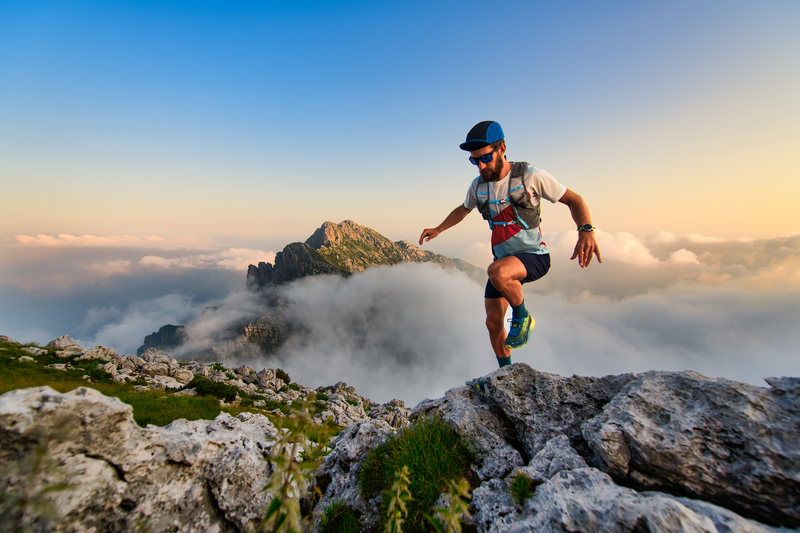
94% of researchers rate our articles as excellent or good
Learn more about the work of our research integrity team to safeguard the quality of each article we publish.
Find out more
ORIGINAL RESEARCH article
Front. Pharmacol. , 22 July 2022
Sec. Predictive Toxicology
Volume 13 - 2022 | https://doi.org/10.3389/fphar.2022.879907
This article is part of the Research Topic Methods and Application in Predictive Toxicology: 2021 View all 6 articles
Chemical structure-based read-across represents a promising method for chemical toxicity evaluation without the need for animal testing; however, a chemical structure is not necessarily related to toxicity. Therefore, in vitro studies were often used for read-across reliability refinement; however, their external validity has been hindered by the gap between in vitro and in vivo conditions. Thus, we developed a virtual DNA microarray, regression analysis–based inductive DNA microarray (RAID), which quantitatively predicts in vivo gene expression profiles based on the chemical structure and/or in vitro transcriptome data. For each gene, elastic-net models were constructed using chemical descriptors and in vitro transcriptome data to predict in vivo data from in vitro data (in vitro to in vivo extrapolation; IVIVE). In feature selection, useful genes for assessing the quantitative structure–activity relationship (QSAR) and IVIVE were identified. Predicted transcriptome data derived from the RAID system reflected the in vivo gene expression profiles of characteristic hepatotoxic substances. Moreover, gene ontology and pathway analysis indicated that nuclear receptor-mediated xenobiotic response and metabolic activation are related to these gene expressions. The identified IVIVE-related genes were associated with fatty acid, xenobiotic, and drug metabolisms, indicating that in vitro studies were effective in evaluating these key events. Furthermore, validation studies revealed that chemical substances associated with these key events could be detected as hepatotoxic biosimilar substances. These results indicated that the RAID system could represent an alternative screening test for a repeated-dose toxicity test and toxicogenomics analyses. Our technology provides a critical solution for IVIVE-based read-across by considering the mode of action and chemical structures.
Non-animal testing to assess the efficacy and safety of chemical substances is one of the key concepts in balancing animal welfare and efficient development. Since the marketing ban in the EU in March 2013 [(EC) No. 1223/2009] (EU, 2009) of cosmetic products and ingredients tested on animal models, safety assessment methodologies independent of animal testing have attracted much attention. Simultaneously, the utilization of non-animal high-throughput technology for optimizing drug discovery processes is becoming highly important in pharmaceuticals (Loiodice et al., 2017; Rognan, 2017; Amano et al., 2020).
Read-across, a process that estimates substance toxicity based on the concept that substances with similar chemical structures have similar biological activities, represents a promising approach and has already been conceptually accepted as a reliable safety risk assessment by some regulatory authorities (ECHA, 2017; European Commission, 2018). Likewise, quantitative structure–activity relationship (QSAR) has been widely used, and impurity characterization received regulatory acceptance (ICH M7). However, since subtle structural differences may elicit different biological responses, supporting the read-across robustness by using biological similarities has been considered important (Ball et al., 2016, 2020; Zhu et al., 2016). Registration, Evaluation, Authorization, and Restriction of Chemicals (REACH) mentions that the read-across performed by registrants often fails to comply with the legal requirements due to defects in the hypothesis and justification of toxicological prediction (ECHA, 2020).
There are two approaches to enhance the reliability of read-across: 1) employment of in vitro data relevant to specific toxicity. Methodologies to incorporate in vitro data within read-across (Ball et al., 2016, 2020; ECHA, 2017; Guo et al., 2019) and some case studies (OECD, 2016a, 2016b, 2018; Nakagawa et al., 2020, 2021) have been reported. However, these approaches can be applied only to specific toxicity end points and substances with a known toxicity and mode of action. Such conditions were previously termed as “local validity” (Patlewicz et al., 2014). 2) The use of biologically similar substances based on their profiles obtained from a large number of bioassays. The United States Environmental Protection Agency’s (US EPA’s) research project, ToxCast and Tox21, provided hundreds of high-throughput screening assays, and several groups employed such biological activity data for toxicological evaluation (Sipes et al., 2013; Berggren et al., 2015; Richard et al., 2021). Although this concept could be applied to substances with little information to elucidate their entire toxicological profiles and find their key mode of action, it is time-consuming and expensive to conduct numerous bioassays for a new candidate substance. By contrast, transcriptome data containing approximately 30,000 gene expression values can be used to estimate perturbated mechanisms through enrichment analysis. Wang et al. (2016) tried to predict drug-induced adverse effects by employing LINCS L1000 data (Subramanian et al., 2017), whereas Iwata et al. (2019) developed a computational method to predict missing values from the LINCS L1000 transcriptomic profiles of various human cell lines and provided new drug therapeutic indications. Genomic data have been considered to be usable in read-across by Health Canada and a research group from the US FDA (Health Canada, 2019; Liu et al., 2019). However, several researchers have shown that in vitro gene expression values are not always highly correlated with in vivo data (Sutherland et al., 2016; Grinberg et al., 2018; Liu et al., 2018). Thus, interpreting toxicological meaning from the in vitro–in vivo relationship and in vitro to in vivo extrapolation (IVIVE) in omics data represents a big challenge for chemical risk assessment. IVIVE was originally researched in toxicokinetics, such as in hepatic clearance and metabolites using hepatocytes (Soars et al., 2007; Umehara and Camenisch, 2012); most recent studies on non-animal testing have focused on predicting plasma concentrations, which is relevant for identification of a margin of exposure in risk assessment (Thomas et al., 2013a; Bell et al., 2018; Li et al., 2021). However, IVIVE should be considered for both toxicokinetics and toxicodynamics. Understanding of the in vitro to in vivo relationship of bioactivity data is also essential for non-animal testing. As an IVIVE study in omics data, Liu et al. (2020) developed a useful in silico strategy to narrow the data gap between in vitro and in vivo conditions. They modified in vitro data using non-generative matrix factorization methods to improve the correlation with in vivo data, which overcame the shortcomings of previous large-scale genomic data predictions regarding the in vitro–in vivo data gap (Liu et al., 2020). Although non-generative matrix factorization enables macroscopic estimation based on a pattern recognition classifying chemical and biological responses, it does not focus on estimation of each gene. As an alternative solution, microscopic estimation of each gene expression was performed based on tensor-train weighted optimization using machine learning (Iwata et al., 2019); however, such comprehensive estimations have not been integrated within an IVIVE study. Therefore, predicting in vivo transcriptomic profiles from in vitro data for IVIVE might not only enhance the robustness of read-across but could also be utilized in other non-animal testing strategies as weight of evidence, such as in Integrated Approaches to Testing and Assessment (IATA) and new approach methods (NAMs) for safety and drug repositioning research.
