- 1School of Traditional Chinese Medicine, Beijing University of Chinese Medicine, Beijing, China
- 2Dongfang Hospital Affiliated to Beijing University of Chinese Medicine, Beijing, China
- 3Guangzhou Key Laboratory of Formula-Pattern of Traditional Chinese Medicine, School of Traditional Chinese Medicine, Jinan University, Guangzhou, China
Background: Paeoniflorin (PF) represents the major bioactive constituent of the traditional Chinese medicine plant Paeonia suffruticosa (Ranunculaceae), which has a long history as a folk medicine in Asian. Paeoniflorin, a bitter pinene monoterpene glycoside, has antidepressant effects, but its potential therapeutic mechanism has not been thoroughly explored.
Methods: Experimental depression in rats was established by the chronic unpredictable mild stress (CUMS) combined with orphan method, and the efficacy of paeoniflorin on depression was evaluated by the sucrose preference test and open field test. The antidepressant mechanism of paeoniflorin was investigated by metabolomic and network pharmacology. The relevant pathways of biomarkers highlighted in metabolomics were explored, and the possible targets of paeoniflorin in the treatment of depression were further revealed through network analysis. The binding activity of paeoniflorin to key targets was verified by molecular docking.
Results: Metabolomics showed that rats with CUMS-induced depression had urine metabolic disorders, which were reversed by paeoniflorin through the regulation of metabolic pathways. Metabolites that play a key role in the function of paeoniflorin include citric acid, thiamine monophosphate, gluconolactone, 5-hydroxyindoleacetic acid and stachyose. Key predicted targets are SLC6A4, TNF, IL6 and SLC6A3. An important metabolic pathway is the Citrate cycle (TCA cycle).
Conclusion: Network integrative analysis in this study showed that paeoniflorin could improve depressive-like symptoms in model rats with CUMS-induced depression and overall correct the disordered metabolic profile through multiple metabolic pathways.
1 Introduction
Depression has become a major public health issue in the world. The World Health Organization ranks depression third top cause of global disease burden, and it is expected to rank first by 2030 (Malhi and Mann 2018). Depression is a high-risk factor for stroke, dementia, hypertension and other diseases. Studies have shown that depression persists 6 months after stroke, which not only seriously affects neurological repair in stroke patients, but also delays the recovery process, thereby increasing the risk of recurrent stroke and increasing mortality (Nickel and Thomalla 2017; Dong et al., 2021). Depression also increases the risk of dementia and Alzheimer’s disease, with potentially higher effects than other known risks (e.g., smoking, high blood sugar, high cholesterol, high homocysteine, vascular factors, etc.,) (Diniz 2018; Alexopoulos 2019; Santabarbara et al., 2019; Weisenbach et al., 2019). In addition, depression is an independent risk factor for hypertension, increasing the risk of hypertension by 42% (Meng et al., 2012; Zhang et al., 2018). Therefore, there is an urgent need to explore a more effective strategy and to find potential drug targets for depression.
Traditional Chinese medicine has been applied for depression treatment for millennia, with demonstrated efficacy, good safety profile and distinct features. Paeoniflorin (PF), a constituent of P. suffruticosa Andr., Paeonia lactiflora Pall. or Paeonia veitchii Lynch, is a monoterpene glycoside. PF possesses multiple activities such as anti-inflammatory, hypoglycemic, antithrombotic, anticonvulsant, cardioprotective and immunomodulatory effects (Xiang et al., 2020; Zhang et al., 2020; Zhou et al., 2020). Recent pharmaceutical studies have shown that paeoniflorin exerts significant antidepressant effects, by upregulating monoaminergic neurotransmitter levels, inhibiting hypothalamic-pituitary-adrenal axis hyperfunction, promoting neuroprotection, promoting hippocampal neurogenesis, upregulating brain-derived neurotrophic factor levels, inhibiting inflammatory responses and reducing nitric oxide levels, among others (Wang et al., 2021). Zhong et al. (2018) found that paeoniflorin ameliorated chronic stress-induced depression-like behavior and neuronal damage in rats by activating the ERK-CREB pathway (Zhong et al., 2018). Chang et al. (2015) demonstrated that paeoniflorin exerts an antidepressant effect by reducing neuroinflammation by inhibiting CASP-11-dependent pyroptosis signal transduction (Chang et al., 2015). Some researchers found that P. lactiflora could regulate CUMS-induced metabolic disorders in rats (Tian et al., 2021). However, studies have not yet elucidated the relationship between the metabolites, metabolic pathways and targets of paeoniflorin antidepressant effects.
Network pharmacology is an emerging discipline that explores the associations of drugs, targets and diseases through network information analysis. It provides a novel research potentially combining the traditional “one target, one drug” strategy. This has been transformed into a “multi-target, multi-component” research method (Meng et al., 2020). This method is consistent with the holistic view of TCM theory. Metabolomics represents a fast-growing field of “omics” that could help comprehensively assess endogenous metabolites in biological samples, making it possible to identify true regulatory models of molecular metabolites in vivo.
In recent years, urinary metabolomics has become an important research method for the discovery of non-invasive biomarkers (Kwon et al., 2020). Urine is the end product of the body’s metabolic network, which is rich in metabolites and can reflect the overall metabolic level in the body. Urine has the characteristics of non-invasive and easy-to-obtain specimens, which can reflect the physiological and pathological states of the body and the response of the body to drugs and toxic substances. It is an ideal object for scientific research (Zhang et al., 2016). Currently, LC-MS is the most widely used technique for urine metabolomics analysis (Wu et al., 2022).
This study employed a combination of network pharmacology and LC-MS-based untargeted metabolomics to explore urinary biomarkers of paeoniflorin in depression therapy. Metabolic pathway and comprehensive metabolic network analyses could help identify major pathways involved in CUMS-induced depression and uncover potential therapeutic targets. This study not only provides a solid foundation and a new perspective for the characterization of paeoniflorin’s anti-depressant effects, but also provides a novel approach for studying the rationality of the compatibility of other traditional Chinese medicines.
2 Material and Methods
2.1 Animals and Treatments
Thirty-two SPF male Sprague-Dawley rats (200 ± 20 g) were provided by Beijing Vital River Laboratory Animal Technology Co., Ltd. [animal license No. SCXK (Beijing) 2016-0011]. Animal housing was carried out under a 12/12 h light-dark cycle at 22°C ± 2°C and 50%–60% humidity, with water and rodent chow ad libitum. Following 1 week of adaptive feeding, the animals were randomized into four groups, including the control (NC, n = 8), model (CUMS, n = 8), paeoniflorin (PF, n = 8) and fluoxetine (FLU, n = 8) groups. The control group had four rats per cage, and the other groups had one animal per cage. Assays involving animals had approval from the Institutional Animal Care and Use Committee of Beijing University of Chinese Medicine, in compliance with the Animal Welfare Guidelines (BUCM-4-2014070401-3001).
From the fourth week of model establishment, all animals were given intragastric administration 30–60 min before model establishment. The 32 rats received the following medications: 1) Control rats, no stressor and intragastric (ig) treatment with distilled water (10 ml/kg body weight); 2) CUMS group, modeling and ig treatment with distilled water (10 ml/kg body weight); 3) PF group, modeling and ig treatment with paeoniflorin (10.0 mg/kg/d, 10 ml/kg body weight) (Yajing 2021); 4) FLU group, modeling and ig treatment with fluoxetine (2.0 mg/kg/d, 10 ml/kg body weight) (Hou et al., 2020).
2.2 Chemicals and Materials
Paeoniflorin was produced by Chengdu Lemeitian Pharmaceutical Technology (Chengdu, China). Paeoniflorin purity: HPLC ≥ 98%. Fluoxetine hydrochloride was provided by Shanghai McLean Biochemical Technology (Shanghai, China). Sucrose was provided by Sinopharm Chemical Reagent (Shanghai, China).
LC/MS-grade methanol, acetonitrile and water were purchased from Fisher Chemical (Roskilde, Denmark). 2-Propanol were purchased from Merck (Darmstadt, Germany). Aormic acid were purchased from CNW (Shanghai, China). 2-Chloro-L-Phenylalanine were purchased from Adamas-beta (Shanghai, China).
2.3 Chronic Unpredictable Mild Stress Model Establishment
Except for control rats, all groups underwent daily exposures in random order to one of the following chronic unpredictable mild stress for 6 weeks: 24-h fasting, 24-h water-deprivation, 5-min ice water swimming at 4°C, 1-min tail clamping (1 cm from the tail root), 3-h behavior restriction, 5-min hot baking (45°C), 24-h strange smell (glacial acetic acid sprayed on the litter for 24 h) and 24-h damp bedding. CUMS was randomly performed as summarized in Table 1. Figure 1A described the experimental schedule.
