- 1Department of Biomedical Sciences Laboratory, Affiliated Dongyang Hospital of Wenzhou Medical University, Dongyang, China
- 2Department of Infectious Diseases, Affiliated Dongyang Hospital of Wenzhou Medical University, Dongyang, China
Objectives: To establish an individualized nomogram to predict the probability of drug-induced liver injury (DILI) in tuberculosis patients receiving anti-tuberculosis treatment.
Methods: The clinical information of patients admitted to a tertiary hospital between January 2010 and December 2022 was retrospectively reviewed from the clinical records. Patients with baseline liver diseases (hepatis B or C infection and fatty liver) or taking liver protective drugs were excluded. The maximum values in liver function test within 180 days after anti-tuberculosis treatment were collected to determine the occurrence of DILI. The candidate variables used for establishing prediction model in this study were the last results within the 30 days before the treatment onset. The final variables were included after univariate and multivariate logistic regression analyses and applied to establish the nomogram model. The discrimination power and prediction accuracy of the prediction model were assessed using the area under the receiver operating characteristic (AUC) curve and a calibration chart. The clinical effectiveness was assessed via decision curve analysis (DCA). The established model was validated in two validation groups.
Results: A total of 1979 patients with 25 variables were enrolled in this study, and the incidence of DILI was 4.2% (n = 83). The patients with complete variables were divided into training group (n = 1,121), validation group I (n = 492) and validation group II (n = 264). Five variables were independent factors for DILI and included in the final prediction model presented as nomogram: age (odds ratio [OR] 1.022, p = 0.023), total bilirubin ≥17.1 μmol/L (OR 11.714, p < 0.001), uric acid (OR 0.977, p = 0.047), neutrophil count (OR 2.145, 0.013) and alcohol consumption (OR 3.209, p = 0.002). The AUCs of the prediction model in the training group, validation group I and validation group II were 0.833, 0.668, and 0.753, respectively. The p-values of calibration charts in the three groups were 0.800, 0.996, and 0.853. The DCA curves of the prediction model were above the two extreme curves.
Conclusion: The nomogram model in this study could effectively predict the DILI risk among patients under anti-tuberculosis drug treatment.
Introduction
Mycobacterium tuberculosis (MTB) is the pathogen that causes tuberculosis (TB) in humans and constitutes a great threat to global public health. The World Health Organization (WHO) reported that approximately 10.6 million people fell ill due to TB in 2021 worldwide, and this number is 4.4% higher than that in 2020 (Global tuberculosis report 2021, 2021; Global tuberculosis report 2022, 2022). Although the success rates of TB treatments can be as high as 86%, TB-caused mortality ranked second after COVID-19–caused mortality among infection-caused deaths (Global tuberculosis report 2022, 2022). Once the diagnosis of TB is confirmed based on clinical manifestations, radiological examination, and laboratory tests, a long course of antibiotic treatment is needed to control the growth of MTB. However, combined drug treatment is often accompanied by adverse effects, among which drug-induced liver injury (DILI) and gastrointestinal syndromes are the two most common events (Zhang et al., 2015; El Hamdouni et al., 2020; Wang et al., 2022a). Adverse effects frequently cause patients to abort the treatment, which might cause treatment failure, induced drug resistance, and transmission of the infection. Thus, it is essential to identify biomarkers for predicting DILI in patients with TB.
Several studies on the possible risk factors for DILI in patients with TB have suggested complex mechanisms underlying DILI (Medina-Caliz et al., 2016; Ashby et al., 2021). In fact, combinatorial drug regimens containing various antibiotics are always prescribed to patients with TB, but the potential association between DILI and such combinatorial regimens has not been explored (Sterling et al., 2011; Ahmad et al., 2018; Lan et al., 2020; Xu et al., 2022). Moreover, the hepatic viral infections, patients’ age, nutritional status, and alcohol-consumption level of patients, as well as their clinical history of liver disease may affect liver function (Medina-Caliz et al., 2016; Sun et al., 2016; Chang et al., 2018; Jiang et al., 2021) and thereby contribute to DILI occurrence (Yew et al., 2018). In clinical practice, the patients with high risk of DILI based on the clinical experiences have been suggested for taking drugs for prophylaxis, resulting into the fact that patients who are not under liver protection are largely ignored (Saito et al., 2016; Xu et al., 2017). Novel urinary (Wang et al., 2022b) or blood (Ho et al., 2021; Wang et al., 2022c; Deng et al., 2022) biomarkers identified via high-throughput methods involving mass spectrometry or sequencing might indicate for potential role in predicting DILI, but they are far away from clinical practice due to uncertainty and high cost.
Routine laboratory examinations used in TB diagnosis may provide us with objective and quantitative parameters that can be screened for risk factors related to DILI. Prediction model can facilitate the screening for the significant risk factors in the real world, which has been found essential in diagnosis, treatment, and prognosis. Although several risk factors have been found associated with DILI, there are limited reports on the application of nomogram to predict DILI-associated risks in a large sample size (Ashby et al., 2021; Raj Mani et al., 2021; Zhong et al., 2021; Zhao et al., 2022). Here, a cohort of TB patients spanning 13 years were retrospectively reviewed, and a nomogram prediction model was established to evaluate the DILI risk following anti-TB treatment among patients who were negative for baseline liver diseases and liver protective drugs.