In this study, we developed a virtual DNA microarray that quantitatively predicts the in vivo gene expression profiles based on the chemical structure and/or in vitro transcriptome data. For each gene, elastic-net models, a regression analysis method that has been used in toxicity prediction with visualization of feature importance (e.g., Fujita et al., 2020), were constructed using chemical descriptors and in vitro transcriptome data. We named the set of prediction models “regression analysis–based inductive DNA microarray (RAID),” which inductively analyzes the mode of action and the key event in adverse effects with reference to the redundant arrays of inexpensive disks (also represented as RAID), a data storage virtualization technology that combines multiple physical disk drive components with the purpose of data redundancy. As RAID (storage technology) complements data based on information of multiple components, we hope that RAID (our microarray) will complement the relationships between multiple media (in vivo gene expression, in vitro gene expression, and chemical structure). Our RAID system achieved a quantitative in vitro to in vivo extrapolation (QIVIVE) by the integration of a structure-based approach (QSAR) with transcriptomic data. Whereas general “Q”IVIVE studies predict dose (or concentration) quantitatively in toxicological or toxicokinetic effects, our “Q”IVIVE predicts in vivo gene expression values quantitatively. Finally, the substance similarities were analyzed by principal component analysis (PCA), which proved useful in understanding the features of toxic substances based on their gene expression profile (Watanabe et al., 2012), using RAID (the virtual microarray) data, in vivo data, in vitro data, and chemical structure data to validate the usefulness of read-across.
No animal experiment was performed in this study. The transcriptome data from DNA microarrays (Affymetrix Rat Genome 230 2.0 chips; Santa Clara, CA, United States) were extracted from the Toxicogenomics Project-Genomics Assisted Toxicity Evaluation system (TG-GATEs). TG-GATEs contains in vitro and in vivo transcriptome data for rat single- and repeated-dose toxicity tests of 170 compounds (Igarashi et al., 2015). The transcriptome data obtained from the livers of rats treated with high doses for 28 days and primary rat hepatocytes treated with high doses for 24 h were downloaded and preprocessed using MAS5 (Gautier et al., 2004). In this study, chemical substances tested in vitro and in vivo those fulfilled a maximum sample number (n = 2 for in vitro and n = 3 for in vivo) and had no incalculable chemical descriptors (described below) were analyzed. Thus, 115 compounds were examined in this study (Table 1).
For the chemical structure data, the alvaDesc chemical descriptors (Mauri, 2020) were calculated using alvaDesc v1.0 software (Alvascience Srl, Lecco, Italy). AlvaDesc can calculate 3,885 2D-descriptors and 1,420 3D-descriptors. However, only 2D-descriptors were used, excluding those with a high pair correlation (>0.95), constant for all substances, and at least one missing value. Consequently, 854 descriptors were calculated. Each descriptor was normalized using the bestNormalize package (ver. 1.8.0) in R (ver. 4.1.1) (https://cran.r-project.org/). This package estimates the optimal normalizing transformation from the Yeo–Johnson transformation, the Box Cox transformation, the log10 transformation, the square root transformation, and the arcsine transformation.
To extrapolate in vitro transcriptome data to in vivo conditions, we developed predictive models for each gene. The predictive models predicting in vivo transcriptome data from chemical descriptors and in vitro data were developed using the elastic net regression method. The value of each cell in the matrix was the fold change on a base 2 logarithmic scale. The set of those predictive models was named a virtual microarray “RAID” (as mentioned in the Introduction section) (Figure 1). To suppress overlearning, the hyperparameters (α and λ) of each model were optimized with a 5-fold cross-validation. We removed the genes that were associated with less than 10 chemical substances inducing differential expression (<1.5-fold change) since it would be difficult to run machine learning scripts on such rare genes. Consequently, RAID was composed of 1,601 prediction models for each gene.
FIGURE 1. Development and implementation of a virtual microarray (RAID) for read-across. GE: gene expression. f(x): predictive models (formula). (A) RAID system development. The predictive model for in vivo transcriptome data for each gene was individually constructed by elastic net regression employing chemical descriptors and in vitro data. The models constructed were defined as a RAID system (a virtual microarray). (B) Workflow of safety evaluation using the RAID system. Chemical descriptors and in vitro gene expression data were inputted to the RAID system and in vivo gene expression data were outputted. The predicted results were analyzed by PCA and enrichment analysis for read-across. This procedure would replace toxicogenomics analysis in in vivo repeated dose study.
To construct RAID that correctly predicts the bioactivities of chemical substances, the quality of training data sets was extremely important, and differentially expressed genes should be determined strictly considering data noise. Hence, we addressed this issue by data processing (feature engineering) and model justification. First, after calculating the fold change values (sample treated groups/solvent control group), the gene differentiation values with low reliability were adjusted. Briefly, the fold change value increments were changed to half (e.g., 1.5 decreased to 1.25) in the sample with the number of flag A (low reliability) ≥2 out of 3 for in vivo and the number of flag A ≥1 out of 2 for in vitro, or in the sample with p-values ranging between 0.05 and 0.1. The fold change values were changed to one-fourth (e.g., 1.4 decreased to 1.1) in the sample with p-value over 0.1 and were treated as 1 (no differentiation) in the sample with flags all A in both in vivo and in vitro. Second, the weight parameters were used in model building. The weight of samples with ≥1.5-fold change was set to 1.5 and ≥4-fold change was set to 2.
Considering the application of RAID to read-across, the gene expression data were visualized by PCA using prcomp function from stats package (ver. 4.1.1), and the probability ellipse frames of toxic and nontoxic substances were drawn using the ggfortify package (ver. 0.4.12) in R to compare in vivo, in vitro, and chemical descriptor data. The toxic class of chemical substances was determined based on previously reported histopathological and serum chemistry findings (Table 1) (Low et al., 2011). Since PCA did not use the toxicity label for classification, partial least squares discriminant analysis (PLS-DA) using the hepatotoxicity label was also conducted to confirm predictive performance (see Supplementary Material). As a reference data point, the biological meaning of genes that contributed to the PCA plot of in vivo data was analyzed using pathway analysis. The loading value of genes in the PCA was defined as length of loadings calculated using the Pythagorean theorem:
and genes with the top 30 loading values in the first and fourth quadrant were analyzed.
To analyze the biological consistency with in vivo data, commonality of principal component–related genes (top and bottom 30 rotations in each PC1 and PC2 of PCA) were visualized using the VennDiagram package (ver. 1.6.20) in R, and enrichment analyses of each categorized gene were conducted using Gene Ontology—biological process and Reactome pathway by Metascape (Zhou et al., 2019). Four categorized genes related to in vivo data (in vivo only, in vivo and RAID, in vivo and in vitro, and all three data) were analyzed to characterize which biological process could be covered by RAID and in vitro data. Furthermore, to characterize genes whose predictive models in RAID used in vitro data, enrichment analysis of the top 20 genes with the highest importance (contribution) for in vitro data in the model was conducted. In the analysis, the Affymetrix probe ID was converted to gene symbol using the biomaRt package (ver. 2.50.2) in R.