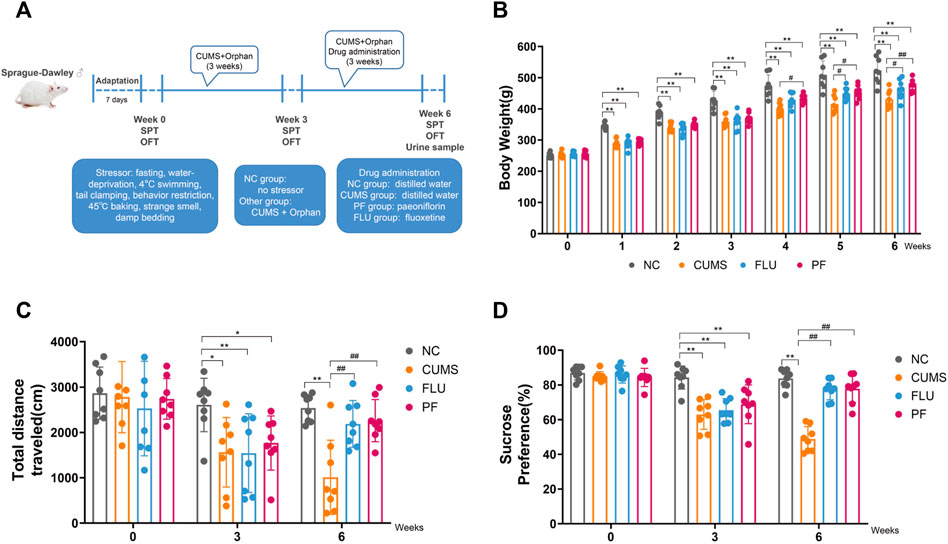
FIGURE 1. (A) Flow chart for establishing a CUMS-induced depression model. (B) Body Weights in various groups. (C) Total distance in various groups. (D) Sugar consumption rates in various groups. Data are mean ± standard deviation, n = 8. *p < 0.05, **p < 0.01 versus NC group; #p < 0.05, ##p < 0.01 versus CUMS group.
2.4 Body Weight Measurements and Behavioral Tests
Body weights in all rats were recorded weekly, and behavioral tests were performed at weeks 0, 3, and 6. Behavioral tests included sucrose preference test and open field test, and all animals underwent three behavioral tests. The first behavioral experiment was carried out after 1 week of adaptive feeding, and then the modeling was started. The second behavioral experiment was carried out 3 weeks after modeling. Modeling continued for 3 weeks after the second behavioral test, and medication was administered during the modeling period. Therefore, in addition to the behavioral testing time, the model was modeled for a total of 6 weeks. A third behavioral experiment was conducted 6 weeks after modeling. Rat urine samples were collected after the third behavioral experiment.
The open field test was carried out for evaluating behavioral characteristics in each group such as autonomous activity, exploration and anxiety. The open field box was opened in the room, and a camera was placed above the center of the open field box and connected to a computer for real-time video recording. Prior to the actual test, the animals were placed in the room 30 min for adaptation. For the test, the animals were placed in the center of the open field box, and their movements were recorded with a high-definition camera for 5 min. Ethovision v3.0 was utilized to assess rat behavior in various groups. After each experiment, 75% ethyl alcohol and clean water were utilized for cleaning the open field box successively. Upon smell dissipation, the next test was carried out; the entire assay was performed in a quiet environment.
The sucrose preference test was carried out in two steps. First, the animals were housed in single cages, with 2 flasks of 1% sucrose solution per cage and ad libitum drinking for 24 h. Next, one flask of 1% sucrose solution and one of purified water were placed per cage, for a 24-h ad libitum drinking. In the training period, the animals underwent a 24-h fasting for food and water. In the following step, a flask of 1% sucrose solution and one of purified water were placed per cage, with the animals allowed a 1-h ad libitum drinking. The sucrose solution and purified water were recorded pre- and post-experiment. Sucrose preference was derived as follows: Sucrose preference (%) = (sucrose intake/total intake) × 100. This assay is mainly utilized for assessing anhedonia level in rats.
2.5 LC-MS-Based Urine Metabolomics
2.5.1 Urine Sample Preparation
After the last behavioral experiment, all animals underwent individual housing in metabolic cages to collect 12-h urine samples. Then, urine specimens were centrifuged (3,500 g, 4°C) for 10 min, and supernatants were kept at −80°C. Urine samples for LC-MS were prepared as follows. First, 200 μl of each urine specimen was placed into a 1.5-ml tube and mixed with 800 μl of extraction solution [methanol-acetonitrile 1:1 (v/v)] containing 0.02 mg/ml of L-2-chlorophenylalanine as an internal standard. Then, after vortexing and mixing for 30 s, low-temperature ultrasonic extraction was performed for 30 min (5°C, 40 kHz). Next, the samples were incubated at −20°C for 30 min, followed by a 15-min centrifugation (13,000 g, 4°C). The resulting supernatants were collected and dried under a nitrogen stream. After drying, 120 μl of acetonitrile-water (1:1) was added for reconstitution, followed by vortex-mixing for 30 s; extraction was carried out by a 5-min low-temperature ultrasonication (5°C, 40 kHz). Finally, a 10-min centrifugation (13,000 g, 4°C) was performed, and supernatants were collected into injection vials for on-machine analysis. In addition, 20 µl supernatants from each sample were mixed as a quality control (QC) sample.
2.5.2 Untargeted LC-MS Analysis
LC-MS was carried out in this study on a UHPLC-QExactiveHF-X system (Thermo Fisher Scientific). Chromatographic conditions were: column, ACQUITYUPLCHSST3 (100 mm × 2.1 mm, 1.8-µm i.d.) (Waters, Milford, United States); mobile phase A, 95% water and 5% acetonitrile (with 0.1% formic acid); mobile phase B, 47.5% acetonitrile, 47.5% isopropanol and 5% water (with 0.1% formic acid); injection volume, 2 μl; column temperature, 40°C. Mass spectrometry was performed in the positive and negative modes with electrospray ionization.
2.5.3 Data Processing
Raw data were imported into ProgenesisQI (Waters Corporation, Milford, United States), a metabolomics software that performs baseline filtering, peak detection, integration, retention time correction, peak alignment and normalization. The resulting data matrix contains retention times, mass/charge ratios and peak intensities, among others. Then, the software was utilized for peak identification, matching MS and MS/MS data with the metabolic database. The MS mass error was < 10 ppm, and metabolite identification was performed according to the secondary mass spectrometry match scores. The Human Metabolome Database (HMDB, http://www.hmdb.ca/), METLIN database (https://metlin.scripps.edu/) and self-built databases were used for retrieval.
2.5.4 Metabolomic Data Analysis
Principal component analysis (PCA) was carried out to detect outliers in rat urine samples and to distinguish the degree of similarity and difference in metabolic profiles of urine specimens among various groups. Next, orthogonal partial least squares discriminant analysis (OPLS-DA) was used for screening for metabolites that may help differentiate groups, parameters R2Y (cum) and Q2 (cum) were utilized to assess the quality of the OPLS-DA model, and metabolites with VIP > 1.2 and p < 0.05 were selected as potential molecular markers. Finally, metabolic pathways were determined based on differential metabolites, with p < 0.05 as a criterion. The analysis software in this study was the Majorbio Cloud Platform (www.majorbio.com). In addition, the potential molecular markers were imported into Cytoscape to generate a “Compound-Reaction-Enzyme-Gene” network.
2.6 Network Analysis
Firstly, the corresponding targets of paeoniflorin were determined using TCMSP (https://tcmsp-e.com/) and SwissTargetPrediction (http://www.swisstargetprediction.ch/). Depression-related targets were collected from the three databases GeneCards, OMIM and DRUGBANK, with “Depression” and “Depressive Disorder” as keywords, and the disease targets collected in the three disease databases were combined, and duplicate targets were deleted. The common target between the target of depression and the target of paeoniflorin was taken as a potential target of paeoniflorin in the treatment of depression. The potential targets were combined with related targets in the “compound-reaction-enzyme-gene” network as potential therapeutic targets of paeoniflorin. VENN diagrams were drawn using website (http://bioinformatics.psb.ugent.be/webtools/Venn/). Gene names were verified with Uniprot (https://www.uniprot.org/).
Secondly, potential therapeutic targets were submitted to STRING (https://string-db.org/) for protein-protein interaction (PPI) detection, and a PPI network was constructed by Cytoscape 3.7.1. Key targets were screened using the degree and betweenness of the plug-in model CytoHubba.
Finally, we used the OmicShare Tools (https://www.omicsshare.com/tools/home/report/goenrich.html) to perform biological pathway enrichment analysis to elucidate the function of each target and its function in signal transduction, including KEGG and GO analyses.
2.7 Molecular Docking
In this paper, AutoDock Vina v1.1.2 was used for molecular docking, and PyMol 2.5 was utilized to process all receptor proteins, including the removal of water molecules, salt ions and small molecules. Docking boxes were defined using PyMOL 2.5 so that all active pockets are wrapped. ADFRsuite 1.0 was used to convert all processed small molecules and receptor proteins to the PDBQT format necessary for AutoDock Vina 1.1.2 docking. The results were visualized and analyzed with PyMol 2.5.
2.8 Statistical Analysis
Data were analyzed with SPSS 20.0 and GraphPad Prism 8.0. All data were presented as mean ± standard deviation (SD). One-way analysis of variance (ANOVA) or nonparametric test was utilized for comparisons on the basis of data normality. p < 0.05 indicated statistical significance.
3 Results
3.1 Paeoniflorin Alleviates Depression-Like Behaviors in Chronic Unpredictable Mild Stress Rats
3.1.1 Paeoniflorin Restores Body Weight in Chronic Unpredictable Mild Stress Rats
Body weight changes in rats of various groups are shown in Figure 1B. Before modeling, body weights were comparable in all groups (p > 0.05). From 1 to 3 weeks after modeling, in comparison with the NC group, body weights in the CUMS, FLU and PF groups were markedly decreased, with statistical differences (p < 0.01). After 1 week of drug treatment, in comparison with the CUMS group, body weights in the PF groups were increased, with statistically significant differences (p = 0.047); compared with the CUMS group, the FLU weight appeared to increase, but the difference was not statistically significant (p = 0.095). Following 2 weeks of drug administration, in comparison with the CUMS group, body weights in the PF and FLU groups were increased, with statistically significant differences (p = 0.012, p = 0.035). Following 3 weeks of drug administration, in comparison with the CUMS group, body weights in the FLU group were increased (p = 0.037), and body weights in the PF group were significantly increased (p = 0.006).
