- 1Department of General Surgery, Hepatobiliary Surgery, Shanghai Institute of Digestive Surgery, Ruijin Hospital, Shanghai Jiao Tong University School of Medicine, Shanghai, China
- 2Department of Clinical Laboratory Medicine, Shanghai Tenth People’s Hospital of Tongji University, Shanghai, China
- 3Department of Infectious Diseases, Ruijin Hospital, Shanghai Jiao Tong University School of Medicine, Shanghai, China
Background: Hepatocellular carcinoma (HCC) is a lethal disease with high relapse and dismal survival rates. Alternative splicing (AS) plays a crucial role in tumor progression. Herein, we aim to integratedly analyze the relapse-associated AS events and construct a signature predicting tumor relapse in stage I–III HCC.
Methods: AS events of stage I–III HCC with tumor relapse or long-term relapse-free survival were profiled to identify the relapse-associated AS events. A splicing network was set up to analyze the correlation between the relapse-associated AS events and splicing factors. Cox regression analysis and receiver operating characteristic curve were performed to develop and validate the relapse-predictive AS signature. Single-sample gene set enrichment analysis (ssGSEA) and the ESTIMATE algorithm were used to assess the immune infiltration status of the HCC microenvironment between different risk subgroups. Unsupervised cluster analysis was conducted to assess the relationship between molecular subtypes and local immune status and clinicopathological features.
Results: In total, 2441 ASs derived from 1634 mRNA were identified as relapse-associated AS events. By analyzing the proteins involved in the relapse-associated AS events, 1573 proteins with 11590 interactions were included in the protein–protein interaction (PPI) network. In total, 16 splicing factors and 61 relapse-associated AS events with 85 interactions were involved in the splicing network. The relevant genes involved in the PPI network and splicing network were also analyzed by Gene Ontology enrichment analysis. Finally, we established a robust 16-gene AS signature for predicting tumor relapse in stage I–III HCC with considerable AUC values in all of the training cohort, testing cohort, and entire cohort. The ssGSEA and ESTIMATE analyses showed that the AS signature was significantly associated with the immune status of the HCC microenvironment. Moreover, four molecular subgroups with distinguishing tumor relapse modes and local immune status were also revealed.
Conclusion: Our study built a novel 16-gene AS signature that robustly predicts tumor relapse and indicates immune activity in stage I–III HCC, which may facilitate the deep mining of the mechanisms associated with tumor relapse and tumor immunity and the development of novel individualized treatment targets for HCC.
Introduction
Liver cancer is the third leading cause of cancer-associated deaths worldwide (Sung et al., 2021). Hepatocellular carcinoma (HCC) is the most predominant type of liver cancer, accounting for 75–85% of cases (Singal et al., 2020). Surgical resection remains the most effective therapy for HCC with curative potential, yet the high frequency of tumor relapse (50–70% for 5 years after surgery) hinders the improved survival (European Association for the Study of the Liver Electronic address and European Association for the Study of the Liver, 2018). Relevant data revealed that 70% and more than 90% of the tumor relapse occurred within 2 and 5 years after surgery, respectively (Zheng et al., 2017). Tumor relapse of HCC is always associated with poor therapeutic response and survival due to aggressive pathological characteristics. Hence, a thorough exploration of the mechanisms underlying tumor relapse and discovery of robust relapse predictive factors for HCC is urgently needed to further improve the long-term prognosis of HCC patients.
Tumor progression is a complex process involving many genetic alterations, which may result in activating oncogenes, inactivating tumor suppressors, or enhancing tumor cells invading normal organs/tissues. The alterations in the genetic central dogma lead to aberrant expression of relevant oncogenes or suppressor genes. Alternative splicing (AS) of mRNA is a common event in the process of the genetic central dogma, which occurs in more than 95% of human multi-exon genes and results in encoding of different splicing and protein isoforms (Nilsen and Graveley, 2010). In addition to that, the translation of mRNA isomer can be downregulated by termination codons originating from AS switches (Climente-Gonzalez et al., 2017). Therefore, AS plays a critical role in the maintenance of homeostasis for cells or organisms, and increasing evidence reveals that dysregulation of AS is closely inclined to various diseases, including tumor development, immune escape, and progression (Urbanski et al., 2018; Zhang et al., 2021a). AS events are regulated by splicing factors (SFs); changed expression or mutations of SFs can lead to complete alterations of AS and may result in tumor-specific splicing isoforms in human cancers (Salton et al., 2015; Wang et al., 2019; Song et al., 2020). Thus, dissecting the tumor-specific AS isoforms and the splicing network between ASs and SFs could provide insights into the mechanisms of tumor development and progression, which may offer prognostic tumor biomarkers and potential therapeutic targets.
Several studies have profiled the tumor-specific AS events in HCC and identified AS signatures that are associated with overall survival in HCC (Zhu et al., 2019; Cai et al., 2020; Wu et al., 2020). However, a comprehensive depiction of the relapse-associated AS events and robust AS signatures predicting tumor relapse in HCC remains lacking. Herein, we integratedly analyze the genome-wide AS events from the HCC cohort in The Cancer Genome Atlas (TCGA) database and illustrate the relapse-associated AS events in stage I–III HCC. More importantly, we built a novel 16-gene AS signature that predicts tumor relapse and indicates immune activity in stage I–III HCC with high performance, shedding light on the individualized therapeutic targets for HCC.
Materials and Methods
Data extraction and pre-processing
Raw RNA sequence data of the liver hepatocellular carcinoma (LIHC) cohort was extracted from the TCGA database (https://portal.gdc.cancer.gov/) (Hutter and Zenklusen, 2018), and the corresponding clinicopathological information including age, sex, hepatitis virus infection status, tumor grade, tumor stage, relapse, and survival status was downloaded from the University of California Santa Cruz (UCSC) Xena platform (https://xena.ucsc.edu/) (Goldman et al., 2020). The inclusion criteria for the present study were as follows: (I) R0 resection was achieved in the surgical procedure; (II) the histopathological diagnosis was HCC; (III) the pathological TNM stage of HCC was stage I, stage II, or stage III; (IV) HCC patients with complete clinicopathological and survival information; (V) HCC patients with overall survival time over than 30 days; (VI) corresponding AS event data were available. According to the inclusion criteria, we finally enrolled 277 HCC patients in this study for further analysis. The mRNA AS profiles of HCC were obtained from TCGA SpliceSeq (https://bioinformatics.mdanderson.org/TCGA-SpliceSeq/) (Ryan et al., 2016). Seven types of AS were illustrated in Figure 1A, namely, alternate acceptor site (AA), alternate donor site (AD), alternate promoter (AP), alternate terminator (AT), exon skip (ES), mutually exclusive exons (ME), and retained intron (RI). The percent-spliced-in (PSI) value, ranging from 0 to 1, was a normalized method to evaluate the AS events. In order to achieve credible data on AS events, the percentage of clinical samples with PSI values greater than or equal to 75% were included in the present study. Furthermore, relevant splicing factor (SF) data were acquired from the SpliceAid-F database (http://www.caspur.it/SpliceAidF) (Giulietti et al., 2013).
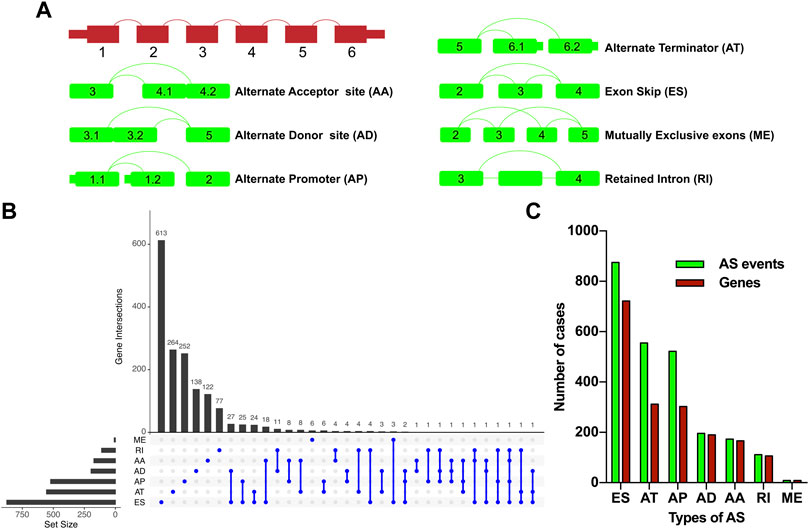
FIGURE 1. Overview of the relapse-associated AS event profiling in HCC. (A) Illustrations for the seven subtypes of AS events including AA, AD, AP, AT, ES, ME, and RI. (B) UpSet plot delineating the overlaps of the seven subtype AS events and the relevant mRNA. One mRNA owns four splicing patterns at the maximum. (C) Number of the seven subtype AS events and the involved genes. Abbreviations: AA, alternate acceptor site; AD, alternate donor site; AP, alternate promoter; AT, alternate terminator; ES, exon skip; ME, mutually exclusive exons; RI, retained intron.
Identification of relapse-associated alternative splicing events in stage I–III hepatocellular carcinoma
The cohort of enrolled stage I–III HCC patients was divided into a relapse group and a long-term relapse-free survival (RFS) group. The relapse group was defined as HCC patients who suffered tumor recurrence or distant metastasis after R0 hepatic resection, and the long-term RFS group was defined as HCC patients without tumor recurrence or distant metastasis after a minimum follow-up time of 3 years after R0 hepatic resection. The propensity score matching (PSM) method was conducted to achieve more balanced groups by matching TNM stage and Path_T, which showed a significant influence on tumor relapse. The relapse group and long-term RFS group were matched as 2:1. The Wilcoxon test was performed to identify relapse-associated AS events, and p-values less than 0.05 were considered statistically significant. The UpSet plot was used for illustrating the relapse-associated AS events. Gene Ontology (GO) functional enrichment analysis was performed using the R package clusterProfiler on the relevant genes of the relapse-associated AS events to identify significantly enriched biological processes (BP), cellular components (CC), and molecular functions (MF).
Construction of protein–protein interaction network and regulatory splicing network
The related genes based on the relapse-associated AS events were further analyzed by performing protein–protein interaction (PPI) analysis on the online STRING Version 11.5 database (www.string-db.org/) (Szklarczyk et al., 2019). MCODE and Cytohubba in Cytoscape (version 3.8.2) were used for clustering the PPI network and selecting hub proteins, respectively. The correlation between the expression level of SFs and the PSI level of the relapse-associated AS events was assessed by Spearman’s test; p values less than 0.01 and the values of correlation coefficient (cor) less than −0.55 or over 0.55 were considered statistically significant. Finally, the results of the PPI network and the regulatory splicing network were visualized by Cytoscape (version 3.8.2).
Survival analysis and alternative splicing signature identification for predicting tumor relapse in stage I–III hepatocellular carcinoma
The enrolled stage I–III HCC patients were randomly divided into the training cohort and testing cohort with a ratio of 7:3. The training cohort was used to build the relapse predictive AS signature, while the testing cohort and entire cohort were used to validate the accuracy. Then, a univariate Cox regression analysis was performed to explore the effects of the identified relapse-associated AS events on RFS in stage I–III HCC, and p values less than 0.05 were considered statistically significant. The data of those AS events were visualized as volcano plot, UpSet plot, and bubble plot. To enhance the robustness of the signature, only AS events with p values less than 0.0005 in the univariate Cox regression model were included for further screening by LASSO Cox regression analysis (Liu et al., 2021a; Zhang et al., 2021b). Finally, multivariate Cox regression analysis was conducted, and AS events with p values less than 0.05 were selected to establish the relapse predictive signature. The predictive accuracy of the final signature was evaluated by risk score analysis, RFS survival analysis, and receiver operating characteristic (ROC) curve. Subgroup analysis was also conducted to further investigate the prognostic significance of the signature via stratifying the entire cohort into different subgroups based on age, gender, T stage, and TNM stage. The cutoff point of the low-risk and high-risk score group was identified using the R package survminer. The univariate, LASSO, and multivariate Cox regression analyses were conducted using R language (version 4.0.3).