For performance evaluation against the quantitative IVIVE, root-mean-square errors (RMSEs) of RAID predicted values to in vivo data were calculated and compared to those of in vitro data. To exclude the differences in gene expression value distribution of each data source, the fold change values were normalized before the RMSEs were calculated. The RMSEs were calculated both for all genes and genes for which in vitro data had importance in the model.
To validate the usefulness of RAID for functional read-across–based analysis of both predicted gene expression profiles and chemical structures, substances that did not contain training data sets for model building (Table 1) were further explored using Ingenuity Pathway Analysis (IPA) (QIAGEN Inc., https://www.qiagenbioinformatics.com/products/ingenuitypathway-analysis). Specifically, substances that may promote the expression of genes (having a known relationship with the gene) that were identified by the PCA and pathway analysis of in vivo data (see the Interpretation of Biological Meaning of Regression Analysis–Based Inductive DNA Microarray Analysis section) were explored using IPA. Chemical descriptors of each substance were analyzed using the alvaDesc v1.0 software (Alvascience Srl, Lecco, Italy), and the gene expression profiles were fulfilled using median values of training data sets. Finally, RAID analyses using constructed predictive models for those substances and reanalyzed PCA data were used to evaluate similarities based on the predicted biological responses.
RAID (predicted transcriptome) data were visualized using PCA (Figure 2). From a higher perspective, two directions mainly composed of toxic substances were identified, and many toxic substances were separated from non-toxic substances via RAID and in vivo data, whereas they could not be separated based on in vitro and chemical descriptor data. Moreover, two common toxic substances groups [e.g., first group (TAA, MP, and HCB) and second group (WY, FFB, BBr, and GFZ) placed in the first and fourth quadrants) were distanced from non-toxic substances along PC1 and PC2 in both RAID and in vivo data, nonetheless the PC1 and PC2 were replaced. The loading plot showed that Cyp1a1 (cytochrome P450, family 1, subfamily A, polypeptide 1), Gpx2 (glutathione peroxidase 2), and Gsta3 (glutathione S-transferase A3) gene expressions were commonly observed in RAID and in in vivo data and enabled the discrimination of TAA, MP, and HCB. Furthermore, Acot1 (acyl-CoA thioesterase 1), Vnn1 (vanin 1), and Cyp4a11 (cytochrome P450, family 4, subfamily A, polypeptide 11) contributed to discriminating WY, FFB, BBr, and GFZ.
FIGURE 2. PCA score plots for chemical substances and the gene loading in the transcriptome data of (A) in vivo, (B) virtual microarray (RAID), and (C) in vitro data. PCA score plot with (D) chemical descriptor data. Uppercase letters in PCA score plots: abbreviations of chemical substances are described in Table 1. Blue: nontoxic substances. Red: hepatotoxic substances. Gene symbols are presented on the arrowhead (loading).
Pathway analysis indicated that the first group–related genes would be associated with a xenobiotic response, such as Cyp1a induction via aryl hydrocarbon receptor (AHR) and carcinogenesis (Figure 3A), and the second group–related genes would be associated with peroxisome proliferative activity characterized by Cyp4a induction via peroxisome proliferator–activated receptor-alpha (PPARa) activation (Figure 3B). To clarify the biological functions that RAID covers, the commonalities between the related genes and principal components were explored (Figure 4 and Table 2). As expected from Figure 2, RAID shared more genes (36; Table 2) with the in vivo data than with the in vitro data (9). Enrichment analysis revealed that the biological processes related to metabolism and detoxification and pathways associated with peroxisomal protein transport were enriched in both in vivo and RAID data, indicating that RAID could cover these functions, and ultimately indicate key functions through pathway analysis (Figure 3). Conversely, although several metabolic processes were enriched within the in vitro data, those biological functions were covered by RAID as well (Figure 4). These results suggest that RAID data allow the detection of more in vivo key toxic events than in vitro transcriptome data.
FIGURE 3. List of genes that have high loading values in the (A) fourth quadrant and (B) first quadrant in the PCA plot of in vivo data, where the first group (TAA, MP, and HCB) and the second group (WY, FFB, BBr, and GFZ) plotted, and their pathway map. The loading value was defined as the loading length in the first or fourth quadrant calculated using the Pythagorean theorem. The pathway map was drawn by upstream regulator analysis using IPA.
FIGURE 4. Commonalities of principal component–related genes and their biological functions analyzed by gene ontology and pathway analyses. Venn diagram of genes related to the first and second principal components of in vivo, a virtual microarray (RAID), and in vitro data.
TABLE 2. Principal components relating common genes in a virtual microarray (RAID) and in vivo data.
For performance confirmation of discriminative analysis for hepatotoxicity, PLS-DA using RAID data allowed us to separate toxic chemicals with high accuracy (Supplementary Table S1). The accuracy using RAID data was better than that without RAID, when calibration and test data set were prepared.
Enrichment analysis of genes whose predictive model used highly relevant in vitro data (top 20 genes for which in vitro data had high importance in all predictive models; Table 3) indicated that in vitro data contributed to estimating the gene expression values associated with metabolic processes of fatty acids, xenobiotics, and drugs and peroxisome proliferative activity (pathway on peroxisome protein import and biological processes associated with the regulation of peroxisome size; Figure 5).
FIGURE 5. Enrichment analysis of in vitro–in vivo extrapolation (IVIVE)–related genes identified in a virtual microarray (RAID) system. Top 20 most important (contribution) genes from the predictive models were analyzed.
To evaluate RAID performance in terms of gene expression values, the RMSEs were calculated for all genes and the genes for which in vitro data had importance in predictive models. Considering RAID would be used in read-across, we compared the RMSEs of RAID data with those of in vitro data, from conventional non-animal test approaches (Figure 6). The RMSEs were lower in RAID, indicating a better performance than what could be obtained using in vitro data.
FIGURE 6. Distribution of RMSEs of a virtual microarray (RAID) and in vitro data of (A) all genes and (B) in vitro genes having importance (contribution) in predictive models. **p < 0.01 (Welch’s t-test).
In PCA with in vivo and RAID data, as well as the pathway analysis of PC-related genes (Figures 2, 3), the genes related to peroxisome proliferative activity and xenobiotic metabolism activity possibly leading to liver cancer, which were respectively characterized by Cyp1a induction via AHR and Cyp4 induction via PPARa, were identified as key features. Thus, potential Cyp1a and Cyp4a inducers were explored using the knowledge-based approach using the IPA software. Moreover, using the top 30 genes identified using PCA (described in the Interpretation of Biological Meaning of Regression Analysis–Based Inductive DNA Microarray Analysis section), upstream regulator analysis focusing on chemical substances was performed, and 20 chemicals were identified. Finally, a total of 21 chemicals (potential Cyp1a inducers: 10 chemicals; potential Cyp4a inducers: 11 chemicals) were selected as candidates for external validation and were subjected to RAID analyses (Table 4). Substances already present in the TG-GATE (training sets) or had uncalculated chemical descriptors data were excluded.
For the PCA, approximately half of the substances were plotted with positive PC scores, which was consistent with the direction expected from the training data set for both potential Cyp1a and Cyp4a inducers (Figure 7). Lastly, pentachlorobiphenyl, polychlorinated biphenyls, and pentachlorodibenzofuran were isolated as Cyp1a inducers, whereas nafenopin, ciprofibrate, and di(2-ethylhexyl)phthalate were isolated as Cyp4a inducers.
