- 1Institute of Biosciences, Federal University of the State of Rio de Janeiro, Rio de Janeiro, Brazil
- 2Observatório de Vigilância e Uso de Medicamentos, Faculty of Pharmacy, Federal University of Rio de Janeiro, Rio de Janeiro, Brazil
- 3Laboratório de Pesquisa em Infecção Hospitalar, Instituto Oswaldo Cruz, Fiocruz, Rio de Janeiro, Brazil
- 4Scientific Computing Program, Oswaldo Cruz Foundation, Rio de Janeiro, Brazil
Antibacterial drugs are a widely used drug class due to the frequency of infectious diseases globally. Risks knowledge should ground these medicines’ selection. Data mining in large databases is essential to identify early safety signals and to support pharmacovigilance systems. We conducted a cross-sectional study to assess adverse drug events related to antibiotics reporting between December 2018 and December 2021 in the Brazilian database (Vigimed/VigiFlow). We used the Reporting Odds Ratio (ROR) disproportionality analysis method to identify disproportionate reporting signals (SDR), referring to statistical combinations between drugs and adverse events. Vancomycin was the most reported antibiotic (n = 1,733), followed by ceftriaxone (n = 1,277) and piperacillin and tazobactam (n = 1,024). We detected 294 safety signals related to antibacterials. We identified azithromycin leading in the number of safety signals (n = 49), followed by polymyxin B (n = 25). Of these, 95 were not provided for in the drug label and had little or no reports in the medical literature. Three serious events are associated with ceftazidime and avibactam, a new drug in the Brazilian market. We also found suicide attempts as a sign associated with amoxicillin/clavulanate. Gait disturbance, a worrying event, especially in the elderly, was associated with azithromycin. Our findings may help guide further pharmacoepidemiologic studies and monitoring safety signals in pharmacovigilance.
Introduction
Individual case safety reports (ICSRs) from the spontaneous reporting system provide an essential information source for studying adverse drug events (ADEs) (Li et al., 2022). The database screening may be used to discover unknown drug-event pairs associated with signals of disproportionate reporting (Dijkstra et al., 2020). The evaluation of a signal encompasses quantitative and qualitative aspects (Meyboom et al., 1997; Hauben and Aronson, 2009). Meyboom and collaborators define a signal is more than just a statistical association, it consists of a hypothesis based on data and arguments (Meyboom et al., 1997) According to the World Health Organization (WHO), a safety signal is an information on a new or known ADE that may be caused by a medicine and is typically generated from more than a single report of a suspected event. A signal does not imply a direct causal relationship between an event and a drug but added with more information (consistent data, biological plausibility, association strength, for example) raises a hypothesis that requires further assessment (World Health Organization, 2022a). Regarding the quantitative aspect, statistical techniques (cluster analysis, link analysis, deviation detection, and disproportionality assessment) can be used to determine and assess the strength of ADE signals by the disproportional analysis (Montastruc et al., 2011).
Several measures of disproportionality reporting are available, and some studies propose direct comparisons of methods using synthetic, noise-free data (Zorych et al., 2013; Dijkstra et al., 2020). The reporting odds ratio (ROR) is the disproportionality method used by Eudravigilance e UMC Uppsala (World Health Organization, 2022a; European Medicines Agency, 2022) and performs well in most cases.
Low and middle-income countries (LMIC) face challenges in establishing efficient pharmacovigilance systems capable of generating data to inform health policies and practices (Kiguba et al., 2021). In Brazil, the largest country in South America, a new ADE notification system was deployed in 2018 (Vogler et al., 2020) by the National Health Surveillance Agency (ANVISA). Vigimed was the name given in Brazil to Vigiflow, a web-based ICSR management system developed by UMC, which replaced Notivisa due to system instability, an inability to import data series or export the database for analysis, and a lack of analytical and statistical tools (Vogler et al., 2020).
Data mining in pharmacovigilance databases may guide 1) additional analytical studies in specific populations that may be more susceptible to ADE occurrence and 2) epidemiologic studies for risk quantification and risk-minimization activities (Emanuel Raschi, 2016). Previous studies from our group found relevant signals of disproportionate reporting in pediatric and oncologic care from the former Brazilian database (Notivisa) (Barcelos et al., 2019; Vieira et al., 2020).
Antibacterials are a strategic drug class for pharmacoepidemiologic studies due to the frequency and their use consequences. These medicines have been associated with ADEs, microbial resistance, morbidity, and mortality worldwide (Tamma et al., 2017; Montastruc et al., 2021; Li et al., 2022). In addition, cultural factors can influence antibiotic use (Touboul-Lundgren et al., 2015). Brazil is one of the countries with the highest consumption of these drugs (World Health Organization, 2018) which raised on COVID-19 disease pandemic (Silva et al., 2021; Ul Mustafa et al., 2021).