Materials and methods
Participants inclusion and exclusion
TB patients who met the inclusion criteria were enrolled via data extraction from clinical record mining database (supported by Le 9 Co., Ltd.): the patients who were diagnosed with tuberculosis infection and started their anti-TB drug treatment between January 2010 and December 2022. The database was constructed based on the clinical records from patients who were admitted to Affiliated Dongyang Hospital of Wenzhou Medical University. Exclusion criteria: the patients who took liver protective drugs for treatment or prophylaxis based on the drug usage record; the patients who were accompanied by HIV infection and liver cancer. The baseline liver diseases including hepatitis caused by HBV or HCV (by immunological test) and fatty liver (by radiological examination) were determined based on the extracted information from clinical record database.
Definition
The definition criteria list for DILI induced by anti-TB treatment included (Global tuberculosis report 2022, 2022) a clear history of taking first-line anti-TB drugs, including isoniazid, rifampicin, ethambutol and pyrazinamide (Global tuberculosis report 2021, 2021). The peak values in liver function test within 180 days following the first drug dose met at least one of these followed terms alanine aminotransferase (ALT) ≥ 3 times the upper limit of normal range (ULN); total bilirubin ≥2 times ULN; under the premise of elevated levels of aspartate aminotransferase (AST), alkaline phosphatase and total bilirubin, at least one ≥2 times ULN. The alcohol consumption was defined as the patient had daily intake of alcohol based on self-description after admission.
Screening the variables to establish a prediction model
All the candidate variables contained clinical and laboratory indexes, which were the last measured values within 30 days before drug use. The variables with missing percentages higher 20% were removed, and the enrolled patients with complete data on the remaining variables were randomly split into training group and validation group I with a ratio of 7:3 (Figure 1A). The process for the identification of the variables used in generating the model was described in Figure 1B. First, univariate analysis was performed to identify variables significantly related to DILI (p < 0.05). The linear relationship between the continual variables and logitp was tested using the Box-Tidwell function. If, a linear relationship was absent (p < 0.05), and the variable was converted into categorical variables based on the normal range. The presence of multicollinearity among the involved variables was defined as variance inflation factors (VIFs) larger than 10, and thus these variables were excluded in further analysis. Second, multivariate analysis and stepwise regression were used to identify variables significantly related to DILI. Based on the finally involved variables, a second validation group (validation group II) was extracted from the population, excluding those had been enrolled in training group and validation group I. The discriminative ability of logistic regression model was assessed based on the area under the receiver operating characteristic (ROC) curve (AUC), model calibration was evaluated using the Hosmer–Lemeshow statistic (p > 0.05 indicated good agreement between predicted and real values) and the net benefit in clinical was evaluated by decision curve analysis (DCA). The prediction power of established model was further validated in two validation groups (Figure 1A). Finally, a risk-assessment model for DILI was developed and displayed as a nomogram graph in the training group.
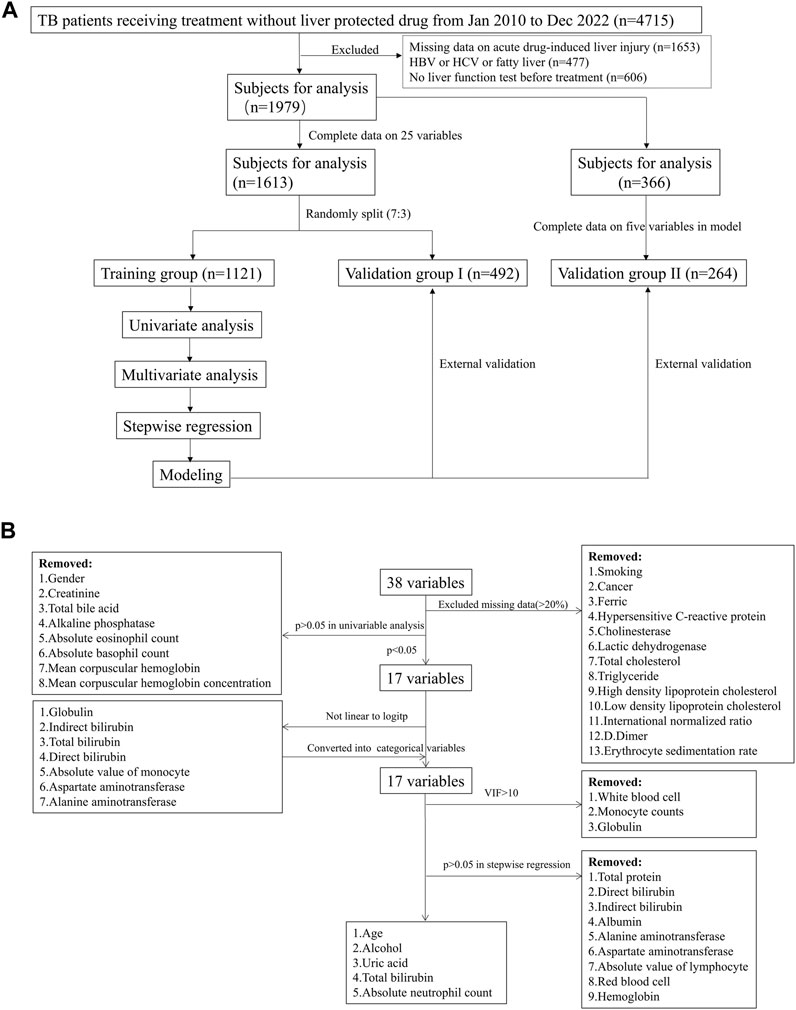
FIGURE 1. Study flowchart in the model establishment. (A) patient inclusion and exclusion in the model; (B) variables screening process in the model.