FIGURE 7. Read-across using PCA plot of external data predicted by a virtual microarray (RAID). (A) Cyp1a and (B) Cyp4a inducing chemical substances were analyzed for validation.
The transcriptome data signatures derived from the RAID (the virtual microarray) system were in good agreement with those of in vivo data, and the technology provided an understanding of the features of hepatotoxic substances based on the toxicological mechanism interpretation. The mechanism of action of the two characteristic toxic substances separated using PCA analysis was shown to be achieved through Cyp1a induction via AHR and Cyp4a induction via PPARa (pathway and gene ontology analysis). The AHR-induced drugs raise safety concerns during developmental periods (Qin et al., 2019), and PPARa-induced drug toxicity requires species differentiation considerations (Ito et al., 2006). Therefore, predicting the involvement of these nuclear receptors and induction of metabolic enzymes is critical for understanding the molecular initiating events and the key events associated with adverse outcome pathway. RAID enables the prediction of gene expression levels, thus exhibiting properties required for the next-generation risk assessment methods.
The first substance group (TAA, MP, and HCB), representing toxic substances commonly differentiated from non-toxic substances using PCA on in vivo and RAID data, has been reported to have carcinogenicity with metabolic activation (Uehara et al., 2008; Hajovsky et al., 2012; US HSS, 2015). Furthermore, these substances have been shown to activate xenobiotic-related receptors, such as AHR inducing Cyp1a (Ushel et al., 2002; Yamashita et al., 2014; Clara et al., 2015). Moreover, in vivo transcriptome data in this study showed that TAA, MP, and HCB induce Cyp1a activation. AHR is known for mediating the toxicity and tumor promoting properties despite the mechanism through which AHR activates carcinogenesis needing to be elucidated (Safe et al., 2013; Murray et al., 2014).
The second substance group (WY, FFB, BBr, and GFZ) includes fibrates which are recognized as PPARa agonists (Schoonjans et al., 1996), implying that induction of Cyp4a via PPARa and perturbation of lipid-related genes are involved as a series of key events. Although another fibrate included in training data—clofibrate (CFB)—was classified as a non-toxic substance according to no serum chemistry findings from a previous study, CFB was shown to act as a PPARa agonist inducing peroxisomal proliferation on hepatocytes (Low et al., 2011) and was plotted around the second group in the PCA. Sustained activation of PPARa signaling and induction of enzymes, such as CYP4A, to increased fatty acid oxidation contributes to sustained oxidative stress in the liver. These changes lead to liver cell damage as hypertrophy and proliferation which contribute to the development of hepatocellular carcinoma (Parimal et al., 2013).
From the perspective of capturing individual gene responses, RAID could detect gene expressions related to major drug metabolism responses in in vivo more broadly (more common principal component–related gene number; Figure 4) and quantitatively (lower RMSE values; Figure 6) than in vitro. The 36 genes that were commonly related to the principal components of in vivo and RAID data contained genes that were known to be involved in drug metabolism and hepatotoxicity. In addition to the genes described above (Cyp1a and Cyp4a), Acot1 acts as an auxiliary enzyme in the oxidation process of various lipids in peroxisomes (Hunt et al., 2012). Furthermore, Vnn1 is expressed by the centrilobular hepatocytes and is involved in lipid and xenobiotic metabolism (Bartucci et al., 2019), whereas Pex11a (peroxisomal biogenesis factor 11 alpha) is involved in peroxisome maintenance and proliferation associated with dyslipidemia (Chen et al., 2018). All of these genes are known as PPARa target genes (Rakhshandehroo et al., 2010; Lake et al., 2016). Thus, these features indicate that RAID can predict possible toxicity by taking into account a broader range of mechanisms than the range of in vitro data. Indeed, the in vivo changes detected using the in vitro data were limited (Figure 4), and the PCA showed that most of the differentially expressed genes were associated with irrelevant nonphysiological conditions. Thus, the IVIVE effect combining the QSAR technique and in vitro data would allow for more precise predictions through de-noising these types of in vitro specific biological responses.
In vitro data contribute to accurate gene expression predictions that could not be achieved with QSAR alone (Figure 2D). In vitro data contributed to the prediction of the mechanism shown in Figure 5. The biological mechanisms related to metabolic processes were consistent with the key mechanisms of characteristic hepatotoxic substances described above, which indicates that in vitro data contributes to the precise predictions obtained using RAID. In addition, whether in vitro responses were observed in the suggested mode of action predicted by the RAID system or not is an important point in terms of weight of evidence. This study provides valuable evidence supporting that transcriptome data should be considered in light of previous reports indicating that in vitro data does not necessarily reflect in vivo conditions (Tamura et al., 2006; Sutherland et al., 2016). Simultaneously, in vitro studies focusing on a specific mechanism should consider the external validity of their findings and whether the findings reflect in vivo situations.
Evaluating the read-across performance using external substances, such as 3,4,5,3′,4′-pentachlorobiphenyl, 2,2′,4,4′-tetrachlorobiphenyl (a type of polychlorinated biphenyl) and pentachlorodibenzofuran (dioxin-like compounds) (Figure 7A), which are known as IARC group 1 carcinogens and Cyp1a1 inducers (EPA,U S, 1996; Walker et al., 2005; National; Toxicology Program, 2006); these were separated as toxic substances. Additionally, benzo(a)pyrene, 3-methylcholanthrene, and 9,10-dimethyl-1,2-benzanthracene plotted apart from the origin of coordinates (PC1 = 0 and PC2 = 0), and are polycyclic aromatic hydrocarbons inducing Cyp1a1 (Moorthy et al., 2007; Pushparajah et al., 2008). Non-carcinogenic chemical substances, such as food components or preservatives, were positioned near the origin, second quadrant or third quadrant, indicating low risk. Furthermore, substances interacting with Cyp4a (Figure 7B), such as ciprofibrate, nafenopin, clofenapate, clofibric acid, and di(2-ethylhexyl) phthalate, which plotted in the area of the 2nd substance group (PC1 > 0), are also known as PPARa agonists (Bocos et al., 1995; Roberts et al., 2002; Yadetie et al., 2003; Currie et al., 2005; Pyper et al., 2010). Chemicals that were not characterized by the PC1 component (PC1 < 0) are not hyperlipidemia drugs. These results suggest that the RAID system effectively classifies substances based on their mode of action as well as the strength of their toxicity, and ultimately contributes to precise read-across. Thus, the RAID system provides a new method for read-across in line with IATA that should be called “a virtual functional read-across”. Here, we showed that substances without high structural similarities might have similar toxicological properties, and our new approach interpreted the shared mechanism of action. This means that RAID considers the qualitative and quantitative similarities of biological responses, which was one of the major issues of QSAR-based read-across. The structural similarities of TAA, MP, and HCB observed using correlation coefficients of the chemical descriptor used for the predictive model, and the maximum common substructure (MCS) similarities with the Tanimoto coefficient, were less than 0.5; however, the homology of RAID and in vivo data was as high as a 0.8 Pearson’s correlation coefficient. Furthermore, achieving such an accurate read-across without using in vitro data will provide a new perspective on the structural information-based predictions.