We aimed to identify and analyze potential safety signals related to antibacterial agents for systemic use from the Brazilian electronic system for the spontaneous report (Vigimed/VigiFlow) from 2018 to 2021. This study is the first to analyze signals of disproportionate reporting involving antibacterials based on data obtained from the new Brazilian electronic reporting system.
Materials and methods
We conducted a registry-based cross-sectional study (European Medicines Agency, 2021). We collected data from the ADE spontaneous reports Brazilian database (Vigimed/Vigiflow) between January and March 2022. The Vigimed platform is currently available in the ANVISA database (Agência Nacional de Vigilância Sanitária, 2022c). We included all reports of antibacterials for systemic according to Anatomical Therapeutic Chemical Classification System - ATC J01) related to 1 December 2018, and 31 December 2021 period (World Health Organization, 2020).
Antibacterials were classified by the fifth level of the Anatomical Therapeutic Chemical (ATC) Code (World Health Organization, 2020). We also considered AWaRe Classification to compare ADE reports by antibiotics with different levels (three groups) of microbial resistance (Habarugira et al., 2021). The Access group includes antibiotics with a lower potential for resistance than the other groups. They are first or second-choice empirical treatments for infectious syndromes. Watch group antibiotics should be prioritized as critical targets of administration and monitoring programs. The Watch group has 11 antibiotics in the WHO Model List of Essential Medicines. Antibiotics in the Reserve group should be treated as a “last resort”. These drugs are vital points of antimicrobial stewardship programs. Seven antibiotics from the reserve group make up the WHO Model List of Essential Medicines (World Health Organization, 2019, 2021).
We compared case/non-case where cases were ICSR with ADE. We created a database containing 1) drug names, 2) reported ADE, and 3) the number of notifications of each ADE for each target drug. According to the European Medicines Agency, we did not consider drug-event pairs with less than three notifications and notifications dealing with medication errors (Aronson, 2009; European Medicines Agency, 2018b).
Concerning statistical analyses, we used the Reporting Odds Ratio (ROR) disproportionality analysis method, as implemented by the EudraVigilance Data Analysis System (EVDAS) used by the European Medicines Agency (European Medicines Agency, 2018a). We used the ROR measure to identify disproportionate reporting signals (SDR), referring to statistical combinations between drugs and ADEs. This method assumes that when a signal (involving a specific ADE) is associated with a drug, it indicates that the ADEs reported more frequently in association with this drug than other drugs (European Medicines Agency, 2006). The ROR calculation considers its 95% confidence interval and the number of individual cases (Van Puijenbroek et al., 2002).
The ROR measure is defined by the formula [(a.d)/(c.b)], where:
• “a” indicates the number of reports that list the target drug P and the target ADE R;
• “b” indicates the number of reports that list the target drug P but not the target ADE R;
• “c” indicates the number of reports that list the target ADE R but not the drug P;
• Finally, “d” indicates the number of reports that do not list the target ADE R or drug P.
When the inferior limit of the ROR’s 95% confidence interval is greater than 1, the association between ADE and the target drug is considered statistically significant (Rothman et al., 2004). Therefore, we consider it an SDR. A situation occurs when c = 0 or all database reports containing a target ADE are associated with only one drug. In this case, there is a division by zero, and it is not possible to calculate the ROR. In this situation, the ROR value is arbitrarily set at 99.9 to reflect the presence of a possible SDR. We also differentiate in the following analysis the cases where 3 ≤ a <5 and a ≥5.
We assess previous information about safety signals found in the drug labels (summary of product characteristics - SPC) available on the ANVISA website (Agência Nacional de Vigilância Sanitária, 2022b). All safety signals not mentioned in SPCs were tabulated. Additionally, we investigated the existence of any complementary data that could reinforce the suspicion of a causal relationship between the antibiotic and the observed event in studies published in the following databases: UpToDate, Pubmed, Embase, Web of Science, and Medline. The search was performed using the drug name according to ATC and Preferred Terms (PT) (International Council for Harmonisation of Technical Requirements for Pharmaceuticals for Human Use, 2020).
Results
We found 12,665 ADE reports involving 53 antibacterials for systemic use (Group J01) between 1 December 2018, and 31 December 2021, in the Vigimed/VigiFlow database (Brazil). The most reported antibiotics were: vancomycin (n = 1,733), ceftriaxone (n = 1,274), piperacillin/tazobactam (n = 1,024), ciprofloxacin (n = 936), and azithromycin (n = 870) (Figure 1).
