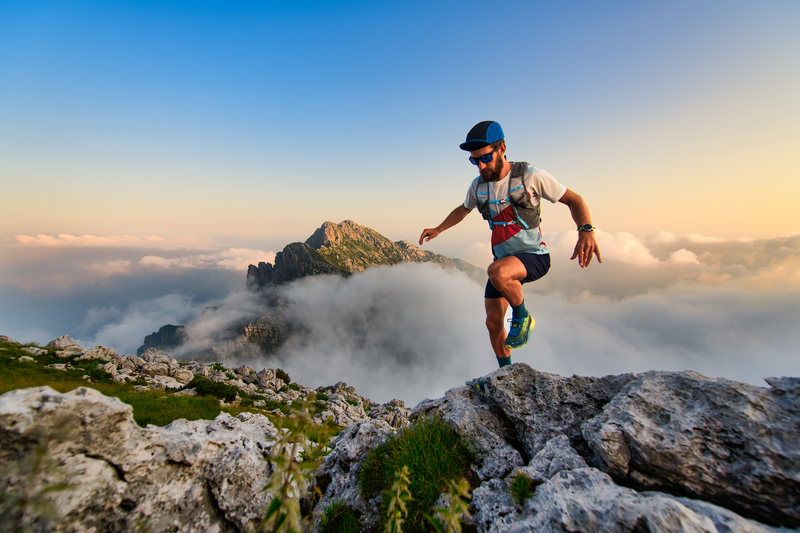
94% of researchers rate our articles as excellent or good
Learn more about the work of our research integrity team to safeguard the quality of each article we publish.
Find out more
ORIGINAL RESEARCH article
Front. Pharmacol. , 23 September 2022
Sec. Obstetric and Pediatric Pharmacology
Volume 13 - 2022 | https://doi.org/10.3389/fphar.2022.957980
This article is part of the Research Topic Precision Medicine in Pediatrics View all 25 articles
Background: Drug-induced kidney injury (DIKI) is one of the most common complications in clinical practice. Detection signals through post-marketing approaches are of great value in preventing DIKI in pediatric patients. This study aimed to propose a quantitative algorithm to detect DIKI signals in children using an electronic health record (EHR) database.
Methods: In this study, 12 years of medical data collected from a constructed data warehouse were analyzed, which contained 575,965 records of inpatients from 1 January 2009 to 31 December 2020. Eligible participants included inpatients aged 28 days to 18 years old. A two-stage procedure was adopted to detect DIKI signals: 1) stage 1: the suspected drugs potentially associated with DIKI were screened by calculating the crude incidence of DIKI events; and 2) stage 2: the associations between suspected drugs and DIKI were identified in the propensity score-matched retrospective cohorts. Unconditional logistic regression was used to analyze the difference in the incidence of DIKI events and to estimate the odds ratio (OR) and 95% confidence interval (CI). Potentially new signals were distinguished from already known associations concerning DIKI by manually reviewing the published literature and drug instructions.
Results: Nine suspected drugs were initially screened from a total of 652 drugs. Six drugs, including diazepam (OR = 1.61, 95%CI: 1.43–1.80), omeprazole (OR = 1.35, 95%CI: 1.17–1.54), ondansetron (OR = 1.49, 95%CI: 1.36–1.63), methotrexate (OR = 1.36, 95%CI: 1.25–1.47), creatine phosphate sodium (OR = 1.13, 95%CI: 1.05–1.22), and cytarabine (OR = 1.17, 95%CI: 1.06–1.28), were demonstrated to be associated with DIKI as positive signals. The remaining three drugs, including vitamin K1 (OR = 1.06, 95%CI: 0.89–1.27), cefamandole (OR = 1.07, 95%CI: 0.94–1.21), and ibuprofen (OR = 1.01, 95%CI: 0.94–1.09), were found not to be associated with DIKI. Of these, creatine phosphate sodium was considered to be a possible new DIKI signal as it had not been reported in both adults and children previously. Moreover, three other drugs, namely, diazepam, omeprazole, and ondansetron, were shown to be new potential signals in pediatrics.
Conclusion: A two-step quantitative procedure to actively explore DIKI signals using real-world data (RWD) was developed. Our findings highlight the potential of EHRs to complement traditional spontaneous reporting systems (SRS) for drug safety signal detection in a pediatric setting.
Drug-induced kidney injury (DIKI), as one of the most common adverse drug reactions (ADRs), is a significantly increased clinical problem worldwide. It may lead to clinical symptoms such as oliguria, anuria, and acute renal failure (Radi, 2019). Studies have shown that the incidence of DIKI in hospitalized patients is about 2%–5%, accounting for 19%–40% of acute kidney injury (AKI) (Hosohata, 2016). Children are at higher risk of developing DIKI compared with adults; several factors contribute to the pediatric renal damage onset, including the immaturity of renal functions and specific pathological conditions (Faught et al., 2015a). Data from critically ill children who have DIKI suggest that survivors develop a risk for the development of chronic kidney disease (Hanna et al., 2016). In addition to that, DIKI is more likely to occur in the post-marketing of drugs than in the pre-marketing setting as its incidence is low (Faught et al., 2015b). This is particularly true for children since they are not frequently included in clinical trials. It is of great value to prevent and reduce DIKI in pediatrics through a post-marketing approach.