PCA analysis was used to understand the features of substances to predict the modes of action and identify biologically similar substances for read-across in this study. The examples of applications of RAID for read-across described above were compared to other methods (Table 5). The RAID system could enhance read-across reliability by estimating toxicity including modes of action, while this was difficult by other methods (e.g., QSAR or read-across using chemical structure data) (Supplementary Figure S1). On the other hand, focusing on certain specific toxicities, discriminant analysis, classifier model, or biomarker analysis might improve the separation of toxic substances. Indeed, as shown in Supplementary Table S1, when the RAID system was applied to discriminating hepatotoxicity, PLS-DA using RAID data showed a good predictive performance, indicating usefulness for the specified toxicity prediction. Thus, the use of RAID data instead of experimental transcriptome data would achieve previously reported biomarker-based classification without using animals. For example, Liu et al. (2017) indicated that certain genes were associated with hepatocellular hypertrophy and hepatocarcinogenesis, as well as markers such as Cyp1a1, Acot1, Stac3 (SH3 and cysteine rich domain 3), and Hdc (histidine decarboxylase), which were correctly evaluated in the present study to characterize hepatotoxic substances. Similarly, the constructed RAID system could be applied to previous studies to predict carcinogenicity or estimate transcriptional benchmark dose by toxicogenomics analysis of short term in vivo studies (Ellinger-ziegelbauer et al., 2008; Thomas et al., 2013b; Matsumoto et al., 2014; Kawamoto et al., 2017).
One important issue that should be considered in toxicological evaluation using the RAID system is consideration of species differences. The RAID system provides mechanistic insights on repeated-dose toxicity in animal models; however, since some species differences have been observed, the suggested mode of action and the corresponding molecules need to be confirmed by toxicologists. The interspecies extrapolations, such as rat-to-human extrapolations, could be achieved by further experiments to construct new RAID systems with these different species’ transcriptome data. In addition, RAID data of substances that were separated as toxic substances in PCA (e.g., TAA, MP, HCB, WY, FFB, BBr, and GFZ) showed high similarity to in vivo data (Supplementary Table S2). Since regression analysis requires certain levels of standard deviation of training data, the RAID accuracy for substances may be related to the number of substances with similar modes of action. Thus, database expansion for several substance groups with minor modes of action would contribute to further improving the accuracy and applicability domain. In addition, evaluation of RAID usefulness for various toxicities is required.
The present approach integrates QSAR and IVIVE and will contribute to other areas of research, such as drug repositioning, which has recently attracted attention toward pharmaceuticals that are available on the market and might be repurposed for new diseases (Jourdan et al., 2020). However, the previously proposed methodologies (Iwata et al., 2018; Lippmann et al., 2018; Zhu et al., 2020; He et al., 2021) have room for improving the IVIVE aspect of in vivo predictions. Thus, our system provides an alternative to screening candidate drugs and exploring new biologically similar drugs at a low cost.
In conclusion, we developed a virtual DNA microarray system that quantitatively predicts in vivo gene expression profiles based on the chemical structure and/or in vitro transcriptome data. Estimated transcriptomes are considered scientifically relevant from PCA data interpretation as well as pathway and GO analysis. Based on its external validation, our system works as an alternative test for repeated dose toxicity tests with toxicogenomics analysis enabling IVIVE and mechanism estimation. Although our technology might have limited applicability domain due to the small data size of chemical substances and their characteristics (using hepatotoxic substances), the concept of the virtual microarray analysis contributes to the 3Rs (reduction, refinement, and replacement) and might benefit much future animal testing.
Publicly available data sets were analyzed in this study. This data can be found at https://dbarchive.biosciencedbc.jp/en/open-tggates/download.html.
YA and HH contributed to the conception and design of the study. YA and HH constructed in silico models, performed enrichment analyses, interpreted the biological meanings of the models, and contributed to statistical analyses. HH collected the data sets from TG-GATE. HH and MY supervised this project. YA and HH drafted the manuscript. All authors contributed to manuscript writing, confirmed the final version of the manuscript, and agreed to the contents.
YA, MY, and HH were employed by the company Kao Corporation.
All claims expressed in this article are solely those of the authors and do not necessarily represent those of their affiliated organizations, or those of the publisher, the editors, and the reviewers. Any product that may be evaluated in this article, or claim that may be made by its manufacturer, is not guaranteed or endorsed by the publisher.
We thank Dr. Osamu Morita, Dr. Kaede Miyata, and Mr. Yasuaki Inoue for their helpful suggestions and valuable discussions to the present study.
The Supplementary Material for this article can be found online at: https://www.frontiersin.org/articles/10.3389/fphar.2022.879907/full#supplementary-material
Amano, Y., Honda, H., Sawada, R., Nukada, Y., Yamane, M., Ikeda, N., et al. (2020). In Silico systems for Predicting Chemical-Induced Side Effects Using Known and Potential Chemical Protein Interactions, Enabling Mechanism Estimation. J. Toxicol. Sci. 45, 137–149. doi:10.2131/jts.45.137
Ball, N., Cronin, M. T., Shen, J., Blackburn, K., Booth, E. D., Bouhifd, M., et al. (2016). Toward Good Read-Across Practice (GRAP) Guidance. ALTEX 33, 149–166. doi:10.14573/altex.1601251
Ball, N., Madden, J., Paini, A., Mathea, M., Palmer, A. D., Sperber, S., et al. (2020). Key Read across Framework Components and Biology Based Improvements. Mutat. Res. 853, 503172. doi:10.1016/j.mrgentox.2020.503172
Bartucci, R., Salvati, A., Olinga, P., and Boersma, Y. L. (2019). Vanin 1: Its Physiological Function and Role in Diseases. Int. J. Mol. Sci. 20, 3891. doi:10.3390/ijms20163891
Bell, S. M., Chang, X., Wambaugh, J. F., Allen, D. G., Bartels, M., Brouwer, K. L. R., et al. (2018). In Vitro to In Vivo Extrapolation for High Throughput Prioritization and Decision Making. Toxicol Vitro 47, 213–227. doi:10.1016/j.tiv.2017.11.016
Berggren, E., Amcoff, P., Benigni, R., Blackburn, K., Carney, E., Cronin, M., et al. (2015). Chemical Safety Assessment Using Read-Across: Assessing the Use of Novel Testing Methods to Strengthen the Evidence Base for Decision Making. Environ. Health Perspect. 123, 1232–1240. doi:10.1289/ehp.1409342
Bocos, C., Göttlicher, M., Gearing, K., Banner, C., Enmark, E., Teboul, M., et al. (1995). Fatty Acid Activation of Peroxisome Proliferator-Activated Receptor (PPAR). J. Steroid Biochem. Mol. Biol. 53, 467–473. doi:10.1016/0960-0760(95)00093-f
Chen, C., Wang, H., Chen, B., Chen, D., Lu, C., Li, H., et al. (2018). Pex11a Deficiency Causes Dyslipidaemia and Obesity in Mice. J. Cell. Mol. Med. 23, 2020–2031. doi:10.1111/jcmm.14108
Currie, R. A., Bombail, V., Oliver, J. D., Moore, D. J., Lim, F. L., Gwilliam, V., et al. (2005). Gene Ontology Mapping as an Unbiased Method for Identifying Molecular Pathways and Processes Affected by Toxicant Exposure: Application to Acute Effects Caused by the Rodent Non-genotoxic Carcinogen Diethylhexylphthalate. Toxicol. Sci. 86, 453–469. doi:10.1093/toxsci/kfi207
de Tomaso Portaz, A. C., Caimi, G. R., Sánchez, M., Chiappini, F., Randi, A. S., Kleiman de Pisarev, D. L., et al. (2015). Hexachlorobenzene Induces Cell Proliferation, and Aryl Hydrocarbon Receptor Expression (AhR) in Rat Liver Preneoplastic Foci, and in the Human Hepatoma Cell Line HepG2. AhR Is a Mediator of ERK1/2 Signaling, and Cell Cycle Regulation in HCB-Treated HepG2 Cells. Toxicology 336, 36–47. doi:10.1016/j.tox.2015.07.013
ECHA (2020). The Use of Alternatives to Testing on Animals for the REACH Regulation. doi:10.2823/092305
Ellinger-ziegelbauer, H., Gmuender, H., Bandenburg, A., and Ahr, H. J. (2008). Prediction of a Carcinogenic Potential of Rat Hepatocarcinogens Using Toxicogenomics Analysis of Short-Term In Vivo Studies. Mutat. Res. 637, 23–39. doi:10.1016/j.mrfmmm.2007.06.010
EPA, U. S (1996). PCBs: Cancer Dose-Response Assessment and Application to Environmental Mixtures, Washington, DC: U.S. Environmental Protection Agency, Office of Research and Development, National Center for Environmental Assessment.