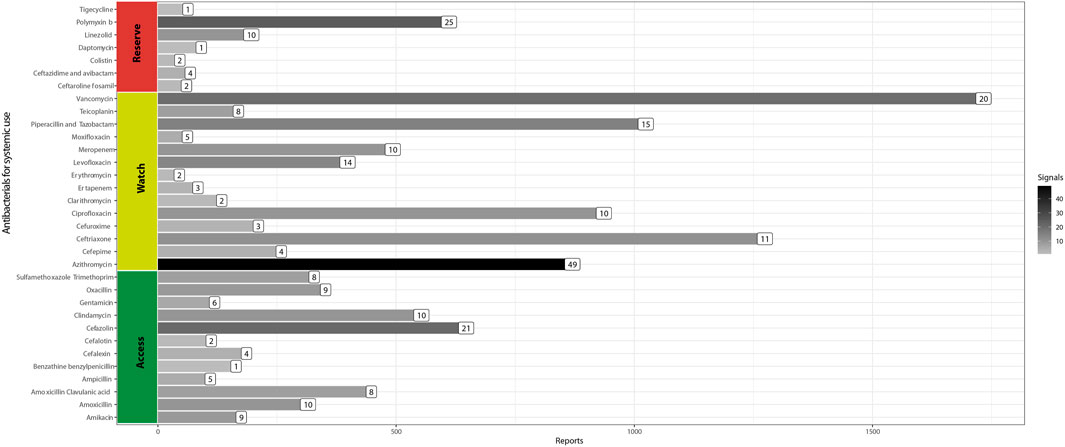
FIGURE 1. Adverse drug event reports and safety signals involving systemic antibacterials (Brazil, 2018–2021).
We obtained 294 safety signals. Azithromycin (n = 49) and polymyxin B (n = 25) were the main antibiotics involved in these signals, as shown by the bar color density in Figure 1. For 67.7% of pairs, the event reported was already described in the label of the respective antibiotic. Table 1 presents the 95 signals, few or not reported in the literature.
Concerning serious safety signals, we found eight involving antibiotics classified as Reserve. Polymyxin B had five serious ADE (depressed level of consciousness, dyspnea, acute respiratory failure, oxygen saturation decreased, and cardio-respiratory arrest) not previously described. Death, depression level of consciousness, and sepsis with ceftazidime/avibactam, an antibiotic recently introduced in the Brazilian market, were also identified in our study (Table 1).
In watch antibiotics, gait disturbance, a worrying event, especially in the elderly, was associated with azithromycin. Suicide attempts with amoxicillin also is a serious and unprecedented potential safety signal. Ineffectiveness, described in other countries, was obtained for azithromycin, cefuroxime, amoxicillin, and amoxicillin/clavulanate (Table 1).
In the access class, we highlight hyperthermia as an event previously described for oxacillin (Table 1).
Discussion
This first study involving the new Brazilian electronic notification system (Vigimed/VigiFlow) found 294 safety signals associated with disproportionate reporting for antibacterials of the three AWaRe classes. Some signals.
Ceftazidime/avibactam is a new antibiotic that began to be marketed in Brazil in 2018 and that seemed to be well tolerated (Cheng et al., 2020). This drug is restricted to infections with no other therapeutic option and is expected to have a lower frequency of use than other agents (Watch and Access) (World Health Organization, 2019). A study that analyzed adverse events from two phases II and III clinical trials did not report any serious adverse events (Cheng et al., 2020). In the report issued by the EMA in 2021, ceftazidime/avibactam was not related to any safety signal (European Medicines Agency, 2018c). However, we found three potentially serious signals related to ceftazidime-avibactam (Table 1). Other pharmacovigilance databases also received reports of the same ADE for this same drug. Eudravigilance and Vigiaccess contain eight and 19 reports of sepsis associated with the same drug, respectively (European Medicines Agency, 2018c; World Health Organization, 2022b). Five reports for the depressed level of consciousness and 82 for death were described in VigiAccess (World Health Organization, 2022b). Ceftazidime/avibactam was the suspicion drug for 123 deaths in the FDA Adverse Event Reporting System (FAERS) (Food and Drug Administration, 2021). Nevertheless, data that support the causality for these ADEs are not yet available.
We found many signals of azithromycin associated with infections and immune system disorders. The possible causal relationship between azithromycin and the reported infections has biological plausibility. The pathophysiological mechanism for this adverse effect refers to local microbiota modification by the azithromycin, eliminating bacteria commensals and allowing the growth and proliferation of fungi and opportunistic microorganisms (Seelig, 1966). For example, there is a risk of candidiasis caused by azithromycin (Wilton et al., 2003). Similarly, microbiological imbalance generated by the previous use of antibiotics might cause broad immunity and metabolism changes, leading to the recurrence of infections, such as pneumonia (Francino, 2015). Withal gait disorders contribute to reduced mobility, fall risk, diminished quality of life, and serious injuries, including significant fractures and head trauma (Pirker and Katzenschlager, 2017). Eventually, drugs cause gait disturbance (Kanao-Kanda et al., 2016), but we did not find gait disturbances as an adverse event associated with azithromycin in the medical literature. Nevertheless, a study reported akathisia (ADE typically attributed to antipsychotics) after exposure to azithromycin (Riesselman and El-Mallakh, 2015). Accumulating azithromycin in brain tissue can prove unpredictable effects (Jaruratanasirikul et al., 1996), a possible mechanism by which azithromycin causes gait disturbance. The pandemic caused by COVID-19 increased azithromycin consumption in Brazil (Del Fiol et al., 2022). This scenario may have contributed to the emergence of safety signals not previously observed (Meyboom et al., 1997) (Figure 1).