In most countries all over the world, national pharmacovigilance relies heavily on spontaneous reporting systems (SRS), in which suspected ADRs are reported to a national coordinating center by health professionals, manufacturers, or directly by patients. However, as a passive monitoring method, SRS has its inherent limitations, such as poor-quality reports, underreporting, and the inability to estimate rates and frequencies of ADRs (Pitts and Le Louet, 2018). Previous studies have demonstrated that 50%–90% of DIKI were unreported using SRS (Yang et al., 2015). Additional resources and methods are, therefore, needed to actively monitor the quantitative aspects of drug safety and to characterize ADRs associated with specific drugs and in specific populations. Recently, real-world data (RWD), especially data collected during routine clinical care in the form of electronic health records (EHRs), have been adopted by healthcare professionals, regulators, and providers to inform decision-making about drug safety. Several emerging pharmacovigilance programs extend the pharmacovigilance capabilities by monitoring drug safety signals actively using EHRs, such as the “Sentinel Initiative” in the United States (Toh et al., 2016), the “Exploring and Understanding Adverse Drug Reaction Project (EU-ADR)" in Europe (de Bie et al., 2015), and the “Adverse Drug Events Active Surveillance and Assessment System (ADE-ASAS)” in China (Chen et al., 2020). More attempts have also been made to develop data-mining methods based on EHRs, such as the “Global Trigger Tool (GTT)” (Garrett et al., 2013) and the “Comparison of the Laboratory Extreme Abnormality Ratio (CLEAR) algorithm” (Yoon et al., 2012). However, these methods are not applicable for the detection of DIKI signals because the confounding factors affecting DIKI are not fully considered. Moreover, most of these studies are conducted on adults, and so far, little is known about pediatric patients in relation to this issue.
Hence, the present study aims at developing a novel quantitative algorithm for DIKI signal detection using a hospital electronic medical record database and analyzing the correlation and characteristics of DIKI signals with the specific drugs in the pediatric population.
This study was conducted using the retrospective inpatient data warehouse of Beijing Children’s Hospital (BCH) from 1 January 2009 to 31 December 2020 (Yu et al., 2020). Approximately, 575,965 records of inpatients under 18 years old were included along with their detailed diagnoses, medications, and laboratory tests. Considering the immature kidney function in the neonates, only inpatients aged 28 days to 18 years old were included. All eligible patient data were exported and de-identified to protect their privacy.
The protocol of the study was approved by the Institutional Review Board (IRB) of BCH, Capital Medical University (approval number: 2018-129), with a waiver of informed consent. This work was reported critically according to the RECORD statement for pharmacoepidemiology (RECORD-PE) statement (Supplementary Table S1).
According to the published Kidney Disease: Improving Global Outcomes (KDIGO) (Ostermann et al., 2020), the serum creatinine (SCr) and the glomerular filtration rate (GFR) were the most important renal function parameters for determining DIKI. Because of the difficulty in measuring directly in clinical settings, the estimated GFR (eGFR) was usually used instead of the GFR and was calculated using the following formula, based on Scr and the normalization constant (Q) (Hoste et al., 2014):
(For children, Q is the median or the average Scr concentration for healthy children and depends linearly on age)
The fourth-degree polynomials for Q are as follows:
After completing the calculation, the normal pediatric reference interval of the eGFR was as follows: 33–84(3–6 months), 57–122(6–12 months), 78–132(12–15 months), 84–150(15–24 months), and 83–143 (24 months–18 years old).
Thus, according to the KDIGO guidelines and the reference interval of pediatric kidney parameters (Zhu, 2015), the trigger for pediatric DIKI was defined as the following events that occurred after medication within the appropriate therapeutic dose range: (1):SCr>130 mmol/L; or (2) out of the reference interval of the eGFR for pediatrics.
The main purpose of this step was to identify the suspected drugs that may cause DIKI to provide candidate drugs for the subsequent correlation analysis. The main steps were as follows (Figure 1):
1) The records with at least two SCr tests in the eligible participants were selected.
2) The records with the initial SCr or eGFR value within the reference range were further included. The report time of SCr tests was recorded as T1. This step aimed to ensure that kidney function was normal before medication.
3) The records with the diagnosis of kidney-related diseases were excluded (Supplementary Table S2). This step aimed to exclude the records where changes in laboratory parameters were primarily due to the progression of the kidney-related disease itself, rather than DIKI. The remaining records were marked as group 1.
