected to chronic restriction stress for 4 weeks. The Control group did not participate in the above two modeling methods and was fed normally. The experimental procedure is shown in Figure 1A.
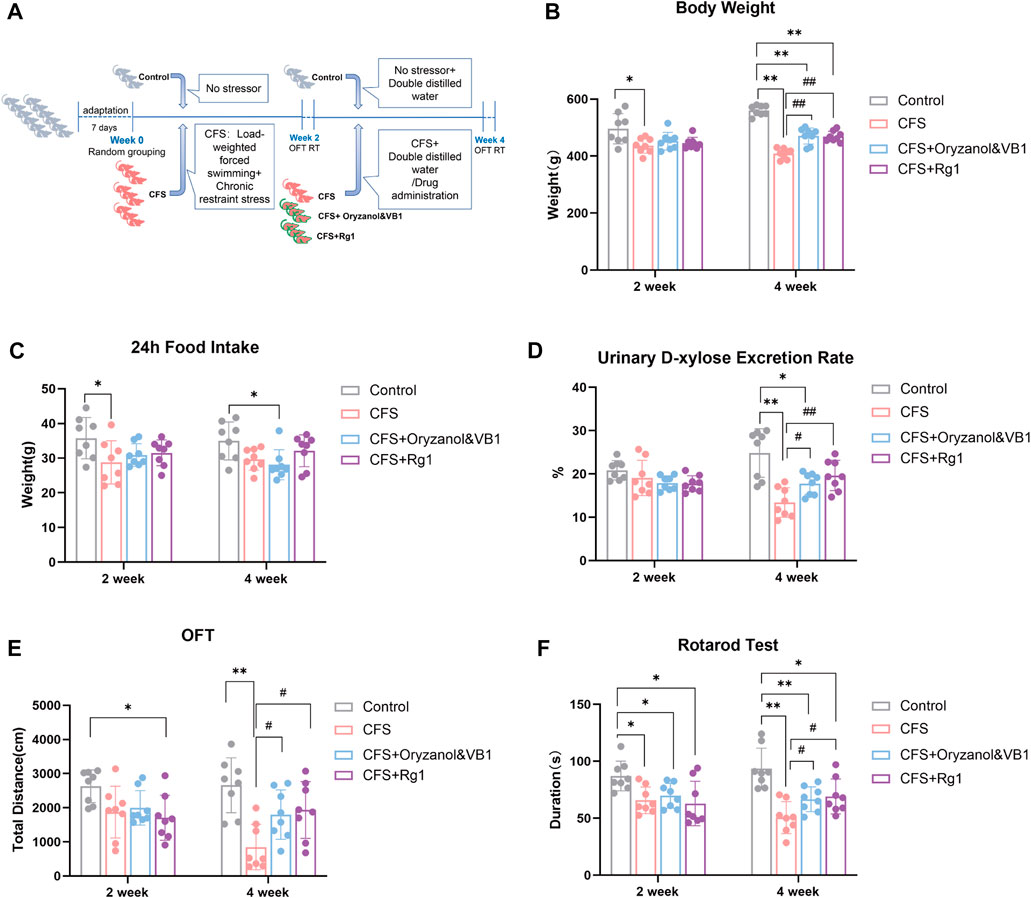
FIGURE 1. (A) Experimental procedure implemented for a CFS rat model. (B) Body weight changes in the four rat groups. (C) 24-h food intake of the four rat groups. (D) 24-h urinary D-xylose excretion rate in the four rat groups. (E) Total travel distance of the four rat groups in the Open Field Test. (F) Time spent on the rod in the four rat groups (Rotarod Test). Data are shown as the mean ± standard deviation, n = 8. *p < 0.05, **p < 0.01 versus control group; #p < 0.05, ##p < 0.01 versus CFS group.
2.4 Evaluation of the CFS model
Body weight, 24-h food intake, urine D-xylose and behavioral tests (including the open field and rotarod tests) were performed 2 and 4 weeks after modeling for evaluation. In the open field test, the EthoVision 3.0 behavioral device of the Noldus Company (Netherlands) was used to analyze videos and calculate the 5-min total distance of spontaneous movement of each rat group. A rat rotating rod fatigue instrument (Anhui Zhenghua Biological Instrument Equipment Co., Ltd. ZH-300B) was used to measure the time during which rats were able to sustain a constant 30rpm speed. The upper limit of the recording time was set to 5 min.
2.5 LC-MS-based serum metabolomics
2.5.1 Sample preparation
100 μL of serum samples were collected in a 2 ml centrifuge tube. The metabolites were extracted from 400 μL of extract solution (methanol: water = 4:1 (v:v)) containing 0.02 mg/ml internal standard (L-2-chlorophenyl alanine). The sample preparation process is described in a previous study (Lei et al., 2022). Equal amounts of the mixture were extracted from all samples and used as QC samples for LC-MS.
2.5.2 Untargeted LC-MS analysis
We used Thermo’s ultra-high-performance liquid chromatography-tandem time-of-flight mass spectrometry UHPLC-Q Exactive HF-X system as the instrument platform. A total of 2 μL samples were separated using an HSS T3 chromatographic column (100 mm × 2.1 mm i. d. 1.8 µm) and then detected by mass spectrometry. The mobile phase A consisted of 95% water and 5% acetonitrile (containing 0.1% formic acid). The mobile phase B consisted of 47.5% acetonitrile, 47.5% isopropyl alcohol, and 5% water (containing 0.1% formic acid). The column temperature was set to 40 °C. The positive and negative ion scanning modes were used for mass spectrum signal acquisition.
2.5.3 Data processing
LC-MS raw data was imported into Progenesis QI (Waters Corporation, Milford, United States) for baseline filtering, peak identification, integration, retention time correction, and peak alignment. This allowed us to obtain a data matrix of the retention time, mass/charge ratio, and peak intensity. We normalized the data matrix for subsequent analysis. The metabolite information was obtained by matching MS and MS/MS mass spectrometry information with the metabolic public databases HMDB (https://hmdb.ca/) and Metlin (https://metlin.scripps.edu/).
2.5.4 Metabolomic data analysis
The pre-processed data was uploaded to the Majorbio Cloud Platform (http://www.majorbio.com/) for data analysis. The R software package ropls (Version 1.6.2) was used for performing principal component analysis (PCA) and orthogonal least partial square discriminant analysis (OPLS-DA). The stability of the model was evaluated using seven cyclic interaction validations. We also performed a Student’s t-test analysis. The selection of differential metabolites was determined based on the variable weight value (VIP) obtained by the OPLS-DA model and the p-value of the student’s t-test. Metabolites with VIP > 1.0 and p < 0.05 were selected as metabolic markers. We use the KEGG database (https://www.kegg.jp/kegg/pathway.html) for uncovering metabolic pathways and identify differences in associated metabolites, with p < 0.05 used as the standard. The Python software package scipy. stats was used for pathway enrichment analysis, and the most relevant biological pathways were extracted using Fisher’s exact test.
2.6 Network analysis
2.6.1 Target prediction
The BATMAN-TCM (http://bionet.ncpsb.org/batman-tcm/) and SwissTargetPrediction (http://www.swisstargetprediction.ch/) databases were used to obtain information on the targets of ginsenoside Rg1.
We identified potential targets by searching CFS keywords on public databases, including Genecards (http://www.genecards.org/), OMIM (https://www.omim.org/), and DRUGBANK (https://go.drugbank.com/).
Differential metabolites were entered into the MetScape database to identify target proteins interacting with these metabolites.
The targets associated with CFS were standardized through the UniProt knowledge Base (http://www.uniprot.org/), converting all retrieved targets into official genetic symbols to facilitate downstream data analysis.
A Venn diagram was drawn using DeepVenn (http://www.deepvenn.com/) to show the common targets of ginsenoside Rg1, the differential metabolites, and CFS-related targets. These genes were considered as potential targets of ginsenoside Rg1 for the treatment of CFS.
2.6.2 Network construction and enrichment analysis
A large number of studies showed proteins exert biological activity through protein-protein interactions. We submitted potential target genes to the Interaction Gene Retrieval Tool (http://string-db.org/) and performed Protein-Protein Interaction Network (PPI) analysis. PPI networks were used to analyze core targets of ginsenoside Rg1 in the CFS-treatment using Cytoscape 3.7.1.
We used OmicShare Tools (https://www.omicshare.com/tools/) for GO and KEGG enrichment analyses to obtain the biological functions and related pathways of ginsenoside Rg1 as potential targets for CFS treatment. The correlation pathways with p < 0.05 were considered as statistically significant.
2.7 Experimental validation
2.7.1 Quantitative real-time PCR
Total RNA in the hippocampus (Hip) and prefrontal cortex (PFC) was extracted with an RNA extraction solution (Servicebio, China). Reverse transcription was performed with a first-strand cDNA synthesis kit (Servicebio, China) following manufacturer’s guidelines. The expression levels were determined using real-time PCR with SYBR Green Mix (Servicebio, China). Relative mRNA expression was calculated using the 2−ΔΔCT method. GAPDH was used as a normalization control for mRNA levels. The primer sequences are shown in Table 1.
2.7.2 Western blot
RIPA lysate was added to the tissues, crushed by ultrasound, and centrifuged at room temperature for 10 min (12,000 rpm, 4°C). After this, we collected the supernatant, which was considered as the total protein solution. The BCA kit was used to measure the concentration of the protein solution. We added 5× protein loading buffer and denatured the protein in a boiling water bath for 5 min. The same amount of protein solution was subjected to sodium dodecyl sulfate-polyacrylamide gel electrophoresis (SDS-PAGE), after which the gel was transferred to the polyvinylidene fluoride (PVDF) membrane. A 5% skim milk solution containing TBS + Tween (TBST) was kept at room temperature for 30 min and added to the primary antibody at 4°C for overnight incubation in a shak (GAPDH (1:2000), AKT1 (1:1000), VEGFA (1:1000), EGFR (1:1000)). After rinsing with phosphate buffered solution tween (PBST) for 3 × 5 min, the membrane was placed in an horseradish peroxidase (HRP) labeled secondary antibody (1:5000) and incubated at room temperature for 1 h. After rinsing with PBST for 3 × 5 min, the gel imaging system was used for image acquisition. The Alpha Innotech software was used to calculate and analyze the gray scale of protein bands.