3.1.2 Paeoniflorin Restores Total Distance in Chronic Unpredictable Mild Stress-Like Depressed Rats in an Open Field Test
The open field test was carried out before modeling, 3 weeks after modeling, and 6 weeks after modeling (Figure 1C). The total moving distances in various groups before modeling were roughly the same, with no statistically significant differences. There were differences in the total moving distances of rats at 3 weeks after modeling. In comparison with the NC group, total moving distances in the CUMS and PF group were reduced (p = 0.014, p = 0.037); in comparison with the NC group, total moving distances in the FLU groups were also markedly reduced (p = 0.003). After 6 weeks of modeling, the situation was reversed. In comparison with the CUMS group, total moving distances in the PF and PLU groups were markedly increased (p < 0.0001, p = 0.002).
3.1.3 Paeoniflorin Responded to the Amount of Sugar Water Consumed by Chronic Unpredictable Mild Stress Rats
The sucrose preference test was performed prior to modeling, 3 weeks after modeling and 6 weeks after modeling (Figure 1D). Before the start of the experiment, sugar consumption rates of various groups of rats were roughly the same, with no statistical differences. After 3 weeks of modeling, there were differences in drinking sugar amounts in the rats. In comparison with the NC group, sugar consumption rates in the CUMS, FLU, and PF groups were markedly decreased, with statistical differences (p < 0.01). The situation was reversed following 3 weeks of continuous treatment with paeoniflorin and fluoxetine. In comparison with the CUMS group, sugar consumption rates in the PF and PLU groups were starkly increased, with statistically significant differences (p < 0.01).
3.2 Applying Metabolomics to Analyze Principal Components
The data of each group were assessed by unsupervised principal component analysis (PCA), and the NC, CUMS and PF groups were significantly separated. These trends indicated differences in metabolic profiles among groups, i.e., significant changes in urinary endogenous metabolites, as shown in Figure 2. There was a partial overlap between the NC and PF groups, which demonstrated after intervention with paeoniflorin in CUMS model rats, the metabolic state in the body tended to be close to that of the normal group. The PF group was distributed between the NC and CUMS groups, suggesting paeoniflorin has a marked regulatory effect on metabolic disorders triggered by CUMS-induced depression-like behavior. The QC samples showed good aggregation, and the results indicated that the entire analysis system had good stability and repeatability, meeting the requirements for metabolomics analysis.
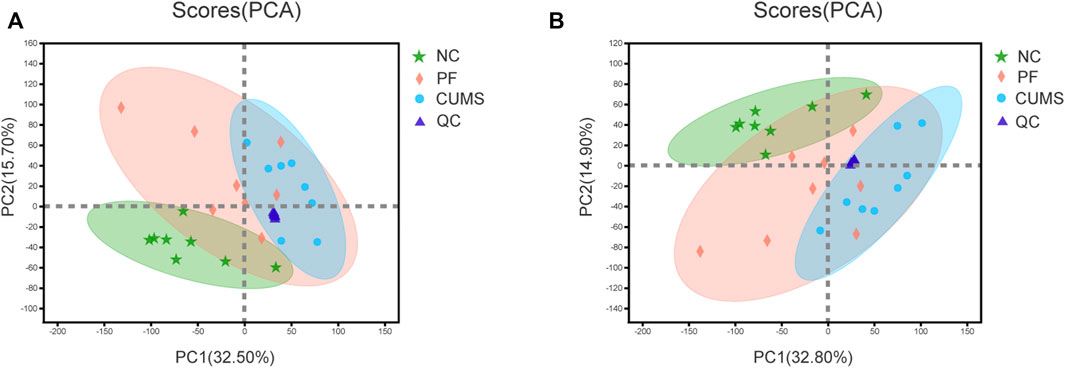
FIGURE 2. PCA score plot. Plot of PCA scores for the NC (
OPLS-DA was used to further define potential biomarkers between group pairs. All loading plots were tested with 200 random permutations to ensure reliability and prevent overfitting. OPLS-DA of NC and CUMS groups showed that samples from the same group clustered together, and samples from different groups were well distinguished (Figures 3A,C). The random permutation test revealed R2X, R2Y and Q2 were 0.475, 0.988 and 0.917 in the positive mode, respectively (Figure 3B), and R2X, R2Y and Q2 were 0.603, 0.996 and 0.938 in the negative mode, respectively (Figure 3D). OPLS-DA of CUMS group and PF group showed that samples from the same group clustered together and samples from different groups distinguished well, as shown in Figures 3E,G. The random permutation test demonstrated R2X, R2Y and Q2 were 0.403, 0.883 and 0.498 in the positive mode, respectively (Figure 3F), and R2X, R2Y and Q2 were 0.453, 0.839 and 0.555 in the negative mode, respectively (Figure 3H). This shows that the model has good explanatory and predictive ability. Based on criteria of VIP > 1.2 and p < 0.05, differential metabolites were obtained as potential molecular markers, as shown in Table 2.
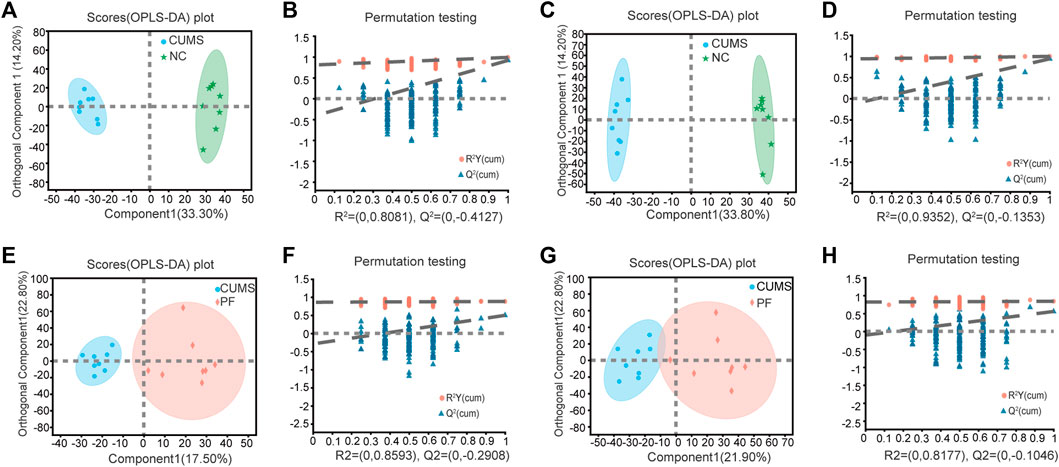
FIGURE 3. Multivariate data analysis of urine metabolites of rat samples. OPLS-DA score plot and permutation test of the NC and CUMS groups (A,B) in the positive ion mode and (C,D) in the negative ion mode. OPLS-DA score plot and permutation test of the CUMS and PF groups (E,F) in the positive ion mode and (G,H) in the negative ion mode.
Among these biomarkers, except 2-{4-[(1E)-1,2-diphenylbut-1-en-1-yl]phenoxy}ethan-1-ol, 9,15-dioxo-11R-hydroxy-2,3,4,5-tetranor-prostan-1,20-dioic acid and Trans-Chlorogenic acid, the levels of 53 biomarkers in CUMS rats were partially reversed by paeoniflorin. Figure 4 shows a heatmap of the relative intensities of biomarkers and the separation between different groups, especially paeoniflorin with a modulating effect on CUMS rats. We found significant changes in the CUMS group compared to the NC group, with elevated levels of 35 metabolites, such as (+/-)-Hexanoylcarnitine, Blumenol C glucoside, and Biopterin. There were decreased levels of 18 metabolites such as Citric acid, Thiamine monophosphate, Gluconolactone, 5-Hydroxyindoleacetic acid. Paeoniflorin significantly restored the levels of Citric acid, Thiamine monophosphate, Gluconolactone, 5-Hydroxyindoleacetic acid and other metabolites in CUMS rats after intervention.