4) The records with abnormal SCr or eGFR test findings, which were considered as DIKI events, were marked as group 2. The time of the first abnormal result was recorded as T2.
5) All drugs during T1-T2 were extracted, and duplicates were removed from groups 1 and 2. Thus, the number of DIKI events (a) and the total number of drug users (b) of each drug were obtained.
6) We calculated the ratio of a/b for each drug. The threshold of the a/b ratio was set according to the range of a/b values of solvents for intravenous infusions, such as normal saline and glucose injections, which can be regarded as the reference value since it is well known that they do not affect DIKI. The a/b values of solvents for intravenous infusions ranged from 0.081 to 0.095. In addition to that, for drugs with less than 2000 users, the number of DIKI events in the exposed group was too low. There may be a greater risk of bias in the subsequent statistical analysis due to insufficient samples. Thus, the criteria for suspected drugs were as follows: (1): ratio (a/b) > 0.10 and (2) b > 2000.
FIGURE 1. Workflow for identifying potential DIKI signals based on the retrospective cohort design. Abbreviations: DIKI: drug-induced kidney injury; EHRs: electronic health records. SCr: serum creatinine; eGFR: estimate glomerular filtration rate; OR: odds ratio.
This step aimed to compare the incidence of DIKI events between the exposed group (i.e., taking the suspected drugs) and the unexposed group (i.e., not taking the suspected drugs) whilst also adjusting for confounding factors through retrospective analysis. This was performed to explore the association between drugs and kidney damage. The following analysis was performed on all suspected drugs found in stage 1 (Figure 1):
1) All records containing suspected drugs were identified.
2) The records with at least one SCr test before and after medication were screened.
3) The records with an initial SCr or eGFR result that was within the normal range before the first medication were included.
4) The records with competing kidney diseases were excluded (Supplementary Table S2).
5) In cases where records showing abnormal SCr or eGFR findings during hospitalization, the records with kidney-protecting drugs before the first report time of the abnormal test were excluded (Supplementary Table S3); for records without SCr or eGFR abnormalities, those who have used kidney-protecting drugs during the entire hospitalization were excluded.
1) All records without the suspected drug were identified.
2) The records with at least two SCr tests during hospitalization remained.
3) The records with an initial SCr or eGFR that was within the normal range after admission were included.
4) The records with competing kidney diseases were excluded (Supplementary Table S2).
5) In cases where records showing abnormal SCr or eGFR findings during hospitalization, the records with kidney-protecting drugs before the first report time of the abnormal test were excluded (Supplementary Table S3); for records without SCr or eGFR abnormalities, those who have used kidney-protecting drugs during the entire hospitalization were excluded.
1) Each exposed record was paired with four unexposed records randomly after adjusting age, gender, admission time, and major diagnosis.
2) The association between suspected drugs and kidney injury was analyzed by unconditional logistic regression. The odds ratio (OR) and 95% confidence interval (CI) were calculated.
3) An OR>1.0 (with the 95%CI lower band >1) indicated a positive signal; otherwise, a negative signal was considered.
A literature search and the summary of product characteristics (SPCs) were used as available knowledge to evaluate the novelty of the positive DIKI signals. Type I signals were defined as those signals that have not been previously documented in research in both children and adults. Type II signals were defined as those drug–DIKI associations that have not been reported in children, although they have been reported in adults. The SPCs were checked from the FDA website (https://www.fda.gov), Micromedex (https://www.ibm.com/watson-health/learn/micromedex), and the drug instructions (https://www.yaozh.com/). Literature research was conducted using PubMed (https://pubmed.ncbi.nlm.nih.gov), Embase (https://www.embase.com), Wanfang (http://www.wanfang.data.com.cn/index.html), and CNKI (http://www. cnki.net/).
MySQL software version 14.14 (Oracle, California, United States) was used as a database management system. The pandas v1.2.2 package in Python 3.7 was performed to summarize data. R 4.2.0 software was used for statistical analysis. The forest plot was visualized by GraphPad Prism 9.3 software.
The propensity score matching (PSM) was used to match the exposed group and unexposed group for the ratio of 1:4. As demographic variables, the distribution of age and gender needs to be comparable in exposed and unexposed groups. Laboratory testing equipment, methods, and capability may change over time in the hospital, which can affect the results of laboratory parameters such as Scr. The patients admitted at similar times in the two groups were, therefore, more comparable. In addition, as the patient’s underlying medical condition may affect the effectiveness of the drugs on renal function, cases with similar diagnoses in two groups need to be matched. Thus, four variables, namely, age, gender, admission time, and main diagnosis, were considered as confounding factors. The logistic regression model was used to calculate propensity scores, with drug exposure or not as dependent variables and four confounding factors as covariates. The nearest neighbor matching principle was used with a caliper of 0.1.