Statistical analysis
The SPSS (version 23) and R 4.2.1 software for Windows were used for statistical analysis. Continuous variables were expressed as median with the corresponding interquartile range, and were compared using either Student’s t-test or the Mann–Whitney U test. Categorical variables were compared using the χ2 test or Fisher’s exact test. A p-value <0.05 was considered of statistical significance.
Results
Characteristics of the study population
A total of 4,715 patients under anti-TB drug treatment were initially included in this study (Figure 1A). 2,259 patients were excluded due to missing information on liver function test in our hospital after receiving treatment (n = 1,653) or before the drug therapy (n = 606). There were 477 patients owned baseline liver diseases including hepatis B, hepatis C or fatty liver, then were removed in further analysis. In the enrolled 1979 patients, 83 (4.2%) developed DILI within 180 days after treatment. There were 25 variables included in analysis after removing the variables with missing data higher than 20% (Figure 1B). Then, 1,613 patients with complete variables were divided into training group (n = 1,121) and validation group I (n = 492), with no significant differences for the involved variables (Supplementary Table S1).
Identification of the variables to be included in the model
Univariate analysis showed that 17 clinical or laboratory variables were significantly associated with DILI (p < 0.05, Table 1 and Figure 1B). Gender, creatinine, total bile acid, alkaline phosphatase, absolute eosinophil count, absolute basophil count, mean corpuscular hemoglobin and mean corpuscular hemoglobin concentration were not found significantly associated with DILI (p > 0.05). Seven variables were converted into categorical variables, including globulin, indirect bilirubin, total bilirubin, direct bilirubin, absolute value of monocyte, aspartate aminotransferase and alanine aminotransferase due to no linearity to logitp (Figure 1B). Three variables (white blood cell, monocyte counts and globulin) were excluded because of multi-collinearity. Among the remaining variables, there was no multi-collinearity (Supplementary Table S2).
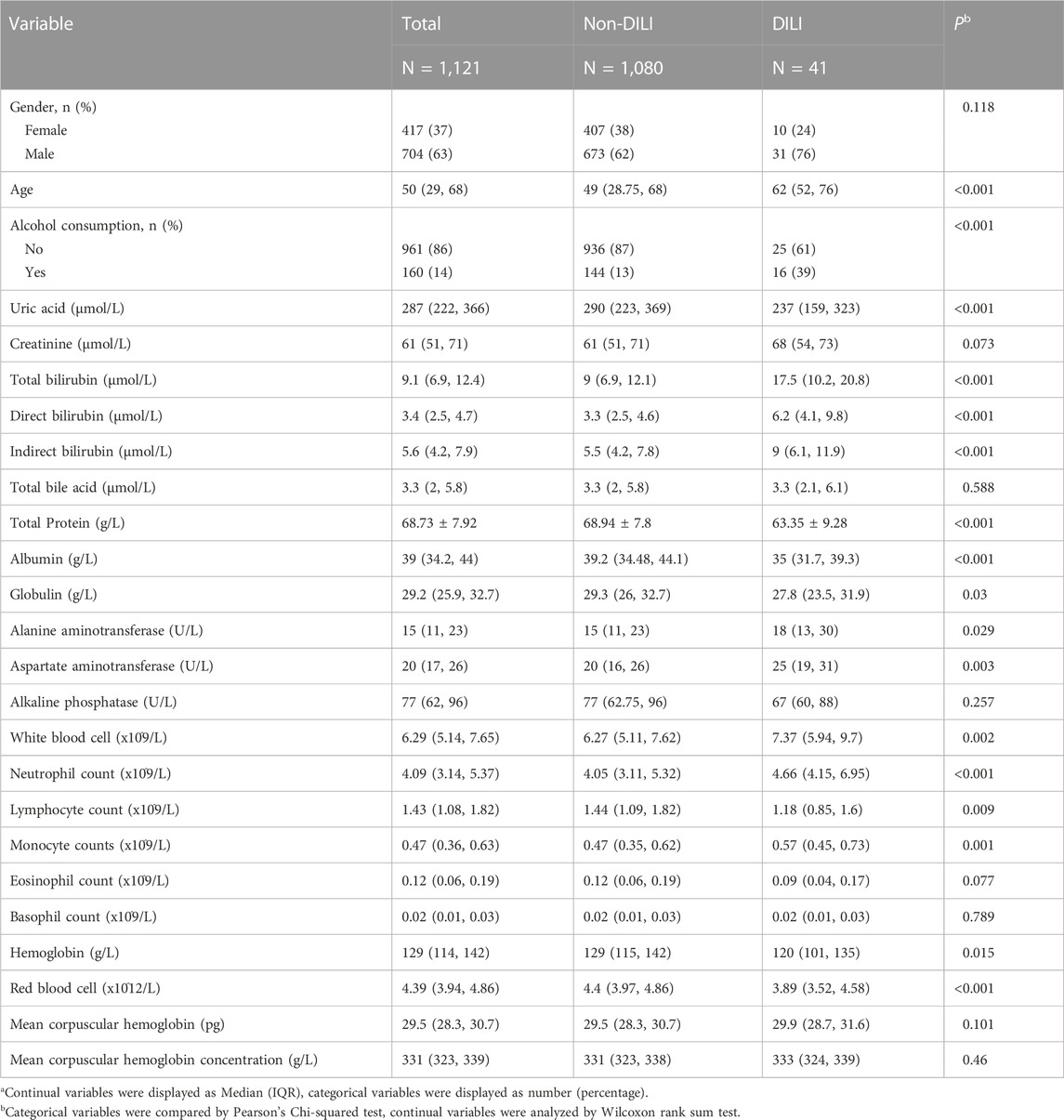
TABLE 1. Univariable analysis of DILI related variables in the training groupa.