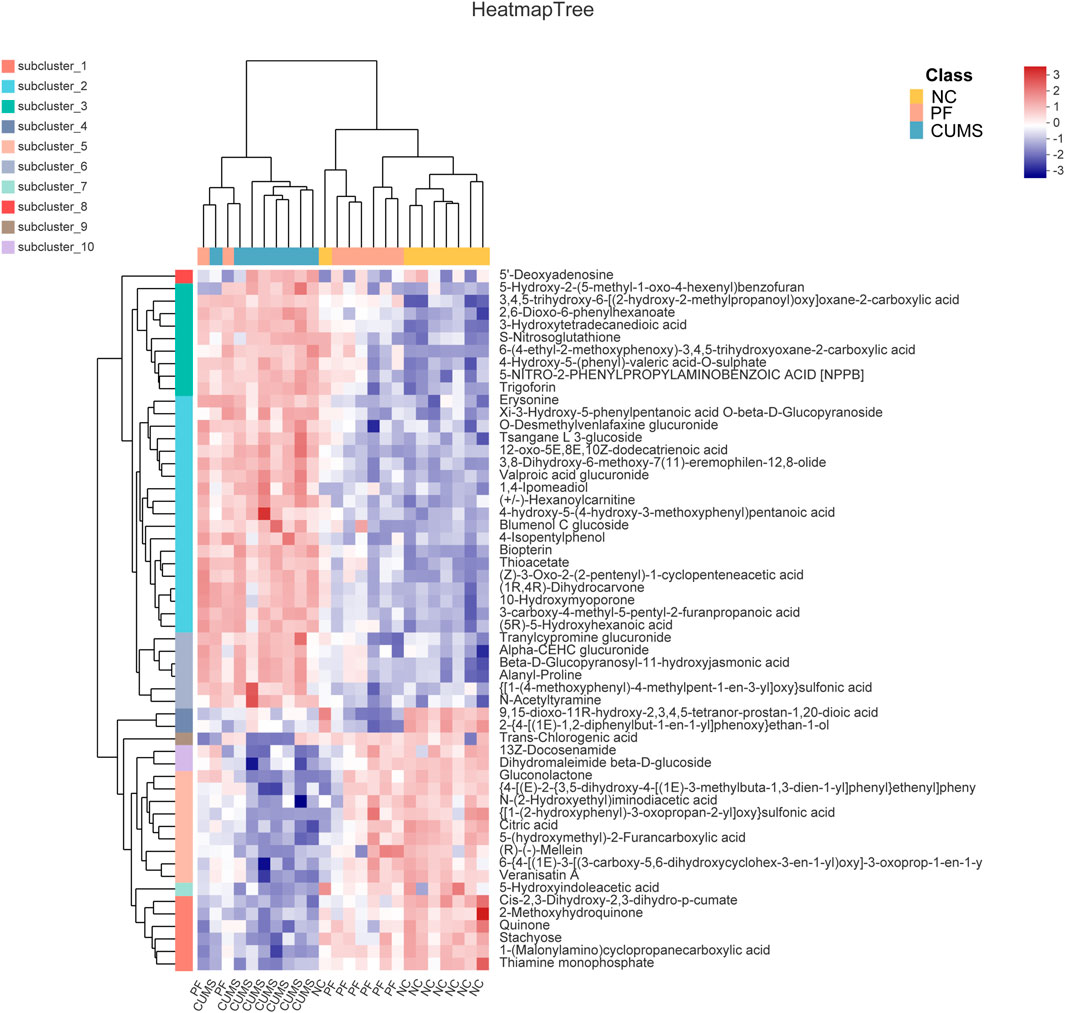
FIGURE 4. Comparison of differentially expressed metabolite levels between different groups. Each row corresponds to data for a specific metabolite, and each column represents the NC, CUMS, or PF group. Colors indicate the relative expression levels of metabolites in this group of samples.
3.3 Analysis of Paeoniflorin-Related Metabolites and Metabolic Pathways
As shown in Figures 5A,B, metabolic pathways including differential metabolites were assessed through the Majorbio Cloud Platform. The KEGG pathways including differential metabolites were counted, among which the carbohydrate metabolism pathway had the most differential metabolites. KEGG analysis was carried out for pathways comprising differential metabolites, and p < 0.05 was used as the screening basis to obtain five important metabolic pathways, including the citrate cycle (TCA cycle), glucagon signaling pathway, thiamine metabolism, taste transduction and alanine, aspartate and glutamate metabolism. The relevant metabolites involved were citric acid, thiamine monophosphate, gluconolactone and 5-hydroxyindoleacetic acid.
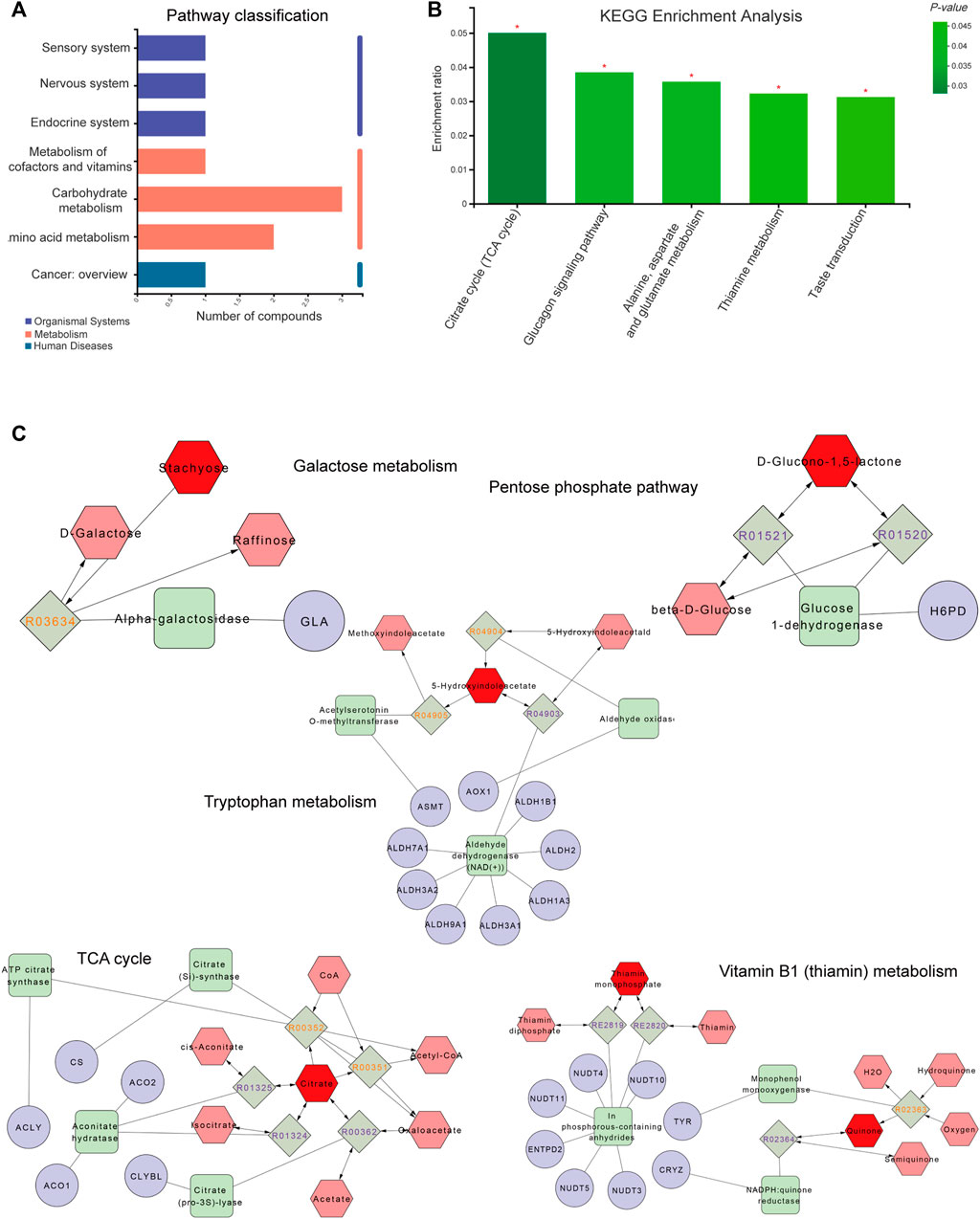
FIGURE 5. Metabolite pathway analysis and networks. (A) The ordinate and abscissa represent the secondary classification of a KEGG metabolic pathway and the amount of related metabolites, respectively. (B) The abscissa and ordinate represent the pathway’s name and enrichment rate, respectively. (C) Compound-reaction-enzyme-gene network. Red hexagons, gray diamonds, green rectangles and purple circles are active compounds, reactions, enzymes and genes, respectively. *p < 0.05, **p < 0.01 and ***p < 0.001.
3.4 Metabolomics Combined With Network Analysis to Predict the Possible Mechanisms Involved in Paeoniflorin
To fully understand the mechanism by which paeoniflorin alleviates depression, differential metabolites were imported into the MetScape plugin of Cytoscape to generate a “Compound-Reaction-Enzyme-Gene” network, and an interaction network combining metabolomics and network pharmacology was built (Figure 5C). The results demonstrated related targets included NUDT4, NUDT5, NUDT3, CRYZ, CS, NUDT10, CLYBL, ALDH2, ALDH3A1, ALDH1B1, ALDH1A3, ALDH9A1, ALDH3A2, GLA, AOX1, ASMT, ACLY, ACO1, ACO2, ALDH7A1, NUDT11, TYR, ENTPD2 and H6PD. Relevant metabolites were thiamin monophosphate, D-glucono-1,5-lactone, stachyose, citrate and 5-hydroxyindoleacetate. The relevant metabolic pathways were the TCA cycle, Vitamin B1 (thiamin) metabolism, tryptophan metabolism, pentose phosphate pathway and galactose metabolism. Combined with KEGG analysis results of differential metabolites, the TCA cycle was the key metabolic pathway of paeoniflorin in the treatment of depression, and citric acid, thiamine monophosphate, gluconolactone, 5-hydroxyindoleacetic acid and stachyose were the key metabolites of paeoniflorin in the treatment of depression.
3.5 Chemical Composition Network Analysis
3.5.1 Potential Targets of Paeoniflorin in Depression Therapy
First, paeoniflorin’s targets were searched in the TCMSP database and SwissTarget website, and 111 targets were retrieved. Totally 1810 depression disease targets were obtained by searching the GeneCards, DRUGBANK, OMIM and TTD databases. The targets of paeoniflorin and the disease targets of depression were combined, and common targets were considered the possible targets of paeoniflorin in depression treatment, 54 in total. A VENN diagram was drawn (Figure 6A). The potential targets were merged with related targets in the “Compound-Reaction-Enzyme-Gene” network, a total of 78 potential targets were used for subsequent analysis.