An unconditional logistic regression was used to analyze the association between suspected drugs and kidney injury. All p-values were reported as two-sided, and p < 0.05 represented statistical significance.
A total of 652 drugs were initially screened in stage 1. After combining drugs with the same ingredients and anatomical therapeutic chemical (ATC) classification, 346 drugs remained. After excluding external drugs and solvents for intravenous infusions (such as normal saline and glucose injection), 48 drugs were left (Supplementary Table S4). According to the inclusion criteria (a/b > 0.10 and b > 2000), a total of nine suspected drugs (diazepam, vitamin K1, cefamandole, omeprazole, ondansetron, ibuprofen, methotrexate, creatine phosphate sodium, and cytarabine) were finally identified. More information is shown in Table 1.
The data extraction workflow of the nine suspected drugs is shown in Supplementary Table S5. The clinical information between the exposed group and the unexposed group is described in Supplementary Table S6. Retrospective cohort analysis showed that nine drugs met the inclusion criteria. Six drugs, namely, diazepam (p = 3.47 × 10–15, OR = 1.61, and 95% CI: 1.43–1.80), omeprazole (p = 4.46 × 10–5, OR = 1.35, and 95% CI: 1.17–1.54), ondansetron (p = 1.95 × 10–16, OR = 1.49, and 95% CI: 1.36–1.63), methotrexate (p = 1.17 × 10–13, OR = 1.36, and 95% CI: 1.25–1.47), creatine phosphate sodium (p = 2.94 × 10–3, OR = 1.13, and 95% CI: 1.05–1.22), and cytarabine (p = 1.76 × 10–3, OR = 1.17, and 95% CI: 1.06–1.28), were found to be associated with DIKI as positive signals. Although the three remaining drugs, namely, vitamin K1 (p = 0.54, OR = 1.06, and 95% CI: 0.89–1.27), cefamandole (p = 0.40, OR = 1.07, and 95% CI: 0.94–1.21), and ibuprofen (p = 0.77, OR = 1.01, and 95% CI: 0.94–1.09), tended toward a positive association with kidney injury, it did not reach statistical significance. The results of nine drugs and their associations with DIKI are shown in Table 2 and Figure 2.
FIGURE 2. Forest plot for the association of suspected drugs with DIKI. Abbreviations: DIKI: drug-induced kidney injury; OR: odds ratio; CI: confidence interval.
According to the current available knowledge, the novelty of the six positive DIKI signals observed in stage 2 was further evaluated (Table 3). Of these, creatine phosphate sodium was found to be a type I signal as it had not previously been reported in either adults or children. Three other drugs, namely, diazepam, omeprazole, and ondansetron, were demonstrated to be type II signals in pediatrics. The other drug–DIKI associations have been confirmed in previous studies.
Medications are the relatively common cause of AKI, especially in pediatrics. DIKI is related to a drugs’ inherent toxicity and how the kidneys are able to handle it. It poses several challenges for clinicians mainly because of the difficulties in timely identification of drugs with potential nephrotoxicity (Starr et al., 2021). In this context, research on RWD is growing for its implication in the surveillance of drug safety. The EHR, as one of the routine RWDs, is a good data source for pharmacovigilance because of its detailed information on clinical events related to medications (Patadia et al., 2015). This study established and applied a two-stage method for detecting DIKI signals in children using an EHR database: first to identify potential suspected drugs and then to conduct a retrospective cohort to analyze the correlation between the specific suspected drugs and renal impairment. We initially discovered nine candidate drugs related to DIKI, and six positive signals for DIKI were identified in further analyses. To the best of our knowledge, one of the drug-DIKI associations (creatine phosphate sodium) has not been previously described in current literature evidence, either in adults or in children. Three other drug-DIKI associations (diazepam, omeprazole, and ondansetron) were not previously reported in children but have already been reported in adults. These drugs may become the critical target drugs for active surveillance and causality assessment in further research.
The association of creatine phosphate sodium with DIKI was found to be a possible new signal in this study. Creatine phosphate sodium is one of the cardioprotective drugs and is widely used in the protection of abnormal myocardial metabolism during myocardial ischemia. A previous study showed that 14.40% cases (28/200) of children were treated with sodium creatine phosphate as prophylactic treatment without a diagnosis, suggesting that there was an overdosage of sodium creatine phosphate in pediatrics (Jin et al., 2022). Another study showed that high doses of creatine phosphate sodium injection may cause large amounts of phosphate intake, which may affect renal function through calcium and purine metabolism disorders, as well as unstable hormone secretion (Yan et al., 2011). Recently, the National Medical Products Administration (NMPA) in China has recommended that serum calcium, serum phosphorus, and renal function should be monitored during the use of creatine phosphate sodium in neonates and premature infants, which also provides a reference for children of other ages. Although creatine phosphate sodium has been on the market for many years, the mechanisms underlying the association between its use and the deterioration of kidney function are still unclear. In light of these findings, further studies should assess the mechanism of kidney injury induced by creatine phosphate sodium in children. For patients with DIKI, suspected drugs were discontinued immediately, followed by symptomatic treatment to preserve the kidney. At the same time, clinicians should also pay attention to regular renal function in children who need long-term and high-dose use of creatine phosphate sodium to better promote the rational use of this drug.