Functional enrichment analysis and immune activity analysis
Kyoto Encyclopedia of Genes and Genomes (KEGG) functional enrichment analysis was performed using the R package clusterProfiler to identify the biological functions and pathways associated with the relapse predictive AS signature. Single-sample gene set enrichment analysis (ssGSEA) was conducted to analyze the local immune infiltration levels of immune cell types, immune-related functions, and pathways in HCC using the R package GSVA (Liu et al., 2021b; Wang et al., 2022). Moreover, the ESTIMATE algorithm was applied using the R package ESTIMATE to evaluate the infiltration degrees of immune and stromal cells within the HCC microenvironment, which further validated the effectuality of ssGSEA analysis.
Identification and analysis of molecular subtype clusters
Considering AS events varied much differently at the personal level, unsupervised consensus clustering was performed by the R package ConsensusClusterPlus to achieve a more robust classification based on the AS events involved in the relapse predictive signature. Elbow and Gap analysis was conducted to identify the optional number of clusters, and the consensus molecular subtype was classified using the R package CMScaller. The correlation between molecular subtypes and RFS was illustrated as the Kaplan–Meier plot, and the correlations between molecular subtypes and local immune infiltration status and clinicopathological features were illustrated as a heatmap.
Results
Identification of relapse-associated alternative splicing events in stage I–III hepatocellular carcinoma
277 patients with stage I–III HCC from the TCGA-LIHC cohort were enrolled in the present study; 131 cases and 39 cases of which were sorted as the relapse group and long-term RFS group, respectively. PSM analysis was conducted to achieve more balanced groups by matching TNM stage and Path_T, which showed a significant influence on tumor relapse. The summary clinicopathological features of the two groups before and after PSM are listed in Table 1. Seven types of AS, namely, AA, AD, AP, AT, ES, ME, and RI are presented in Figure 1A. To identify the AS events associated with HCC relapse, we compared the PSI values of the HCC patients in the two groups. Then, we identified 2,441 significantly differently expressed AS events derived from 1,634 mRNA, containing 875 ES from 722 mRNA, 555 AT from 312 mRNA, 522 AP from 303 mRNA, 196 AD from 190 mRNA, 173 AA from 166 mRNA, 111 RI from 106 mRNA, and nine ME from nine mRNA (Figure 1C). The most frequent AS type was ES which accounted for more than half of the total relapse-associated AS events, while ME was the least common AS type. The overview of these AS events was depicted in the UpSet plot, which also presented the overlapping of the relapse-associated AS events and relevant mRNA in detail (Figure 1B). As illustrated in the UpSet plot, diverse types of AS events might be derived from one mRNA, and one single mRNA could possess four splicing patterns at the maximum. To investigate the potential function of the relapse-associated AS events, the relevant genes of which underwent GO enrichment analysis were analyzed. Representative significant enriched terms (p < 0.05) in the three GO categories (BP, CC, and MF) are shown in Supplementary Figure S1.
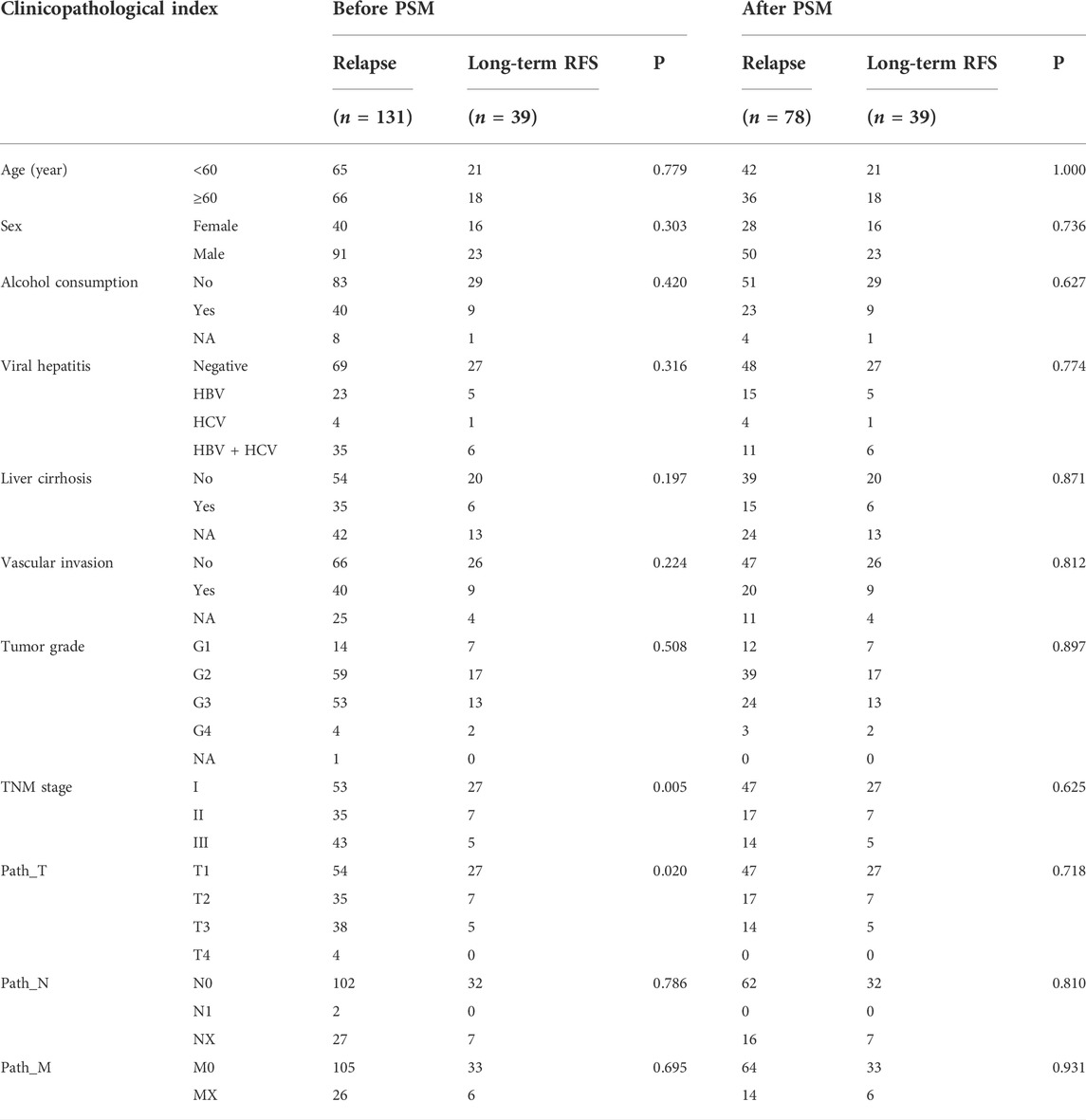
TABLE 1. Clinicopathological characteristics of HCC patients in relapse and long-term RFS groups before and after PSM.
Construction of protein–protein interaction network and regulatory splicing network
The PPI network was constructed based on the relevant proteins of the relapse-associated AS events, totally including 1573 proteins with 11590 interactions (Supplementary Table S1). Meanwhile, 34 clusters were identified, and the individual score of those clusters ranged from 2.500 to 31.937. Figures 2A–C in detail depicted the top three clusters, which scored 31.937, 18.222, and 10.367. In the cluster owning the highest score, 64 proteins with 1006 interactions were included (Figure 2A); in the cluster ranking second, 55 proteins with 492 interactions were included (Figure 2B); in the following cluster, 61 proteins with 311 interactions were included (Figure 2C). Among the proteins included in the cluster with the highest score, ten proteins (AKT1, ALB, HNRNPA1, VEGFA, SRSF1, RHOA, RPS3, MDM2, CUL1, and PTBP1) were identified as hub proteins, in which, AKT1 and ALB obtained the top place followed by HNRNPA1, VEGFA, and SRSF1, while PTBP1 was the tail ender (Figure 2D).
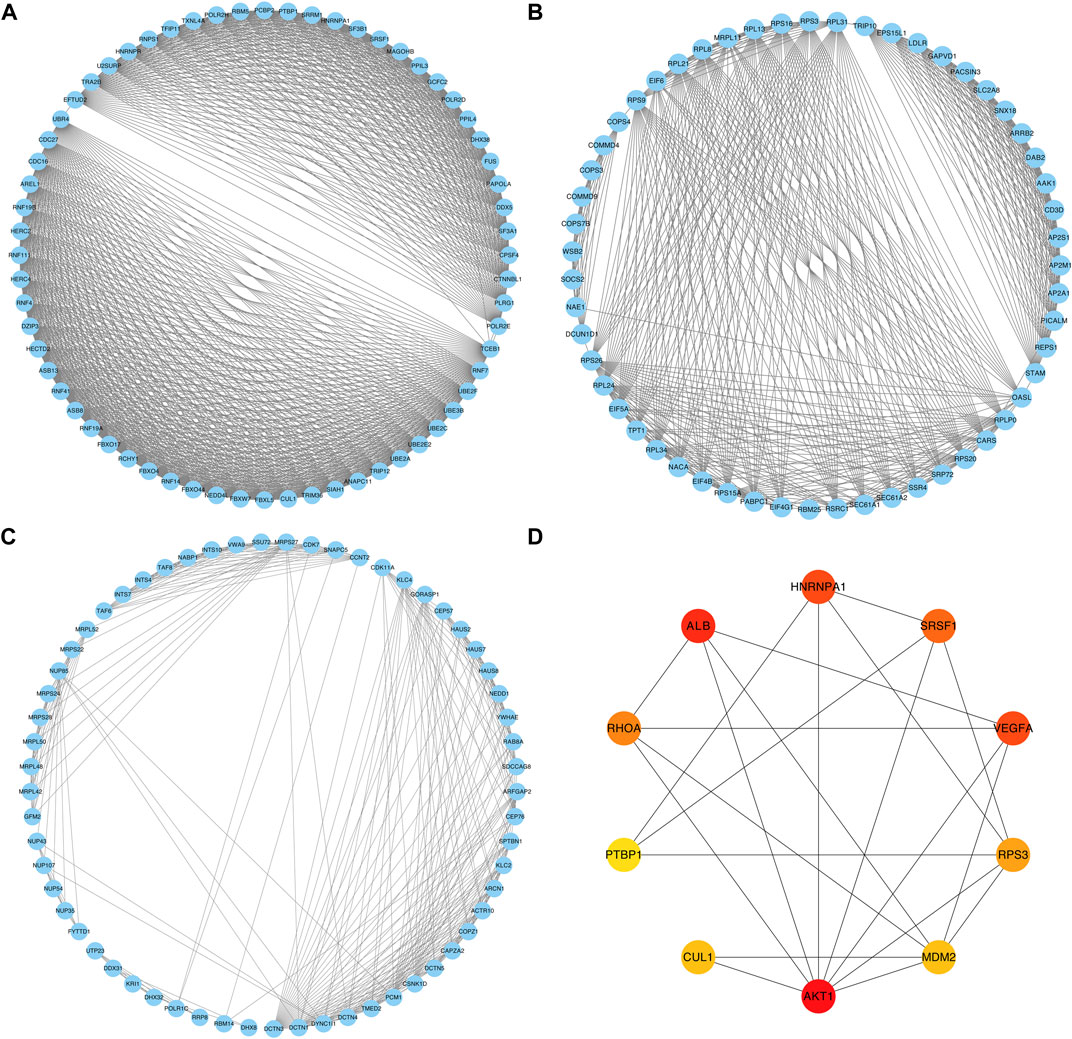
FIGURE 2. PPI networks of the relevant proteins of the relapse-associated AS events. (A) PPI network of the cluster with the highest score. (B) PPI network of the cluster ranking second. (C) PPI network of the cluster ranking third. (D) Network of the top ten hub proteins in the cluster owning the highest score. The color of the nodes represents the rank of hub proteins ordered as follows: red, orange, and yellow.