Our study showed ineffectiveness signals for two antibacterials classified as Watch (cefuroxime and azithromycin) and amoxicillin, azithromycin, and amoxicillin/clavulanate. The preferred terms “ineffective drug,” “therapeutic product effects decreased,” and “pathogen resistance” are triggers for monitoring microbial resistance in pharmacovigilance databases (Habarugira et al., 2021). We expect the investigation of these specific signals in observational studies in the Brazilian population.
We found five previously unreported signals associated with polymyxin B. Depressed level of consciousness and cardiorespiratory arrest may be secondary to hyponatremia, an adverse event associated with polymyxin B previously (Rodriguez et al., 1970). Drug interactions of polymyxin B with other drugs might cause respiratory symptoms, such as neuromuscular blockers and aminoglycosides (Pohlmann, 1966). Furthermore, in 1964, a report on respiratory arrest associated with polymyxin B infusion was published (Small, 1964). To our knowledge, there have been no other similar reports.
We described suicide attempts and amoxicillin as a signal in our analysis. We found no previous report of amoxicillin-related suicidal behavior in the medical literature. However, in the FAERS database, suicide attempt events associated with amoxicillin also were reported (n = 73) (Food and Drug Administration, 2021). Suicidal behavior is heterogeneous and multifactorial, influenced by complex interactions between biological, psychological, and social factors. Some antibiotics cause suicidal ideation by daedal neuropharmacological interactions involving the GABAergic receptor (Samyde et al., 2016). So, the existence of causal relations cannot be ruled out. In the same way, we did not find reports of the pair chest pain or asthenia with amoxicillin and clavulanate, but for this second ADE, there are 1111 reports of suspicion with this drug in the VigiAccess (World Health Organization, 2022b). Despite being an antimicrobial that has been on the market for a long time and is widely used in outpatient settings, the safety signals should be monitored due to its seriousness and the demographic, genetic, and nutritional patterns in different populations (World Health Organization, 2012).
Fluoroquinolones are antibiotics with numerous adverse effects well established by regulatory agencies (Samyde et al., 2016; Agência Nacional de Vigilância Sanitária, 2019; Food and Drug Administration, 2019). Notwithstanding, some ADE might be underestimated. According to levofloxacin labels, the incidence of anxiety associated with the drug occurs in less than 1%, and there is no report of loss of consciousness as a primary event associated with levofloxacin. However, levofloxacin may trigger important neurologic events (Mazzei et al., 2012; Scavone et al., 2020), resulting in loss of consciousness.
We described several signals without any mention in literature for some antibiotics (ceftaroline fosamil, meropenem, ceftriaxone, piperacillin and tazobactam, teicoplanin, tobramycin, moxifloxacin, and cefazolin) in Table 1. Such signals have less relevance for monitoring given their lower severity and lack of information to strengthen the hypothesis of a causal relationship. Despite everything, knowledge about pharmacovigilance is dynamic, and new data may be added in the future.
ANVISA’s Pharmacovigilance Management - Gerência de Farmacovigilância (GFARM)- evaluates notifications received at Vigimed/Vigiflow and forwards them to the WHO Uppsala Center. If the Uppsala Center emits any signal, GFARM initiates an investigation process. If the investigation confirms the causality between the event and drug exposure, GFARM alerts health professionals and drug users and establishes complementary regulatory measures (e.g., drug label change) (Agência Nacional de Vigilância Sanitária, 2022a).
We performed an exploratory analysis of crude data from the Vigimed/Vigiflow database to detect safety signals of antibacterials in a marked strategic period by initial reports in this new Brazilian database and increase of this class of drugs consumption for this population (Sartelli et al., 2020). Disproportionality analysis in pharmacovigilance databases is an effective method for the early detection of these signals, especially for rare ADEs (European Medicines Agency, 2022). Nevertheless, this tool has inherent limitations in data mining studies from spontaneous reporting systems. Some ADE may be underreported, and the absence of a clinical history prevents the analysis of the causality of the reported ADE. We use the Reporting Odds Ratio measure, an example of a frequentist approach for Disproportionality Analysis in pharmacovigilance. It is generally accepted that neither bayesian nor frequentist methods are better than the other (Harpaz et al., 2012; Huang et al., 2014). Frequentist approaches are more computationally efficient than Bayesian measures but may generate more false positives. The Bayesian methods also incorporate information about disproportionality and sample size in a single dimension. Nevertheless, none of the approaches can effectively address reporting biases or confounding in spontaneous reporting systems (Harpaz et al., 2012).