2.8 Statistical analysis
Statistical analysis was performed using SPSS (version 20.0) and GraphPad Prism (version 9.0). All data are expressed as the mean ± standard deviation (
3 Results
3.1 Effects of CFS modeling on body weight, food intake, and urinary D-xylose excretion rate in rats
3.1.1 Weight
The body weight of rats in each group is shown in Figure 1B. After 2 weeks of modeling, the rats in the CFS group had lower weight compared to the Control group (p = 0.0443). There were no significant differences among model groups. After 4 weeks of modeling, the body weight of the three modeling groups decreased significantly compared with the Control group (p < 0.0001). Finally, rat weight in both Oryzanol&VB1 and Rg1 groups recovered significantly compared to the CFS group (p = 0.0005, p < 0.0001).
3.1.2 Food intake
The 24-h food intake of each group is shown in Figure 1C. After 2 weeks, the food intake of rats in the CFS group was lower than in the Control group (p = 0.0237). We found no significant differences among the model groups. After 4 weeks, food intake decreased in the Oryzanol&VB1 group compared to Control (p = 0.0240). No significant differences were found between the CFS, the Oryzanol&VB-1 and the Rg1 groups.
3.1.3 Urinary D-xylose excretion rate
The 24-h urinary D-xylose excretion rate of rats in each group is shown in Figure 1D. We found no differences between groups after 2 weeks. However, after 4 weeks of modelling, the urinary D-xylose-excretion rate in the CFS and Oryzanol&VB-1 groups was significantly decreased compared to the Control group (p = 0.0011, p = 0.0228), and a significant recovery occurred in the Oryzanol&VB1 and Rg1 groups compared to CFS (p = 0.0230, p = 0.0054).
These results show that CFS modeling produces significant effects on body weight and the urinary D-xylose excretion rate of rats, but no significant differences in food intake. We also note that body weight and urinary D-xylose excretion rate were further decreased in the CFS group over the course of the last 2 weeks of treatment. Crucially, ginsenoside Rg1 intervention could significantly reverse this course, suggesting it is able to improve gastrointestinal absorption.
3.2 CFS modeling, spontaneous activity and rotarod test
3.2.1 Open field test
The 5-min total movement distance of each group is shown in Figure 1E. Rats in the Rg1 group moved less than those in the Control group after 2 weeks (p = 0.0187), but there were no significant differences among model groups. After 4 weeks, the total movement distance of rats in the CFS group was significantly decreased compared to the Control group (p = 0.0007), with a recovery observed in the Oryzanol&VB1 and Rg1 groups (p = 0.0296, p = 0.0229).
3.2.2 Rotarod test
The time spent on the rod is shown in Figure 1F. Duration decreased in all model groups after 2 weeks (p = 0.0105, p = 0.0306, p = 0.0323), with no significant differences among them. These differences to the Control group became significant after 4 weeks (p = 0.0004, p = 0.0097, p = 0.0286), with both Oryzanol&VB1 and Rg1 groups recovering (p = 0.0421, p = 0.0440).
These results show CFS modeling had a significant effect on rat spontaneous activity and muscle fatigue. Over the course of the last 2 weeks of modeling, the groups treated with ginsenoside Rg1 recovered significantly, showing its significant anti-fatigue effect.
3.3 Metabolomics analysis of serum samples
In order to study changes in endogenous serum metabolites in CFS rats and reveal the mechanisms of ginsenoside Rg1-mediated treatment, we comprehensively scanned serum metabolites of Control, CFS and CFS + Rg1 groups using the UHPLC-QExactiveHF-X system. Principal component analysis (PCA) showed significant differences between these groups. QC analysis showed obvious sample clustering, indicating the analytical method is stable and repeatable. These results are illustrated in Figure 2.
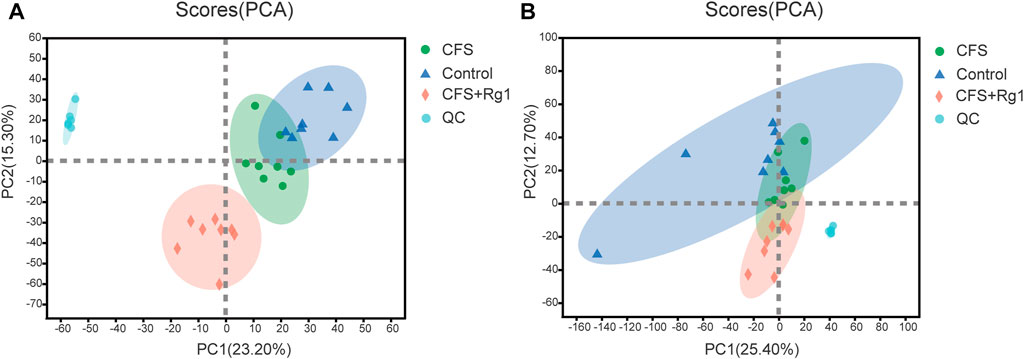
FIGURE 2. PCA scores for Control (blue), CFS (green) and CFS + Rg1 (pink) groups in positive and negative ion modes (A, B), n = 8.
OPLS-DA was further used to screen for potential biomarkers. As shown in Figures 3A–D, samples from the Control and the CFS groups were significantly different. The R2X, R2Y and Q2 values of permutation tests applied in the positive ion mode were 0.259, 0.99 and 0.739, respectively; and 0.567, 0.998 and 0.709, respectively, in the negative ion mode. The CFS and the CFS + Rg1 groups were also clearly different. In these groups, the R2X, R2Y and Q2 of permutation tests in the positive ion mode were 0.308, 0.993 and 0.84, respectively; and 0.383, 0.999 and 0.766, respectively, in the negative ion mode. These results are shown in Figures 3E–H and indicate the model has good explanatory and predictive abilities, with no overfitting present.
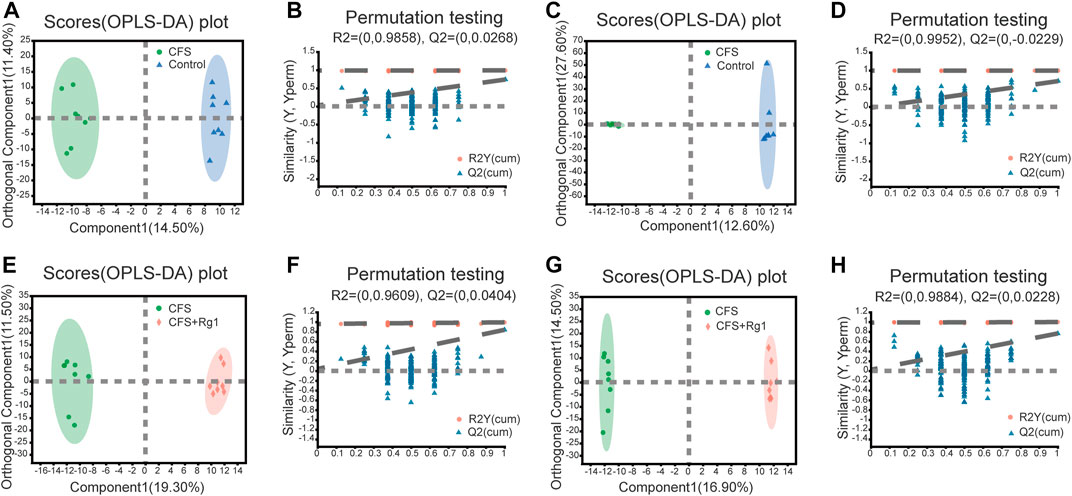
FIGURE 3. OPLS-DA and permutation tests in the positive (A, B) and negative (C, D) ion modes of Control and CFS groups, n = 8. OPLS-DA and permutation tests in positive (E, F) and negative (G, H) ion modes in CFS and CFS + Rg1 groups, n = 8.
We found a total of 34 differential metabolites that are potential biomarkers using the screening conditions VIP > 1.0 and p < 0.05 (Table 2). Of these, 12 and 22 metabolites were increased and decreased, respectively, in the CFS group compared to Control. Ginsenoside Rg1 treatment could restore Polyoxyethylene 40 monostearate, Mannose 6-phosphate, Taurine, Petasitenine and 5,6,7-trihydroxy-2-[7-hydroxy-2-methyl-2-(4-methylpent-3-en-1-yl)-2H-chromen-6-yl]-3,4-dihydro-2H-1-benzopyran-4-one levels in CFS rats, while lowering 3,4-Dehydrothiomorpholine-3-carboxylate, P-Tolyl Sulfate, MEDICA 16, {4-[(1Z)-2-hydroxy-3-oxobut-1-en-1-yl]-2-methoxyphenyl}oxidanesulfonic acid, (S)-5′-Deoxy-5'-(methylsulfinyl)adenosine, Norophthalmic acid, and Alpha-CEHC. ROC curve analysis showed good diagnostic effect for these metabolites (AUC > 0.7; Figure 4, Figure 5).
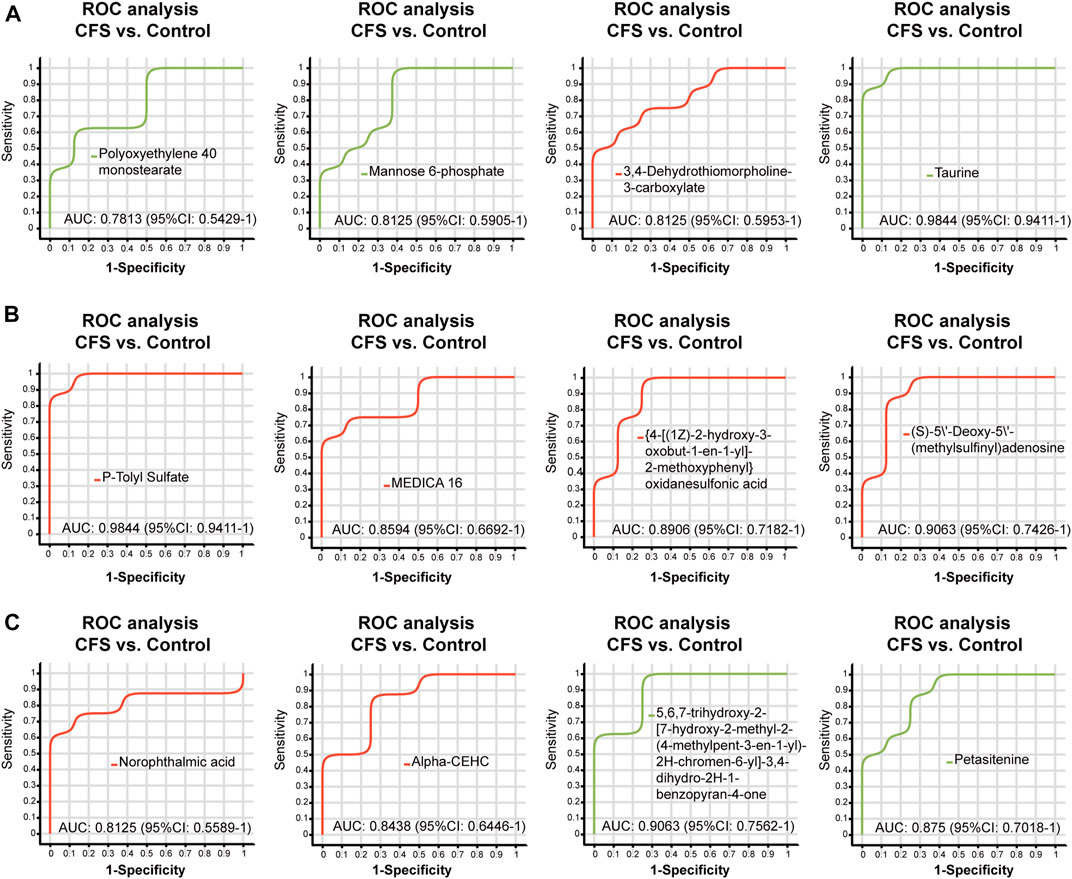
FIGURE 4. ROC curve analysis of differential metabolites (A–C) regulated by ginsenoside Rg1 (CFS vs. Control). The closer the AUC is to 1, the better the diagnostic prediction. AUC has low accuracy between 0.5 and 0.7, and high accuracy above 0.7.