A regulatory splicing network was constructed by analyzing the correlation of the expression level of the SFs and the PSI level of the relapse-associated AS events with a strict standard. In total, 16 SFs were significantly related to 60 relapse-associated AS events by forming 85 interactions (including 36 positive and 49 negative regulation) in the splicing network. Moreover, 27 relapse-associated AS events were significantly upregulated and the others were significantly downregulated in the relapse group, as compared with the long-term RFS group. ARAF-88922-AT, ARAF-88921-AT, FMO5-7368-AT, MRPS24-79351-AT, and MRPS24-79350-AT were considered the hub relapse-associated AS events in the splicing network. Meanwhile, PCBP1, RBM25, QKI, TIA1, and RBFOX2 were identified as the core SFs, which owned the most interaction with the relapse-associated AS events, implying their dominant position in determining the relapse-associated AS events in stage I–III HCC (Figure 3A; Supplementary Table S2). Subsequently, the relevant genes involved in the regulatory splicing network underwent GO enrichment analysis. Representative significant enriched terms (p < 0.05) in the three GO categories (BP, CC, and MF) are shown in Figure 3B.
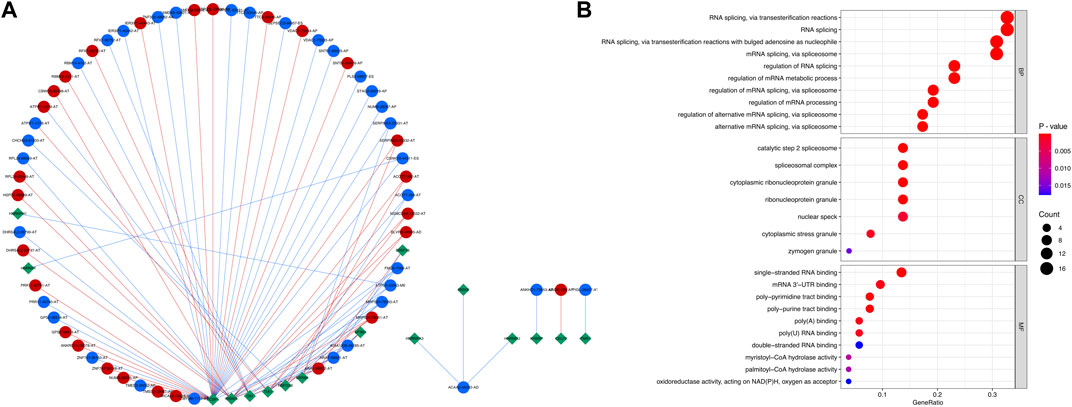
FIGURE 3. Regulatory splicing network and functional enrichment analysis of the SFs and relapse-associated AS events. (A) Regulatory splicing network of the SFs and relapse-associated AS events. Diamond nodes represent SF, and the circle nodes represent AS events. The AS events represented by red nodes and blue nodes were upregulated and downregulated, respectively, by comparison of the relapse group to the long-term RFS group. The red and blue lines showed positive and negative correlations between SFs and AS events, respectively. (B) Functional enrichment analysis of the relevant genes involved in the regulatory splicing network. The top ten significant enriched terms in the three GO categories (BP, CC, and MF) were shown. Abbreviations: BP, biological processes; CC, cellular components; GO, Gene Ontology; MF, molecular functions.
Survival analysis and relapse predictive alternative splicing signature construction
Univariate Cox regression analysis was further performed to explore the prognostic value of the relapse-associated AS events mentioned earlier. A total of 496 AS events derived from 377 mRNA were detected to be prognostically significant (p < 0.05). The statistically significant AS events (Z-score < −2 or > 2, p < 0.05) are shown in the Volcano plot and Upset plot (Figures 4A,B). The Upset plot also showed the overlapping of the statistically significant AS events and relevant mRNA in detail (Figure 4B). ME was the only AS type that was not involved in the statistically significant AS events, and ES was still the most frequent AS type. A bubble plot was further performed to depict the representative top 20 AS events in each AS type (Figure 4C). SETMAR-62996-AA, SERBP1-3355-AA, and COMT-61102-AA in AS type of AA; TAF6-80899-AD, C6orf1-75778-AD and RPS16-49830-AD in AS type of AD; TJP2-86533-AP, SERPIND1-61191-AP, and SERPIND1-61190-AP in AS type of AP; SUFU-12963-AT, AP1S2-88571-AT, and AP1S2-88569-AT in AS type of AT; FAM98C.49642.ES, COMMD4-31852-ES, and PRDX5-16639-ES in AS type of ES; SYNGR2-43774-RI, CLU.83171.RI, and RNASEH2C.16916.RI in the AS type of RI were the top three AS events in each AS type. Subsequently, the AS events with p values less than 0.0005 in the univariate Cox regression were further screened by LASSO Cox regression analysis. The results of cross-validation for tuning parameter selection and LASSO coefficient profiles of the AS events are shown in Figures 5A,B. Through minimum criteria, 66 AS events with non-zero coefficients at lambda.min were filtered by the LASSO Cox regression analysis.
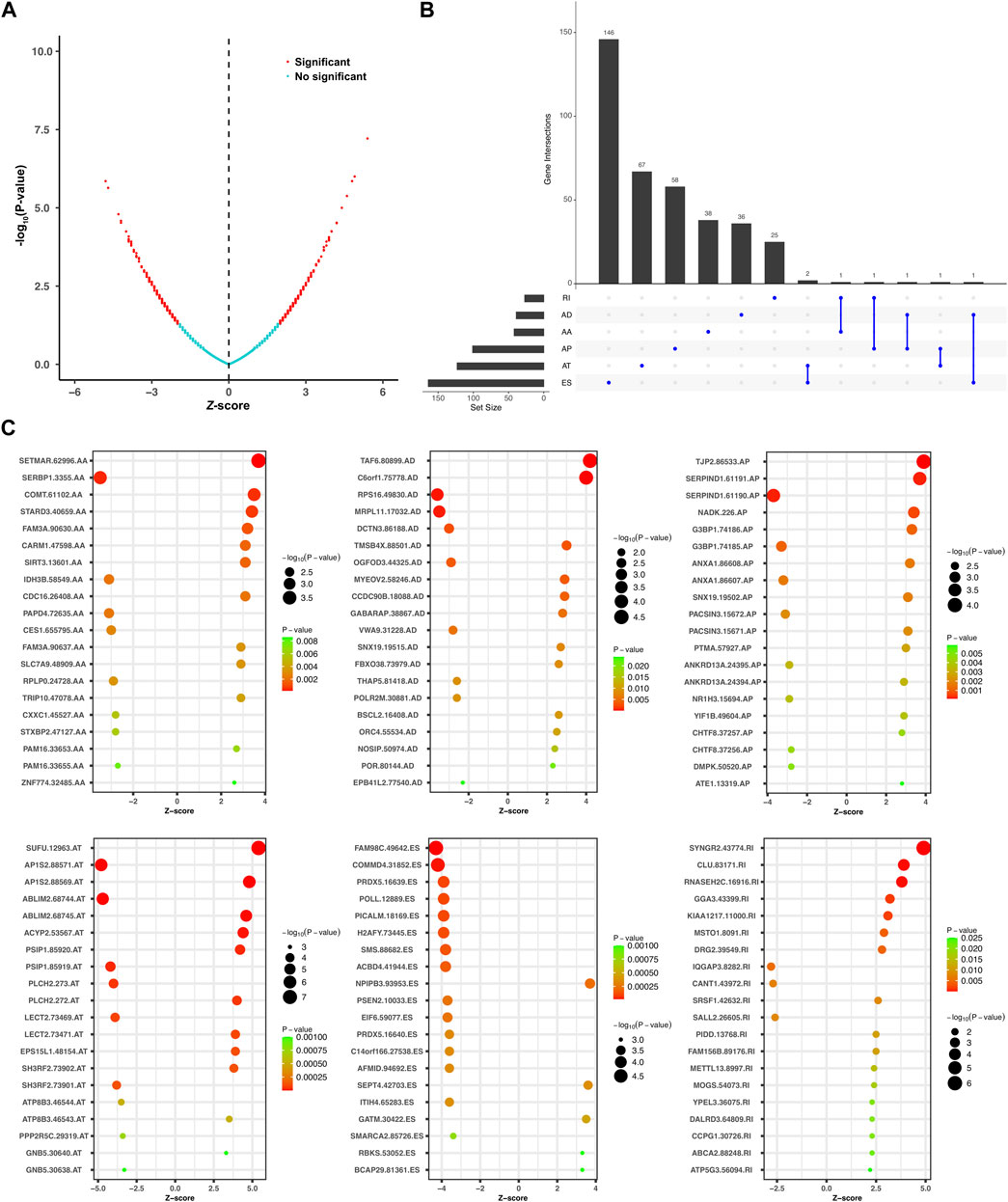
FIGURE 4. Overview of the relapse-associated AS events from initial screening by univariate Cox regression analysis. (A) Volcano plot showing the statistically significant relapse-associated AS events, which was presented by red. (B) UpSet plot delineating the overlaps of the statistically significant relapse-associated AS events and the relevant mRNA. One mRNA owns two splicing patterns at the maximum. (C) Bubble plots delineating the distribution of each type of statistically significant relapse-associated AS events after univariate Cox regression analysis. Representative top 20 AS events in AA, AD, AP, AT, ES, and RI were shown.