Besides that, every disproportionality measure might require a new reinvestigation of data, including pharmacodynamic analysis of biological plausibility (Montastruc et al., 2011). All in all, signal management consists of several pharmacovigilance processes: 1) signal detection, 2) prioritization, 3) validation, 4) evaluation, and 5) outcome documentation (European Medicine Agency, 2018). Although this analysis may have shown serious undetectable signals in other countries, our findings are limited to Brazil.Boletim de Farmacovigilância No13, 2020.
Conclusion
Antibiotics were widely used during the COVID-19 pandemic, resulting in a suitable scenario to observe unreported safety signals. Our study detected 95 new safety signals. Three serious signals are associated with ceftazidime/avibactam, a drug recently introduced in the Brazilian market. Suicidal behavior was related to the commonly prescribed antibiotic in community infections, amoxicillin. Gait disturbance, a worrying event, especially in the elderly, was associated with azithromycin. Given the seriousness of these potential signals, we suggested further pharmacoepidemiologic studies for more investigation and monitoring by the regulatory agencies.
Data availability statement
Publicly available datasets were analyzed in this study. This data can be found here: https://www.gov.br/anvisa/pt-br/acessoainformacao/dadosabertos/informacoes-analiticas/notificacoes-de-farmacovigilancia.
Author contributions
LB, FS, and EL conceived and presented the idea. LB and AS were responsible for data collection. LB and FS were responsible for the statistical analysis. AS, AC-A, LB, and EL performed data analysis. All authors discussed the results and contributed to the final manuscript.
Acknowledgments
We thank the undergraduate student Constanza Xavier Borges Barbosa (Programa Institucional de Bolsa de Iniciação Científica–PIBIC–Federal University of Rio de Janeiro) for her assistance in data collection.
Conflict of interest
The authors declare that the research was conducted in the absence of any commercial or financial relationships that could be construed as a potential conflict of interest.
Publisher’s note
All claims expressed in this article are solely those of the authors and do not necessarily represent those of their affiliated organizations, or those of the publisher, the editors and the reviewers. Any product that may be evaluated in this article, or claim that may be made by its manufacturer, is not guaranteed or endorsed by the publisher.
References
Agência Nacional de Vigilância Sanitária (2019). Informações sobre a segurança das fluoroquinolonas - informações Técnicas - Anvisa. Available at: http://antigo.anvisa.gov.br/informacoes-tecnicas13?p_p_id=101_INSTANCE_WvKKx2fhdjM2&p_p_col_id=column-1&p_p_col_pos=1&p_p_col_count=2&_101_INSTANCE_WvKKx2fhdjM2_groupId=33868&_101_INSTANCE_WvKKx2fhdjM2_urlTitle=informacoes-sobre-a-seguranca-das-fluoroquinolonas&_101_INSTANCE_WvKKx2fhdjM2_struts_action=%2Fasset_publisher%2Fview_content&_101_INSTANCE_WvKKx2fhdjM2_assetEntryId=5482585&_101_INSTANCE_WvKKx2fhdjM2_type=content [Accessed August 4, 2022].
Agência Nacional de Vigilância Sanitária (2022b). Consultas - agência nacional de Vigilância Sanitária. Available at: https://consultas.anvisa.gov.br/#/(Accessed August 4, 2022).
Agência Nacional de Vigilância Sanitária (2022c). Notificações de farmacovigilância — português (Brasil). Available at: https://www.gov.br/anvisa/pt-br/acessoainformacao/dadosabertos/informacoes-analiticas/notificacoes-de-farmacovigilancia (Accessed August 4, 2022).
Aronson, J. K. (2009). Medication errors: Definitions and classification. Br. J. Clin. Pharmacol. 67, 599–604. doi:10.1111/j.1365-2125.2009.03415.x
Barcelos, F. C., Matos, G. C., de, Silva, M. J. S., da, Silva, F. A. B. da, and Lima, E. da C. (2019). Frontiers in Pharmacology 10. Available at: https://www.frontiersin.org/articles/10.3389/fphar.2019.00498 (Accessed August 4, 2022).Suspected adverse drug reactions related to breast cancer chemotherapy: Disproportionality analysis of the Brazilian spontaneous reporting system.
Boletim de Farmacovigilância No13. 2020. Boletim de Farmacovigilância No13. Available at: https://www.gov.br/anvisa/pt-br/centraisdeconteudo/publicacoes/monitoramento/farmacovigilancia/boletins-de-farmacovigilancia/boletim-de-farmacovigilancia-no-13.pdf.