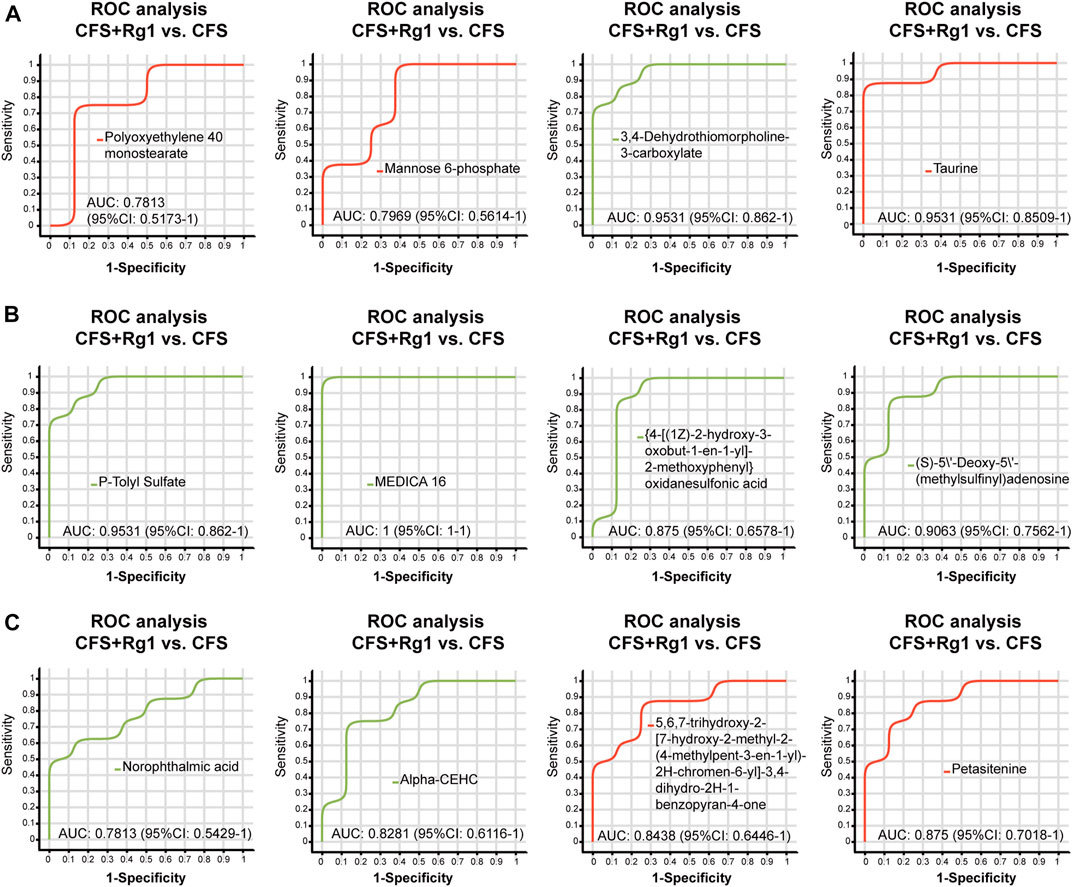
FIGURE 5. ROC curve analysis of differential metabolites (A–C) regulated by ginsenoside Rg1 (CFS + Rg1 vs. CFS). The closer the AUC is to 1, the better the diagnostic prediction. AUC has low accuracy between 0.5 and 0.7, and high accuracy above 0.7.
3.4 Potential biomarker identification and metabolic pathway analysis
KEGG pathway enrichment analysis of differential metabolites was performed using the Majorbio Cloud Platform. We identified nine pathways using p < 0.05 (Figure 6A) associated with the metabolites L-Aspartic Acid, Taurine, Mannose 6-phosphate, LysoPC(18:0), PC(17:0/0:0), and PC(18:0/0:0). Five of these pathways are particularly associated with ginsenoside Rg1 treatment of CFS, including Taurine and hypotaurine metabolism; Arginine biosynthesis; Ether lipid metabolism; Alanine, aspartate and glutamate metabolism; and Pantothenate and CoA biosynthesis. The Lipid metabolism category included the highest number of differential metabolites (Figure 6B).
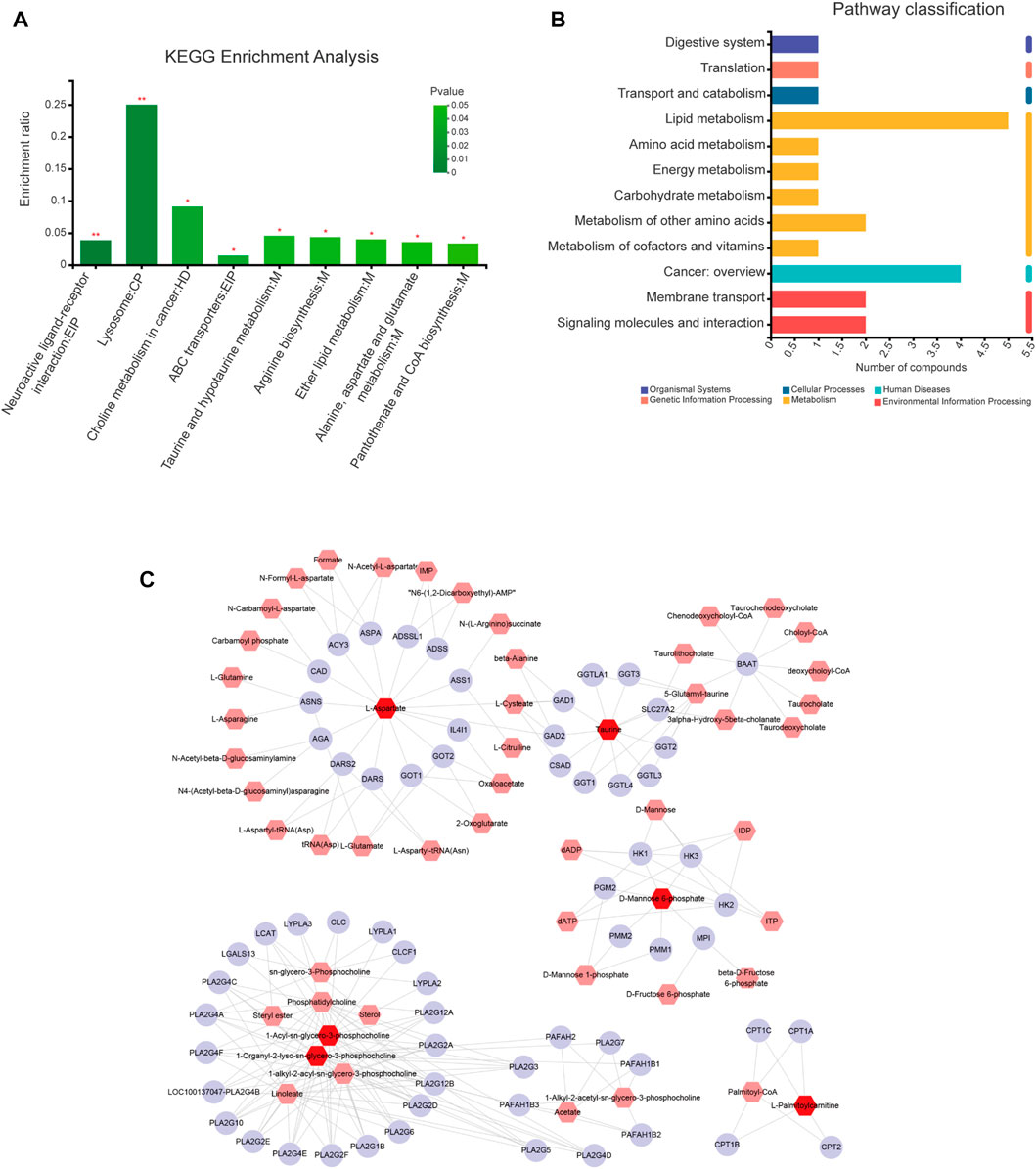
FIGURE 6. Pathway and network analyses of differential metabolites. (A) KEGG enrichment analysis of differential metabolites. (B) KEGG classification analysis of differential metabolites. (C) “Metabolite-gene” network for anti-fatigue effects of ginsenoside Rg1. Red, pink, and purple nodes represent differential metabolites, interacting metabolites, and interacting proteins, respectively. The edges represent biochemical reactions.
The differential metabolites were imported into the MetScape database to construct a “Compound Gene” network (Figure 6C) consisting of 64 target proteins. The related differential metabolites included were L-Aspartic Acid, Taurine, Mannose 6-phosphate, LysoPC(18:0) (1-Organyl-2-lyso-sn-glycero-3-phosphocholine), PC(17:0/0:0) (1-Acyl-sn-glycero-3-phosphocholine), and Palmitoyl-L-carnitine. Our results demonstrate that Taurine and Mannose 6-phosphate are key metabolic markers of ginsenoside Rg1 treatment of CFS.
3.5 Network analysis
3.5.1 Ginsenoside Rg1 acts on potential CFS targets
A total of 107 ginsenoside Rg1 targets were obtained through the BATMAN-TCM and SwissTargetPrediction databases. In addition, a total of 3796 disease targets of CFS were obtained from the Genecards, OMIM, and DRUGBANK databases. The differential metabolites were imported into the MetScape database to construct a “Compound-Gene” network, allowing us to identify a total of 64 target proteins (Figure 6C).