EU (2009). Regulation (EC) No 1223/2009 of the European Parliament and of the Council of 30 November 2009 on Cosmetics Products. Off. J. Eur. Union L 342, 59–209.
European Commission (2018). The SCCS Notes of Guidance for the Testing of Cosmetic Ingredients and Their Safety Evaluation 10th Revision. Avaliable at: https://ec.europa.eu/health/system/files/2019-02/sccs_o_224_0.pdf.
Fujita, Y., Morita, O., and Honda, H. (2020). In Silico model for Chemical-Induced Chromosomal Damages Elucidates Mode of Action and Irrelevant Positives. Genes (Basel) 11, 1181. doi:10.3390/genes11101181
Gautier, L., Cope, L., Bolstad, B. M., and Irizarry, R. A. (2004). affy--analysis of Affymetrix GeneChip Data at the Probe Level. Bioinformatics 20, 307–315. doi:10.1093/bioinformatics/btg405
Grinberg, M., Stöber, R. M., Albrecht, W., Edlund, K., Schug, M., Godoy, P., et al. (2018). Toxicogenomics Directory of Rat Hepatotoxicants In Vivo and in Cultivated Hepatocytes. Arch. Toxicol. 92, 3517–3533. doi:10.1007/s00204-018-2352-3
Guo, Y., Zhao, L., Zhang, X., and Zhu, H. (2019). Using a Hybrid Read-Across Method to Evaluate Chemical Toxicity Based on Chemical Structure and Biological Data. Ecotoxicol. Environ. Saf. 178, 178–187. doi:10.1016/j.ecoenv.2019.04.019
Hajovsky, H., Hu, G., Koen, Y., Sarma, D., Cui, W., Moore, D. S., et al. (2012). Metabolism and Toxicity of Thioacetamide and Thioacetamide S-Oxide in Rat Hepatocytes. Chem. Res. Toxicol. 25, 1955–1963. doi:10.1021/tx3002719
Hamadeh, H. K., Knight, B. L., Haugen, A. C., Sieber, S., Amin, R. P., Bushel, P. R., et al. (2002). Methapyrilene Toxicity: Anchorage of Pathologic Observations to Gene Expression Alterations. Toxicol. Pathol. 30, 470–482. doi:10.1080/01926230290105712
He, B., Hou, F., Ren, C., Bing, P., and Xiao, X. (2021). A Review of Current In Silico Methods for Repositioning Drugs and Chemical Compounds. Front. Oncol. 11, 711225. doi:10.3389/fonc.2021.711225
Health Canada (2019). Evaluation of the Use of Toxicogenomics in Risk Assessment at Health Canada: An Exploratory Document on Current Health Canada Practices for the Use of Toxicogenomics in Risk Assessment. Avaliable at: https://www.canada.ca/en/health-canada/services/publications/science-research-data/evaluation-use-toxicogenomics-risk-assessment.html.
Hunt, M. C., Siponen, M. I., and Alexson, S. E. (2012). The Emerging Role of Acyl-CoA Thioesterases and Acyltransferases in Regulating Peroxisomal Lipid Metabolism. Biochim. Biophys. Acta 1822, 1397–1410. doi:10.1016/j.bbadis.2012.03.009
Igarashi, Y., Nakatsu, N., Yamashita, T., Ono, A., Ohno, Y., Urushidani, T., et al. (2015). Open TG-GATEs: A Large-Scale Toxicogenomics Database. Nucleic Acids Res. 43, D921–D927. doi:10.1093/nar/gku955
Ito, O., Nakamura, Y., Tan, L., Ishizuka, T., Sasaki, Y., Minami, N., et al. (2006). Expression of Cytochrome P-450 4 Enzymes in the Kidney and Liver: Regulation by PPAR and Species-Difference between Rat and Human. Mol. Cell. Biochem. 284, 141–148. doi:10.1007/s11010-005-9038-x
Iwata, M., Hirose, L., Kohara, H., Liao, J., Sawada, R., Akiyoshi, S., et al. (2018). Pathway-based Drug Repositioning for Cancers: Computational Prediction and Experimental Validation. J. Med. Chem. 61, 9583–9595. doi:10.1021/acs.jmedchem.8b01044
Iwata, M., Yuan, L., Zhao, Q., Tabei, Y., Berenger, F., Sawada, R., et al. (2019). Predicting Drug-Induced Transcriptome Responses of a Wide Range of Human Cell Lines by a Novel Tensor-Train Decomposition Algorithm. Bioinformatics 35, i191–i199. doi:10.1093/bioinformatics/btz313
Jourdan, J. P., Bureau, R., Rochais, C., and Dallemagne, P. (2020). Drug Repositioning: a Brief Overview. J. Pharm. Pharmacol. 72, 1145–1151. doi:10.1111/jphp.13273
Kawamoto, T., Ito, Y., Morita, O., and Honda, H. (2017). Mechanism-based Risk Assessment Strategy for Drug-Induced Cholestasis Using the Transcriptional Benchmark Dose Derived by Toxicogenomics. J. Toxicol. Sci. 42, 427–436. doi:10.2131/jts.42.427
Lake, A. D., Wood, C. E., Bhat, V. S., Chorley, B. N., Carswell, G. K., Sey, Y. M., et al. (2016). Dose and Effect Thresholds for Early Key Events in a PPARα-Mediated Mode of Action. Toxicol. Sci. 149, 312–325. doi:10.1093/toxsci/kfv236
Li, H., Yuan, H., Middleton, A., Li, J., Nicol, B., Carmichael, P., et al. (2021). Next Generation Risk Assessment (NGRA): Bridging In Vitro Points-Of-Departure to Human Safety Assessment Using Physiologically-Based Kinetic (PBK) Modelling - A Case Study of Doxorubicin with Dose Metrics Considerations. Toxicol. Vitro 74, 105171. doi:10.1016/j.tiv.2021.105171
Lippmann, C., Kringel, D., Ultsch, A., and Lötsch, J. (2018). Computational Functional Genomics-Based Approaches in Analgesic Drug Discovery and Repurposing. Pharmacogenomics 19, 783–797. doi:10.2217/pgs-2018-0036
Liu, S., Kawamoto, T., Morita, O., Yoshinari, K., and Honda, H. (2017). Discriminating between Adaptive and Carcinogenic Liver Hypertrophy in Rat Studies Using Logistic Ridge Regression Analysis of Toxicogenomic Data: The Mode of Action and Predictive Models. Toxicol. Appl. Pharmacol. 318, 79–87. doi:10.1016/j.taap.2017.01.006
Liu, Y., Jing, R., Wen, Z., and Li, M. (2020). Narrowing the Gap between In Vitro and In Vivo Genetic Profiles by Deconvoluting Toxicogenomic Data In Silico. Front. Pharmacol. 10, 1489. doi:10.3389/fphar.2019.01489
Liu, Z., Delavan, B., Roberts, R., and Tong, W. (2018). Transcriptional Responses Reveal Similarities between Preclinical Rat Liver Testing Systems. Front. Genet. 9, 74–10. doi:10.3389/fgene.2018.00074
Liu, Z., Huang, R., Roberts, R., and Tong, W. (2019). Toxicogenomics: A 2020 Vision. Trends Pharmacol. Sci. 40, 92–103. doi:10.1016/j.tips.2018.12.001