Cheng, K., Newell, P., Chow, J. W., Broadhurst, H., Wilson, D., Yates, K., et al. (2020). Safety profile of ceftazidime-avibactam: Pooled data from the adult phase II and phase III clinical trial programme. Drug Saf. 43, 751–766. doi:10.1007/s40264-020-00934-3
Del Fiol, F. de S., Bergamaschi, C. de C., De Andrade, I. P., Lopes, L. C., Silva, M. T., and Barberato-Filho, S. (2022). Consumption trends of antibiotics in Brazil during the COVID-19 pandemic. Frontiers in Pharmacology. 13. 844818. doi:10.3389/fphar.2022.844818
Dijkstra, L., Garling, M., Foraita, R., and Pigeot, I. (2020). Adverse drug reaction or innocent bystander? A systematic comparison of statistical discovery methods for spontaneous reporting systems. Pharmacoepidemiol. Drug Saf. 29, 396–403. doi:10.1002/pds.4970
Emanuel Raschi, F. D. P. (2016). Drug utilization research and pharmacovigilance drug utilization research: Methods and applications, 399–407. Available at: https://onlinelibrary.wiley.com/doi/10.1002/9781118949740.ch39 (Accessed August 4, 2022).
European Medicine Agency (2018). Signal management. Eur. Med. Agency. Available at: https://www.ema.europa.eu/en/human-regulatory/post-authorisation/pharmacovigilance/signal-management (Accessed August 1, 2022).
European Medicines Agency (2006). Guideline on the use of statistical signal detection methods in the eudravigilance data analysis system. Available at: https://www.ema.europa.eu/en/documents/regulatory-procedural-guideline/draft-guideline-use-statistical-signal-detection-methods-eudravigilance-data-analysis-system_en.pdf.
European Medicines Agency (2018a). EudraVigilance. Eur. Med. Agency. Available at: https://www.ema.europa.eu/en/human-regulatory/research-development/pharmacovigilance/eudravigilance (Accessed August 4, 2022).
European Medicines Agency (2018b). Medication errors. Eur. Med. Agency. Available at: https://www.ema.europa.eu/en/human-regulatory/post-authorisation/pharmacovigilance/medication-errors (Accessed August 4, 2022).
European Medicines Agency (2018c). Periodic safety update reports (PSURs). Eur. Med. Agency. Available at: https://www.ema.europa.eu/en/human-regulatory/post-authorisation/pharmacovigilance/periodic-safety-update-reports-psurs (Accessed August 4, 2022).
European Medicines Agency (2021). Guideline on registry-based studies. Available at: https://www.ema.europa.eu/en/documents/scientific-guideline/guideline-registry-based-studies_en-0.pdf (Accessed March 22, 2022).
European Medicines Agency (2022). Screening for adverse reactions in EudraVigilance. Available at: https://www.ema.europa.eu/en/documents/other/screening-adverse-reactions-eudravigilance_en.pdf (Accessed August 4, 2022).
Food and Drug Administration, O. (2019). Of the. FDA In Brief: FDA warns that fluoroquinolone antibiotics can cause aortic aneurysm in certain patients. FDA. Available at: https://www.fda.gov/news-events/fda-brief/fda-brief-fda-warns-fluoroquinolone-antibiotics-can-cause-aortic-aneurysm-certain-patients (Accessed August 10, 2021).
Food and Drug Administration, (2021). FDA adverse event reporting system (FAERS) public dashboard. FDA. Available at: https://www.fda.gov/drugs/questions-and-answers-fdas-adverse-event-reporting-system-faers/fda-adverse-event-reporting-system-faers-public-dashboard (Accessed August 4, 2022).
Francino, M. P. (2015). Antibiotics and the human gut microbiome: Dysbioses and accumulation of resistances. Front. Microbiol. 6, 1543. doi:10.3389/fmicb.2015.01543
Habarugira, J. M. V., Härmark, L., and Figueras, A. (2021). Pharmacovigilance data as a trigger to identify antimicrobial resistance and inappropriate use of antibiotics: A study using reports from The Netherlands pharmacovigilance centre. Antibiot. (Basel) 10, 1512. doi:10.3390/antibiotics10121512
Harpaz, R., DuMouchel, W., Shah, N. H., Madigan, D., Ryan, P., and Friedman, C. (2012). Novel data mining methodologies for adverse drug event discovery and analysis. Clin. Pharmacol. Ther. 91, 1010–1021. doi:10.1038/clpt.2012.50
Hauben, M., and Aronson, J. K. (2009). Defining ‘signal’ and its subtypes in pharmacovigilance based on a systematic review of previous definitions. Drug Saf. 32, 99–110. doi:10.2165/00002018-200932020-00003
Huang, L., Guo, T., Zalkikar, J. N., and Tiwari, R. C. (2014). A review of statistical methods for safety surveillance. Ther. Innov. Regul. Sci. 48, 98–108. doi:10.1177/2168479013514236
Jaruratanasirikul, S., Hortiwakul, R., Tantisarasart, T., Phuenpathom, N., and Tussanasunthornwong, S. (1996). Distribution of azithromycin into brain tissue, cerebrospinal fluid, and aqueous humor of the eye. Antimicrob. Agents Chemother. 40, 825–826. doi:10.1128/AAC.40.3.825
Kanao-Kanda, M., Kanda, H., Takahata, O., and Kunisawa, T. (2016). A case of gait disturbance caused by low-dose gabapentin. Ther. Clin. Risk Manag. 12, 927–929. doi:10.2147/TCRM.S107350
Kiguba, R., Olsson, S., and Waitt, C. (2021). Pharmacovigilance in low- and middle-income countries: A review with particular focus on africa. Br. J. Clin. Pharmacol. 1. 1. doi:10.1111/bcp.15193
Li, H., Deng, J., Yu, P., and Ren, X. (2022). Drug-related deaths in China: An analysis of a spontaneous reporting system. Front. Pharmacol. 13, 771953. doi:10.3389/fphar.2022.771953
Mazzei, D., Accardo, J., Ferrari, A., and Primavera, A. (2012). Levofloxacin neurotoxicity and non-convulsive status epilepticus (NCSE): A case report. Clin. Neurol. Neurosurg. 114, 1371–1373. doi:10.1016/j.clineuro.2012.03.029
MedDRA, . (2020). International Council for Harmonisation of Technical Requirements for Pharmaceuticals for human use . Organisation | MedDRA. Available at: https://www.meddra.org/about-meddra/organisation (Accessed August 4, 2022).