There were 72 identical targets of CFS and ginsenoside Rg1, and 15 identical targets of CFS and differential metabolites, totaling 87 potential ginsenoside Rg1 targets for CFS treatment (Figure 7A).
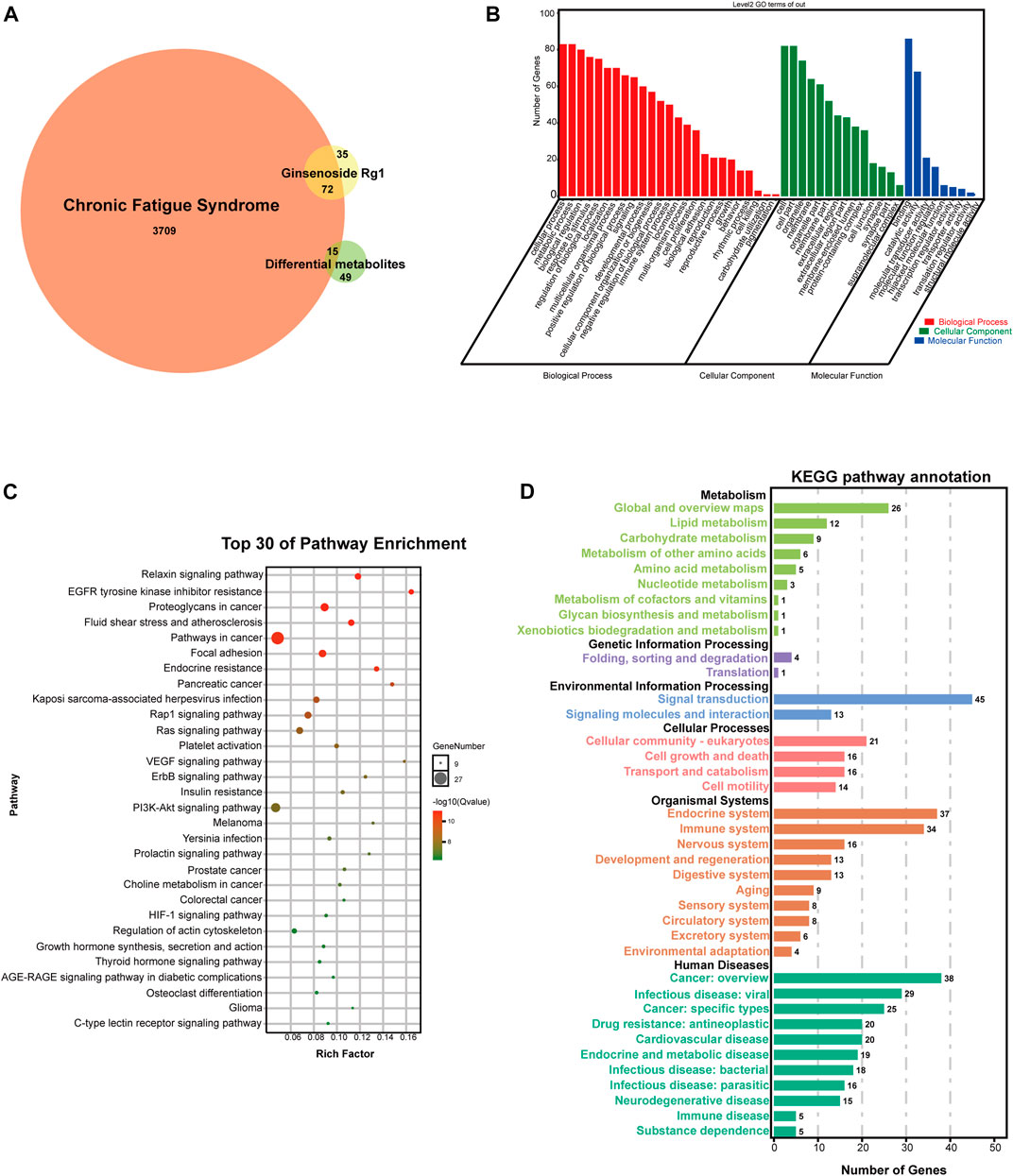
FIGURE 7. Network analysis of potential targets. (A) VENN diagram of potential targets. (B) GO analysis of potential targets. (C) KEGG pathway enrichment analysis of potential targets. (D) KEGG pathway annotation of potential targets.
3.5.2 Ginsenoside Rg1 pathways of action
We used the OmicShare cloud platform to perform GO enrichment analysis and elucidate the biological associations of potential targets. We identified a total of 48 GO terms for ginsenoside Rg1, 25 for biological processes (BP), 14 for molecular functions (MF), and nine for cellular components (CC) (Figure 7B). BP results predicted the involvement of a high number of genes in cellular process, metabolic process, biological regulation, response to stimulus, localization, multicellular organismal process, positive developmental process, negative regulation of biological process, immune system process, locomotion, multi-organism proces, cell proliferation, and biological adhesion. In addition, CC and MF results revealed that the anti-fatigue effects of ginsenoside Rg1 were mainly associated with cell, organelle, membrane, extracellular region, membrane-enclosed lumen, protein-containing complex, cell junction, synapse, supramolecular complex, catalytic activity, molecular transducer activity, molecular function regulator, hijacked molecular function, transcription regulator activity.
We also performed KEGG analysis to evaluate the functional pathways associated with ginsenoside Rg1 treatment of CFS. Figure 7C shows the top 30 signaling pathways obtained from the KEGG enrichment, while Figure 7D shows KEGG pathway annotation. The main therapeutic pathways associated with ginsenoside Rg1 treatment are EGFR tyrosine kinase inhibitor resistance, Relaxin signaling pathway, Endocrine resistance, Rap1 signaling pathway, Ras signaling pathway, VEGF signaling pathway, ErbB signaling pathway, PI3K-Akt signaling pathway, Prolactin signaling pathway, HIF-1 signaling pathway. An annotated statistical diagram of these pathways revealed ginsenoside Rg1 anti-fatigue effect impacts the Immune system, Nervous system, Endocrine system, Lipid metabolism, Amino acid metabolism, Metabolism of cofactors and vitamins.
3.5.3 PPI network analysis of ginsenoside Rg1’s anti-fatigue effects
The protein-protein interaction relationship of potential targets was obtained through the STRING database and consisted of a PPI network containing 87 nodes and 587 edges. This information was imported into CytoScape3.7.1 software to visualize network relationships. The CytoHubba function was used to calculate the Degree. The results show that the top three targets were AKT1, VEGFA and EGFR, with degrees of 49, 44 and 43, respectively. These targets were therefore considered as key anti-fatigue targets of ginsenoside Rg1 (Figure 8).
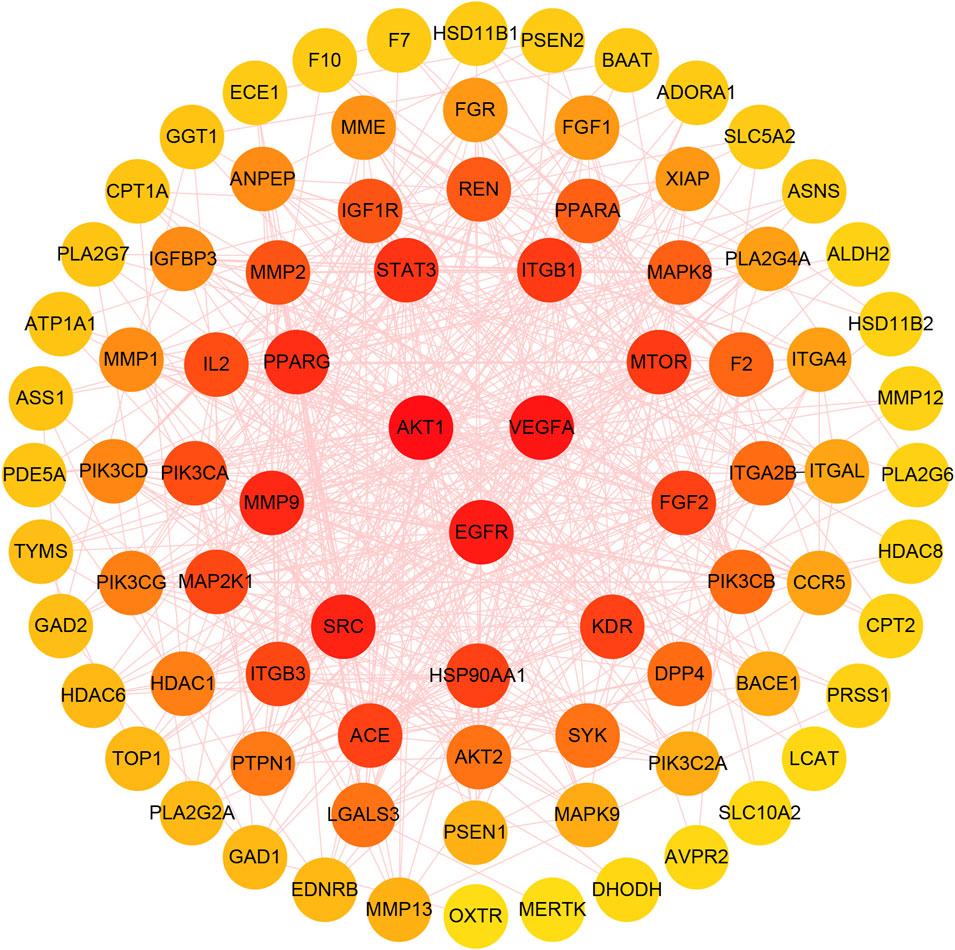
FIGURE 8. The gradual change of circle colors from red to yellow represents the change in Degree values, from large to small. The three targets in the center of the figure have the highest Degree values.
3.6 Effects of ginsenoside Rg1 treatment on mRNA expression of AKT1, VEGFA and EGFR
The mRNA expressions of AKT1, VEGFA and EGFR in the rat hippocampus and prefrontal cortex are shown in Figures 9A,B. In the hippocampus, VEGFA expression was increased in the CFS + Rg1 group compared to the Control group (p = 0.043), while the CFS + Rg1 showed increased expressions of AKT1 and VEGFA compared to CFS (p = 0.001, p = 0.015). The expression of EGFR remained relatively constant. As for the prefrontal cortex, VEGFA expression was significantly decreased in CFS + Rg1 compared to Control (p = 0.005), and EGFR expression was significantly increased in CFS (p = 0.002). In addition, VEGFA and EGFR levels were significantly lower in the CFS+ Rg1 group compared with the CFS group (p = 0.006, p < 0.001) and EGFR levels were lower in the CFS+ Oryzanol&VB1 group (p = 0.038). These results show ginsenoside Rg1 regulates EGFR in the prefrontal cortex of CFS rats.