Finally, five parameters (age, alcohol, uric acid, total bilirubin and neutrophil count) were significantly related to DILI by stepwise regression (Table 2; Figure 1B). According to odds ratio (OR), the following four parameters were high-risk factors for DILI in patients with TB during anti-TB treatments: age (OR 1.022 [1.003–1.042], p = 0.023), elevated total bilirubin (OR 11.714 [5.837–23.692], p < 0.001), neutrophil count (OR 2.145 [1.078–4.700], p = 0.013), and alcohol consumption (OR 3.209 [1.520–6.652], p = 0.002). Conversely, uric acid (OR 0.977 [0.993–1.000], p = 0.047) was the protective parameter against the development of DILI (Table 2).
Development of a risk-prediction model for drug-induced liver injury in the training group
To evaluate the discriminative ability of the logistic regression model, the final prediction model was constructed in the training group based on five variables: age, elevated total bilirubin, neutrophil count, uric acid and alcohol consumption. The AUC of prediction model was 0.833, with a sensitivity of 0.759 and specificity of 0.805 at the threshold of 0.030 (Figure 2A). The DCA curve of established model was far from the two reference curves (Figure 2B). The calibration curve owned a p-value of 0.800 based on the Hosmer & Lemeshow test (Figure 2C).
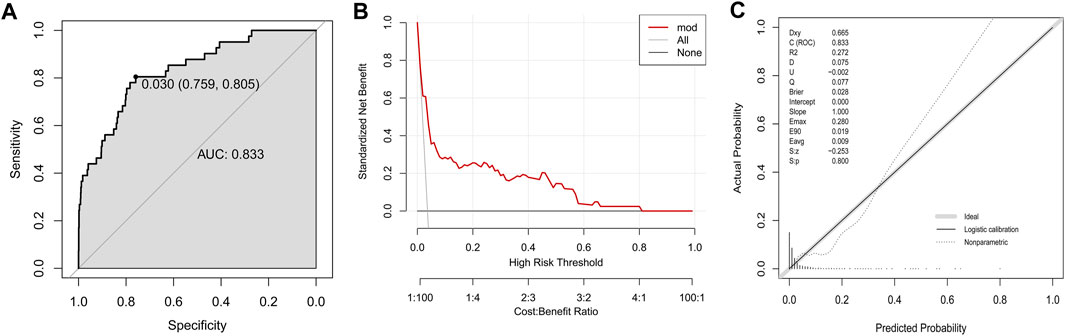
FIGURE 2. Development of a model to predict DILI in the training group. (A) The ROC curve; (B) Decision curve; (C) Calibration plot.
Validation of the risk-prediction model in external data sets
In the validation group I that owned comparable baseline variables to training group, this established model showed a moderate discrimination power (AUC, 0.668, Figure 3A). The DCA curve in the validation group I was near to the two reference lines (Figure 3B). For calibration curve, the p-value based on Hosmer & Lemeshow test was 0.996 (Figure 3C).
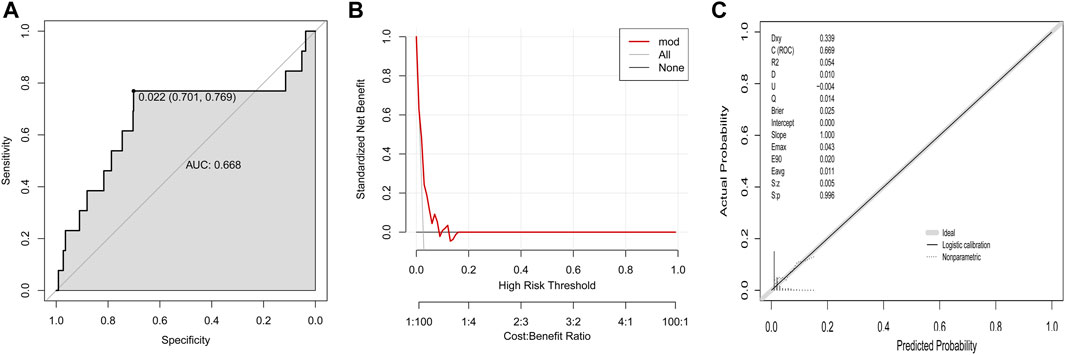
FIGURE 3. Validation of established model to predict DILI risk in the validation group I (A) ROC curve; (B) Decision curve; (C) Calibration plot.
Another validation population (validation group II, n = 264) was extracted from the 1979 patients with complete information on final five variables after excluding those involved in training group and validation group I. Fourteen variables showed significant differences between training group and validation group II (Supplementary Table S1). The established prediction model in validation group II owned an AUC of 0.753 (Figure 4A), a DCA curve far from the two reference lines (Figure 4B) and a p-value of 0.853 in calibration curve (Figure 4C).