Three other drug-DIKI associations (diazepam, omeprazole, and ondansetron) were identified as potentially new signals in the pediatric population. Diazepam is a common sedative–hypnotic drug in clinical settings, and the ADRs described in SPC include drowsiness, fatigue, muscle weakness, urinary retention, or incontinence. A nationwide population-based retrospective cohort study showed that diazepam had a significant correlation with increased chronic kidney disease risk in the population aged >18 years old (adjusted hazard ratio (HR) = 1.627, 95 CI%:1.527–1.736) (Liao et al., 2020). One of the possible mechanisms is that propylene glycol is contained in parenteral formulations of diazepam, which may cause AKI and proximal tubule injury (Cawley, 2001). In addition, omeprazole is a proton pump inhibitor (PPI) that selectively inhibits the H + -K + -ATPase in the gastric parietal cell membrane. It is widely used in the treatment of peptic ulcers, reflux esophagitis, and Zollinger–Ehrlich syndrome in pediatrics (Kato et al., 2021). Serious ADRs such as acute interstitial nephritis (AIN) and renal failure have also been reported in adults (Nadri and Althaf, 2014). Simpson et al. (2006) reported that the incidence of AIN caused by omeprazole was 8/100,000 (95% Cl: 2.6/100,000 to 18.7/100,000) (Simpson et al., 2006). Other studies have shown that omeprazole can cause a high incidence of renal damage in the elderly (Klatte et al., 2017). The underlying mechanism was drug-induced immune damage, including acute and chronic interstitial nephritis and tubulointerstitial nephritis (Torlot and Whitehead, 2016). Furthermore, ondansetron is a preferred anti-emetic in critical care to treat nausea and vomiting. A recent pharmacoepidemiology study reported that ondansetron may be associated with an increased risk of AKI (Gray et al., 2022). Another work showed that ondansetron can enhance cisplatin-induced nephrotoxicity via inhibition of multiple toxins and extrusion proteins (Li et al., 2013). However, the associations between the aforementioned three drugs and renal impairment were mainly reported in adults. We should also remember that most of the drugs currently used in pediatric clinical practices were originally developed for adults, and for the majority of them, the mechanism of renal toxicity in children is still to be clarified. Our results may provide more clues and need to be validated in a larger pediatric population.
This study also found that both methotrexate (MTX) and cytarabine were associated with a degree of kidney injury, which was consistent with previous reports in the literature. They are both common antineoplastic agents and are widely used in acute lymphoblastic leukemia (ALL) and non-Hodgkin’s lymphoma in pediatrics. A retrospective analysis found that the first high-dose methotrexate (HDMTX) course (OR = 1.767) and methotrexate dose per body surface area (OR = 1.944) significantly correlated with AKI in 336 ALL children (Cheng et al., 2018). HDMTX-associated AKI with delayed MTX clearance has been linked to an excess in MTX-induced toxicities (Heuschkel et al., 2022). AKI develops due to the precipitation of MTX and its metabolites within the tubular lumens of the kidney (Perazella and Izzedine, 2015). Furthermore, MTX has been shown to induce the formation of oxygen radicals with subsequent cellular injury, associated with decreased adenosine deaminase activity (Pinheiro et al., 2010). In addition, a case report showed that low-dose cytarabine could induce hepatic and renal dysfunction in a patient with myelodysplastic syndrome, indicating that careful observation should be carried out in clinical practice (Tanaka et al., 1999). As an anti-tumor drug with weak cell specificity, cytarabine affects all actively growing cells by inhibiting the synthesis of DNA, including renal tubular epithelial cells, thereby causing nephrotoxicity (Pannu and Nadim, 2008). The aforementioned results support the reliability of our two-stage method in DIKI signal detection.
The main strength of this study is its capability to retrospectively observe a large number of children and adolescents in a “real-world” setting by combining data from longitudinal EHRs. This two-stage designed approach has certain advantages in comparison with other methods based on abnormal values of laboratory tests (Yoon et al., 2012) or GTT. In the first stage, we roughly assessed the potential to select the suspected drugs, increasing the efficiency of subsequent analysis. Furthermore, more confounders, such as kidney disease and medications that may affect the level of laboratory indicators, were considered. In addition, the algorithm execution does not require manual review and can be developed into an automated program in the future, which is more suitable for the detection of ADEs based on large-scale databases. The final results suggest that our method can be used as a useful tool to detect DIKI signals for use by clinicians and regulatory agencies.