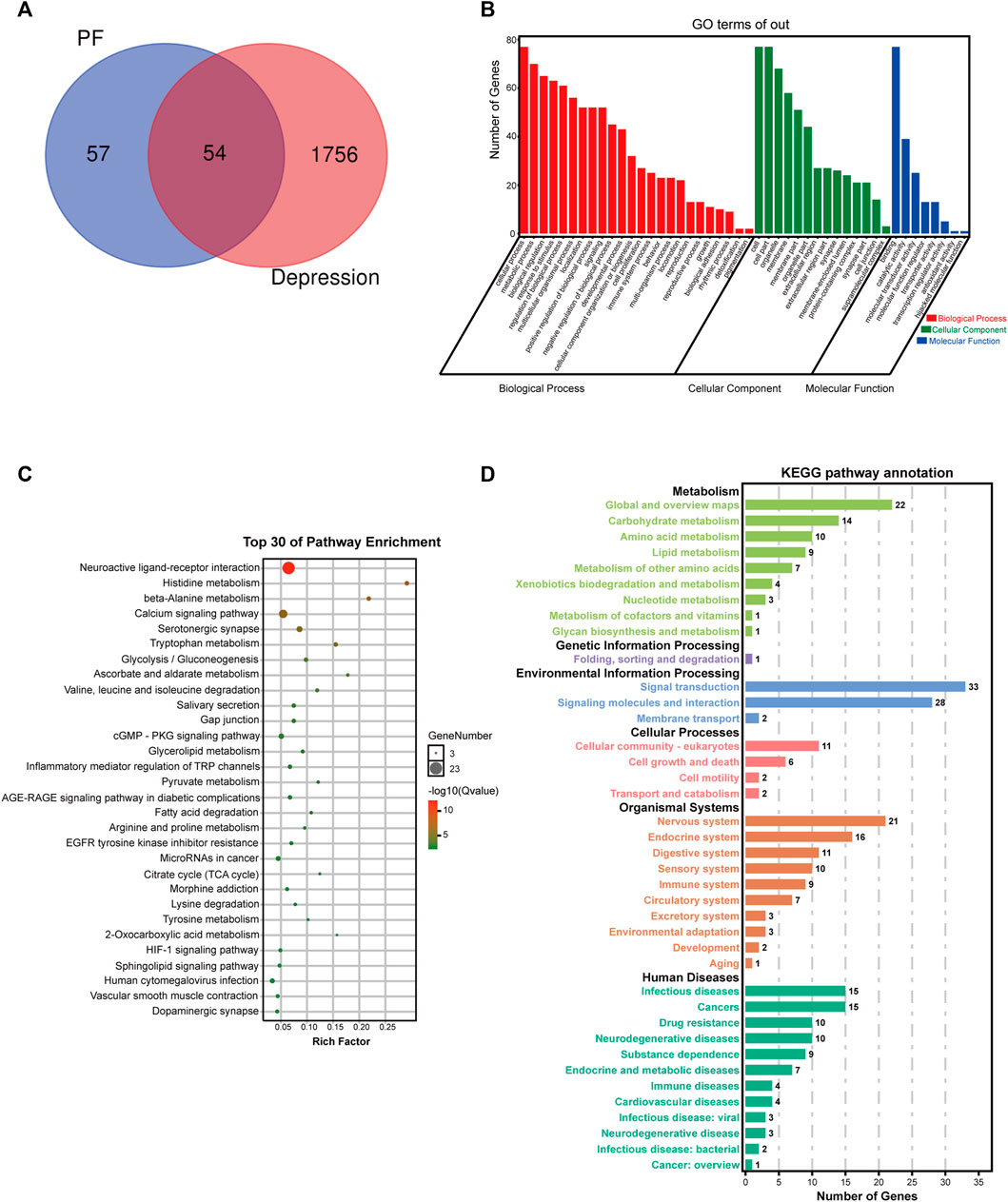
FIGURE 6. Potential target network analysis. (A) Venn diagram of potential targets. (B) GO analysis of potential targets. (C) Top 30 KEGG pathways of potential targets. (D) KEGG pathway annotation.
3.5.2 Pathway Prediction of Paeoniflorin by KEGG and GO
To elucidate the biological characteristics of potential targets, GO enrichment analysis was performed with the OmicShare Tools. Totally 46 GO terms were detected, including 24 biological process (BP), 8 molecular function (MF) and 14 cellular component (CC) terms (Figure 6B). BP terms involved multiple genes were significantly enriched in cellular process, metabolic process, biological regulation, response to stimulus, multicellular organismal process, localization, signaling, developmental process, cellular component organization or biogenesis, cell proliferation, immune system process, locomotion, etc. In addition, CC and MF terms associated with paeoniflorin’s antidepressant effect were cell, organelle, membrane, extracellular region, synapse, membrane-enclosed lumen, protein-containing complex, binding, catalytic activity, molecular transducer activity, molecular function regulator, antioxidant activity, etc.
To further explore these potential targets, KEGG analysis was carried out to illustrate the functional activities involved in paeoniflorin’s therapeutic effect on depression. Figure 6C shows the top 30 signaling pathways obtained by KEGG analysis, as well as the classification statistics of signaling pathways enriched by KEGG analysis (Figure 6D). The results of KEGG enrichment analysis showed the main pathways associated with paeoniflorin’s antidepressant effect included neuroactive ligand-receptor interaction, histidine metabolism, calcium signaling pathway, beta-alanine metabolism, serotonergic synapse, tryptophan metabolism, glycolysis/gluconeogenesis, ascorbate and aldarate metabolism, cGMP-PKG signaling pathway, inflammatory mediator regulation of TRP channels, citrate cycle (TCA cycle), HIF-1 signaling pathway and sphingolipid signaling pathway. The B-class classification statistics of various pathways showed that paeoniflorin mainly exerts antidepressant effects through activities such as the nervous system, carbohydrate metabolism, amino acid metabolism and signal transduction.
3.5.3 Protein-Protein Interaction Network and Major Antidepressant Targets of Paeoniflorin
The protein-protein interaction relationships of potential targets were obtained based on the STRING database. This PPI network consisting of 78 nodes and 293 edges. The information was imported into CytoScape 3.7.1 to visualize the PPI network relationship, and the CytoHubba function was used to calculate degree values. The results showed that the four targets with the largest degree values were SLC6A4, TNF, IL6 and SLC6A3. Therefore, these four targets were considered key targets of paeoniflorin in the treatment of depression, as shown in Figure 7.
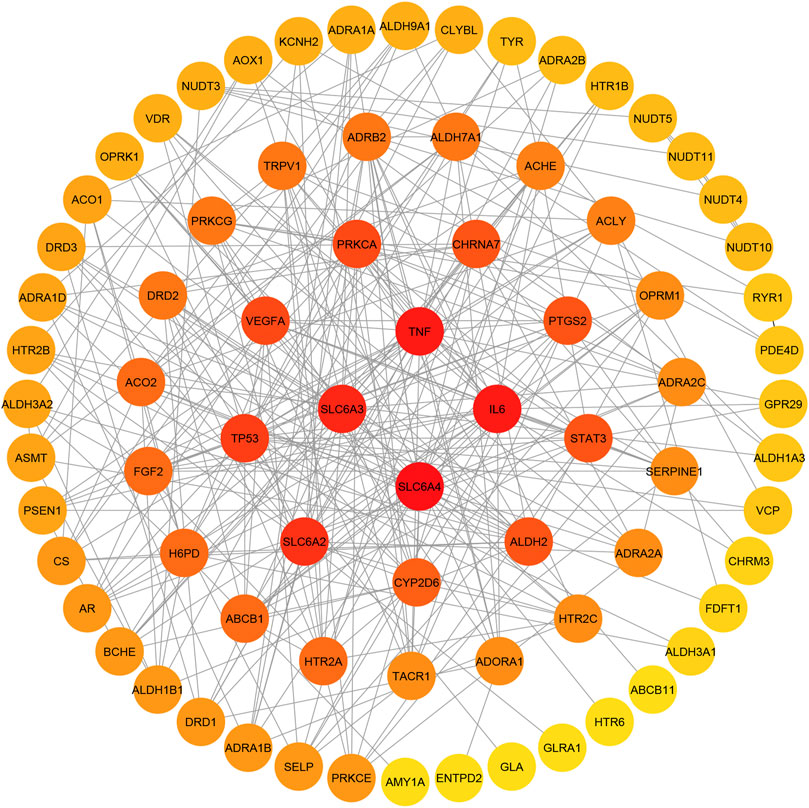
FIGURE 7. Potential target interaction network (PPI). The color of the circle changes from red to yellow to represent the change of the degree value of the target point from large to small; the four target points in the center have the largest degree values.
3.6 Components-Targets Docking Analysis
According to the generated PPI network, the four key targets were used for molecular docking. The docking binding of paeoniflorin to each target is shown in Table 3. Molecular docking showed that paeoniflorin had good binding activity to key targets. In particular, the binding energy of paeoniflorin to SLC6A4 was −10.2 kcal/mol, suggesting that the combination is closely bound and has high activity potential. The binding mode of paeoniflorin to key targets is shown in Figure 8.