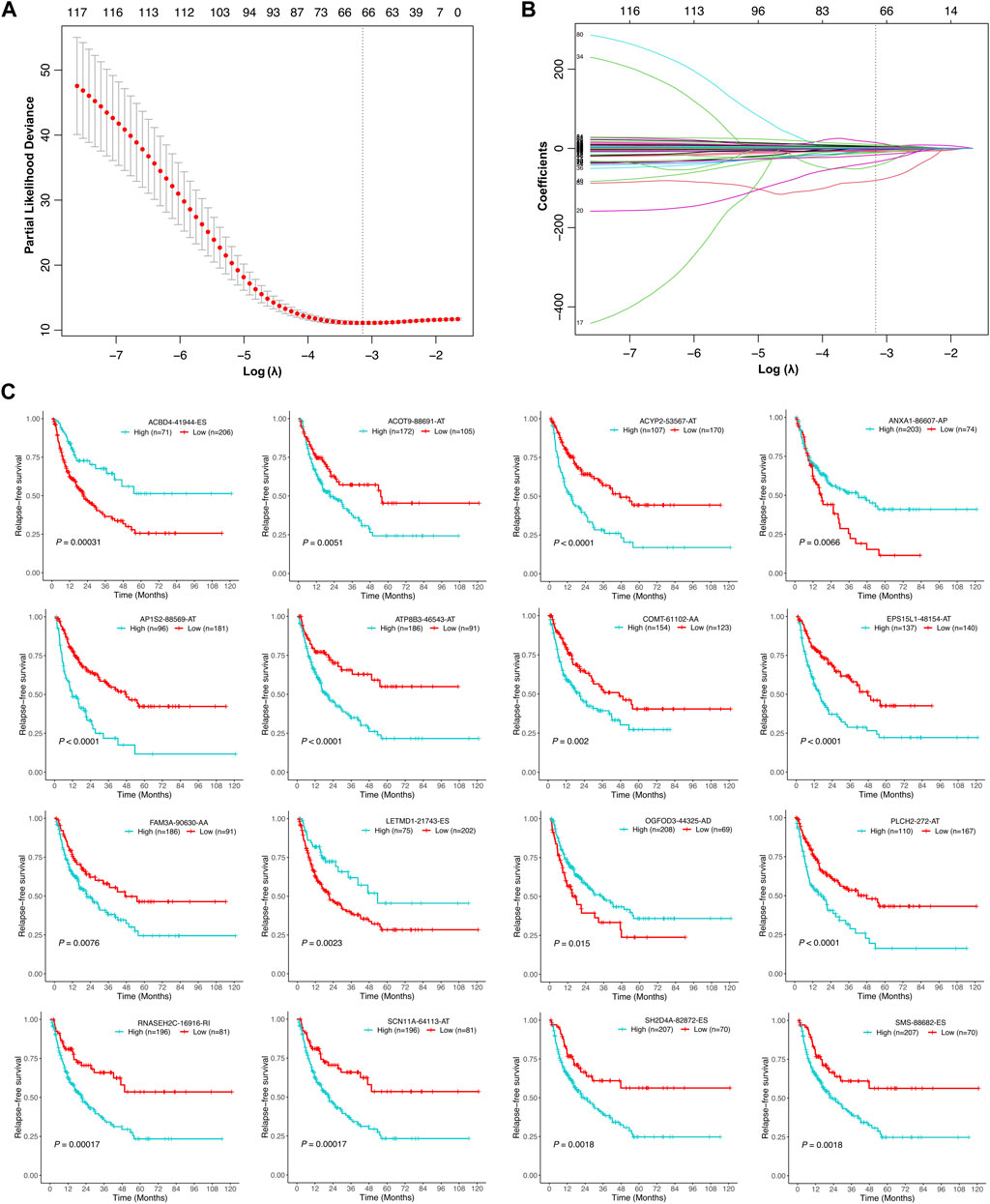
FIGURE 5. Selection of the optimal relapse-associated AS events for constructing the relapse predictive signature. (A) Cross-validation for tuning parameter selection in the LASSO Cox regression. The optimal value determined by minimum criteria is marked by a dotted vertical line. (B) LASSO coefficient profiles of the candidate relapse-associated AS events in the LASSO Cox regression. The optimal value determined by minimum criteria is marked by a dotted vertical line. (C) Kaplan–Meier survival analysis of the individual AS events involved in the relapse predictive signature.
Finally, those 66 AS events were analyzed by multivariate Cox regression analysis. AS events with p values less than 0.05 were selected to establish the relapse predictive signature, and 16 AS events were included (Table 2). Kaplan–Meier survival analysis revealed that all the 16 AS events were significantly associated with the RFS of HCC patients; high expression of ACOT9-88691-AT, ACYP2-53567-AT, AP1S2-88569-AT, ATP8B3-46543-AT, COMT-61102-AA, EPS15L1-48154-AT, FAM3A-90630-AA, PLCH2-272-AT, RNASEH2C-16916-RI, SCN11A-64113-AT, SH2D4A-82872-ES, and SMS-88682-ES was correlated with poor RFS in HCC, whereas low expression of ACBD4-41944-ES, ANXA1-86607-AP, LETMD1-21743-ES, and OGFOD3-44325-AD was correlated with poor RFS in HCC (Figure 5C). Based on the 16-gene AS signature, the following formula was developed to calculate the risk score of tumor relapse: risk score = (−9.15 × PSI value of ACBD4-41944-ES) + (6.14 × PSI value of ACOT9-88691-AT) + (12.70 × PSI value of ACYP2-53567-AT) + (−41.19 × PSI value of ANXA1-86607-AP) + (3.01 × PSI value of AP1S2-88569-AT) + (2.65 × PSI value of ATP8B3-46543-AT) + (20.94 × PSI value of COMT-61102-AA) + (2.98 × PSI value of EPS15L1-48154-AT) + (4.98 × PSI value of FAM3A-90630-AA) + (−1.48 × PSI value of LETMD1-21743-ES) + (−6.67 × PSI value of OGFOD3-44325-AD) + (1.65 × PSI value of PLCH2-272-AT) + (3.77 × PSI value of RNASEH2C-16916-RI) + (9.59 × PSI value of SCN11A-64113-AT) + (8.13 × PSI value of SH2D4A-82872-ES) + (−80.63 × PSI value of SMS-88682-ES).
The cutoff point of the risk score was determined using the R package survminer. According to the cutoff point, the three cohorts of the stage I–III HCC patients (the training cohort, testing cohort, and entire cohort) were divided into the low-risk group and high-risk group, respectively. Risk score and RFS survival analysis suggested that the relapse predictive signature has great efficiency in distinguishing the low-risk and high-risk groups in all three cohorts (Figures 6A–C). To further assess the performance of the relapse predictive signature, the ROC curve was plotted at different time points after surgery. The AUC values of different ROC curves at 1, 2, 3, and 5 year after surgery were considerable and stable in all three cohorts, which displayed good relapse predictive performance (Figures 6A–C). The relationship between the risk score and the clinicopathological features in the entire cohort was illustrated as a heatmap, and significant correlations were identified between the risk score and tumor relapse, tumor stage, and T classification (Figure 6D). Furthermore, univariate and multivariate Cox regression analysis was performed to assess the significance of the clinicopathological characteristics and risk score. The results showed that the risk score was an independent predictor of tumor relapse in HCC patients (Supplementary Figure S2).
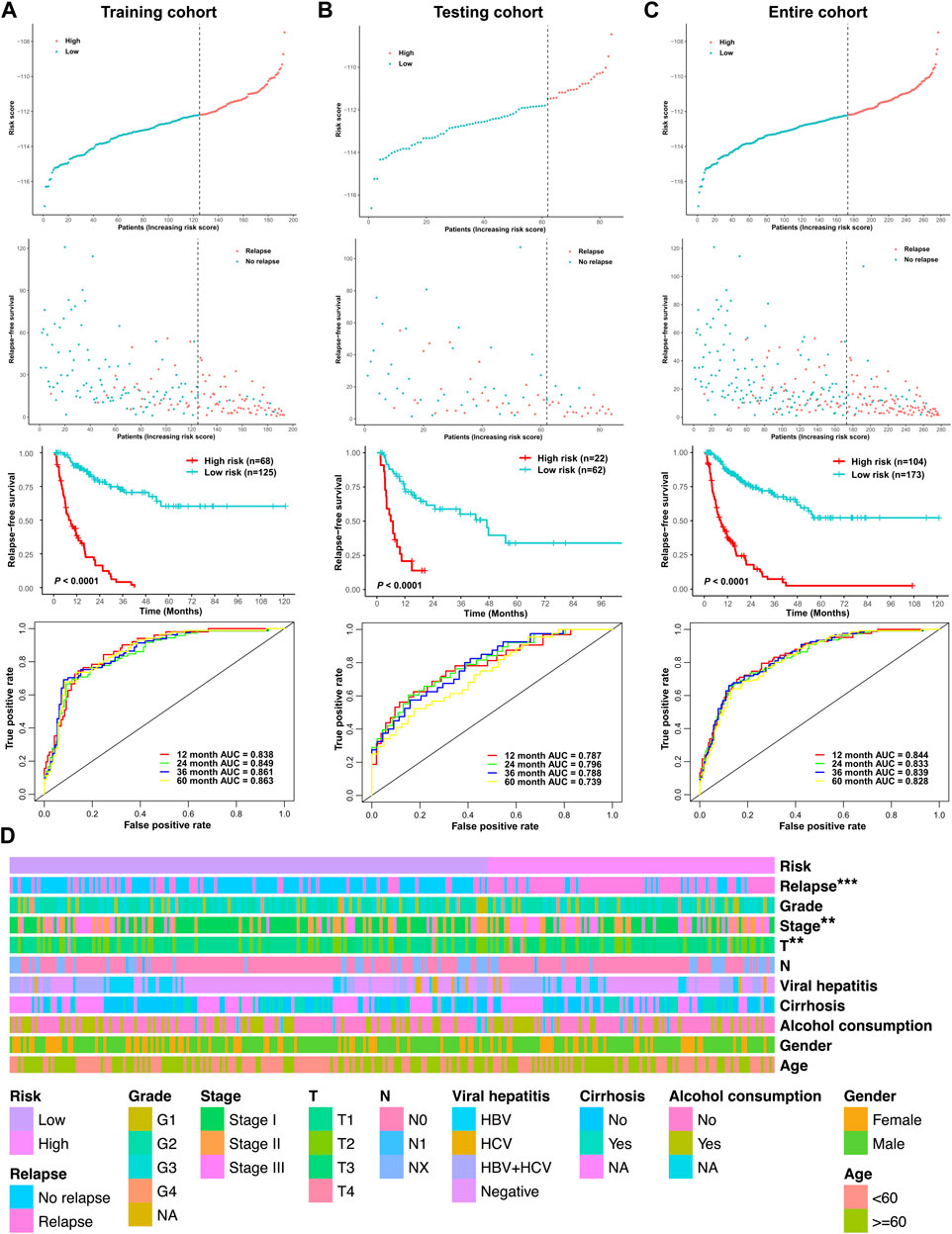
FIGURE 6. Assessment of the efficacy of the signature in predicting tumor relapse in HCC. (A) Efficacy of the relapse predictive signature in the training cohort. (B) Efficacy of the relapse predictive signature in the testing cohort. (C) Efficacy of the relapse predictive signature in the entire cohort. The upper panel depicted the risk score analysis, the middle panel depicted the Kaplan–Meier survival analysis, and the lower panel depicted the ROC curve analysis. (D) Heatmap of the relationship between the risk score and the clinicopathological features in the entire cohort. **p < 0.01 and ***p < 0.001.
To further investigate the prognostic significance of the relapse predictive signature in HCC, subgroup analysis was conducted via stratifying the entire cohort into different subgroups according to age (< 60 and ≥ 60), gender (male and female), T stage (T1 + T2 and T3 + T4), and TNM stage (stage I + stage II and stage III). The results of Kaplan–Meier survival analysis and ROC curve analysis showed that the relapse predictive signature was stable and had a great performance in different subgroups (Figures 7A–D).