Loiodice, S., Nogueira da Costa, A., and Atienzar, F. (2017). Current Trends in In Silico, In Vitro Toxicology, and Safety Biomarkers in Early Drug Development. Drug Chem. Toxicol. 42, 113–121. doi:10.1080/01480545.2017.1400044
Low, Y., Uehara, T., Minowa, Y., Yamada, H., Ohno, Y., Urushidani, T., et al. (2011). Predicting Drug-Induced Hepatotoxicity Using QSAR and Toxicogenomics Approaches. Chem. Res. Toxicol. 24, 1251–1262. doi:10.1021/tx200148a
Matsumoto, H., Saito, F., and Takeyoshi, M. (2014). CARCINOscreen®: New Short-Term Prediction Method for Hepatocarcinogenicity of Chemicals Based on Hepatic Transcript Profiling in Rats. J. Toxicol. Sci. 39, 725–734. doi:10.2131/jts.39.725
Mauri, A. (2020). “alvaDesc: A Tool to Calculate and Analyze Molecular Descriptors and Fingerprints,” in Ecotoxicological QSARs. Methods in Pharmacology and Toxicology. Editor K. Roy (New York, NY): Humana), 801–820. doi:10.1007/978-1-0716-0150-1_32
Moorthy, B., Muthiah, K., Fazili, I. S., Kondraganti, S. R., Wang, L., Couroucli, X. I., et al. (2007). 3-Methylcholanthrene Elicits DNA Adduct Formation in the CYP1A1 Promoter Region and Attenuates Reporter Gene Expression in Rat H4IIE Cells. Biochem. Biophys. Res. Commun. 354, 1071–1077. doi:10.1016/j.bbrc.2007.01.103
Murray, I. A., Patterson, A. D., and Perdew, G. H. (2014). Aryl Hydrocarbon Receptor Ligands in Cancer: Friend and Foe. Nat. Rev. Cancer 14, 801–814. doi:10.1038/nrc3846
Nakagawa, S., Okamoto, M., Nukada, Y., and Morita, O. (2020). Comparison of the Potential Mechanisms for Hepatotoxicity of P-Dialkoxy Chlorobenzenes in Rat Primary Hepatocytes for Read-Across. Regul. Toxicol. Pharmacol. 113, 104617. doi:10.1016/j.yrtph.2020.104617
Nakagawa, S., Okamoto, M., Yoshihara, K., Nukada, Y., and Morita, O. (2021). Grouping of Chemicals Based on the Potential Mechanisms of Hepatotoxicity of Naphthalene and Structurally Similar Chemicals Using In Vitro Testing for Read-Across and its Validation. Regul. Toxicol. Pharmacol. 121, 104874. doi:10.1016/j.yrtph.2021.104874
OECD (2018). Case Study on Grouping and Read-Across for Nanomaterials ─ Genotoxicity of Nano-TiO2 (Series on Testing and Assessment No. 292), 1–56. Avaliable at: https://www.oecd.org/officialdocuments/publicdisplaydocumentpdf/?cote=ENV/JM/MONO(2018)28&docLanguage=En.
OECD (2016a). Case Study on the Use of an Integrated Approach to to Testing and Assessment for Hepatotoxicity of Allyl Esters, (Series on Testing and Assessment No. 253), 1–33. Avaliable at: https://www.oecd.org/officialdocuments/publicdisplaydocumentpdf/?cote=env/jm/mono(2016)51&doclanguage=en.
OECD (2016b). Case Study on the Use of Integrated Approaches for Testing and Assessment for in Vitro Mutagenicity of 3,3’ Dimethoxybenzidine (DMOB) Based Direct Dyes (Series on Testing and Assessment No. 251, 1–49. Avaliable at: https://www.oecd.org/officialdocuments/publicdisplaydocumentpdf/?cote=env/jm/mono(2016)49&doclanguage=en.
Parimal, M., Navin, V., and Janardan, K. R. (2013). “Peroxisomes and Their Key Role in Cellular Signaling and Metabolism,” in Peroxisome Proliferator-Activated Receptor-α Signaling in Hepatocarcinogenesis” in Peroxisomes and Their Key Role in Cellular Signaling and Metabolism. Editor L. A. del Río (Dordrecht: Springer), 69. doi:10.1007/978-94-007-6889-5
Patlewicz, G., Ball, N., Becker, R. A., Booth, E. D., Cronin, M. T., Kroese, D., et al. (2014). Read-across Approaches-Mmisconceptions, Promises and Challenges Ahead. ALTEX 31, 387–396. doi:10.14573/altex.1410071
Pushparajah, D. S., Umachandran, M., Nazir, T., Plant, K. E., Plant, N., Lewis, D. F., et al. (2008). Up-regulation of CYP1A/B in Rat Lung and Liver, and Human Liver Precision-Cut Slices by a Series of Polycyclic Aromatic Hydrocarbons; Association with the Ah Locus and Importance of Molecular Size. Toxicol Vitro 22, 128–145. doi:10.1016/j.tiv.2007.08.014
Pyper, S. R., Viswakarma, N., Yu, S., and Reddy, J. K. (2010). PPARalpha: Energy Combustion, Hypolipidemia, Inflammation and Cancer. Nucl. Recept. Signal. 8, e002. doi:10.1621/nrs.08002
Qin, C., Aslamkhan, A. G., Pearson, K., Tanis, K. Q., Podtelezhnikov, A., Frank, E., et al. (2019). AhR Activation in Pharmaceutical Development: Applying Liver Gene Expression Biomarker Thresholds to Identify Doses Associated with Tumorigenic Risks in Rats. Toxicol. Sci. 171, 4–55. doi:10.1093/toxsci/kfz125
Rakhshandehroo, M., Knoch, B., Müller, M., and Kersten, S. (2010). Peroxisome Proliferator-Activated Receptor Alpha Target Genes. PPAR Res. 2010, 1–20. doi:10.1155/2010/612089
Richard, A. M., Huang, R., Waidyanatha, S., Shinn, P., Collins, B. J., Thillainadarajah, I., et al. (2021). The Tox21 10K Compound Library: Collaborative Chemistry Advancing Toxicology. Chem. Res. Toxicol. 34, 189–216. doi:10.1021/acs.chemrestox.0c00264
Roberts, R. A., Chevalier, S., Hasmall, S. C., James, N. H., Cosulich, S. C., and Macdonald, N. (2002). PPAR Alpha and the Regulation of Cell Division and Apoptosis. Toxicology 181-182, 167–170. doi:10.1016/s0300-483x(02)00275-5
Rognan, D. (2017). The Impact of In Silico Screening in the Discovery of Novel and Safer Drug Candidates. Pharmacol. Ther. 175, 47–66. doi:10.1016/j.pharmthera.2017.02.034
Safe, S., Lee, S. O., and Jin, U. H. (2013). Role of the Aryl Hydrocarbon Receptor in Carcinogenesis and Potential as a Drug Target. Toxicol. Sci. 135, 1–16. doi:10.1093/toxsci/kft128
Schoonjans, K., Staels, B., and Auwerx, J. (1996). Role of the Peroxisome Proliferator-Activated Receptor (PPAR) in Mediating the Effects of Fibrates and Fatty Acids on Gene Expression. J. Lipid Res. 37, 907–925. doi:10.1016/S0022-2275(20)42003-6