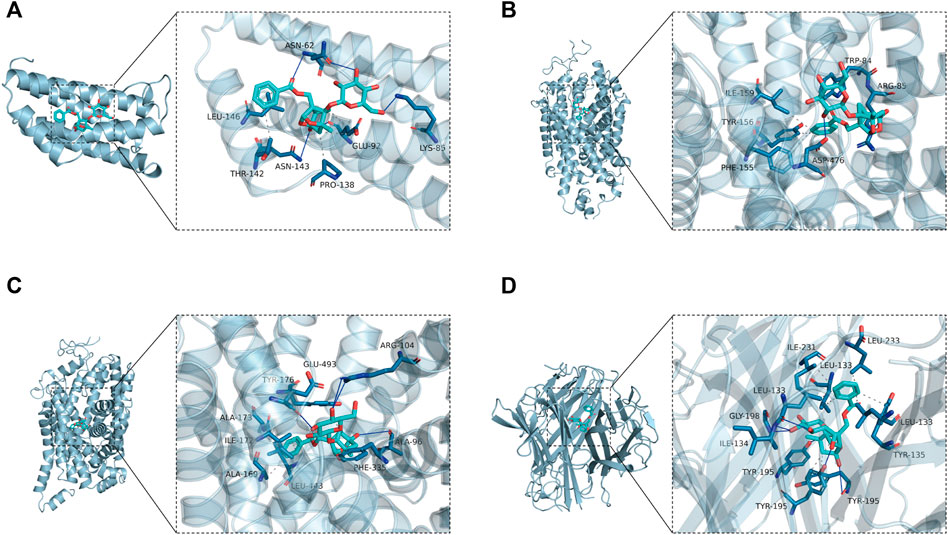
FIGURE 8. Molecular docking-predicted binding mode. (A) Molecule docking of PF binding to IL6. (B) Molecule docking of PF binding to SLC6A3. (C) Molecule docking of PF binding to SLC6A4. (D) Molecule docking of PF binding to TNF. Left panel, overall view; right panel, partial view; cyan stick, small molecule; light blue cartoon, protein; blue line, hydrogen bond; gray dotted line, hydrophobic interaction.
As shown in Figure 8A, paeoniflorin binds to the middle of the IL6 helix, and forms hydrogen bonds with ASN-62, ASN-143 and LYS-85 on the protein. In addition, it also forms hydrophobic interactions with GLU-92, PRO-138, THR-142 and LEU-146. Hydrogen bonding and hydrophobic interactions are the main forces that maintain the binding of small molecules and proteins. As shown in Figure 8B, paeoniflorin can form hydrogen bonds with ASP-476 and ARG-85 on the protein. In addition, it can also form hydrophobic interactions with ILE-159, TYR-156, PHE-155 and TRP-84 on the protein to further strengthen the binding between the molecule and the protein. As shown in Figure 8C, similar to paeoniflorin/SLC6A3, paeoniflorin also binds inside the helix of the protein, and the binding is maintained mainly by hydrogen bonds and hydrophobic interactions. For example, small molecules form hydrogen bonds with TYR-176, ARG-104 and ALA-96 in proteins. It also forms hydrophobic interactions with ALA-173, ILE-172, ALA-169, LEU-443, PEH-335 and TYR-176. As shown in Figure 8D, paeoniflorin binds to the inner active channel formed by TNF trimers, and can form hydrogen bonds with LEU-133, ILE-134 and TYR-195 in the channel. It can also form hydrophobic interaction with LEU-133, ILE-231, LEU-233, LEU-133 and TYR-195 in proteins.
4 Discussion
Depression is the most common mental disorder in clinical. Depression is largely underdiagnosed and rarely treated due to stigma, lack of effective treatments and insufficient mental health resources (Smith 2014). In recent years, the prevalence of depression has increased year by year in different age groups, making it one of the most important global health problems (Park et al., 2019). Current treatments for depression include antidepressants and somatic therapy (Voineskos et al., 2020). Among them, antidepressants are the most commonly used treatments for patients with depression. However, it is known that only 20%–40% of depressed patients respond to low doses of these drugs, and the risk of adverse effects increases with higher doses (de Oliveira et al., 2022). Studies have found that herbal medicine has a strong antidepressant effect with few adverse reactions (Wang et al., 2017; Chi et al., 2019). In our previous experiments, we confirmed that paeoniflorin is one of the main components of Xiaoyaosan, a classic antidepressant herbal medicine (Ding et al., 2020).
In this study, we explored the targets, efficacy and underlying mechanisms of paeoniflorin in the treatment of depression through metabolomics and network analysis. To verify the antidepressant effect of paeoniflorin, CUMS combined with Orphan was established to induce depression in rats. As previously reported by others, CUMS is currently a well-recognized animal model of depression, and the animal model established in combination with orphanage can stably simulate the core symptom of human depression, namely anhedonia (Willner et al., 1987; Hill et al., 2012; Hu et al., 2017; Zheng et al., 2021). In the present study, CUMS led to a decrease in the sucrose preference rate in SPT and a decrease in total travel distance in OFT, which could be reversed by paeoniflorin. Depression is often related to loss of appetite, fatigue and anhedonia, showing that CUMS-induced changes in these parameters are similar to clinical symptoms of depression (Goldstein Ferber et al., 2021). These results demonstrate that the antidepressant-like effects of paeoniflorin are associated with chronic stress-induced decreased motor activity and anhedonia in rats.
In an LC-MS-based untargeted urine metabolomic analysis, we identified 56 metabolic biomarkers. CUMS rats had reduced levels of 18 metabolites, such as Citric acid, Quinone, Thiamine monophosphate, and 5-Hydroxyindoleacetic acid, which were consistent with findings on metabolite levels in other depression (Zhang et al., 2013; Gao et al., 2014; Ding et al., 2015; Sun et al., 2022). After paeoniflorin intervention, 18 metabolites decreased in CUMS rats were upregulated, and they exerted antidepressant effects mainly by regulating citrate cycle (TCA cycle), glucagon signaling pathway, thiamine metabolism, taste transduction and amino acid metabolism.
Multiple studies have reported an association between the TCA cycle and depression. Some researchers believe that the TCA cycle is specific and blocked in depression (Mishra et al., 2020; Ting et al., 2020; Tian et al., 2022). Mitochondrial dysfunction or changes in brain energy metabolism may be involved in the pathogenesis of depression (Bansal and Kuhad 2016; Kolar et al., 2021). Citric acid is a key intermediate in the TCA cycle and part of the energy metabolism pathway. In the present study, we found that the levels of citric acid were decreased in depressed rats compared with controls. In contrast, PF significantly upregulated abnormal citric acid levels in the urine of depressed rats. It suggested that paeoniflorin improved the biomarkers of TCA cycle in CUMS-induced depression in rats.
Studies have shown that the pathogenesis of depression is related to the dysfunction of amino acid metabolism (Nagasawa et al., 2012). Glutamate is an important excitatory neurotransmitter that plays a crucial role in regulating neuroplasticity, learning and memory (Deutschenbaur et al., 2016). Chronic stress reduces the structure and function of glutamatergic neurons, resulting in reduced volume of cortical and limbic structures associated with depression (Duman et al., 2019). Glucagon signaling pathway mainly assists glucagon to play a role in raising blood sugar, which can promote the catabolism of glucose. A genome-wide study of suicidal behaviors in depression found that the Glucagon signaling pathway was most abundant in major depression disorder (Zhao et al., 2020). Bendix et al. (2017) found that lower glucagon levels in plasma and cerebrospinal fluid were associated with suicidal behavior (Bendix et al., 2017). Thiamine is essential for normal brain function, and its deficiency leads to metabolic disturbances, specific lesions, oxidative damage, and reduced hippocampal neurogenesis (Vignisse et al., 2017). Zhang et al. (2013) reported a negative correlation between thiamine nutritional status and depressive symptoms in Chinese elderly (Zhang et al., 2013). A transcriptomic analysis found that the taste transduction pathway is downregulated in female unipolar depression, and the authors suggest that the decreased taste perception in depressed patients is attributable to decreased expression levels of taste transduction pathway genes (Dmitrzak-Weglarz et al., 2021).
Network analysis showed that SLC6A4, TNF, IL6, and SLC6A3 were the key targets of paeoniflorin for its antidepressant effect. Molecular docking showed the interaction mode between paeoniflorin and the target protein, and both paeoniflorin and the target protein had good binding activity. The docking results initially revealed the material basis of paeoniflorin in the treatment of depression. SLC6A4 has been shown to be associated with psychiatric disorders, can be used to explain the association between major depressive disorder and suicidal tendencies, and is the most promising therapeutic target for future depression research (Park et al., 2019; Hande et al., 2021). TNF is an important cytokine contributing to the inflammatory hypothesis of depression, has an important function in the pathophysiology of depression, and represents a potential marker of depression (Liu et al., 2020). In a meta-analysis, it was shown that depression can be predicted by measuring IL6 (Colasanto et al., 2020). SLC6A3 can affect depressive symptoms and is a novel candidate biomarker gene for suicidal behavior (Rafikova et al., 2021). Therefore, the reliability of our findings can be fully demonstrated based on the above literature evidence.
5 Conclusion
In the present work, paeoniflorin’s antidepressant effect on CUMS-associated depression was systematically investigated by combining LC-MS-based metabolomics with network pharmacology analysis. Totally 56 biomarkers were identified, with significant changes involved in citrate cycle (TCA cycle), glucagon signaling pathway, thiamine metabolism, taste transduction, vitamin B1 (thiamin) metabolism, tryptophan metabolism, pentose phosphate pathway, galactose metabolism and alanine, aspartate and glutamate metabolism. Moreover, citric acid, thiamine monophosphate, gluconolactone, 5-hydroxyindoleacetic acid and stachyose were selected as key markers, and SLC6A4, TNF, IL6, and SLC6A3 were identified as key targets, which may be related to the antidepressant effect of paeoniflorin. Molecular docking validated the key targets showed good affinities with PF.
To the best of our knowledge, this study provides the first demonstration that PF regulates multiple biological pathways and metabolic changes to attenuate depression-like symptoms by influencing the key target proteins. These findings contribute to a deeper understanding of the mechanism of paeoniflorin in the treatment of CUMS rats. However, we performed multivariate statistical analysis only by untargeted metabolomics. Also, we did not screen for metabolic biomarkers in CUMS rat brain tissue. Therefore, future studies will focus on validating the results by targeted metabolomics and screening metabolic biomarkers in the brain tissue of paeoniflorin-treated CUMS rats. Furthermore, in-depth validation studies are warranted to better understand the regulatory mechanism of paeoniflorin, which is expected to help develop paeoniflorin as a potential complementary drug for the treatment of depression.