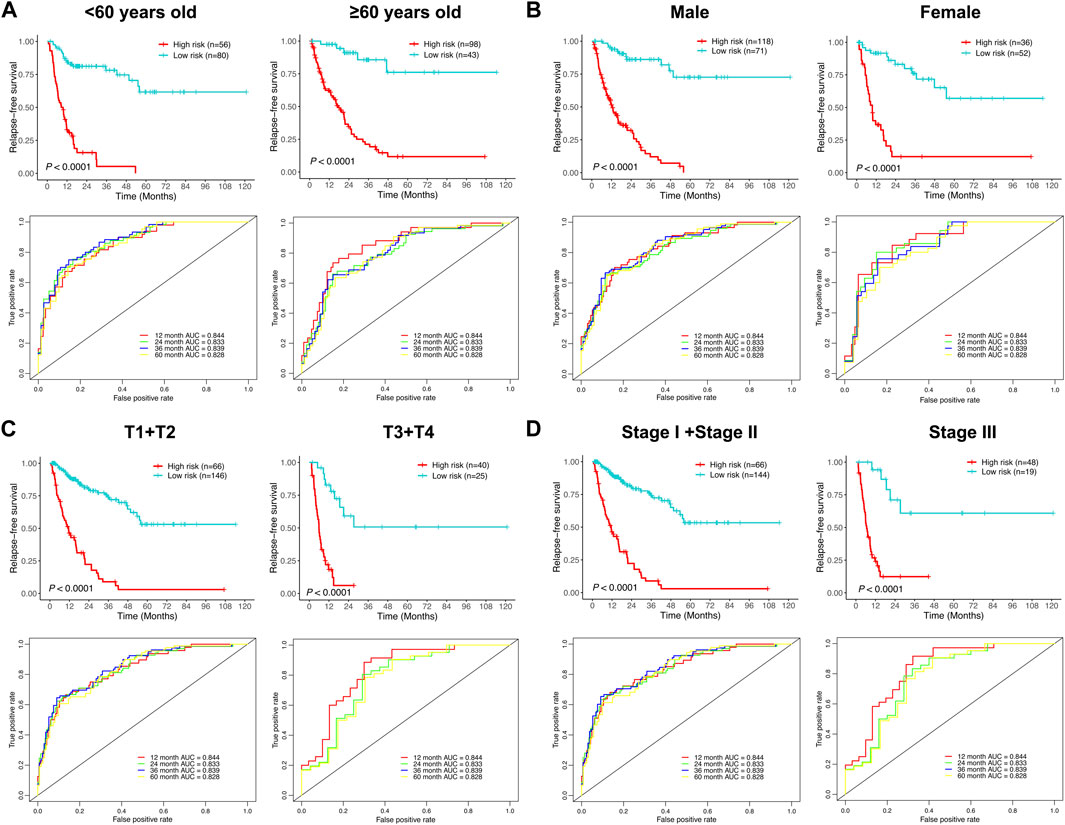
FIGURE 7. Assessment of the efficacy of the signature in diverse subgroups with different clinicopathological features. (A) Age (< 60 and ≥ 60). (B) Gender (male and female). (C) T stage (T1 + T2 and T3 + T4). (D) TNM stage (stage I + stage II and stage III). The upper panel depicted the Kaplan–Meier survival analysis, and the lower panel depicted the ROC curve analysis.
Functional enrichment analysis and immune activity analysis based on the risk mode
Due to the different tumor relapse patterns of HCC patients in the low-risk group and high-risk group, differentially expressed genes between the two risk subgroups were explored and subjected to KEGG functional enrichment analysis. The KEGG pathway analysis revealed that several immune-related pathways were significantly enriched, such as graft-versus-host disease, intestinal immune network for IgA production, primary immunodeficiency, allograft rejection, antigen processing and presentation, B-cell receptor signaling pathway, viral protein interaction with cytokine and cytokine receptor, cell adhesion molecules, and human T-cell leukemia virus 1 infection (Figure 8A).
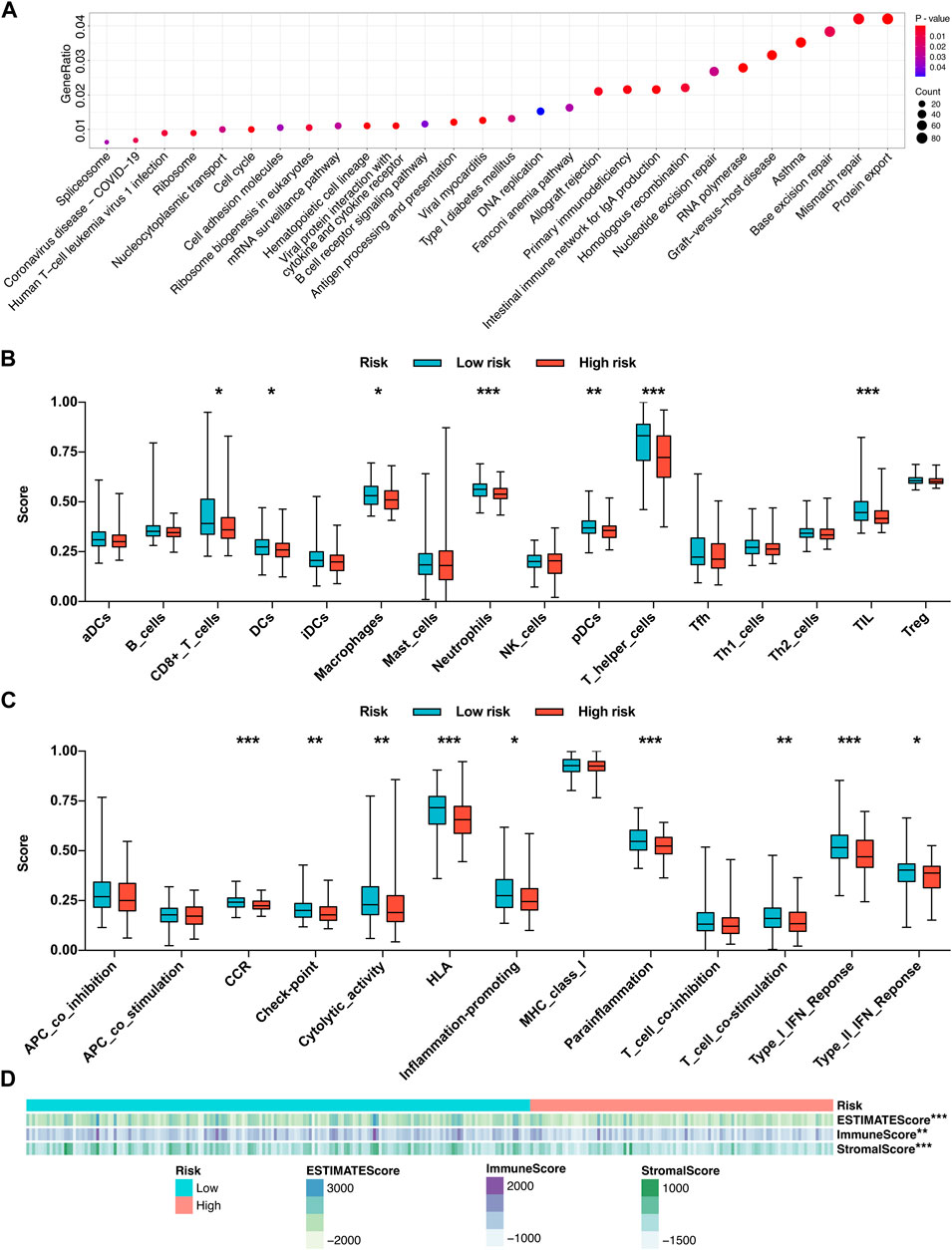
FIGURE 8. Functional enrichment analysis and immune activity analysis based on the risk mode. (A) KEGG functional enrichment analysis of the differentially expressed genes between the two risk subgroups. (B) Infiltration fractions of 16 immune cell types in the two risk subgroups. (C) Comparisons of 13 immune-related functions in the two risk subgroups. (D) Heatmap of the relationship between the risk score and the infiltration degrees of immune and stromal cells within the tumor microenvironment. *p < 0.05, **p < 0.01, and ***p < 0.001.
Driven by the results of KEGG pathway analysis, we further investigated the local immune characteristics of the two risk subgroups using ssGSEA analysis, and the enrichment scores of ssGSEA for 16 immune cells and 13 immune-related functions or pathways between the two risk subgroups were compared. The low-risk group showed higher local infiltration fractions of several immune cell types, including CD8+ T cells, dendritic cells (DCs), macrophages, neutrophils, plasmacytoid DCs (pDCs), T helper (Th) cells, and tumor-infiltrating lymphocytes (TILs) (Figure 8B). Similarly, the local immune functions of chemokine receptors (CCR), check point, cytolytic activity, human lymphocyte histocompatibility antigen (HLA), inflammation-promoting, parainflammation, T cell co-stimulation, type I interferon (IFN) response, type II IFN response were also more activated in the low-risk group (Figure 8C). Moreover, the ESTIMATE algorithm was applied to evaluate the infiltration degrees of immune and stromal cells within the tumor microenvironment. The results revealed that the low-risk group exhibited significantly higher ESTIMATE score, immune score, and stromal score, which further confirmed the ssGSEA results (Figure 8D). Conclusively, those findings suggested that the local immune activity within the HCC microenvironment, which may benefit the antitumor effects, is more activated in the low-risk group.
Molecular subtype clusters associated with tumor relapse and local immune status
Unsupervised consensus clustering was further conducted for all the stage I–III HCC patients, based on the AS events involved in the relapse predictive signature. Elbow and Gap analysis showed that the optimal number of clusters was four groups (Figures 9A,B). Then, the four clusters were defined by the distribution of the consensus value of each sample, which was classified as follows: cluster 1 (n = 89, 32.1%), cluster 2 (n = 44, 15.9%), cluster 3 (n = 71, 25.6%), and cluster 4 (n = 73, 26.4%) (Figure 9C). The Kaplan–Meier curve was plotted to evaluate the association between clustering and RFS. The results revealed that different molecular subtype clusters were related with diverse RFS patterns as shown in Figure 9D. Cluster 2 had the best RFS of the four clusters followed by cluster 3, while cluster 1 and cluster 4 showed relatively poor outcomes in the RFS analysis. Finally, the association of the four molecular subtype clusters and the local immune infiltration status and clinicopathological features was illustrated as a heatmap, and significant correlations were identified between the molecular subtype cluster and tumor relapse, ESTIMATE score, and immune score (Figure 9E). Collectively, these data clearly indicated that the molecular subtype clustering based on the relapse predictive signature has a good performance in distinguishing the different relapse patterns and local immune infiltration status between diverse clusters.