Despite the strengths of EHRs, they have inherent limitations, including incomplete case capture, selection bias, unmeasured confounding, and the inability to infer causality. Thus, some limitations to this study should be considered. First, only the records that met the criteria were included in the study. The associations between suspected drugs and DIKI remain unknown in those excluded records. Second, while our method has controlled four important variables, it does not adjust drug–drug interactions, drug–dose response, or disease severity covariates in the model. Third, the EHR data from a single-site system was used in this study. More efforts are needed to validate the feasibility of the approach and the DIKI signals in other EHR databases in the future. Lastly, it is important to realize that this method is a tool to assist with signal detection but does not ensure causality assessment of ADRs. All potentially new signals require further evaluation in hypothesis-testing studies to better account for bias and confounding.
In summary, this study shows that the RWDs, especially the EHRs, are potentially valuable resources for post-marketing drug safety surveillance. Our findings provide six candidate drug-DIKI associations using medical record databases. Future research is warranted to assess the causality of DIKI signals and formulate strategies for drug risk management in pediatrics. Nowadays, China has initiated the project “China ADR Sentinel Surveillance Alliance” (CASSA) with at least 300 medical facilities. Also of note, BCH was the first pediatric hospital in CASSA. More attention will be paid to integrating the method based on the CASSA platform to explore multi-center pharmacovigilance research.
DIKI is one of the most common problems in clinical practice, especially in hospitalized patients. In this work, we proposed a quantitative two-stage procedure to explore potential DIKI signals using RWD. Six positive signals of DIKI, including four new signals in children, were detected. Our findings highlight the potential of EHRs to complement traditional SRS for drug safety signal detection and strengthening in a pediatric setting. It is hoped that current efforts to identify DIKI signals will allow us to modify practice and reduce unnecessary harm in the future.
The raw data supporting the conclusion of this article will be made available by the authors, without undue reservation.
The protocol of the study was approved by the Institutional Review Board (IRB) of Beijing Children’s Hospital, Capital Medical University (approval number: 2018-129), with a waiver of informed consent.
XW and XP undertook work of framework design and overall guidance of the whole research. YY and XN took responsibility for the data collection. YY, YZ, and YX performed data processing and statistical analysis. YY and WC were responsible for the manuscript writing and data interpretation. XP provided important methodological advice.
This work was supported by the Clinical Toxicology Program of the Chinese Society of Toxicology (CST2020CT108), the Children's Medication Project of Beijing Children's Hospital (2020) (YZYB202006), the National Natural Science Foundation of China (Nos. 72174128, 82102688 and 82204149), the Hainan Free Trade Port Boao Hope City Real World Study Project (HNLC2022RWS015), and the Clinical Technology Training Program for Comprehensive Evaluation of Pediatric Medication (CNHDRC-KJ-W-2021-58).
The authors declare that the research was conducted in the absence of any commercial or financial relationships that could be construed as a potential conflict of interest.
All claims expressed in this article are solely those of the authors and do not necessarily represent those of their affiliated organizations, or those of the publisher, the editors, and the reviewers. Any product that may be evaluated in this article, or claim that may be made by its manufacturer, is not guaranteed or endorsed by the publisher.
The Supplementary Material for this article can be found online at: https://www.frontiersin.org/articles/10.3389/fphar.2022.957980/full#supplementary-material.
Cawley, M. J. (2001). Short-term lorazepam infusion and concern for propylene glycol toxicity: Case report and review. Pharmacotherapy 21 (9), 1140–1144. doi:10.1592/phco.21.13.1140.34611
Chen, C., Jia, W., Guo, D., Zhu, M., Xu, Y., Wang, X., et al. (2020). Development of a computer-assisted adverse drug events alarm and assessment system for hospital inpatients in China. Ther. Innov. Regul. Sci. 54 (1), 32–41. doi:10.1007/s43441-019-00027-z