Data Availability Statement
The original contributions presented in the study are included in the article/supplementary material, further inquiries can be directed to the corresponding authors.
Ethics Statement
The animal study was reviewed and approved by Institutional Animal Care and Use Committee of Beijing University of Chinese Medicine.
Author Contributions
CL wrote the manuscript. JC, YL, and LF revised and proof-read the manuscript. CL, JC, XW, ZH, YM, and MY performed the experiments. ZC and ZX assisted on dealing with the statistical data. All authors reviewed the manuscript and approved its submission.
Funding
This work was supported by National Natural Science Foundation of China (No. 82174278, 81973748).
Conflict of Interest
The authors declare that the research was conducted in the absence of any commercial or financial relationships that could be construed as a potential conflict of interest.
Publisher’s Note
All claims expressed in this article are solely those of the authors and do not necessarily represent those of their affiliated organizations, or those of the publisher, the editors and the reviewers. Any product that may be evaluated in this article, or claim that may be made by its manufacturer, is not guaranteed or endorsed by the publisher.
References
Alexopoulos, G. S. (2019). Mechanisms and Treatment of Late-Life Depression. Transl. Psychiatry 9 (1), 188. doi:10.1038/s41398-019-0514-6
Bansal, Y., and Kuhad, A. (2016). Mitochondrial Dysfunction in Depression. Curr. Neuropharmacol. 14 (6), 610–618. doi:10.2174/1570159x14666160229114755
Bendix, M., Uvnäs-Moberg, K., Petersson, M., Kaldo, V., Åsberg, M., and Jokinen, J. (2017). Insulin and Glucagon in Plasma and Cerebrospinal Fluid in Suicide Attempters and Healthy Controls. Psychoneuroendocrinology 81, 1–7. doi:10.1016/j.psyneuen.2017.03.019
Chang, X., Jia, H., Zhou, C., Zhang, H., Yu, M., Yang, J., et al. (2015). Role of Bai-Shao towards the Antidepressant Effect of Chaihu-Shu-Gan-San Using Metabonomics Integrated with Chemical Fingerprinting. J. Chromatogr. B Anal. Technol. Biomed. Life Sci. 1006, 16–29. doi:10.1016/j.jchromb.2015.10.007
Chi, X., Wang, S., Baloch, Z., Zhang, H., Li, X., Zhang, Z., et al. (2019). Research Progress on Classical Traditional Chinese Medicine Formula Lily Bulb and Rehmannia Decoction in the Treatment of Depression. Biomed. Pharmacother. 112, 108616. doi:10.1016/j.biopha.2019.108616
Colasanto, M., Madigan, S., and Korczak, D. J. (2020). Depression and Inflammation Among Children and Adolescents: A Meta-Analysis. J. Affect Disord. 277, 940–948. doi:10.1016/j.jad.2020.09.025
de Oliveira, L. R. S., Machado, F. S. M., Rocha-Dias, I., Cod, E. M., De Sousa, R. A. L., and Cassilhas, R. C. (2022). An Overview of the Molecular and Physiological Antidepressant Mechanisms of Physical Exercise in Animal Models of Depression. Mol. Biol. Rep. 2022. doi:10.1007/s11033-022-07156-z
Deutschenbaur, L., Beck, J., Kiyhankhadiv, A., Mühlhauser, M., Borgwardt, S., Walter, M., et al. (2016). Role of Calcium, Glutamate and NMDA in Major Depression and Therapeutic Application. Prog. Neuropsychopharmacol. Biol. Psychiatry 64, 325–333. doi:10.1016/j.pnpbp.2015.02.015
Ding, F., Wu, J., Liu, C., Bian, Q., Qiu, W., Ma, Q., et al. (2020). Effect of Xiaoyaosan on Colon Morphology and Intestinal Permeability in Rats with Chronic Unpredictable Mild Stress. Front. Pharmacol. 11, 1069. doi:10.3389/fphar.2020.01069
Ding, L., Zhang, X., Guo, H., Yuan, J., Li, S., Hu, W., et al. (2015). The Functional Study of a Chinese Herbal Compounded Antidepressant Medicine--Jie Yu Chu Fan Capsule on Chronic Unpredictable Mild Stress Mouse Model. Plos One 10 (7), e0133405. doi:10.1371/journal.pone.0133405
Diniz, B. S. (2018). The Molecular Intersection Between Senescence and Major Depression in the Elderly. Am. J. Geriatr. Psychiatry 26 (11), 1097–1105. doi:10.1016/j.jagp.2018.07.005
Dmitrzak-Weglarz, M., Szczepankiewicz, A., Rybakowski, J., Kapelski, P., Bilska, K., Skibinska, M., et al. (2021). Transcriptomic Profiling as Biological Markers of Depression - A Pilot Study in Unipolar and Bipolar Women. World J. Biol. Psychiatry 22 (10), 744–756. doi:10.1080/15622975.2021.1907715
Dong, L. M., Williams, L. S., Brown, D. L., Case, E., Morgenstern, L. B., and Lisabeth, L. D. (2021). Prevalence and Course of Depression during the First Year after Mild to Moderate Stroke. J. of the Am. Heart Assoc. 10 (13), e020494. doi:10.1161/jaha.120.020494
Duman, R. S., Sanacora, G., and Krystal, J. H. (2019). Altered Connectivity in Depression: GABA and Glutamate Neurotransmitter Deficits and Reversal by Novel Treatments. Neuron 102 (1), 75–90. doi:10.1016/j.neuron.2019.03.013
Gao, X., Guo, B., Yang, L., Liu, J., Zhang, X., Qin, X., et al. (2014). Selection and Dynamic Metabolic Response of Rat Biomarkers by Metabonomics and Multivariate Statistical Analysis Combined with GC-MS. Pharmacol. Biochem. Behav. 117, 85–91. doi:10.1016/j.pbb.2013.12.013
Goldstein Ferber, S., Weller, A., Yadid, G., and Friedman, A. (2021). Discovering the Lost Reward: Critical Locations for Endocannabinoid Modulation of the Cortico-Striatal Loop that Are Implicated in Major Depression. Int. J. Mol. Sci. 22 (4), 1867. doi:10.3390/ijms22041867
Hande, S. H., Krishna, S. M., Sahote, K. K., Dev, N., Erl, T. P., Ramakrishna, K., et al. (2021). Population Genetic Variation of SLC6A4 Gene, Associated with Neurophysiological Development. J. Genet. 100, 16. doi:10.1007/s12041-021-01266-6
Hill, M. N., Hellemans, K. G., Verma, P., Gorzalka, B. B., and Weinberg, J. (2012). Neurobiology of Chronic Mild Stress: Parallels to Major Depression. Neurosci. Biobehav Rev. 36 (9), 2085–2117. doi:10.1016/j.neubiorev.2012.07.001
Hou, Y., Liu, Y., Liu, C., Yan, Z., Ma, Q., Chen, J., et al. (2020). Xiaoyaosan Regulates Depression-Related Behaviors with Physical Symptoms by Modulating Orexin A/OxR1 in the Hypothalamus. Anat. Rec. Hob. 303 (8), 2144–2153. doi:10.1002/ar.24386
Hu, C., Luo, Y., Wang, H., Kuang, S., Liang, G., Yang, Y., et al. (2017). Re-evaluation of the Interrelationships Among the Behavioral Tests in Rats Exposed to Chronic Unpredictable Mild Stress. PLoS One 12 (9), e0185129. doi:10.1371/journal.pone.0185129
Kolar, D., Kleteckova, L., Brozka, H., and Vales, K. (2021). Mini-review: Brain Energy Metabolism and its Role in Animal Models of Depression, Bipolar Disorder, Schizophrenia and Autism. Neurosci. Lett. 760, 136003. doi:10.1016/j.neulet.2021.136003
Kwon, H. N., Lee, H., Park, J. W., Kim, Y. H., Park, S., and Kim, J. J. (2020). Screening for Early Gastric Cancer Using a Noninvasive Urine Metabolomics Approach. Cancers (Basel) 12 (10), 2904. doi:10.3390/cancers12102904
Liu, J. J., Wei, Y. B., Strawbridge, R., Bao, Y., Chang, S., Shi, L., et al. (2020). Peripheral Cytokine Levels and Response to Antidepressant Treatment in Depression: a Systematic Review and Meta-Analysis. Mol. Psychiatry 25 (2), 339–350. doi:10.1038/s41380-019-0474-5
Malhi, G. S., and Mann, J. J. (2018). Depression. Lancet 392 (10161), 2299–2312. doi:10.1016/S0140-6736(18)31948-2
Meng, L., Chen, D., Yang, Y., Zheng, Y., and Hui, R. (2012). Depression Increases the Risk of Hypertension Incidence: a Meta-Analysis of Prospective Cohort Studies. J. Hypertens. 30 (5), 842–851. doi:10.1097/HJH.0b013e32835080b7
Meng, X., Ma, J., Kang, S. Y., Jung, H. W., and Park, Y. K. (2020). Jowiseungki Decoction Affects Diabetic Nephropathy in Mice through Renal Injury Inhibition as Evidenced by Network Pharmacology and Gut Microbiota Analyses. Chin. Med. 15, 24. doi:10.1186/s13020-020-00306-0