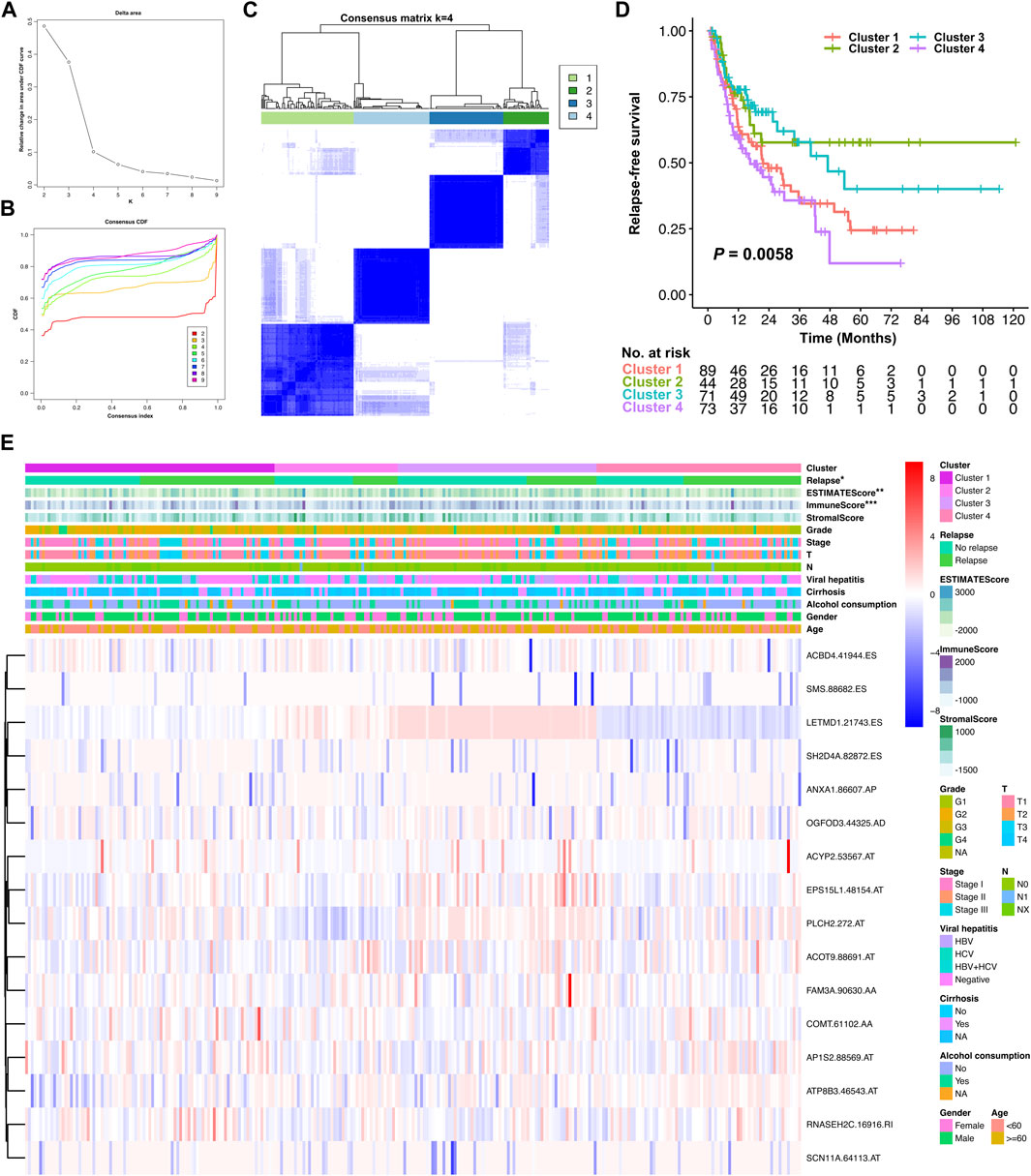
FIGURE 9. Identification of relapse-associated AS clusters related to clinical outcomes and molecular subtypes. (A) Elbow analysis for identifying the optimal number of clusters. (B) Gap analysis for identifying the optimal number of clusters. (C) Consensus clustering matrix depicting the four clusters of the patients. (D) Kaplan–Meier survival analysis of the four clusters of HCC patients. (E) Heatmap of the relationship of the four molecular subtype clusters and the local immune infiltration status, clinicopathological characteristics. *p < 0.05, **p < 0.01, and ***p < 0.001.
Discussion
Despite the great progress in surveillance and therapeutic strategies improving overall survival, the clinical outcomes of HCC remain dismal due to high frequency of tumor relapse, even after curative surgery (Bosch et al., 2004; Rahbari et al., 2011). With HCC relapse, the therapeutic options are limited and the treatment response is usually poor due to the aggressive pathological characteristics, which certainly leads to poor prognosis. Early precise warning and detection of HCC with a high risk of tumor relapse may be the optimal maneuver to settle this issue. Until now, the TNM staging system of the American Joint Committee on Cancer (AJCC), together with other prognostic staging systems (BCLC, CLIP, and JIS classification of HCC), is most commonly used to assess the prognosis of HCC (Pons et al., 2005; Amin et al., 2017). However, these prognostic staging systems are mostly focused on clinical features but ignore the genetic and epigenetic dysregulations in the process of HCC development and progression, which always makes them not sufficient for efficiently predicting tumor relapse or prognosis of HCC. Development of robust signatures based on the molecular biological process in HCC to predict tumor relapse would be probable to supplement the current prognostic staging systems and guide the therapeutic strategies after surgery, further improving the prognosis of HCC patients. AS is an important biological process and has been demonstrated to play a critical role in the genetic central dogma (Nilsen and Graveley, 2010; Kozlovski et al., 2017). Dysregulation of AS is closely inclined to tumor development, immune escape, drug resistance, and progression (Sciarrillo et al., 2020; Zhang et al., 2021a; Su and Huang, 2021). However, there is little literature dissecting the mechanism of AS in tumor relapse of HCC, and a comprehensive depiction of the relapse-associated AS events and robust AS signatures predicting tumor relapse in HCC remains lacking.
In the present study, we focused on stage I–III HCC received curative resection and comprehensively analyzed the relapse-associated AS events in these cases. In total, 2441 ASs derived from 1634 mRNA were identified as relapse-associated AS events. ES was determined as the most common AS event among the seven AS subtypes, implying its critical role in the tumor relapse of HCC. In the PPI network based on the relevant proteins of these AS events, 34 clusters including 1573 proteins with 11590 interactions were identified. The complicacy of the PPI network suggested that the tumor relapse of HCC is not driven by one or two AS events but by an integrated network. We further surveyed the ten hub proteins in the cluster with the highest score and found most of the hub proteins has been demonstrated to figure prominently in the development and progression of various tumor types. The top one hub protein AKT1, as one isoform of protein kinase B (PKB or AKT), has been reported to be involved in many tumor growth-related biology processes such as cell proliferation, apoptosis, growth, metabolism, and tumor angiogenesis and inflammatory cell infiltration by regulating mTOR, GSK3, BAD, p27KIP1, FoxO, and MDM2 signaling (Somanath et al., 2009; Hers et al., 2011; Fruman and Rommel, 2014; Mundi et al., 2016). Other two high-ranking hub proteins (HNRNPA1 and VEGFA) were also proved to play a vital role in tumor cell biology. HNRNPA1 has been reported overexpressed in many malignancies such as lung cancer, myeloma, leukemia, and Burkitt lymphoma (Roy et al., 2017). It accelerates cell cycle progression and aerobic glycolysis by activating telomerase to promote tumor growth, controls the anti-apoptotic signaling to enhance tumor maintenance and drug resistance, and advances the metastatic dissemination of cancer cells, all of which make HNRNPA1 promote various stages of cancer progression (Ting et al., 2009; Ko et al., 2014; Yu et al., 2015). VEGFA signaling played a crucial role in the progression of angiogenesis-related diseases, particularly in cancers; agents blocking VEGFA have been reported that could effectively inhibit tumor growth and metastatic spread (Claesson-Welsh and Welsh, 2013). The middle-ranking hub protein RHOA was well known as a signal mediator associated with multiple biological events such as cell polarity, cell morphology phenotypes, and migration, all of which are essential for progression of diverse malignancies (Nam et al., 2019). Another middle-ranking hub protein, RPS3, has been demonstrated to facilitate hepatocarcinogenesis through posttranscriptionally regulating SIRT1 (Zhao et al., 2019a). Moreover, hub protein MDM2, as E3 ubiquitin ligase, modulated tumor development and progression by forming an autoregulatory feedback loop with p53, which resulted in increased ubiquitin-mediated degradation of p53 (Konopleva et al., 2020). Other hub proteins SRSF1 and PTBP1 were considered splicing factors involved in cell proliferation, cell cycle progression, apoptosis, invasion, and migration, dysregulated expression of which has been validated to be associated with tumorigenesis and diminished immune response (Paz et al., 2021; Taniguchi et al., 2021). These previous research studies also validated the accuracy and convincement of our investigation. Similarly, in the regulatory splicing network, many AS events and SFs were associated with tumor cell biology. PCBP1, with the most interaction with the relapse-associated AS events in the core SFs, was identified as an intracellular immune checkpoint for maintaining the functions of effector T cells in tumor immunity and has been reported to inhibit HCC cell invasion by regulating the alternative splicing of CD44 (Zhang et al., 2010; Ansa-Addo et al., 2020). RBM25 acts as a tumor suppressor and regulator of MYC activity by controlling the splicing of the MYC inhibitor BIN1 (Ge et al., 2019). TIA1 and RBFOX2 were reportedly associated with tumor relapse or metastasis in malignancies (Hong et al., 2020; Mochizuki et al., 2021). Among the hub relapse-associated AS events in the splicing network, two AS events derived from one mRNA (ARAF-88922-AT and ARAF-88921-AT) were noted due to the most significance in the analysis. ARAF, as one isoform of the RAF family of kinases, has been proved to have an obligatory role in promoting MAPK activity and cell migration in a cell type-dependent manner, and ARAF mutations were identified in various tumor types which associated with resistance to RAF inhibitors (Mooz et al., 2014; ARAF Mutations Limit Response to RAF Dimer Inhibition, 2021). The results suggested that the dysregulated AS events of ARAF regulated by SFs may become a novel part of the mechanisms of tumor relapse in HCC, which needs further experimental investigation.
Subsequently, univariate Cox regression analysis was further performed, which identified 496 AS events derived from 377 mRNA to be prognostically significant. Based on the results, LASSO and multivariate Cox regression analysis were performed with tough screening standards and finally established a robust 16-gene AS signature for predicting tumor relapse in stage I–III HCC. According to the survival analysis, this novel relapse predictive AS signature displayed good performance in distinguishing the low-risk and high-risk groups in all of the training cohort, testing cohort, and entire cohort. The AUC values of different ROC curves at 1, 2, 3, and 5 year after surgery were all considerable in all the three cohorts, which indicated the great efficiency of the signature. Moreover, the results of subgroup analysis suggested that the relapse predictive signature was stable and has great performance in different conditions. After exploring the differentially expressed genes between the low- and high-risk groups, we found that these genes were significantly associated with immune-related pathways. Based on those results, the AS events involved in the signature may have important roles in tumor progression and regulate the immune microenvironment in HCC. The relevant genes of several AS events in this signature have been reported to play similar roles in human cancers by previous studies. For example, ACYP2 was reported to contribute to the malignant progression of glioma by promoting Ca2+ efflux and the subsequent activation of c-Myc and STAT3 signals (Li et al., 2020). Moreover, ACYP2 gene polymorphism was associated with the risk of cirrhosis developing into liver cancer, and high ACYP2 expression was associated with better overall survival in HCC, which indicated its important role in HCC progression (Zhao et al., 2019b). ANXA1 was highlighted as a biomarker in oncology, and manipulation of ANX1 in cancers can influence the metastatic behavior of the tumor cells by modulating inflammation, immune response, and angiogenesis (Delorme et al., 2021). COMT was considered a tumor suppressor that is associated with anticarcinogenesis, antiproliferation, pro-apoptosis, anti-angiogenesis, and anti-inflammation (Bastos et al., 2017). RNASEH2C was reported as a metastasis susceptibility gene and modulator of T cell-mediated immune response in breast cancer (Deasy et al., 2019). SH2D4A was identified as a chromosome 8p tumor suppressor and positively correlated with effector and regulatory T cell infiltration by blocking IL-6 signaling in HCC, which implies its crucial role in HCC (Ploeger et al., 2016). Furthermore, our ssGSEA analysis demonstrated that the local infiltration fractions of several important immune cells, including CD8 + T cells, DCs, macrophages, neutrophils, pDCs, Th cells, and TILs, were significantly higher in the lower-risk group than in the high-risk group. Similarly, the local immune functions of CCR, checkpoint, cytolytic activity, HLA, inflammation-promoting, parainflammation, T cell co-stimulation, type I IFN response, and type II IFN response were also more activated in the low-risk group than in the high-risk group. Moreover, ESTIMATE analysis revealed that the low-risk group exhibited significantly higher ESTIMATE score, immune score, and stromal score, which further confirmed the ssGSEA results. Finally, unsupervised consensus clustering was further conducted based on the AS events involved in the relapse predictive signature, and the entire cohort of the included stage I–III HCC patients was divided into four molecular subtype clusters. The molecular subtype clustering exhibited good performance in distinguishing the different relapse patterns and local immune infiltration status between diverse clusters, which further verified the power of the signature.