Cheng, D-H., Lu, H., Liu, T-T., Zou, X-Q., and Pang, H-M. (2018). Identification of risk factors in high-dose methotrexate-induced acute kidney injury in childhood acute lymphoblastic leukemia. Chemotherapy 63 (2), 101–107. doi:10.1159/000486823
de Bie, S., Coloma, P. M., Ferrajolo, C., Verhamme, K. M., Trifiro, G., Schuemie, M. J., et al. (2015). The role of electronic healthcare record databases in paediatric drug safety surveillance: A retrospective cohort study. Br. J. Clin. Pharmacol. 80 (2), 304–314. doi:10.1111/bcp.12610
Faught, L. N., Greff, M. J., Rieder, M. J., and Koren, G. (2015). Drug-induced acute kidney injury in children. Br. J. Clin. Pharmacol. 80 (4), 901–909. doi:10.1111/bcp.12554
Faught, L. N., Greff, M. J. E., Rieder, M. J., and Koren, G. (2015). Drug-induced acute kidney injury in children. Br. J. Clin. Pharmacol. 80 (4), 901–909. doi:10.1111/bcp.12554
Garrett, P. R., Sammer, C., Nelson, A., Paisley, K. A., Jones, C., Shapiro, E., et al. (2013). Developing and implementing a standardized process for global trigger tool application across a large health system. Jt. Comm. J. Qual. Patient Saf. 39 (7), 292–297. doi:10.1016/s1553-7250(13)39041-2
Gray, M., Priyanka, P., Kane-Gill, S., Wang, L., and Kellum, J. A. (2022). Kidney and mortality Outcomes associated with ondansetron in critically ill patients. J. Intensive Care Med., 088506662110735. doi:10.1177/08850666211073582
Hanna, M. H., Askenazi, D. J., and Selewski, D. T. (2016). Drug-induced acute kidney injury in neonates. Curr. Opin. Pediatr. 28 (2), 180–187. doi:10.1097/MOP.0000000000000311
Heuschkel, S., Kretschmann, T., Teipel, R., Bonin, S., Richter, S., Quick, S., et al. (2022). Half-dose glucarpidase as efficient rescue for toxic methotrexate levels in patients with acute kidney injury. Cancer Chemother. Pharmacol. 89 (1), 41–48. doi:10.1007/s00280-021-04361-8
Hosohata, K. (2016). Role of oxidative stress in drug-induced kidney injury. Int. J. Mol. Sci. 17 (11), E1826. doi:10.3390/ijms17111826
Hoste, L., Dubourg, L., Selistre, L., De Souza, V. C., Ranchin, B., Hadj-Aissa, A., et al. (2014). A new equation to estimate the glomerular filtration rate in children, adolescents and young adults. Nephrol. Dial. Transpl. 29 (5), 1082–1091. doi:10.1093/ndt/gft277
Jin, X., Xinghui, Z., Jiong, L., and Qingtao, W. (2022). Establishment and application of DUE criteria for creatine phosphate sodium in a Children’s hospital. China Pharm. 23 (5), 923–925.
Kato, K., Mizuno, T., Koseki, T., Ito, Y., Hatano, M., Takahashi, K., et al. (2021). Concomitant proton pump inhibitors and immune checkpoint inhibitors increase nephritis frequency. Vivo 35 (5), 2831–2840. doi:10.21873/invivo.12570
Klatte, D. C. F., Gasparini, A., Xu, H., Deco, P., Trevisan, M., Johansson, A. L. V., et al. (2017). Association between proton pump inhibitor use and risk of progression of chronic kidney disease. Gastroenterology 153 (3), 702–710. doi:10.1053/j.gastro.2017.05.046
Li, Q., Guo, D., Dong, Z., Zhang, W., Zhang, L., Huang, S-M., et al. (20132013). Ondansetron can enhance cisplatin-induced nephrotoxicity via inhibition of multiple toxin and extrusion proteins (MATEs). Toxicol. Appl. Pharmacol. 273 (1), 100–109. doi:10.1016/j.taap.2013.08.024
Liao, C. Y., Chung, C. H., Lu, K. C., Cheng, C. Y., Yang, S. S., Chien, W. C., et al. (2020). Taking sleeping pills and the risk of chronic kidney disease: A nationwide population-based retrospective cohort study. Front. Pharmacol. 11, 524113. doi:10.3389/fphar.2020.524113
Nadri, Q., and Althaf, M. M. (2014). Granulomatous tubulointerstitial nephritis secondary to omeprazole. BMJ Case Rep., bcr2014203842. doi:10.1136/bcr-2014-203842
Ostermann, M., Bellomo, R., Burdmann, E. A., Doi, K., Endre, Z. H., Goldstein, S. L., et al. (2020). Controversies in acute kidney injury: Conclusions from a kidney disease: Improving global Outcomes (KDIGO) conference. Kidney Int. 98 (2), 294–309. doi:10.1016/j.kint.2020.04.020
Pannu, N., and Nadim, M. K. (2008). An overview of drug-induced acute kidney injury. Crit. Care Med. 36, S216–S223. doi:10.1097/CCM.0b013e318168e375
Patadia, V. K., Schuemie, M. J., Coloma, P., Herings, R., van der Lei, J., Straus, S., et al. (2015). Evaluating performance of electronic healthcare records and spontaneous reporting data in drug safety signal detection. Int. J. Clin. Pharm. 37 (1), 94–104. doi:10.1007/s11096-014-0044-5
Perazella, M. A., and Izzedine, H. (2015). New drug toxicities in the onco-nephrology world. Kidney Int. 87 (5), 909–917. doi:10.1038/ki.2015.30