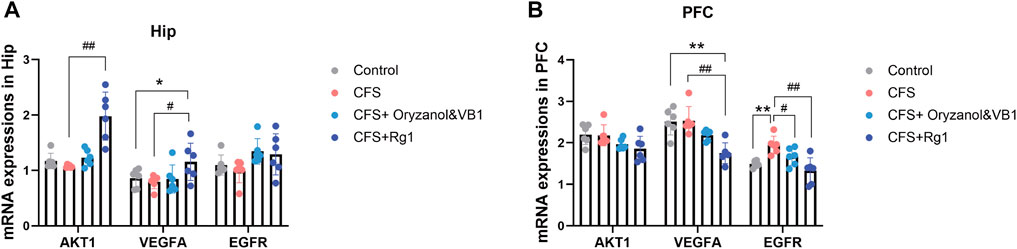
FIGURE 9. (A) Relative mRNA expression of AKT1, VEGFA and EGFR in the hippocampus (Hip) after 2 weeks of treatment. (B) Relative mRNA expression of AKT1, VEGFA and EGFR in the prefrontal cortex (PFC) after 2 weeks of treatment. Data are mean ± standard deviation, n = 6. *p < 0.05, **p < 0.01 versus Control group; #p < 0.05, ##p < 0.01 versus CFS group.
3.7 Protein expression levels of AKT1, VEGFA and EGFR
We performed western blot analysis to confirm the effect of ginsenoside Rg1 on the protein levels of AKT1, VEGFA and EGFR in the hippocampus and prefrontal cortex. As shown in Figure 10 (A, B, C), ginsenoside Rg1 up-regulates AKT1, EGFR and down-regulates VEGFA protein expression in the hippocampus, despite no statistical significance (p > 0.05). In the prefrontal cortex, ginsenoside Rg1 up-regulates VEGFA and down-regulates EGFR protein expression, with no statistical significance (p > 0.05) (Figures 10 D, E, F). EGFR protein and mRNA levels were similar in the prefrontal cortex. These results show ginsenoside Rg1 produces anti-fatigue effects by down-regulating EGFR expression.
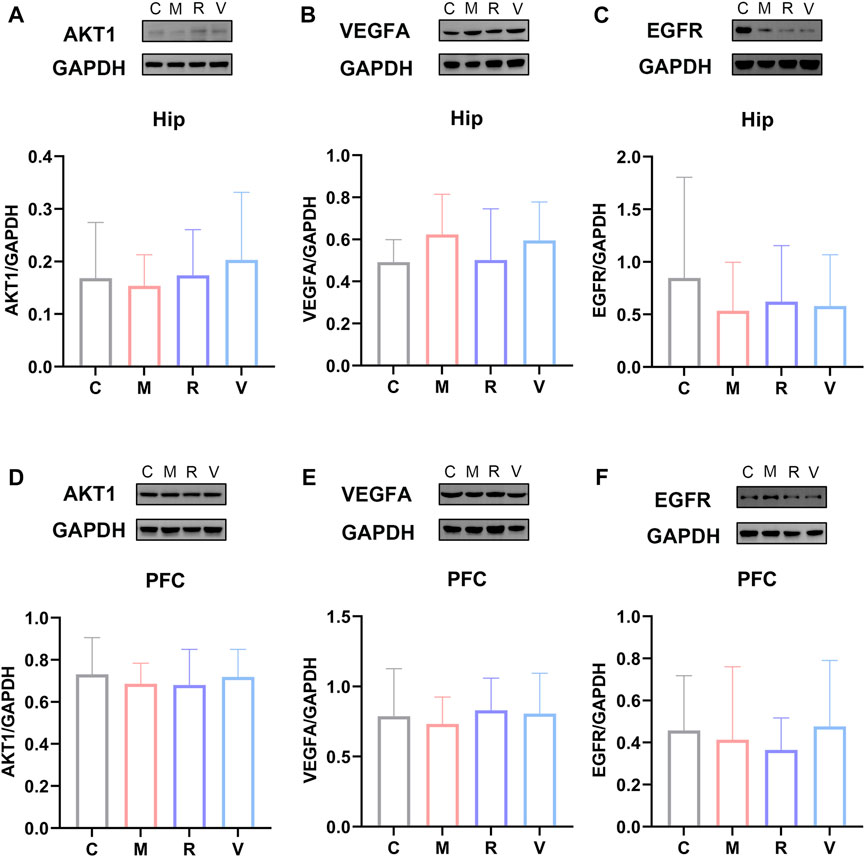
FIGURE 10. Relative protein expression of AKT1 (A), VEGFA (B), and EGFR (C) in the hippocampus. Relative protein abundance of AKT1 (D), VEGFA (E) and EGFR (F) in the prefrontal cortex. C: Control group, M: CFS group, R: CFS + Rg1 group, and V: CFS+ Oryzanol&VB1 group. Data are mean ± standard deviation, n = 6. *p < 0.05, **p < 0.01 versus Control group; #p < 0.05, ##p < 0.01 versus CFS group.
4 Discussion
Chronic fatigue syndrome, also known as myalgic encephalomyelitis, is an underappreciated debilitating disease with a significant impact on patient quality of life (Bornstein et al., 2022). CFS is characterized by chronic, disabling and multi-system disease with no effective treatment methods or diagnostic markers available at present (König et al., 2021; Wirth et al., 2021). CFS is associated with a variety of diseases, such as brucellosis, coronavirus infection, depression, and cancer. Recent studies found that patients recovering from COVID-19 may have persistent debilitating symptoms, and CFS remains the most dominant and common trait in these patients (Bansal et al., 2022). Current treatment guidelines for CFS mainly include cognitive behavioral therapy, graded exercise program and symptomatic therapy (Sapra and Bhandari, 2022), with limited clinical efficacy (Fang et al., 2022). In China, herbal medicine is widely used to treat CFS (Joung et al., 2019; Shin et al., 2021), with a previous meta-analysis showing TCM is safe and effective for CFS treatment (Zhang et al., 2022), which has sparked interest in its application (Wang et al., 2014; Dai et al., 2019; Zhang et al., 2020; Yang et al., 2022).
In this study, we explored the metabolic fingerprints and potential mechanisms of action of ginsenoside Rg1 in CFS treatment. We used multi-factor modeling (load-weighted forced swimming and restriction) to verify the anti-fatigue effects of ginsenoside Rg1 in CFS rats. The forced swimming animal model presents the core symptoms of CFS, such as fatigue and behavioral and cognitive abnormalities, but also pathological changes that have been found in CFS clinical studies (Sarma et al., 2015; Li and Han, 2022). Similarly, chronic restriction is simple to implement and can significantly induce fatigue symptoms (Li and Han, 2022). The rotarod test is widely used to assess athletic endurance with high reproducibility. In fact, the improvement of exercise tolerance is the strongest evidence for the existence of anti-fatigue effects (Kwon et al., 2021). In this study, CFS rats showed a decline in exercise tolerance that could be reversed by ginsenoside Rg1. The Open field test is a classic experimental method that reflects the spontaneous activity behavior of rats in an unfamiliar environment and is used to evaluate the locomotor ability of rats (Ferreira et al., 2022). We showed that the total movement distance in the CFS group was significantly reduced, as illustrated by decreased exercise ability that is also observed in clinical CFS patients (Aerenhouts et al., 2015). D-xylose is a common symptom of spleen deficiency and often used to evaluate intestinal absorption (Zeyue et al., 2022). We found that the D-xylose-excretion rate of CFS rats was statistically different from that of the Control group, indicating that a spleen deficiency syndrome model was successfully established. Accordingly, under similar feeding conditions, CFS mainly affects the gastrointestinal absorption function of rats to induce weight loss. These results demonstrated that the anti-fatigue effect of ginsenoside Rg1 was related to exercise tolerance, spontaneous activity and intestinal absorption function in CFS rats.
We identified 34 differential metabolites in the LC-MS analysis of non-targeted serum metabolites. These metabolites are involved in Taurine and hypotaurine metabolism, Arginine biosynthesis, Ether lipid metabolism, Alanine, aspartate and glutamate metabolism, and Pantothenate and CoA biosynthesis. In addition, we have found that Taurine and Mannose 6-phosphate are key metabolic markers of ginsenoside Rg1 in the treatment of CFS. Previous results showed that the differential metabolites identified here were also associated with CFS in different contexts and animal models, which attests the validity of our work. Among these metabolites, Taurine plays a specifically important role (Germain et al., 2017; Glass et al., 2023). Germain et al. found that CFS patients had low Taurine content, which was mainly affected by lipid metabolism and amino acids (Germain et al., 2017). Taurine is an internal metabolite that acts as an antioxidant and anti-fatigue agent (Schaffer and Kim, 2018), and represents the second most abundant amino acid in human muscle after glutamic acid (Ripps and Shen, 2012). Corsetti et al. suggested that Taurine content is a useful indicator of muscle injury and possible long-term fatigue (Corsetti et al., 2016), as documented by other studies (Luckose et al., 2015; Khalil et al., 2018; Thirupathi et al., 2018; Vidot et al., 2018). Yatabe et al. found that the use of Taurine significantly increased the duration of running time to exhaustion in rats (Yatabe et al., 2009). Moreover, a systematic review showed low-dose Taurine (0.05 g) can reduce muscle fatigue, which suggests it may be an effective drug, especially in high-intensity activities (Chen et al., 2021). Interestingly, Taurine used as a nutritional supplement promotes recovery from muscle injury caused by vigorous exercise (Wei et al., 2021). In addition, Liu et al. found that the therapeutic effect of ginseng on spleen deficiency rats may be realized by regulating Taurine and hypotaurine metabolism, and its metabolites can be used as potential biomarkers for the diagnosis and monitoring of spleen deficiency (Liu et al., 2022). Armstrong et al. analyzed the metabolic profile of the blood and urine of CFS patients and found that CFS patients had abnormal metabolism of alanine, aspartate, glutamate and other energy (Armstrong et al., 2015). These findings confirm the reliability of our results.