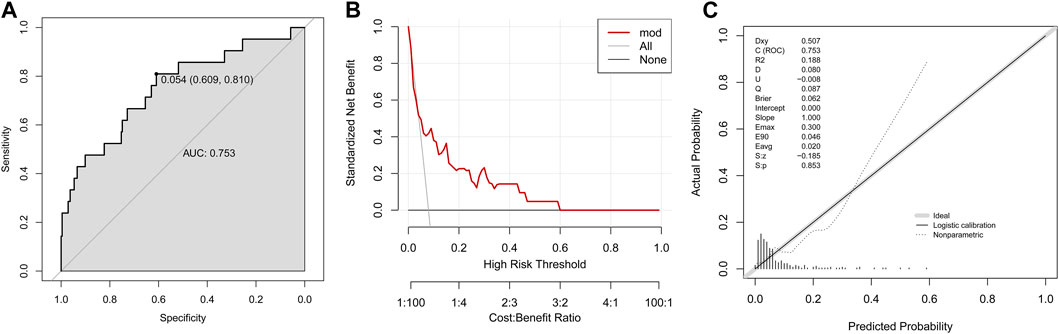
FIGURE 4. Validation of established model to predict DILI risk in the validation group II. (A) ROC curve; (B) Decision curve; (C) Calibration plot.
Establishment of a nomogram graph
Based on the established logistic regression model, a nomogram for presenting the significance of involved variables for predicting the risk of DILI among the patients was established (Figure 5). Individual points corresponding to each measured variable could be obtained by vertically matching them to the top reference point line, and total point could be calculated by summing all the individual points. The risk of DILI could be read by vertically matching the total point to the risk line.
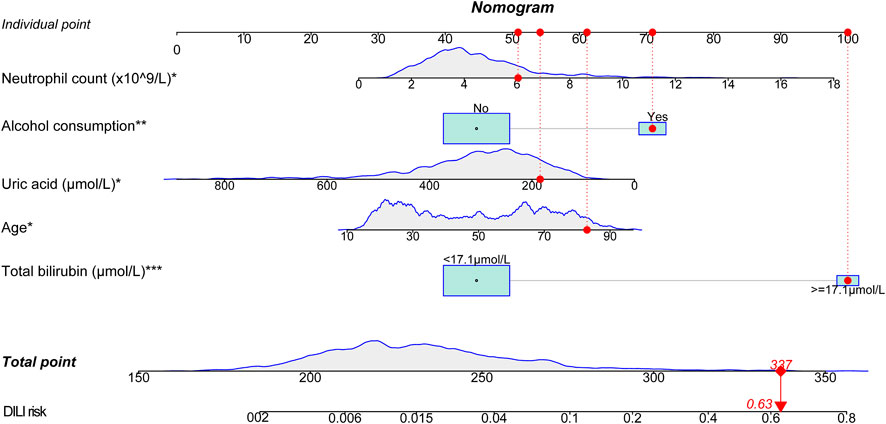
FIGURE 5. Nomogram for predicting DILI in the training group. A patient was given as an example indicated by red dots in figure (81 years old, total bilirubin of 52 μmol/L, uric acid of 159 μmol/L, neutrophil count of 6.41 × 10^9/L, with alcohol consumption). The total score is 337 points, corresponding to a DILI risk of 0.63. The variables with symbol * indicated for significance in the model, *p < 0.05, **p < 0.01, ***p < 0.001.
Discussion
DILI in patients receiving anti-TB agents can interrupt the therapy process, and identification of associated risk factors can help clinical staff to take preventive measures against it. Here, a nomogram model was established based on five clinical parameters (age and levels of total bilirubin, uric acid, neutrophil account, and alcohol consumption) assessed before the treatment onset. This model could predict the DILI risk in patients receiving first-line anti-TB agents who did not take any hepatoprotectants, and has a good discrimination power.
A 6-month regimen is recommended by WHO composing of four first-line anti-TB agents (isoniazid, rifampicin, ethambutol, and pyrazinamide) for patients with TB (W HO, 2022). The regimen could be modified based on the age of the patient, resulting in a treatment success rate >85%. However, DILI is a serious side effect of such regimens, with an incidence rate of 2.0%–28%, and may largely be due to the pyrazinamide in the regimen (Ghosh et al., 2021). Among the patients analyzed in this study, 4.3% suffered from DILI. The incidence of hepatotoxicity in this study might be lower than that reported by others, as patients with baseline liver diseases and receiving liver protective drugs were excluded (Abbara et al., 2017; Latief et al., 2017; Raj Mani et al., 2021). This study assessed several possible factors for their ability to predict future DILI among TB patients negative for liver-related diseases, who might be easily ignored by doctors. Moreover, hepatoprotectants were not used in enrolled patients, giving the chance to investigate the real DILI incidence and related variables (Chen et al., 2022).