Meyboom, R. H. B., Egberts, A. C. G., Edwards, I. R., Hekster, Y. A., de Koning, F. H. P., and Gribnau, F. W. J. (1997). Principles of signal detection in pharmacovigilance. Drug Saf. 16, 355–365. doi:10.2165/00002018-199716060-00002
Montastruc, J.-L., Sommet, A., Bagheri, H., and Lapeyre-Mestre, M. (2011). Benefits and strengths of the disproportionality analysis for identification of adverse drug reactions in a pharmacovigilance database. Br. J. Clin. Pharmacol. 72, 905–908. doi:10.1111/j.1365-2125.2011.04037.x
Montastruc, J.-L., Lafaurie, M., de Canecaude, C., Durrieu, G., Sommet, A., Montastruc, F., et al. (2021). Fatal adverse drug reactions: A worldwide perspective in the World health organization pharmacovigilance database. Br. J. Clin. Pharmacol. 87, 4334–4340. doi:10.1111/bcp.14851
Pirker, W., and Katzenschlager, R. (2017). Gait disorders in adults and the elderly : A clinical guide. Wien. Klin. Wochenschr. 129, 81–95. doi:10.1007/s00508-016-1096-4
Pohlmann, G. (1966). Respiratory arrest associated with intravenous administration of polymyxin B sulfate. JAMA 196, 181–183. doi:10.1001/jama.1966.03100150127040
Riesselman, A., and El-Mallakh, R. S. (2015). Akathisia with azithromycin. Ann. Pharmacother. 49, 609. doi:10.1177/1060028015570728
Rodriguez, V., Green, S., and Bodey, G. P. (1970). Serum electrolyte abnormalities associated with the administration of polymyxin B in febrile leukemic patients. Clin. Pharmacol. Ther. 11, 106–111. doi:10.1002/cpt1970111106
Rothman, K. J., Lanes, S., and Sacks, S. T. (2004). The reporting odds ratio and its advantages over the proportional reporting ratio. Pharmacoepidemiol. Drug Saf. 13, 519–523. doi:10.1002/pds.1001
Samyde, J., Petit, P., Hillaire-Buys, D., and Faillie, J.-L. (2016). Quinolone antibiotics and suicidal behavior: Analysis of the World health organization’s adverse drug reactions database and discussion of potential mechanisms. Psychopharmacol. Berl. 233, 2503–2511. doi:10.1007/s00213-016-4300-3
Sartelli, M., Hardcastle, C., Catena, F., Chichom-Mefire, A., Coccolini, F., et al. (2020). Antibiotic use in low and middle-income countries and the challenges of antimicrobial resistance in surgery. Antibiot. (Basel) 9, 497. doi:10.3390/antibiotics9080497
Scavone, C., Mascolo, A., Ruggiero, R., Sportiello, L., Rafaniello, C., Berrino, L., et al. (2020). Quinolones-induced musculoskeletal, neurological, and psychiatric ADRs: A pharmacovigilance study based on data from the Italian spontaneous reporting system. Frontiers in Pharmacology. 11. 1. doi:10.3389/fphar.2020.00428
Seelig, M. S. (1966). Mechanisms by which antibiotics increase the incidence and severity of candidiasis and alter the immunological defenses. Bacteriol. Rev. 30, 442–459. doi:10.1128/br.30.2.442-459.1966
Silva, A. R. O., Salgado, D. R., Lopes, L. P. N., Castanheira, D., Emmerick, I. C. M., and Lima, E. C. (2021). Increased use of antibiotics in the intensive care unit during coronavirus disease (COVID-19) pandemic in a Brazilian hospital. Frontiers in Pharmacology 12. 1. doi:10.3389/fphar.2021.778386
Small, G. A. (1964). Report of a case. Respiratory paralysis after a large dose of intraperitoneal polymyxin B. And bacitracin. Anesth. Analg. 43, 137–139. doi:10.1213/00000539-196403000-00003
Tamma, P. D., Avdic, E., Li, D. X., Dzintars, K., and Cosgrove, S. E. (2017). Association of adverse events with antibiotic use in hospitalized patients. JAMA Intern. Med. 177, 1308–1315. doi:10.1001/jamainternmed.2017.1938
Touboul-Lundgren, P., Jensen, S., Drai, J., and Lindbæk, M. (2015). Identification of cultural determinants of antibiotic use cited in primary care in europe: A mixed research synthesis study of integrated design “culture is all around us. BMC Public Health 15, 908. doi:10.1186/s12889-015-2254-8