Conclusion
In summary, our study first provides an overview of the relapse-associated AS events in stage I–III HCC and further constructed a novel AS signature that robustly stratifies tumor relapse risk, indicates immune activity, and facilitates identifying molecular subtypes in stage I–III HCC. The results of the study facilitate the deep mining of the mechanisms associated with tumor relapse and tumor immunity and the development of novel individualized treatment targets for HCC.
Data availability statement
The datasets presented in this study can be found in online repositories. The names of the repository/repositories and accession number(s) can be found in the article/Supplementary Material.
Ethics statement
Ethical review and approval was not required for the study on human participants in accordance with the local legislation and institutional requirements. Written informed consent for participation was not required for this study in accordance with the national legislation and the institutional requirements.
Author contributions
Conception and design: X-XC, DM, and Y-JC. Development of methodology: X-XC, B-HZ, Y-CL, and DM. Acquisition of data: X-XC, B-HZ, Y-CL, Z-QL, and C-YC. Analysis and interpretation of data: X-XC, Z-QL, C-YC, and Y-CY. Writing, review, and/or revision of the manuscript: X-XC, DM, and Y-JC. Study supervision: X-XC, DM, and Y-JC.
Funding
This study was supported by the National Natural Science Foundation of China (81902961 and 82102464) and the Sailing Program of Shanghai Science and Technology Commission (19YF1407400).
Acknowledgments
The authors thank the staff working in TCGA for data managing and software developing. The authors also acknowledge Sheng Chen, Jun-Qing Wang, Ye Lu, Xiao-Yong Gong, Jia-Jun Ren, Feng Ye, Feng-Jie Hao, and Qiang Cai for their valuable advices and great supports in this study.
Conflict of interest
The authors declare that the research was conducted in the absence of any commercial or financial relationships that could be construed as a potential conflict of interest.
Publisher’s note
All claims expressed in this article are solely those of the authors and do not necessarily represent those of their affiliated organizations, or those of the publisher, the editors, and the reviewers. Any product that may be evaluated in this article, or claim that may be made by its manufacturer, is not guaranteed or endorsed by the publisher.
Supplementary material
The Supplementary Material for this article can be found online at: https://www.frontiersin.org/articles/10.3389/fphar.2022.939912/full#supplementary-material
SUPPLEMENTARY TABLE S1 | Information of the PPI network.
SUPPLEMENTARY TABLE S2 | Information of SF–AS correlation network.
SUPPLEMENTARY FIGURE S1 | Functional enrichment analysis of the relevant genes of the relapse-associated AS events. Top ten significant enriched terms in the three GO categories (BP, CC, and MF) were shown. Abbreviations: BP, biological processes; CC, cellular components; GO, Gene Ontology; MF, molecular functions.
SUPPLEMENTARY FIGURE S2 | Forest plots depicting the results of Cox regression analysis. (A) Univariate Cox regression analysis; (B) multivariate Cox regression analysis.
References
Amin, M. B., Greene, F. L., Edge, S. B., Compton, C. C., Gershenwald, J. E., Brookland, R. K., et al. (2017). The Eighth Edition AJCC Cancer Staging Manual: Continuing to build a bridge from a population-based to a more "personalized" approach to cancer staging. Ca. Cancer J. Clin. 67, 93–99. doi:10.3322/caac.21388
Ansa-Addo, E. A., Huang, H. C., Riesenberg, B., Iamsawat, S., Borucki, D., Nelson, M. H., et al. (2020). RNA binding protein PCBP1 is an intracellular immune checkpoint for shaping T cell responses in cancer immunity. Sci. Adv. 6, eaaz3865. doi:10.1126/sciadv.aaz3865
ARAF Mutations Limit Response to RAF Dimer Inhibition (2021). ARAF mutations Limit response to RAF dimer inhibition. Cancer Discov. 11, 1610. doi:10.1158/2159-8290.CD-RW2021-069
Bastos, P., Gomes, T., and Ribeiro, L. (2017). Catechol-O-Methyltransferase (COMT): An update on its role in cancer, neurological and cardiovascular diseases. Rev. Physiol. Biochem. Pharmacol. 173, 1–39. doi:10.1007/112_2017_2
Bosch, F. X., Ribes, J., Diaz, M., and Cleries, R. (2004). Primary liver cancer: Worldwide incidence and trends. Gastroenterology 127, S5–S16. doi:10.1053/j.gastro.2004.09.011
Cai, Y., Xia, J., Wang, N., and Zhou, H. (2020). Identification of prognostic alternative splicing signatures in Hepatitis B or/and C viruses related hepatocellular carcinoma. Genomics 112, 3396–3406. doi:10.1016/j.ygeno.2020.06.002
Claesson-Welsh, L., and Welsh, M. (2013). VEGFA and tumour angiogenesis. J. Intern. Med. 273, 114–127. doi:10.1111/joim.12019
Climente-Gonzalez, H., Porta-Pardo, E., Godzik, A., and Eyras, E. (2017). The functional impact of alternative splicing in cancer. Cell. Rep. 20, 2215–2226. doi:10.1016/j.celrep.2017.08.012
Deasy, S. K., Uehara, R., Vodnala, S. K., Yang, H. H., Dass, R. A., Hu, Y., et al. (2019). Aicardi-Goutieres syndrome gene Rnaseh2c is a metastasis susceptibility gene in breast cancer. PLoS Genet. 15, e1008020. doi:10.1371/journal.pgen.1008020
Delorme, S., Privat, M., Sonnier, N., Rouanet, J., Witkowski, T., Kossai, M., et al. (2021). New insight into the role of ANXA1 in melanoma progression: Involvement of stromal expression in dissemination. Am. J. Cancer Res. 11, 1600–1615.
European Association for the Study of the Liver (2018). EASL clinical practice guidelines: Management of hepatocellular carcinoma. J. Hepatol. 69, 182–236. doi:10.1016/j.jhep.2018.03.019
Fruman, D. A., and Rommel, C. (2014). PI3K and cancer: Lessons, challenges and opportunities. Nat. Rev. Drug Discov. 13, 140–156. doi:10.1038/nrd4204
Ge, Y., Schuster, M. B., Pundhir, S., Rapin, N., Bagger, F. O., Sidiropoulos, N., et al. (2019). The splicing factor RBM25 controls MYC activity in acute myeloid leukemia. Nat. Commun. 10, 172. doi:10.1038/s41467-018-08076-y
Giulietti, M., Piva, F., D'Antonio, M., D'Onorio De Meo, P., Paoletti, D., Castrignano, T., et al. (2013). SpliceAid-F: A database of human splicing factors and their RNA-binding sites. Nucleic Acids Res. 41, D125–D131. doi:10.1093/nar/gks997
Goldman, M. J., Craft, B., Hastie, M., Repecka, K., McDade, F., Kamath, A., et al. (2020). Visualizing and interpreting cancer genomics data via the Xena platform. Nat. Biotechnol. 38, 675–678. doi:10.1038/s41587-020-0546-8
Hers, I., Vincent, E. E., and Tavare, J. M. (2011). Akt signalling in health and disease. Cell. Signal. 23, 1515–1527. doi:10.1016/j.cellsig.2011.05.004
Hong, W., Hu, Y., Fan, Z., Gao, R., Yang, R., Bi, J., et al. (2020). In silico identification of EP400 and TIA1 as critical transcription factors involved in human hepatocellular carcinoma relapse. Oncol. Lett. 19, 952–964. doi:10.3892/ol.2019.11171
Hutter, C., and Zenklusen, J. C. (2018). The cancer genome Atlas: Creating lasting value beyond its data. Cell. 173, 283–285. doi:10.1016/j.cell.2018.03.042
Ko, C. C., Chen, Y. J., Chen, C. T., Liu, Y. C., Cheng, F. C., Hsu, K. C., et al. (2014). Chemical proteomics identifies heterogeneous nuclear ribonucleoprotein (hnRNP) A1 as the molecular target of quercetin in its anti-cancer effects in PC-3 cells. J. Biol. Chem. 289, 22078–22089. doi:10.1074/jbc.M114.553248
Konopleva, M., Martinelli, G., Daver, N., Papayannidis, C., Wei, A., Higgins, B., et al. (2020). MDM2 inhibition: An important step forward in cancer therapy. Leukemia 34, 2858–2874. doi:10.1038/s41375-020-0949-z
Kozlovski, I., Siegfried, Z., Amar-Schwartz, A., and Karni, R. (2017). The role of RNA alternative splicing in regulating cancer metabolism. Hum. Genet. 136, 1113–1127. doi:10.1007/s00439-017-1803-x
Li, M., Ruan, B., Wei, J., Yang, Q., Chen, M., Ji, M., et al. (2020). ACYP2 contributes to malignant progression of glioma through promoting Ca(2+) efflux and subsequently activating c-Myc and STAT3 signals. J. Exp. Clin. Cancer Res. 39, 106. doi:10.1186/s13046-020-01607-w
Liu, Z., Lu, T., Li, J., Wang, L., Xu, K., Dang, Q., et al. (2021a). Development and clinical validation of a novel six-gene signature for accurately predicting the recurrence risk of patients with stage II/III colorectal cancer. Cancer Cell. Int. 21, 359. doi:10.1186/s12935-021-02070-z
Liu, Z., Weng, S., Xu, H., Wang, L., Liu, L., Zhang, Y., et al. (2021b). Computational recognition and clinical verification of TGF-beta-derived miRNA signature with potential implications in prognosis and immunotherapy of intrahepatic cholangiocarcinoma. Front. Oncol. 11, 757919. doi:10.3389/fonc.2021.757919
Mochizuki, Y., Funayama, R., Shirota, M., Kikukawa, Y., Ohira, M., Karasawa, H., et al. (2021). Alternative microexon splicing by RBFOX2 and PTBP1 is associated with metastasis in colorectal cancer. Int. J. Cancer 149, 1787–1800. doi:10.1002/ijc.33758
Mooz, J., Oberoi-Khanuja, T. K., Harms, G. S., Wang, W., Jaiswal, B. S., Seshagiri, S., et al. (2014). Dimerization of the kinase ARAF promotes MAPK pathway activation and cell migration. Sci. Signal. 7, ra73. doi:10.1126/scisignal.2005484
Mundi, P. S., Sachdev, J., McCourt, C., and Kalinsky, K. (2016). AKT in cancer: New molecular insights and advances in drug development. Br. J. Clin. Pharmacol. 82, 943–956. doi:10.1111/bcp.13021