In this study, five objective parameters were involved in the prediction model, and these parameters can be easily obtained in clinical practice before the anti-tuberculosis treatment. Elevated levels of total bilirubin might be associated with metabolism dysfunction in liver, eventually increasing the DILI risk once the treatment starts. As the sum of indirect bilirubin and direct bilirubin, the increased total bilirubin might be the results of reduced uptake ability by the liver and weakened excretion by the kidney. In this study, the kidney related variable creatinine was comparable between patients with DILI and without DILI, indicating for key role of liver dysfunction in elevated total bilirubin instead of kidney impairment. Though other studies have indicated the significance of ALT and AST levels for predicting DILI, these two variables were not the significant factors in this study (Mushiroda et al., 2016; Jiang et al., 2021). The inconsistency could be partly due to the differences in patients’ inclusion criteria, as patients with baseline liver diseases owned higher DILI risks (Jiang et al., 2021). In this study, uric acid was negatively associated with DILI risk, which could be partly explained by its anti-oxidant role in plasma (El Ridi and Tallima, 2017). In another way, lower uric acid in plasma might be caused by impaired reabsorption by kidney, with consequently increased uric acid in urine from patients with DILI revealed by urine metabolomics (Wang et al., 2022b). Moreover, neutrophil was positively associated with the risk of DILI in this study, which is consistent to the fact that neutrophil is always involved in inflammation and excessive or dysfunctional neutrophils lead into tissue damage (Wang and Liu, 2021). The high level of neutrophil in blood would provide enough source for infiltrating into liver when accumulative cytotoxic drug metabolites impaired the hepatocytes, resulting into an increased risk of liver injury (Cho and Szabo, 2021). Alcohol consumption and age were also found closely associated with DILI in this study, and these two factors have been widely reported by others (Abbara et al., 2017; Yew et al., 2018; Song et al., 2019).
Although several studies on DILI risk factors have been conducted, these studies involved limited numbers of patients and mostly focused on screening for risk factors (Lee et al., 2016; Medina-Caliz et al., 2016; Mushiroda et al., 2016; Raj Mani et al., 2021). Our nomogram prediction model could show the exact risk based on five parameters and has a good predictive ability regarding the ROC value, sensitivity, and specificity (Zhao et al., 2022). By using the XGBoost algorithm, Zhong et al. have established a model that can predict DILI with high accuracy and interpretability. However, their model involves the accumulative dose of anti-TB agents used during a treatment course, and this dose cannot be foreseen before starting the treatment (Zhong et al., 2021). In the validation step, two groups were enrolled in this study, representing for populations with comparable baselines and with different baselines, respectively. The prediction ability of established model in training model reduced in validate group with comparable baselines, which might be caused by limited DILI cases in validation. When validated in group with different baselines, the model showed increased prediction ability comparing to group with comparable baselines, suggesting its application possibility in other population.
There were some limitations in this study. Despite the large sample size, all the patients were from a single center, and thus the prediction power of established model need be validated in other regions. The duration between the treatment onset and DILI occurrence was not available from the database. Several parameters were not available for some of the involved patients due to the retrospective nature of the study, resulting in the exclusion of more than 50% of the patients. In addition, the treatment duration and accumulative drug dosage were not available from the database.
Conclusion
TB patients frequently suffer from DILI after receiving first-line anti-TB agents, though they were not accompanied by liver-related diseases before treatment, including HBV infection, fatty liver and liver cancers. A nomogram model based on five variables before anti-TB treatment (age, total bilirubin, neutrophil count, alcohol consumption and uric acid) can predict future DILI with a good discriminative power. This prediction model based on these easily accessible parameters can help clinical staff to evaluate the DILI risk before the treatment and take active intervenes to prevent the occurrence of DILI in patients with high risk.
Data availability statement
The original contributions presented in the study are included in the article/Supplementary Material, further inquiries can be directed to the corresponding author.
Ethics statement
The studies involving human participants were reviewed and approved by the Ethics Committee and Institutional Review Board of Dongyang People’s Hospital (No: 2021-YX-221). Written informed consent for participation was not required for this study in accordance with the national legislation and the institutional requirements.
Author contributions
SJ and XP designed the study, established and validated the prediction model, and wrote the manuscript; BL collected and analyzed the data. All authors listed have made a substantial, direct, and intellectual contribution to the work and approved it for publication.
Funding
This study is supported by the Public Science and Technology Research Project of Jinhua under 2022-4-262.
Conflict of interest
The authors declare that the research was conducted in the absence of any commercial or financial relationships that could be construed as a potential conflict of interest.
Publisher’s note
All claims expressed in this article are solely those of the authors and do not necessarily represent those of their affiliated organizations, or those of the publisher, the editors and the reviewers. Any product that may be evaluated in this article, or claim that may be made by its manufacturer, is not guaranteed or endorsed by the publisher.