Ul Mustafa, Z., Salman, M., Aldeyab, M., Kow, C. S., and Hasan, S. S. (2021). Antimicrobial consumption among hospitalized patients with COVID-19 in Pakistan. SN Compr. Clin. Med. 3, 1691–1695. doi:10.1007/s42399-021-00966-5
Van Puijenbroek, E. P., Bate, A., Leufkens, H. G. M., Lindquist, M., Orre, R., and Egberts, A. C. G. (2002). A comparison of measures of disproportionality for signal detection in spontaneous reporting systems for adverse drug reactions. Pharmacoepidemiol. Drug Saf. 11, 3–10. doi:10.1002/pds.668
Vieira, J. M. de L., de Matos, G. C., da Silva, F. A. B., Bracken, L. E., Peak, M., and Lima, E. da C. (2020). Serious adverse drug reactions and safety signals in children: A nationwide database study. Front. Pharmacol. 11, 964. doi:10.3389/fphar.2020.00964
Vogler, M., Ricci Conesa, H., de Araújo Ferreira, K., Moreira Cruz, F., Simioni Gasparotto, F., Fleck, K., et al. (2020). Electronic reporting systems in pharmacovigilance: The implementation of VigiFlow in Brazil. Pharm. Med. 34, 327–334. doi:10.1007/s40290-020-00349-6
Wilton, L., Kollarova, M., Heeley, E., and Shakir, S. (2003). Relative risk of vaginal candidiasis after use of antibiotics compared with antidepressants in women: Postmarketing surveillance data in england. Drug Saf. 26, 589–597. doi:10.2165/00002018-200326080-00005
World Health Organization (2012). A practical handbook on the pharmacovigilance of medicines used in the treatment of tuberculosis: enhancing the safety of the TB patient. Geneva. World Health Organization. Available at: https://apps.who.int/iris/handle/10665/336226 (Accessed August 4, 2022).
World Health Organization (2018). WHO report on surveillance of antibiotic consumption: 2016-2018 early implementation. Available at: https://apps.who.int/iris/handle/10665/277359 (Accessed August 4, 2022).
World Health Organization (2019). AWaRe policy brief. Available at: https://adoptaware.org/assets/pdf/aware_policy_brief.pdf (Accessed July 27, 2021).
World Health Organization (2020). WHOCC - ATC/DDD index. Available at: https://www.whocc.no/atc_ddd_index/(Accessed August 12, 2021).
World Health Organization (2021). WHO Model lists of essential medicines. Available at: https://www.who.int/groups/expert-committee-on-selection-and-use-of-essential-medicines/essential-medicines-lists (Accessed August 4, 2022).
World Health Organization (2022a). Uppsala monitoring centre. Available at: https://who-umc.org/(Accessed August 4, 2022).
World Health Organization (2022b). VigiAccess. Available at: https://www.vigiaccess.org/(Accessed August 3, 2022).
Keywords: pharmacovigilance, adverse drug reaction reporting systems, anti-bacterial agents, drug-related side effects and adverse reactions, safety signals, spontaneous reports
Citation: Barbosa LHLA, Silva ARO, Carvalho-Assef APD, Lima EC and da Silva FAB (2022) Potential safety signals for antibacterial agents from the Brazilian national pharmacovigilance database (Vigimed/VigiFlow). Front. Pharmacol. 13:948339. doi: 10.3389/fphar.2022.948339
Received: 19 May 2022; Accepted: 02 September 2022;
Published: 20 September 2022.
Edited by:
Luciane Cruz Lopes, University of Sorocaba, BrazilReviewed by:
Mohammad Daud Ali, Mohammed Al Mana College for Health Sciences (MACHS), Saudi ArabiaTatiane Ribeiro, University of São Paulo, Brazil
Copyright © 2022 Barbosa, Silva, Carvalho-Assef, Lima and da Silva. This is an open-access article distributed under the terms of the Creative Commons Attribution License (CC BY). The use, distribution or reproduction in other forums is permitted, provided the original author(s) and the copyright owner(s) are credited and that the original publication in this journal is cited, in accordance with accepted academic practice. No use, distribution or reproduction is permitted which does not comply with these terms.
*Correspondence: Elisangela Costa Lima, eclima.ufrj@gmail.com; Fabricio Alves Barbosa da Silva, fabricio.silva@fiocruz.br