Network analysis showed that AKT1, VEGFA and EGFR were the key targets of ginsenoside Rg1 for enabling anti-fatigue effects. AKT1 encodes serine/threonine kinases that play an important role in several normal and pathological cell processes (Millischer et al., 2020), and a deficiency in AKT1 increases energy consumption (Wan et al., 2012). A randomized controlled trial found that AKT1 is an important core target for the treatment of cancer-induced fatigue (Cui et al., 2022). Furthermore, Liu et al. found that ginsenosides could enhance mice body ability and play an anti-fatigue role by upregulating the expression of AKT1 (Liu et al., 2019). We present similar results here and showed ginsenoside Rg1 up-regulates AKT1 protein expression despite not significantly. VEGFA is a key regulator of vascular growth. Angiogenesis in skeletal muscle can maintain oxygen and nutrient supply and clear metabolic byproducts that may lead to fatigue (Mohamad Shalan et al., 2016), which is associated with decreased levels of VEGFA (Wågström et al., 2021). A study on metabolic gene polymorphisms conducted progressive fatigue tests on athletes and identified VEGFA rs2010963C as an “endurance allele” of elite athletes (Ahmetov et al., 2009). We showed that CFS rats have decreased VEGFA levels in the prefrontal cortex, similar to patients with chronic fatigue (Landi et al., 2016).
The pathogenesis of CFS is closely related to the central nervous system (Mohamad Shalan et al., 2016; Sapra and Bhandari, 2022), with this syndrome being included in the neurology category (8E49) in ICD-11 (Gandasegui et al., 2021). In fatigue tests, reduced hippocampus activation is associated with HPA axis dysfunction and higher fatigue ratings (Klaassen et al., 2013). Saury JM et al. believes that the hippocampus plays an important role in the pathogenesis of CFS, and the trigger factors of CFS will also affect the hippocampus, leading to neurocognitive defects and disorders in the regulation of the pressure system and pain perception, which will further affect the hippocampus and trigger a vicious cycle of increased disability (Saury, 2016). There is a negative correlation between fatigue and hippocampus volume in CFS patients (Thapaliya et al., 2022). In addition, the prefrontal cortex has been identified as critical to fatigue (Ifuku et al., 2014). Bilateral prefrontal cortex volume was reduced in CFS patients, and the level of volume reduction in the right prefrontal cortex was correlated with the severity of fatigue (Nakatomi et al., 2014). An fMRI study found abnormal signals of functional activity in the prefrontal cortex of CFS patients (Caseras et al., 2006). These findings all support a strong link between the hippocampus, the prefrontal cortex, and CFS. We further performed PCR and Western Blot analyses on three key targets on the hippocampus and prefrontal cortex of CFS rats. Our results suggest that ginsenoside Rg1 can down-regulate the EGFR protein and mRNA expression. These observations have far-reaching significance for exploring the anti-fatigue mechanism of ginsenoside Rg1 at the molecular level. EGFR is an epidermal growth factor receptor and membrane surface receptor with tyrosine kinase activity (Cheng et al., 2022). Elevated levels of circulating ligands of EGFR, such as epidermal growth factor (EGF) and transforming growth factor α(TGF-α), can inhibit neural signals that drive normal behavior (Rich et al., 2017). Moreover, EGFR activation can stimulate the production of reactive oxygen species (ROS) by stimulating the PI3K pathway (Mansour et al., 2021). Previous studies showed ROS-induced mitochondrial function decline is related to fatigue (Nicolson, 2007; Pieczenik and Neustadt, 2007), and long-term oxidative stress can trigger CFS (Morris et al., 2017). At the same time, the level of oxidative stress in CFS patients is increased and correlated with clinical symptoms (Kennedy et al., 2005). Excessive ROS triggers oxidative stress, which is positively correlated with the severity of CFS symptoms (Gupta et al., 2010). Recent studies showed serum samples from CFS patients induce the production of ROS and nitric oxide in human HMC3 microglia (Gottschalk et al., 2022). A randomized controlled trial found that ginseng can reduce ROS levels in chronic fatigue patients and play an anti-fatigue role (Kim et al., 2013), which corroborates our observations.
5 Conclusion
This study explored for the first time the pharmacological mechanisms of ginsenoside Rg1 in CFS treatment using a comprehensive metabolomic model, network analysis and biological methods. We systematically elucidated the multi-target and multi-mechanisms behind ginsenoside Rg1 anti-fatigue effects. Ginsenoside Rg1 can effectively treat CFS by regulating expression levels of AKT1, VEGFA and EGFR, and interfering with the metabolism of Taurine and Mannose 6-phosphate. We experimentally confirmed that EGFR is the most critical target. These findings provide a theoretical basis for the clinical application of ginsenoside Rg1 for CFS treatment.
Data availability statement
The raw data supporting the conclusions of this article will be made available by the authors, without undue reservation.
Ethics statement
The animal study was reviewed and approved by Animal Care and Therapy Ethics Committee of the Beijing University of Chinese Medicine.
Author contributions
CL wrote the manuscript. YL and JC designed the experiments. ZH, YM, YQ, MY, XX and LL performed the experiments. XZ and YJ assisted on dealing with the statistical data. YL and JC revised and proof-read the manuscript. All the authors have read and approved the final version of the manuscript.
Funding
This work was supported by Beijing Natural Science Foundation (No. 7212182), National Natural Science Foundation of China (No. 81603514).
Conflict of interest
The authors declare that the research was conducted in the absence of any commercial or financial relationships that could be construed as a potential conflict of interest.
Publisher’s note
All claims expressed in this article are solely those of the authors and do not necessarily represent those of their affiliated organizations, or those of the publisher, the editors and the reviewers. Any product that may be evaluated in this article, or claim that may be made by its manufacturer, is not guaranteed or endorsed by the publisher.
References
Aerenhouts, D., Ickmans, K., Clarys, P., Zinzen, E., Meersdom, G., Lambrecht, L., et al. (2015). 'Sleep characteristics, exercise capacity and physical activity in patients with chronic fatigue syndrome. Disabil. Rehabil. 37, 2044–2050. doi:10.3109/09638288.2014.993093
Ahmetov, , Williams, A. G., Popov, D. V., Lyubaeva, E. V., Hakimullina, A. M., Fedotovskaya, O. N., et al. (2009). 'The combined impact of metabolic gene polymorphisms on elite endurance athlete status and related phenotypes. Hum. Genet. 126, 751–761. doi:10.1007/s00439-009-0728-4
Armstrong, C. W., McGregor, N. R., Butt, H. L., and Gooley, P. R. (2014). 'Metabolism in chronic fatigue syndrome. Adv. Clin. Chem. 66, 121–172. doi:10.1016/b978-0-12-801401-1.00005-0
Armstrong, C. W., McGregor, N. R., Lewis, D. P., Butt, H. L., and Gooley, P. R. (2015). 'Metabolic profiling reveals anomalous energy metabolism and oxidative stress pathways in chronic fatigue syndrome patients. Metabolomics 11, 1626–1639. doi:10.1007/s11306-015-0816-5
Bansal, R., Gubbi, S., and Koch, C. A. (2022). 'COVID-19 and chronic fatigue syndrome: An endocrine perspective. J. Clin. Transl. Endocrinol. 27, 100284. doi:10.1016/j.jcte.2021.100284
Bested, A. C., and Marshall, L. M. (2015). Review of myalgic encephalomyelitis/chronic fatigue syndrome: An evidence-based approach to diagnosis and management by clinicians. Rev. Environ. Health 30, 223–249. doi:10.1515/reveh-2015-0026
Bornstein, S. R., Voit-Bak, K., Donate, T., Rodionov, R. N., Gainetdinov, R. R., Tselmin, S., et al. (2022). Chronic post-COVID-19 syndrome and chronic fatigue syndrome: Is there a role for extracorporeal apheresis? Mol. Psychiatry 27, 34–37. doi:10.1038/s41380-021-01148-4
Caseras, X., Mataix-Cols, D., Giampietro, V., Rimes, K. A., Brammer, M., Zelaya, F., et al. (2006). 'Probing the working memory system in chronic fatigue syndrome: A functional magnetic resonance imaging study using the n-back task. Psychosom. Med. 68, 947–955. doi:10.1097/01.psy.0000242770.50979.5f
Chaves-Filho, A. J. M., Macedo, D. S., de Lucena, D. F., and Maes, M. (2019). 'Shared microglial mechanisms underpinning depression and chronic fatigue syndrome and their comorbidities. Behav. Brain Res. 372, 111975. doi:10.1016/j.bbr.2019.111975
Chen, Q., Li, Z., Pinho, R. A., Gupta, R. C., Ugbolue, U. C., Thirupathi, A., et al. (2021). 'The dose response of taurine on aerobic and strength exercises: A systematic review. Front. Physiol. 12, 700352. doi:10.3389/fphys.2021.700352
Cheng, L., Wang, F., Li, Z. H., Wen, C., Ding, L., Zhang, S. B., et al. (2022). 'Study on the active components and mechanism of Suanzaoren decoction in improving cognitive impairment caused by sleep deprivation. J. Ethnopharmacol. 296, 115502. doi:10.1016/j.jep.2022.115502
Corsetti, R., Barassi, A., Perego, S., Sansoni, V., Rossi, A., Damele, C. A., et al. (2016). 'Changes in urinary amino acids excretion in relationship with muscle activity markers over a professional cycling stage race: In search of fatigue markers. Amino Acids 48, 183–192. doi:10.1007/s00726-015-2077-z
Cui, Y., Mi, J., Feng, Y., Li, L., Wang, Y., Hu, J., et al. (2022). '[Huangqi sijunzi decoction for treating cancer-related fatigue in breast cancer patients: A randomized trial and network pharmacology study]. Nan Fang. Yi Ke Da Xue Xue Bao 42, 649–657. doi:10.12122/j.issn.1673-4254.2022.05.04
Cvejic, E., Birch, R. C., and Vollmer-Conna, U. (2016). 'Cognitive dysfunction in chronic fatigue syndrome: A review of recent evidence. Curr. Rheumatol. Rep. 18, 24. doi:10.1007/s11926-016-0577-9
Dai, L., Zhou, W. J., Wang, M., Zhou, S. G., and Ji, G. (2019). 'Efficacy and safety of sijunzi decoction for chronic fatigue syndrome with spleen deficiency pattern: Study protocol for a randomized, double-blind, placebo-controlled trial. Ann. Transl. Med. 7, 587. doi:10.21037/atm.2019.09.136
Fang, Y., Yue, B. W., Ma, H. B., and Yuan, Y. P. (2022). Acupuncture and moxibustion for chronic fatigue syndrome: A systematic review and network meta-analysis, 101, e29310. doi:10.1097/md.0000000000029310Med. Baltim.