Supplementary material
The Supplementary Material for this article can be found online at: https://www.frontiersin.org/articles/10.3389/fphar.2023.1153815/full#supplementary-material
References
Abbara, A., Chitty, S., Roe, J. .K., Ghani, R., Collin, S. .M., Ritchie, A., et al. (2017). Drug-induced liver injury from antituberculous treatment: A retrospective study from a large TB centre in the UK. BMC Infect. Dis. 17 (1), 231. doi:10.1186/s12879-017-2330-z
Ahmad, N., Javaid, A., Syed Sulaiman, S. .A., Afridi, A. .K., Zainab, A., and Khan, A. .H. (2018). Occurrence, management, and risk factors for adverse drug reactions in multidrug resistant tuberculosis patients. Am. J. Ther. 25 (5), e533–e540. doi:10.1097/MJT.0000000000000421
Ashby, K., Zhuang, W., Gonzalez-Jimenez, A., Alvarez-Alvarez, I., Lucena, M. .I., Andrade, R. .J., et al. (2021). Elevated bilirubin, alkaline phosphatase at onset, and drug metabolism are associated with prolonged recovery from DILI. J. Hepatol. 75 (2), 333–341. doi:10.1016/j.jhep.2021.03.021
Chang, T. .E., Huang, Y. .S., Chang, C. .H., Perng, C. .L., Huang, Y. .H., and Hou, M. .C. (2018). The susceptibility of anti-tuberculosis drug-induced liver injury and chronic hepatitis C infection: A systematic review and meta-analysis. J. Chin. Med. Assoc. 81 (2), 111–118. doi:10.1016/j.jcma.2017.10.002
Chen, Q., Hu, A., Ma, A., Jiang, F., Xiao, Y., Chen, Y., et al. (2022). Effectiveness of prophylactic use of hepatoprotectants for tuberculosis drug-induced liver injury: A population-based cohort analysis involving 6,743 Chinese patients. Front. Pharmacol. 13, 813682. doi:10.3389/fphar.2022.813682
Cho, Y., and Szabo, G. (2021). Two faces of neutrophils in liver disease development and progression. Hepatology 74 (1), 503–512. doi:10.1002/hep.31680
Deng, Y., Luo, X., Li, X., Xiao, Y., Xu, B., and Tong, H. (2022). Screening of biomarkers and toxicity mechanisms of rifampicin-induced liver injury based on targeted bile acid metabolomics. Front. Pharmacol. 13, 925509. doi:10.3389/fphar.2022.925509
El Hamdouni, M., Ahid, S., Bourkadi, J. .E., Benamor, J., Hassar, M., and Cherrah, Y. (2020). Incidence of adverse reactions caused by first-line anti-tuberculosis drugs and treatment outcome of pulmonary tuberculosis patients in Morocco. Infection 48 (1), 43–50. doi:10.1007/s15010-019-01324-3
El Ridi, R., and Tallima, H. (2017). Physiological functions and pathogenic potential of uric acid: A review. J. Adv. Res. 8 (5), 487–493. doi:10.1016/j.jare.2017.03.003
Ghosh, A., Onsager, C., Mason, A., Arriola, L., Lee, W., and Mubayi, A. (2021). The role of oxygen intake and liver enzyme on the dynamics of damaged hepatocytes: Implications to ischaemic liver injury via a mathematical model. PLoS One 16 (4), e0230833. doi:10.1371/journal.pone.0230833
Global tuberculosis report 2021 (2021). Global tuberculosis report 2021. Geneva: World Health Organization. Licence: CC BY-NC-SA 3.0 IGO.
Global tuberculosis report 2022 (2022). Global tuberculosis report 2022. Geneva: World Health Organization. Licence: CC BY-NC-SA 3.0 IGO.
Ho, C. .M., Chen, C. .L., Chang, C. .H., Lee, M. .R., Wang, J. .Y., Hu, R. .H., et al. (2021). Circulatory inflammatory mediators in the prediction of anti-tuberculous drug-induced liver injury using RUCAM for causality assessment. Biomedicines 9 (8), 891. doi:10.3390/biomedicines9080891
Jiang, F., Yan, H., Liang, L., Du, J., Jin, S., Yang, S., et al. (2021). Incidence and risk factors of anti-tuberculosis drug induced liver injury (DILI): Large cohort study involving 4652 Chinese adult tuberculosis patients. Liver Int. 41 (7), 1565–1575. doi:10.1111/liv.14896
Lan, Z., Ahmad, N., Baghaei, P., Barkane, L., Benedetti, A., Brode, S. .K., et al. (2020). Drug-associated adverse events in the treatment of multidrug-resistant tuberculosis: An individual patient data meta-analysis. Lancet Respir. Med. 8 (4), 383–394. doi:10.1016/S2213-2600(20)30047-3
Latief, M., Dar, W. .R., Sofi, N., Dar, I. .A., Kasana, B., Hussain, M., et al. (2017). Novel risk factors and early detection of anti tubercular treatment induced liver injury-Looking beyond American Thoracic Society Guidelines. Indian J. Tuberc. 64 (1), 26–32. doi:10.1016/j.ijtb.2016.11.002
Lee, C. .M., Lee, S. .S., Lee, J. .M., Cho, H. .C., Kim, W. .S., Kim, H. .J., et al. (2016). Early monitoring for detection of antituberculous drug-induced hepatotoxicity. Korean J. Intern Med. 31 (1), 65–72. doi:10.3904/kjim.2016.31.1.65
Medina-Caliz, I., Robles-Diaz, M., Garcia-Munoz, B., Stephens, C., Ortega-Alonso, A., Garcia-Cortes, M., et al. (2016). Definition and risk factors for chronicity following acute idiosyncratic drug-induced liver injury. J. Hepatol. 65 (3), 532–542. doi:10.1016/j.jhep.2016.05.003