Mishra, P. K., Adusumilli, M., Deolal, P., Mason, G. F., Kumar, A., and Patel, A. B. (2020). Impaired Neuronal and Astroglial Metabolic Activity in Chronic Unpredictable Mild Stress Model of Depression: Reversal of Behavioral and Metabolic Deficit with Lanicemine. Neurochem. Int. 137, 104750. doi:10.1016/j.neuint.2020.104750
Nagasawa, M., Ogino, Y., Kurata, K., Otsuka, T., Yoshida, J., Tomonaga, S., et al. (2012). Hypothesis with Abnormal Amino Acid Metabolism in Depression and Stress Vulnerability in Wistar Kyoto Rats. Amino Acids 43 (5), 2101–2111. doi:10.1007/s00726-012-1294-y
Nickel, A., and Thomalla, G. (2017). Post-Stroke Depression: Impact of Lesion Location and Methodological Limitations-A Topical Review. Front. Neurol. 8, 498. doi:10.3389/fneur.2017.00498
Park, C., Rosenblat, J. D., Brietzke, E., Pan, Z., Lee, Y., Cao, B., et al. (2019). Stress, Epigenetics and Depression: A Systematic Review. Neurosci. Biobehav Rev. 102, 139–152. doi:10.1016/j.neubiorev.2019.04.010
Rafikova, E., Shadrina, M., Slominsky, P., Guekht, A., Ryskov, A., Shibalev, D., et al. (2021). SLC6A3 (DAT1) as a Novel Candidate Biomarker Gene for Suicidal Behavior. Genes (Basel) 12 (6), 861. doi:10.3390/genes12060861
Santabarbara, J., Sevil-Perez, A., Olaya, B., Gracia-Garcia, P., and Lopez-Anton, R. (2019). Clinically Relevant Late-Life Depression as Risk Factor of Dementia: a Systematic Review and Meta-Analysis of Prospective Cohort Studies. Rev. Neurol. 68 (12), 493–502. doi:10.33588/rn.6812.2018398
Sun, D. C., Wang, R. R., Xu, H., Zhu, X. H., Sun, Y., Qiao, S. Q., et al. (2022). A Network Pharmacology-Based Study on Antidepressant Effect of Salicornia Europaea L. Extract with Experimental Support in Chronic Unpredictable Mild Stress Model Mice. Chin. J. Integr. Med. 28 (4), 339–348. doi:10.1007/s11655-022-2879-2
Tian, D. D., Wang, M., Liu, A., Gao, M. R., Qiu, C., Yu, W., et al. (2021). Antidepressant Effect of Paeoniflorin Is through Inhibiting Pyroptosis CASP-11/GSDMD Pathway. Mol. Neurobiol. 58 (2), 761–776. doi:10.1007/s12035-020-02144-5
Tian, J. S., Wu, W. Z., Liu, S. B., Ling-Hu, T., Zhao, Y. H., Gao, Y., et al. (2022). Stable Isotope-Resolved Metabolomics Studies on Corticosteroid-Induced PC12 Cells: A Strategy for Evaluating Glucose Catabolism in an In Vitro Model of Depression. J. Proteome Res. 21 (3), 788–797. doi:10.1021/acs.jproteome.1c00516
Ting, L. H., Gao, Y., Li, A. P., Shi, B. Y., Tian, J. S., and Qin, X. M. (2020). A Unique Insight for Energy Metabolism Disorders in Depression Based on Chronic Unpredictable Mild Stress Rats Using Stable Isotope-Resolved Metabolomics. J. of Pharm. and Biomed. Analysis 191, 113588. doi:10.1016/j.jpba.2020.113588
Vignisse, J., Sambon, M., Gorlova, A., Pavlov, D., Caron, N., Malgrange, B., et al. (2017). Thiamine and Benfotiamine Prevent Stress-Induced Suppression of Hippocampal Neurogenesis in Mice Exposed to Predation without Affecting Brain Thiamine Diphosphate Levels. Mol. Cell Neurosci. 82, 126–136. doi:10.1016/j.mcn.2017.05.005
Voineskos, D., Daskalakis, Z. J., and Blumberger, D. M. (2020). Management of Treatment-Resistant Depression: Challenges and Strategies. Neuropsychiatr. Dis. Treat. 16, 221–234. doi:10.2147/NDT.S198774
Wang, X. L., Feng, S. T., Wang, Y. T., Chen, N. H., Wang, Z. Z., and Zhang, Y. (2021). Paeoniflorin: A Neuroprotective Monoterpenoid Glycoside with Promising Anti-depressive Properties. Phytomedicine 90, 153669. doi:10.1016/j.phymed.2021.153669
Wang, Y., Li, M., Liang, Y., Yang, Y., Liu, Z., Yao, K., et al. (2017). Chinese Herbal Medicine for the Treatment of Depression: Applications, Efficacies and Mechanisms. Curr. Pharm. Des. 23 (34), 5180–5190. doi:10.2174/1381612823666170918120018
Weisenbach, S. L., Kim, J., Hammers, D., Konopacki, K., and Koppelmans, V. (2019). Linking Late Life Depression and Alzheimer's Disease: Mechanisms and Resilience. Curr. Behav. Neurosci. Rep. 6 (3), 103–112. doi:10.1007/s40473-019-00180-7
Willner, P., Towell, A., Sampson, D., Sophokleous, S., and Muscat, R. (1987). Reduction of Sucrose Preference by Chronic Unpredictable Mild Stress, and its Restoration by a Tricyclic Antidepressant. Psychopharmacol. Berl. 93 (3), 358–364. doi:10.1007/BF00187257
Wu, D. T., Chang, Y. J., Wang, Y., Ai, M., and Kuang, H. X. (2022). Application Research Progress of Urine Metabolomics in Systemic Lupus Erythematosus. Med. Rev. 28 (04), 677–681.
Xiang, Y., Zhang, Q., Wei, S., Huang, C., Li, Z., and Gao, Y. (2020). Paeoniflorin: a Monoterpene Glycoside from Plants of Paeoniaceae Family with Diverse Anticancer Activities. J. Pharm. Pharmacol. 72 (4), 483–495. doi:10.1111/jphp.13204
Yajing, H. (2021). Antidepressant Mechanism of Xiaoyaosan and Paeoniflorin Based on the Inhibition of Hippocampal Neuron Apoptosis by miR-29b. Chaoyang(China): Beijing University of Chinese Medicine.
Zhang, C. G., Cheng, Q., Tang, Y. B., and Wang, G. (2016). Research Progress in the Application of Urine Metabolomics in the Diagnosis and Mechanism Exploration of Neurodegenerative Diseases. Diagnostics Theory and Pract. 15 (01), 77–79.
Zhang, G., Ding, H., Chen, H., Ye, X., Li, H., Lin, X., et al. (2013). Thiamine Nutritional Status and Depressive Symptoms Are Inversely Associated Among Older Chinese Adults. J. Nutr. 143 (1), 53–58. doi:10.3945/jn.112.167007
Zhang, Y., Chen, Y., and Ma, L. (2018). Depression and Cardiovascular Disease in Elderly: Current Understanding. J. Clin. Neurosci. 47. 1–5. doi:10.1016/j.jocn.2017.09.022
Zhang, Y., Liang, Y., Liu, H., Huang, Y., Li, H., and Chen, B. (2020). Paeoniflorin Attenuates Gestational Diabetes via Akt/mTOR Pathway in a Rat Model. Food Nutr. Res. 64. doi:10.29219/fnr.v64.4362
Zhao, Y., Wang, L., Wu, Y., Lu, Z., and Zhang, S. (2020). Genome-wide Study of Key Genes and Scoring System as Potential Noninvasive Biomarkers for Detection of Suicide Behavior in Major Depression Disorder. Bioengineered 11 (1), 1189–1196. doi:10.1080/21655979.2020.1831349
Zheng, R., He, J., Xiong, F., Wang, X., Han, L., and He, Y. (2021). Effects of Fuhe Decoction on the Behavior and Serum Monoamine Neurotransmitters of CUMS Combined with Orphan-Induced Depression Model Rats. Chin. Med. Her. 27 (05), 29–33.
Zhong, X., Li, G., Qiu, F., and Huang, Z. (2018). Paeoniflorin Ameliorates Chronic Stress-Induced Depression-like Behaviors and Neuronal Damages in Rats via Activation of the ERK-CREB Pathway. Front. Psychiatry 9, 772. doi:10.3389/fpsyt.2018.00772
Keywords: paeoniflorin, CUMS, metabolomics, SLC6A4, TNF, IL6, network analysis, Slc6a3
Citation: Lei C, Chen Z, Fan L, Xue Z, Chen J, Wang X, Huang Z, Men Y, Yu M, Liu Y and Chen J (2022) Integrating Metabolomics and Network Analysis for Exploring the Mechanism Underlying the Antidepressant Activity of Paeoniflorin in Rats With CUMS-Induced Depression. Front. Pharmacol. 13:904190. doi: 10.3389/fphar.2022.904190
Received: 25 March 2022; Accepted: 23 May 2022;
Published: 13 June 2022.
Edited by:
Tingting Zhou, Second Military Medical University, ChinaReviewed by:
Li-Na Gao, Jining Medical University, ChinaAmany Mohammed Gad, Sinai University, Egypt
Chunnian He, Chinese Academy of Medical Sciences and Peking Union Medical College, China
Peijun Ju, Shanghai Mental Health Center, China
Copyright © 2022 Lei, Chen, Fan, Xue, Chen, Wang, Huang, Men, Yu, Liu and Chen. This is an open-access article distributed under the terms of the Creative Commons Attribution License (CC BY). The use, distribution or reproduction in other forums is permitted, provided the original author(s) and the copyright owner(s) are credited and that the original publication in this journal is cited, in accordance with accepted academic practice. No use, distribution or reproduction is permitted which does not comply with these terms.
*Correspondence: Yueyun Liu, Y2hsb2Vsb3VAMTI2LmNvbQ==; Jiaxu Chen, Y2hlbmppYXh1QGhvdG1haWwuY29t