Pinheiro, F. V., Pimentel, V. C., Bona, K. S. D., Scola, G., Salvador, M., Funchal, C., et al. (2010). Decrease of adenosine deaminase activity and increase of the lipid peroxidation after acute methotrexate treatment in young rats: Protective effects of grape seed extract. Cell. biochem. Funct. 28 (1), 89–94. doi:10.1002/cbf.1627
Pitts, P. J., and Le Louet, H. (2018). Advancing drug safety through prospective pharmacovigilance. Ther. Innov. Regul. Sci. 52 (4), 400–402. doi:10.1177/2168479018766887
Radi, Z. A. (2019). Kidney pathophysiology, Toxicology, and drug-induced injury in drug development. Int. J. Toxicol. 38 (3), 215–227. doi:10.1177/1091581819831701
Simpson, I. J., Marshall, M. R., Pilmore, H., Manley, P., Williams, L., Thein, H., et al. (2006). Proton pump inhibitors and acute interstitial nephritis: Report and analysis of 15 cases. Nephrology 11 (5), 381–385. doi:10.1111/j.1440-1797.2006.00651.x
Starr, M. C., Charlton, J. R., Guillet, R., Reidy, K., Tipple, T. E., Jetton, J. G., et al. (2021). Advances in neonatal acute kidney injury. Pediatrics 148 (5), e2021051220. doi:10.1542/peds.2021-051220
Tanaka, M., Kanamori, H., Yamaji, S., Mishima, A., Fujita, H., Fujisawa, S., et al. (1999). Low-dose cytarabine-induced hepatic and renal dysfunction in a patient with myelodysplastic syndrome. Anticancer. Drugs 10 (3), 289–291. doi:10.1097/00001813-199903000-00006
Toh, S., Hampp, C., Reichman, M. E., Graham, D. J., Balakrishnan, S., Pucino, F., et al. (2016). Risk for hospitalized heart failure among new users of saxagliptin, sitagliptin, and other antihyperglycemic drugs: A retrospective cohort study. Ann. Intern. Med. 164 (11), 705–714. doi:10.7326/M15-2568
Torlot, F. J., and Whitehead, D. J. (2016). Acute interstitial nephritis caused by two different proton pump inhibitors. Br. J. Hosp. Med. 77 (1), 50–51. doi:10.12968/hmed.2016.77.1.50
Yan, H., Jichun, Z., and Xiaoyue, X. (2011). Evaluation on the safety of creatine phosphate sodium for injection. Chin. J. Hosp. Pharm. 31 (22), 1871–1874.
Yang, L., Xing, G., Wang, L., Wu, Y., Li, S., Xu, G., et al. (2015). Acute kidney injury in China: A cross-sectional survey. Lancet 386 (10002), 1465–1471. doi:10.1016/S0140-6736(15)00344-X
Yoon, D., Park, M. Y., Choi, N. K., Park, B. J., Kim, J. H., and Park, R. W. (2012). Detection of adverse drug reaction signals using an electronic health records database: Comparison of the Laboratory Extreme Abnormality Ratio (CLEAR) algorithm. Clin. Pharmacol. Ther. 91 (3), 467–474. doi:10.1038/clpt.2011.248
Yu, Y., Nie, X., Song, Z., Xie, Y., Zhang, X., Du, Z., et al. (2020). Signal detection of potentially drug-induced liver injury in children using electronic health records. Front. Pediatr. 8, 171. doi:10.3389/fped.2020.00171
Keywords: drug-induced kidney injury, children, active monitoring, electronic health records, signal detection
Citation: Yu Y, Nie X, Zhao Y, Cao W, Xie Y, Peng X and Wang X (2022) Detection of pediatric drug-induced kidney injury signals using a hospital electronic medical record database. Front. Pharmacol. 13:957980. doi: 10.3389/fphar.2022.957980
Received: 31 May 2022; Accepted: 15 August 2022;
Published: 23 September 2022.
Edited by:
Catherine M. T. Sherwin, Wright State University, United StatesReviewed by:
Dmitriy Sychev, Ministry of Health, RussiaCopyright © 2022 Yu, Nie, Zhao, Cao, Xie, Peng and Wang. This is an open-access article distributed under the terms of the Creative Commons Attribution License (CC BY). The use, distribution or reproduction in other forums is permitted, provided the original author(s) and the copyright owner(s) are credited and that the original publication in this journal is cited, in accordance with accepted academic practice. No use, distribution or reproduction is permitted which does not comply with these terms.
*Correspondence: Xiaoling Wang, d2FuZ3hpYW9saW5nQGJjaC5jb20uY24=; Xiaoxia Peng, cGVuZ3hpYW94aWFAYmNoLmNvbS5jbg==
†These authors have contributed equally to this work and share senior authorship
Disclaimer: All claims expressed in this article are solely those of the authors and do not necessarily represent those of their affiliated organizations, or those of the publisher, the editors and the reviewers. Any product that may be evaluated in this article or claim that may be made by its manufacturer is not guaranteed or endorsed by the publisher.
Research integrity at Frontiers
Learn more about the work of our research integrity team to safeguard the quality of each article we publish.