Nam, S., Kim, J. H., and Lee, D. H. (2019). RHOA in gastric cancer: Functional roles and therapeutic potential. Front. Genet. 10, 438. doi:10.3389/fgene.2019.00438
Nilsen, T. W., and Graveley, B. R. (2010). Expansion of the eukaryotic proteome by alternative splicing. Nature 463, 457–463. doi:10.1038/nature08909
Paz, S., Ritchie, A., Mauer, C., and Caputi, M. (2021). The RNA binding protein SRSF1 is a master switch of gene expression and regulation in the immune system. Cytokine Growth Factor Rev. 57, 19–26. doi:10.1016/j.cytogfr.2020.10.008
Ploeger, C., Waldburger, N., Fraas, A., Goeppert, B., Pusch, S., Breuhahn, K., et al. (2016). Chromosome 8p tumor suppressor genes SH2D4A and SORBS3 cooperate to inhibit interleukin-6 signaling in hepatocellular carcinoma. Hepatology 64, 828–842. doi:10.1002/hep.28684
Pons, F., Varela, M., and Llovet, J. M. (2005). Staging systems in hepatocellular carcinoma. HPB Oxf. 7, 35–41. doi:10.1080/13651820410024058
Rahbari, N. N., Mehrabi, A., Mollberg, N. M., Muller, S. A., Koch, M., Buchler, M. W., et al. (2011). Hepatocellular carcinoma: Current management and perspectives for the future. Ann. Surg. 253, 453–469. doi:10.1097/SLA.0b013e31820d944f
Roy, R., Huang, Y., Seckl, M. J., and Pardo, O. E. (2017). Emerging roles of hnRNPA1 in modulating malignant transformation. WIREs RNA 8, e1431. doi:10.1002/wrna.1431
Ryan, M., Wong, W. C., Brown, R., Akbani, R., Su, X., Broom, B., et al. (2016). TCGASpliceSeq a compendium of alternative mRNA splicing in cancer. Nucleic Acids Res. 44, D1018–D1022. doi:10.1093/nar/gkv1288
Salton, M., Kasprzak, W. K., Voss, T., Shapiro, B. A., Poulikakos, P. I., and Misteli, T. (2015). Inhibition of vemurafenib-resistant melanoma by interference with pre-mRNA splicing. Nat. Commun. 6, 7103. doi:10.1038/ncomms8103
Sciarrillo, R., Wojtuszkiewicz, A., Assaraf, Y. G., Jansen, G., Kaspers, G. J. L., Giovannetti, E., et al. (2020). The role of alternative splicing in cancer: From oncogenesis to drug resistance. Drug resist. updat. 53, 100728. doi:10.1016/j.drup.2020.100728
Singal, A. G., Lampertico, P., and Nahon, P. (2020). Epidemiology and surveillance for hepatocellular carcinoma: New trends. J. Hepatol. 72, 250–261. doi:10.1016/j.jhep.2019.08.025
Somanath, P. R., Vijai, J., Kichina, J. V., Byzova, T., and Kandel, E. S. (2009). The role of PAK-1 in activation of MAP kinase cascade and oncogenic transformation by Akt. Oncogene 28, 2365–2369. doi:10.1038/onc.2009.114
Song, H., Sun, N., Lin, L., Wei, S., Zeng, K., Liu, W., et al. (2020). Splicing factor PRPF6 upregulates oncogenic androgen receptor signaling pathway in hepatocellular carcinoma. Cancer Sci. 111, 3665–3678. doi:10.1111/cas.14595
Su, Z., and Huang, D. (2021). Alternative splicing of pre-mRNA in the control of immune activity. Genes (Basel) 12, 574. doi:10.3390/genes12040574
Sung, H., Ferlay, J., Siegel, R. L., Laversanne, M., Soerjomataram, I., Jemal, A., et al. (2021). Global cancer statistics 2020: GLOBOCAN estimates of incidence and mortality worldwide for 36 cancers in 185 countries. Ca. Cancer J. Clin. 71, 209–249. doi:10.3322/caac.21660
Szklarczyk, D., Gable, A. L., Lyon, D., Junge, A., Wyder, S., Huerta-Cepas, J., et al. (2019). STRING v11: Protein-protein association networks with increased coverage, supporting functional discovery in genome-wide experimental datasets. Nucleic Acids Res. 47, D607–D613. doi:10.1093/nar/gky1131
Taniguchi, K., Uchiyama, K., and Akao, Y. (2021). PTBP1-targeting microRNAs regulate cancer-specific energy metabolism through the modulation of PKM1/M2 splicing. Cancer Sci. 112, 41–50. doi:10.1111/cas.14694
Ting, N. S., Pohorelic, B., Yu, Y., Lees-Miller, S. P., and Beattie, T. L. (2009). The human telomerase RNA component, hTR, activates the DNA-dependent protein kinase to phosphorylate heterogeneous nuclear ribonucleoprotein A1. Nucleic Acids Res. 37, 6105–6115. doi:10.1093/nar/gkp636
Urbanski, L. M., Leclair, N., and Anczukow, O. (2018). Alternative-splicing defects in cancer: Splicing regulators and their downstream targets, guiding the way to novel cancer therapeutics. Wiley Interdiscip. Rev. RNA 9, e1476. doi:10.1002/wrna.1476
Wang, H., Zhang, C. Z., Lu, S. X., Zhang, M. F., Liu, L. L., Luo, R. Z., et al. (2019). A coiled-coil domain containing 50 splice variant is modulated by serine/arginine-rich splicing factor 3 and promotes hepatocellular carcinoma in mice by the ras signaling pathway. Hepatology 69, 179–195. doi:10.1002/hep.30147
Wang, L., Liu, Z., Zhu, R., Liang, R., Wang, W., Li, J., et al. (2022). Multi-omics landscape and clinical significance of a SMAD4-driven immune signature: Implications for risk stratification and frontline therapies in pancreatic cancer. Comput. Struct. Biotechnol. J. 20, 1154–1167. doi:10.1016/j.csbj.2022.02.031
Wu, F., Chen, Q., Liu, C., Duan, X., Hu, J., Liu, J., et al. (2020). Profiles of prognostic alternative splicing signature in hepatocellular carcinoma. Cancer Med. 9, 2171–2180. doi:10.1002/cam4.2875
Yu, C., Guo, J., Liu, Y., Jia, J., Jia, R., and Fan, M. (2015). Oral squamous cancer cell exploits hnRNP A1 to regulate cell cycle and proliferation. J. Cell. Physiol. 230, 2252–2261. doi:10.1002/jcp.24956
Zhang, T., Huang, X. H., Dong, L., Hu, D., Ge, C., Zhan, Y. Q., et al. (2010). PCBP-1 regulates alternative splicing of the CD44 gene and inhibits invasion in human hepatoma cell line HepG2 cells. Mol. Cancer 9, 72. doi:10.1186/1476-4598-9-72
Zhang, Y., Liu, Z., Li, X., Liu, L., Wang, L., Han, X., et al. (2021a). Comprehensive molecular analyses of a six-gene signature for predicting late recurrence of hepatocellular carcinoma. Front. Oncol. 11, 732447. doi:10.3389/fonc.2021.732447
Zhang, Y., Qian, J., Gu, C., and Yang, Y. (2021b). Alternative splicing and cancer: A systematic review. Signal Transduct. Target. Ther. 6, 78. doi:10.1038/s41392-021-00486-7
Zhao, L., Cao, J., Hu, K., Wang, P., Li, G., He, X., et al. (2019a). RNA-binding protein RPS3 contributes to hepatocarcinogenesis by post-transcriptionally up-regulating SIRT1. Nucleic Acids Res. 47, 2011–2028. doi:10.1093/nar/gky1209
Zhao, W., Liu, X., Yu, Z., Xiong, Z., Wu, J., Sun, Y., et al. (2019b). Associations between polymorphisms of the ACYP2 gene and liver cancer risk: A case-control study and meta-analysis. Mol. Genet. Genomic Med. 7, e00716. doi:10.1002/mgg3.716
Zheng, J., Kuk, D., Gonen, M., Balachandran, V. P., Kingham, T. P., Allen, P. J., et al. (2017). Actual 10-year survivors after resection of hepatocellular carcinoma. Ann. Surg. Oncol. 24, 1358–1366. doi:10.1245/s10434-016-5713-2
Zhu, G. Q., Zhou, Y. J., Qiu, L. X., Wang, B., Yang, Y., Liao, W. T., et al. (2019). Prognostic alternative mRNA splicing signature in hepatocellular carcinoma: A study based on large-scale sequencing data. Carcinogenesis 40, 1077–1085. doi:10.1093/carcin/bgz073
Glossary
HCC Hepatocellular carcinoma
AS Alternative splicing
SFs Splicing factors
TCGA The Cancer Genome Atlas
LIHC Liver hepatocellular carcinoma
UCSC University of California Santa Cruz
AA Alternate acceptor site
AD Alternate donor site
AP Alternate promoter
AT Alternate terminator
ES Exon skip
ME Mutually exclusive exons
RI Retained intron
PSI Percent-spliced-in
SF Splicing factor
RFS Relapse-free survival
PSM Propensity score matching
GO Gene Ontology
BP Biological processes
CC Cellular components
MF Molecular functions
PPI Protein–protein interaction
cor Correlation coefficient
ROC Receiver operating characteristic
KEGG Kyoto Encyclopedia of Genes and Genomes
ssGSEA Single-sample gene set enrichment analysis
DCs Dendritic cells
pDCs Plasmacytoid DCs
Th T helper
TILs Tumor-infiltrating lymphocytes
CCR Chemokine receptors
HLA Human lymphocyte histocompatibility antigen
IFN Interferon
AJCC American Joint Committee on Cancer
PKB Protein kinase B
Keywords: hepatocellular carcinoma, alternative splicing, tumor relapse, prognosis, immunity
Citation: Chen X-X, Zhang B-H, Lu Y-C, Li Z-Q, Chen C-Y, Yang Y-C, Chen Y-J and Ma D (2022) A novel 16-gene alternative mRNA splicing signature predicts tumor relapse and indicates immune activity in stage I–III hepatocellular carcinoma. Front. Pharmacol. 13:939912. doi: 10.3389/fphar.2022.939912
Received: 09 May 2022; Accepted: 28 July 2022;
Published: 06 September 2022.
Edited by:
Yan Liu, Grossman School of Medicine, New York University, United StatesReviewed by:
Fei Li, Anhui Medical University, ChinaLibo Wang, First Affiliated Hospital of Zhengzhou University, China
Copyright © 2022 Chen, Zhang, Lu, Li, Chen, Yang, Chen and Ma. This is an open-access article distributed under the terms of the Creative Commons Attribution License (CC BY). The use, distribution or reproduction in other forums is permitted, provided the original author(s) and the copyright owner(s) are credited and that the original publication in this journal is cited, in accordance with accepted academic practice. No use, distribution or reproduction is permitted which does not comply with these terms.
*Correspondence: Xu-Xiao Chen, Y2hlbnh1eGlhbzA5MDJAMTYzLmNvbQ==; Yong-Jun Chen, c3dha2NoZW55akBob3RtYWlsLmNvbQ==; Di Ma, bWQxMTIwN0AxNjMuY29t.
†These authors have contributed equally to this work