Feng, Yichong., Xu, Zhiwei., Pan, Huashan., and Zhao, Ziming. (2010). 'Effects of ginsenoside Rg1 on structure and function of rat skeletal muscle with exercise-induced fatigue. J. Guangzhou Univ. Traditional Chin. Med. 27, 40–44. doi:10.13359/j.cnki.gzxbtcm.2010.01.018
Ferreira, J. S., Leite Junior, J. B., de Mello Bastos, J. M., Samuels, R. I., Carey, R. J., and Carrera, M. P. (2022). 'A new method to study learning and memory using spontaneous locomotor activity in an open-field arena. J. Neurosci. Methods 366, 109429. doi:10.1016/j.jneumeth.2021.109429
Gandasegui, I. M., Laka, L. A., Gargiulo, P. Á., Gómez-Esteban, J. C., and Sánchez, J. L. (2021). Myalgic encephalomyelitis/chronic fatigue syndrome: A neurological entity? Med. Kaunas. 57, 1030. doi:10.3390/medicina57101030
Geng, L., and Wang, Y. (2012). Spleen deficiency is the core pathogenesis of chronic fatigue syndrome. J. Heilongjiang Traditional Chin. Med. 41, 8–9. doi:10.3969/j.issn.1000-9906.2012.04.003
Germain, A., Ruppert, D., Levine, S. M., and Hanson, M. R. (2017). 'Metabolic profiling of a myalgic encephalomyelitis/chronic fatigue syndrome discovery cohort reveals disturbances in fatty acid and lipid metabolism. Mol. Biosyst. 13, 371–379. doi:10.1039/c6mb00600k
Glass, K. A., Germain, A., Huang, Y. V., and Hanson, M. R. (2023). 'Urine metabolomics exposes anomalous recovery after maximal exertion in female ME/CFS patients. Int. J. Mol. Sci. 24, 3685. doi:10.3390/ijms24043685
Gottschalk, G., Peterson, D., Knox, K., Maynard, M., Whelan, R. J., and Roy, A. (2022). 'Elevated ATG13 in serum of patients with ME/CFS stimulates oxidative stress response in microglial cells via activation of receptor for advanced glycation end products (RAGE). Mol. Cell. Neurosci. 120, 103731. doi:10.1016/j.mcn.2022.103731
Gupta, A., Vij, G., and Chopra, K. (2010). 'Possible role of oxidative stress and immunological activation in mouse model of chronic fatigue syndrome and its attenuation by olive extract. J. Neuroimmunol. 226, 3–7. doi:10.1016/j.jneuroim.2010.05.021
He, J., Yu, Q., Wu, C., Sun, Z., Wu, X., Liu, R., et al. (2020). 'Acupuncture of the Beishu acupoint participates in regulatory effects of ginsenoside Rg1 on T cell subsets of rats with chronic fatigue syndrome. Ann. Palliat. Med. 9, 3436–3446. doi:10.21037/apm-20-1714
Heinrich, M., Appendino, G., Efferth, T., Fürst, R., Izzo, A. A., Kayser, O., et al. (2020). 'Best practice in research - overcoming common challenges in phytopharmacological research. J. Ethnopharmacol. 246, 112230. doi:10.1016/j.jep.2019.112230
Ifuku, M., Hossain, S. M., Noda, M., and Katafuchi, T. (2014). 'Induction of interleukin-1β by activated microglia is a prerequisite for immunologically induced fatigue. Eur. J. Neurosci. 40, 3253–3263. doi:10.1111/ejn.12668
Joung, J. Y., Lee, J. S., Cho, J. H., Lee, D. S., Ahn, Y. C., and Son, C. G. (2019). 'The efficacy and safety of myelophil, an ethanol extract mixture of astragali radix and salviae radix, for chronic fatigue syndrome: A randomized clinical trial. Front. Pharmacol. 10, 991. doi:10.3389/fphar.2019.00991
Kennedy, G., Spence, V. A., McLaren, M., Hill, A., Underwood, C., and Belch, J. J. (2005). 'Oxidative stress levels are raised in chronic fatigue syndrome and are associated with clinical symptoms. Free Radic. Biol. Med. 39, 584–589. doi:10.1016/j.freeradbiomed.2005.04.020
Khalil, R. M., Abdo, W. S., Saad, A., and Khedr, E. G. (2018). 'Muscle proteolytic system modulation through the effect of taurine on mice bearing muscular atrophy. Mol. Cell. Biochem. 444, 161–168. doi:10.1007/s11010-017-3240-5
Kim, H. G., Cho, J. H., Yoo, S. R., Lee, J. S., Han, J. M., Lee, N. H., et al. (2013). 'Antifatigue effects of Panax ginseng C.A. Meyer: A randomised, double-blind, placebo-controlled trial. PLoS One 8, e61271. doi:10.1371/journal.pone.0061271
Klaassen, E. B., de Groot, R. H., Evers, E. A., Nicolson, N. A., Veltman, D. J., and Jolles, J. (2013). 'Cortisol and induced cognitive fatigue: Effects on memory activation in healthy males. Biol. Psychol. 94, 167–174. doi:10.1016/j.biopsycho.2013.05.015
König, R. S., Albrich, W. C., Kahlert, C. R., Bahr, L. S., Löber, U., Vernazza, P., et al. (2021). The gut microbiome in myalgic encephalomyelitis (ME)/Chronic fatigue syndrome (CFS). Front. Immunol. 12, 628741. doi:10.3389/fimmu.2021.628741
Kwon, D. A., Kim, Y. S., Kim, S. K., Baek, S. H., Kim, H. K., and Lee, H. S. (2021). 'Antioxidant and antifatigue effect of a standardized fraction (HemoHIM) from Angelica gigas, Cnidium officinale, and Paeonia lactiflora. Pharm. Biol. 59, 391–400. doi:10.1080/13880209.2021.1900878
Landi, A., Broadhurst, D., Vernon, S. D., Tyrrell, D. L., and Houghton, M. (2016). 'Reductions in circulating levels of IL-16, IL-7 and VEGF-A in myalgic encephalomyelitis/chronic fatigue syndrome. Cytokine 78, 27–36. doi:10.1016/j.cyto.2015.11.018
Lei, C., Chen, Z., Fan, L., Xue, Z., Chen, J., Wang, X., et al. (2022). 'Integrating metabolomics and network analysis for exploring the mechanism underlying the antidepressant activity of paeoniflorin in rats with CUMS-induced depression. Front. Pharmacol. 13, 904190. doi:10.3389/fphar.2022.904190
Li, Y., and Han, X. (2022). 'Construction and evaluation of animal models of chronic fatigue syndrome. Chin. J. Exp. Traditional Med. Formulae 29, 234–242. doi:10.13422/j.cnki.syfjx.20230999
Li, Q., Wang, L., Fang, X., and Zhao, L. (2022). 'Highly efficient biotransformation of notoginsenoside R1 into ginsenoside Rg1 by dictyoglomus thermophilum β-xylosidase xln-DT. J. Microbiol. Biotechnol. 32, 447–457. doi:10.4014/jmb.2111.11020
Lim, E. J., Lee, J. S., Lee, E. J., Jeong, S. J., Park, H. Y., Ahn, Y. C., et al. (2021). 'Nationwide epidemiological characteristics of chronic fatigue syndrome in South Korea. J. Transl. Med. 19, 502. doi:10.1186/s12967-021-03170-0
Liu, F. X., Lin, Z. X., Zhang, H. L., Zhang, Z. Q., Yang, K. Q., Fan, X. F., et al. (2019). '[Analysis of anti-fatigue mechanism and potential targets of ginseng]. Zhongguo Zhong Yao Za Zhi 44, 5479–5487. doi:10.19540/j.cnki.cjcmm.20190805.401
Liu, M., Li, F., Cai, Y., Xie, D., Wu, Y., Zhang, M., et al. (2022). 'Intervention effects of ginseng on spleen-qi deficiency in rats revealed by GC-MS-based metabonomic approach. J. Pharm. Biomed. Anal. 217, 114834. doi:10.1016/j.jpba.2022.114834
Luckose, F., Pandey, M. C., and Radhakrishna, K. (2015). 'Effects of amino acid derivatives on physical, mental, and physiological activities. Crit. Rev. Food Sci. Nutr. 55, 1793–1807. doi:10.1080/10408398.2012.708368
Ma, P., Peng, Y., Zhao, L., Liu, F., and Li, X. (2021). Differential effect of polysaccharide and nonpolysaccharide components in Sijunzi decoction on spleen deficiency syndrome and their mechanisms. Phytomedicine 93, 153790. doi:10.1016/j.phymed.2021.153790
Mansour, H. M., Fawzy, H. M., El-Khatib, A. S., and Khattab, M. M. (2021). 'Potential repositioning of anti-cancer EGFR inhibitors in alzheimer's disease: Current perspectives and challenging prospects. Neuroscience 469, 191–196. doi:10.1016/j.neuroscience.2021.06.013
Millischer, V., Matheson, G. J., Martinsson, L., Römer, I. E., Schalling, M., Lavebratt, C., et al. (2020). 'AKT1 and genetic vulnerability to bipolar disorder. Psychiatry Res. 284, 112677. doi:10.1016/j.psychres.2019.112677
Mohamad Shalan, N. A., Mustapha, N. M., and Mohamed, S. (2016). 'Morinda citrifolia leaf enhanced performance by improving angiogenesis, mitochondrial biogenesis, antioxidant, anti-inflammatory & stress responses. Food Chem. 212, 443–452. doi:10.1016/j.foodchem.2016.05.179
Mohanan, P., Subramaniyam, S., Mathiyalagan, R., and Yang, D. C. (2018). 'Molecular signaling of ginsenosides Rb1, Rg1, and Rg3 and their mode of actions. J. Ginseng Res. 42, 123–132. doi:10.1016/j.jgr.2017.01.008
Moore, Y., Serafimova, T., Anderson, N., King, H., Richards, A., Brigden, A., et al. (2021). 'Recovery from chronic fatigue syndrome: A systematic review-heterogeneity of definition limits study comparison. Arch. Dis. Child. 106, 1087–1094. doi:10.1136/archdischild-2020-320196
Morris, G., Berk, M., Klein, H., Walder, K., Galecki, P., and Maes, M. (2017). 'Nitrosative stress, hypernitrosylation, and autoimmune responses to nitrosylated proteins: New pathways in neuroprogressive disorders including depression and chronic fatigue syndrome. Mol. Neurobiol. 54, 4271–4291. doi:10.1007/s12035-016-9975-2
Nacul, L., Authier, F. J., Scheibenbogen, C., Lorusso, L., Helland, I. B., Martin, J. A., et al. (2021). 'European network on myalgic encephalomyelitis/chronic fatigue syndrome (EUROMENE): Expert consensus on the diagnosis, service provision, and Care of people with ME/CFS in Europe. Med. Kaunas. 57, 510. doi:10.3390/medicina57050510
Nagy-Szakal, D., Barupal, D. K., Lee, B., Che, X., Williams, B. L., Kahn, E. J. R., et al. (2018). 'Insights into myalgic encephalomyelitis/chronic fatigue syndrome phenotypes through comprehensive metabolomics. Sci. Rep. 8, 10056. doi:10.1038/s41598-018-28477-9
Nakatomi, Y., Mizuno, K., Ishii, A., Wada, Y., Tanaka, M., Tazawa, S., et al. (2014). 'Neuroinflammation in patients with chronic fatigue syndrome/myalgic encephalomyelitis: An 11C-(R)-PK11195 PET study. J. Nucl. Med. 55, 945–950. doi:10.2967/jnumed.113.131045
Nguyen, C. B., Kumar, S., Zucknick, M., Kristensen, V. N., Gjerstad, J., Nilsen, H., et al. (2019). 'Associations between clinical symptoms, plasma norepinephrine and deregulated immune gene networks in subgroups of adolescent with Chronic Fatigue Syndrome. Brain Behav. Immun. 76, 82–96. doi:10.1016/j.bbi.2018.11.008
Nicolson, G. L. (2007). 'Metabolic syndrome and mitochondrial function: Molecular replacement and antioxidant supplements to prevent membrane peroxidation and restore mitochondrial function. J. Cell. Biochem. 100, 1352–1369. doi:10.1002/jcb.21247
Pieczenik, S. R., and Neustadt, J. (2007). 'Mitochondrial dysfunction and molecular pathways of disease. Exp. Mol. Pathol. 83, 84–92. doi:10.1016/j.yexmp.2006.09.008
Rich, T., Zhao, F., Cruciani, R. A., Cella, D., Manola, J., and Fisch, M. J. (2017). 'Association of fatigue and depression with circulating levels of proinflammatory cytokines and epidermal growth factor receptor ligands: A correlative study of a placebo-controlled fatigue trial. Cancer Manag. Res. 9, 1–10. doi:10.2147/cmar.S115835
Ripps, H., and Shen, W. (2012). Review: Taurine: A "very essential" amino acid. Mol. Vis. 18, 2673–2686.