Sipes, N. S., Martin, M. T., Kothiya, P., Reif, D. M., Judson, R. S., Richard, A. M., et al. (2013). Profiling 976 ToxCast Chemicals across 331 Enzymatic and Receptor Signaling Assays. Chem. Res. Toxicol. 26, 878–895. doi:10.1021/tx400021f
Soars, M. G., McGinnity, D. F., Grime, K., and Riley, R. J. (2007). The Pivotal Role of Hepatocytes in Drug Discovery. Chem. Biol. Interact. 168, 2–15. doi:10.1016/j.cbi.2006.11.002
Subramanian, A., Narayan, R., Corsello, S. M., Peck, D. D., Natoli, T. E., Lu, X., et al. (2017). A Next Generation Connectivity Map: L1000 Platform and the First 1,000,000 Profiles. Cell. 171, 1437–e17. doi:10.1016/j.cell.2017.10.049
Sutherland, J. J., Jolly, R. A., Goldstein, K. M., and Stevens, J. L. (2016). Assessing Concordance of Drug-Induced Transcriptional Response in Rodent Liver and Cultured Hepatocytes. PLoS Comput. Biol. 12, e1004847–31. doi:10.1371/journal.pcbi.1004847
Tamura, K., Ono, A., Miyagishima, T., Nagao, T., and Urushidani, T. (2006). Profiling of Gene Expression in Rat Liver and Rat Primary Cultured Hepatocytes Treated with Peroxisome Proliferators. J. Toxicol. Sci. 31, 471–490. doi:10.2131/jts.31.471
Thomas, R. S., Philbert, M. A., Auerbach, S. S., Wetmore, B. A., Devito, M. J., Cote, I., et al. (2013a). Incorporating New Technologies into Toxicity Testing and Risk Assessment: Moving from 21st Century Vision to a Data-Driven Framework. Toxicol. Sci. 136, 4–18. doi:10.1093/toxsci/kft178
Thomas, R. S., Wesselkamper, S. C., Wang, N. C., Zhao, Q. J., Petersen, D. D., Lambert, J. C., et al. (2013b). Temporal Concordance between Apical and Transcriptional Points of Departure for Chemical Risk Assessment. Toxicol. Sci. 134, 180–194. doi:10.1093/toxsci/kft094
Toxicology Program, National. (2006). NTP Toxicology and Carcinogenesis Studies of 3,3',4,4',5-pentachlorobiphenyl (PCB 126) (CAS No. 57465-28-8) in Female Harlan Sprague-Dawley Rats (Gavage Studies). Natl. Toxicol. Program Tech. Rep. Ser. 520, 4–246.
Uehara, T., Kiyosawa, N., Hirode, M., Omura, K., Shimizu, T., Ono, A., et al. (2008). Gene Expression Profiling of Methapyrilene-Induced Hepatotoxicity in Rat. J. Toxicol. Sci. 33, 37–50. doi:10.2131/jts.33.37
Umehara, K., and Camenisch, G. (2012). Novel In Vitro-In Vivo Extrapolation (IVIVE) Method to Predict Hepatic Organ Clearance in Rat. Pharm. Res. 29, 603–617. doi:10.1007/s11095-011-0607-2
Walker, N. J., Crockett, P. W., Nyska, A., Brix, A. E., Jokinen, M. P., Sells, D. M., et al. (2005). Dose-additive Carcinogenicity of a Defined Mixture of "Dioxin-like Compounds". Environ. Health Perspect. 113, 43–48. doi:10.1289/ehp.7351
Wang, Z., Clark, N. R., and Ma'ayan, A. (2016). Drug-induced Adverse Events Prediction with the LINCS L1000 Data. Bioinformatics 32, 2338–2345. doi:10.1093/bioinformatics/btw168
Watanabe, T., Suzuki, T., Natsume, M., Nakajima, M., Narumi, K., Hamada, S., et al. (2012). Discrimination of Genotoxic and Non-genotoxic Hepatocarcinogens by Statistical Analysis Based on Gene Expression Profiling in the Mouse Liver as Determined by Quantitative Real-Time PCR. Mutat. Res. 747, 164–175. doi:10.1016/j.mrgentox.2012.04.011
Yadetie, F., Laegreid, A., Bakke, I., Kusnierczyk, W., Komorowski, J., Waldum, H. L., et al. (2003). Liver Gene Expression in Rats in Response to the Peroxisome Proliferator-Activated Receptor-Alpha Agonist Ciprofibrate. Physiol. Genomics 15, 9–19. doi:10.1152/physiolgenomics.00064.2003
Yamashita, Y., Ueyama, T., Nishi, T., Yamamoto, Y., Kawakoshi, A., Sunami, S., et al. (2014). Nrf2-inducing Anti-oxidation Stress Response in the Rat Liver-Nnew Beneficial Effect of Lansoprazole. PLoS One 9, e97419. doi:10.1371/journal.pone.0097419
Zhou, Y., Zhou, B., Pache, L., Chang, M., Khodabakhshi, A. H., Tanaseichuk, O., et al. (2019). Metascape Provides a Biologist-Oriented Resource for the Analysis of Systems-Level Datasets. Nat. Commun. 10, 1523. doi:10.1038/s41467-019-09234-6
Zhu, H., Bouhifd, M., Donley, E., Egnash, L., Kleinstreuer, N., Kroese, E. D., et al. (2016). Supporting Read-Across Using Biological Data. ALTEX 33, 167–182. doi:10.14573/altex.1601252
Keywords: oligonucleotide array, gene expression analysis, hepatotoxicity, alternative method, new approach methodology
Citation: Amano Y, Yamane M and Honda H (2022) RAID: Regression Analysis–Based Inductive DNA Microarray for Precise Read-Across. Front. Pharmacol. 13:879907. doi: 10.3389/fphar.2022.879907
Received: 20 February 2022; Accepted: 30 May 2022;
Published: 22 July 2022.
Edited by:
Xiaohui Fan, Zhejiang University, ChinaReviewed by:
Romualdo Benigni, National Institute of Health (NIH) (ISS), ItalyCopyright © 2022 Amano, Yamane and Honda. This is an open-access article distributed under the terms of the Creative Commons Attribution License (CC BY). The use, distribution or reproduction in other forums is permitted, provided the original author(s) and the copyright owner(s) are credited and that the original publication in this journal is cited, in accordance with accepted academic practice. No use, distribution or reproduction is permitted which does not comply with these terms.
*Correspondence: Hiroshi Honda, aG9uZGEuaGlyb3NoaUBrYW8uY29t
Disclaimer: All claims expressed in this article are solely those of the authors and do not necessarily represent those of their affiliated organizations, or those of the publisher, the editors and the reviewers. Any product that may be evaluated in this article or claim that may be made by its manufacturer is not guaranteed or endorsed by the publisher.
Research integrity at Frontiers
Learn more about the work of our research integrity team to safeguard the quality of each article we publish.