Mushiroda, T., Yanai, H., Yoshiyama, T., Sasaki, Y., Okumura, M., Ogata, H., et al. (2016). Development of a prediction system for anti-tuberculosis drug-induced liver injury in Japanese patients. Hum. Genome Var. 3, 16014. doi:10.1038/hgv.2016.14
Raj Mani, S. .S., Iyyadurai, R., Mishra, A. .K., Manjunath, K., Prasad, J., Lakshmanan, J., et al. (2021). Predicting antitubercular drug-induced liver injury and its outcome and introducing a novel scoring system. Int. J. Mycobacteriol 10 (2), 116–121. doi:10.4103/ijmy.ijmy_15_21
Saito, Z., Kaneko, Y., Kinoshita, A., Kurita, Y., Odashima, K., Horikiri, T., et al. (2016). Effectiveness of hepatoprotective drugs for anti-tuberculosis drug-induced hepatotoxicity: A retrospective analysis. BMC Infect. Dis. 16 (1), 668. doi:10.1186/s12879-016-2000-6
Song, J. .H., Yoon, S. .Y., Park, T. .Y., Heo, E. .Y., Kim, D. .K., Chung, H. .S., et al. (2019). The clinical impact of drug-induced hepatotoxicity on anti-tuberculosis therapy: A case control study. Respir. Res. 20 (1), 283. doi:10.1186/s12931-019-1256-y
Sterling, T. .R., Villarino, M. .E., Borisov, A. .S., Shang, N., Gordin, F., Bliven-Sizemore, E., et al. (2011). Three months of rifapentine and isoniazid for latent tuberculosis infection. N. Engl. J. Med. 365 (23), 2155–2166. doi:10.1056/NEJMoa1104875
Sun, Q., Zhang, Q., Gu, J., Sun, W. .W., Wang, P., Bai, C., et al. (2016). Prevalence, risk factors, management, and treatment outcomes of first-line antituberculous drug-induced liver injury: A prospective cohort study. Pharmacoepidemiol Drug Saf. 25 (8), 908–917. doi:10.1002/pds.3988
WHO, WHO consolidated guidelines on tuberculosis. Module 4: Treatment - drug-susceptible tuberculosis treatment. Geneva: World Health Organization; 2022. Licence: CC BY-NC-SA 3.0 IGO.
Wang, C. .Y., Deng, Y., Li, P., Zheng, S., Chen, G., Zhou, G., et al. (2022). Prediction of biochemical nonresolution in patients with chronic drug-induced liver injury: A large multicenter study. Hepatology 75 (6), 1373–1385. doi:10.1002/hep.32283
Wang, M. .G., Wu, S. .Q., Zhang, M. .M., and He, J. .Q. (2022). Plasma metabolomic and lipidomic alterations associated with anti-tuberculosis drug-induced liver injury. Front. Pharmacol. 13, 1044808. doi:10.3389/fphar.2022.1044808
Wang, M. .G., Wu, S. .Q., Zhang, M. .M., and He, J. .Q. (2022). Urine metabolomics and microbiome analyses reveal the mechanism of anti-tuberculosis drug-induced liver injury, as assessed for causality using the updated rucam: A prospective study. Front. Immunol. 13, 1002126. doi:10.3389/fimmu.2022.1002126
Wang, Y., and Liu, Y. (2021). Neutrophil-induced liver injury and interactions between neutrophils and liver sinusoidal endothelial cells. Inflammation 44 (4), 1246–1262. doi:10.1007/s10753-021-01442-x
Xu, L., Zhang, F., Xu, C., Liu, K. .G., Wu, W., and Tian, Y. .X. (2017). Is the prophylactic use of hepatoprotectants necessary in anti-tuberculosis treatment? Chemotherapy 62 (5), 269–278. doi:10.1159/000465515
Xu, N., Yang, J. .X., and Yang, J. (2022). Incidence and associated risk factors of antituberculosis drug-induced hepatotoxicity among hospitalised patients in Wuhan, China. Eur. J. Hosp. Pharm. 29 (4), 217–221. doi:10.1136/ejhpharm-2020-002433
Yew, W. .W., Chang, K. .C., and Chan, D. .P. (2018). Oxidative stress and first-line antituberculosis drug-induced hepatotoxicity. Antimicrob. Agents Chemother. 62 (8), 026377–e2717. doi:10.1128/AAC.02637-17
Zhang, T., Du, J., Yin, X., Xue, F., Liu, Y., Li, R., et al. (2015). Adverse events in treating smear-positive tuberculosis patients in China. Int. J. Environ. Res. Public Health 13 (1), 86. doi:10.3390/ijerph13010086
Zhao, P., Chen, J., Yang, Y. .Q., and Peng, Y. (2022). [Nomogram model for predicting risk of anti-tuberculosis drug-induced liver injury among inpatients with tuberculosis]. Zhonghua Jie He He Hu Xi Za Zhi 45 (2), 171–176. doi:10.3760/cma.j.cn112147-20210705-00467
Keywords: nomogram, drug-induced liver injury, anti-tuberculosis treatment, prediction model, risk factors
Citation: Ji S, Lu B and Pan X (2023) A nomogram model to predict the risk of drug-induced liver injury in patients receiving anti-tuberculosis treatment. Front. Pharmacol. 14:1153815. doi: 10.3389/fphar.2023.1153815
Received: 07 February 2023; Accepted: 09 May 2023;
Published: 18 May 2023.
Edited by:
Zhengsheng Zou, Fifth Medical Center of the PLA General Hospital, ChinaCopyright © 2023 Ji, Lu and Pan. This is an open-access article distributed under the terms of the Creative Commons Attribution License (CC BY). The use, distribution or reproduction in other forums is permitted, provided the original author(s) and the copyright owner(s) are credited and that the original publication in this journal is cited, in accordance with accepted academic practice. No use, distribution or reproduction is permitted which does not comply with these terms.
*Correspondence: Xinling Pan, cGFuZmVuZ3l1d3V6dUAxNjMuY29t