Sandler, C. X., and Lloyd, A. R. (2020). 'Chronic fatigue syndrome: Progress and possibilities. Med. J. Aust. 212, 428–433. doi:10.5694/mja2.50553
Sapra, A., and Bhandari, P. (2022). “'Chronic fatigue syndrome,” in StatPearls (Treasure Island (FL): StatPearls Publishing Copyright © 2022, StatPearls Publishing LLC).
Sarma, P., Borah, M., and Das, S. (2015). 'Evaluation of the effect of ethanolic extract of fruit pulp of Cassia fistula Linn. on forced swimming induced chronic fatigue syndrome in mice. Res. Pharm. Sci. 10, 206–213. doi:10.1016/0016-6480(84)90098-4
Saury, J. M. (2016). The role of the hippocampus in the pathogenesis of myalgic encephalomyelitis/chronic fatigue syndrome (ME/CFS). Med. Hypotheses 86, 30–38. doi:10.1016/j.mehy.2015.11.024
Schaffer, S., and Kim, H. W. (2018). 'Effects and mechanisms of taurine as a therapeutic agent. Biomol. Ther. Seoul. 26, 225–241. doi:10.4062/biomolther.2017.251
Shao, C., Ren, Y., Wang, Z., Kang, C., Jiang, H., and Chi, A. (2017). Detection of urine metabolites in a rat model of chronic fatigue syndrome before and after exercise. Biomed. Res. Int. 2017, 8182020. doi:10.1155/2017/8182020
Shi, J., Shen, J., Xie, J., Zhi, J., and Xu, Y. (2018). Chronic fatigue syndrome in Chinese middle-school students. Med. Baltim. 97, e9716. doi:10.1097/md.0000000000009716
Shin, S., Park, S. J., and Hwang, M. (2021). 'Effectiveness a herbal medicine (Sipjeondaebo-tang) on adults with chronic fatigue syndrome: A randomized, double-blind, placebo-controlled trial. Integr. Med. Res. 10, 100664. doi:10.1016/j.imr.2020.100664
Thapaliya, K., Staines, D., Marshall-Gradisnik, S., Su, J., and Barnden, L. (2022). 'Volumetric differences in hippocampal subfields and associations with clinical measures in myalgic encephalomyelitis/chronic fatigue syndrome. J. Neurosci. Res. 100, 1476–1486. doi:10.1002/jnr.25048
Thirupathi, A., Freitas, S., Sorato, H. R., Pedroso, G. S., Effting, P. S., Damiani, A. P., et al. (2018). 'Modulatory effects of taurine on metabolic and oxidative stress parameters in a mice model of muscle overuse. Nutrition 54, 158–164. doi:10.1016/j.nut.2018.03.058
Vidot, H., Cvejic, E., Carey, S., Strasser, S. I., McCaughan, G. W., Allman-Farinelli, M., et al. (2018). 'Randomised clinical trial: Oral taurine supplementation versus placebo reduces muscle cramps in patients with chronic liver disease. Aliment. Pharmacol. Ther. 48, 704–712. doi:10.1111/apt.14950
Wågström, P., Nilsdotter-Augustinsson, Å., Nilsson, M., Björkander, J., Dahle, C., and Nyström, S. (2021). 'Fatigue is common in immunoglobulin G subclass deficiency and correlates with inflammatory response and need for immunoglobulin replacement therapy. Front. Immunol. 12, 797336. doi:10.3389/fimmu.2021.797336
Wan, M., Easton, R. M., Gleason, C. E., Monks, B. R., Ueki, K., Kahn, C. R., et al. (2012). 'Loss of Akt1 in mice increases energy expenditure and protects against diet-induced obesity. Mol. Cell. Biol. 32, 96–106. doi:10.1128/mcb.05806-11
Wang, Y. Y., Li, X. X., Liu, J. P., Luo, H., Ma, L. X., and Alraek, T. (2014). 'Traditional Chinese medicine for chronic fatigue syndrome: A systematic review of randomized clinical trials. Complement. Ther. Med. 22, 826–833. doi:10.1016/j.ctim.2014.06.004
Wang, P., Wang, Q., Yang, B., Zhao, S., and Kuang, H. (2015). 'The progress of metabolomics study in traditional Chinese medicine research. Am. J. Chin. Med. 43, 1281–1310. doi:10.1142/s0192415x15500731
Wei, C., Zhao, S., Zhang, Y., Gu, W., Kumar Sarker, S., Liu, S., et al. (2021). 'Effect of multiple-nutrient supplement on muscle damage, liver, and kidney function after exercising under heat: Based on a pilot study and a randomised controlled trial. Front. Nutr. 8, 740741. doi:10.3389/fnut.2021.740741
Wirth, K. J., Scheibenbogen, C., and Paul, F. (2021). 'An attempt to explain the neurological symptoms of Myalgic Encephalomyelitis/Chronic Fatigue Syndrome. J. Transl. Med. 19, 471. doi:10.1186/s12967-021-03143-3
Xu, Y. X., Luo, H. S., Sun, D., Wang, R., and Cai, J. (2019). '[Acupuncture in the treatment of chronic fatigue syndrome based on "interaction of brain and kidney" in TCM: A randomized controlled trial]. Zhongguo Zhen Jiu 39, 123–127. doi:10.13703/j.0255-2930.2019.02.003
Yang, J., Shin, K. M., Abu Dabrh, A. M., Bierle, D. M., Zhou, X., Bauer, B. A., et al. (2022). 'Ginseng for the treatment of chronic fatigue syndrome: A systematic review of clinical studies. Glob. Adv. Health Med. 11, 2164957x221079790. doi:10.1177/2164957x221079790
Yatabe, Y., Miyakawa, S., Ohmori, H., Mishima, H., and Adachi, T. (2009). 'Effects of taurine administration on exercise. Adv. Exp. Med. Biol. 643, 245–252. doi:10.1007/978-0-387-75681-3_25
Yousuf, S., Liu, H., Yingshu, Z., Zahid, D., Ghayas, H., Li, M., et al. (2022). 'Ginsenoside Rg1 modulates intestinal microbiota and supports re-generation of immune cells in dexamethasone-treated mice. Acta Microbiol. Immunol. Hung 69, 259–269. doi:10.1556/030.2022.01881
Zeyue, Y. U., Liyu, H., Zongyuan, L. I., Jianhui, S., Hongying, C., Hairu, H., et al. (2022). 'Correlation between slow transit constipation and spleen deficiency, and gut microbiota: A pilot study. J. Tradit. Chin. Med. 42, 353–363. doi:10.19852/j.cnki.jtcm.20220408.002
Zhang, X., Wang, M., and Zhou, S. (2020). Advances in clinical research on traditional Chinese medicine treatment of chronic fatigue syndrome. Evid. Based Complement. Altern. Med. 2020, 4715679. doi:10.1155/2020/4715679
Keywords: ginsenoside Rg1, metabolomics, network pharmacology, EGFR, AKT1, VEGFA, taurine, Mannose 6-phosphate
Citation: Lei C, Chen J, Huang Z, Men Y, Qian Y, Yu M, Xu X, Li L, Zhao X, Jiang Y and Liu Y (2023) Ginsenoside Rg1 can reverse fatigue behavior in CFS rats by regulating EGFR and affecting Taurine and Mannose 6-phosphate metabolism. Front. Pharmacol. 14:1163638. doi: 10.3389/fphar.2023.1163638
Received: 11 February 2023; Accepted: 30 March 2023;
Published: 10 April 2023.
Edited by:
Ochuko Lucky Erukainure, University of the Free State, South AfricaReviewed by:
Rebecca Reddy, Durban University of Technology, South AfricaViola Anuli Nicholas-Okpara, Federal Institute of Industrial Research Oshodi, Nigeria
Copyright © 2023 Lei, Chen, Huang, Men, Qian, Yu, Xu, Li, Zhao, Jiang and Liu. This is an open-access article distributed under the terms of the Creative Commons Attribution License (CC BY). The use, distribution or reproduction in other forums is permitted, provided the original author(s) and the copyright owner(s) are credited and that the original publication in this journal is cited, in accordance with accepted academic practice. No use, distribution or reproduction is permitted which does not comply with these terms.
*Correspondence: Yueyun Liu, chloelou@126.com
†These authors share first authorship