- 1Key Laboratory of Animal Disease Diagnostics and Immunology, Ministry of Agriculture, MOE International Joint Collaborative Research Laboratory for Animal Health and Food Safety, College of Veterinary Medicine, Nanjing Agricultural University, Nanjing, China
- 2Jiangsu Co-Innovation Center for Prevention and Control of Important Animal Infectious Diseases and Zoonoses, Yangzhou, China
Porcine epidemic diarrhea virus (PEDV) is an alphacoronavirus (α-CoV) that causes high mortality in suckling piglets, leading to severe economic losses worldwide. No effective vaccine or commercial antiviral drug is readily available. Several replicative enzymes are responsible for coronavirus replication. In this study, the potential candidates targeting replicative enzymes (PLP2, 3CLpro, RdRp, NTPase, and NendoU) were screened from 187,119 compounds in ZINC natural products library, and seven compounds had high binding potential to NTPase and showed drug-like property. Among them, ZINC12899676 was identified to significantly inhibit the NTPase activity of PEDV by targeting its active pocket and causing its conformational change, and ZINC12899676 significantly inhibited PEDV replication in IPEC-J2 cells. It first demonstrated that ZINC12899676 inhibits PEDV replication by targeting NTPase, and then, NTPase may serve as a novel target for anti-PEDV.
Introduction
Porcine epidemic diarrhea virus (PEDV), a member of the genera Alphacoronavirus in the Coronavirinae subfamily, widely spreads and causes lethal diarrhea in suckling piglets across the world (Jung and Saif, 2015). Due to multiple transmission routes and the case of wild boars harboring PEDV, the commercial swine industry faces greater challenges (Lee et al., 2016; Bevins et al., 2018). Co-infections of PEDV with other viruses complicate disease control and prevention (Chen et al., 2018; Guo et al., 2020; Zhang H et al., 2021). Unfortunately, there is still a lack of effective vaccines against PEDV (Kweon et al., 1999; Lee, 2015; Lin et al., 2016). Antiviral drugs may be a promising pathway to fight against viral infections. For example, acyclovir is approved for the treatment of primary and recurrent genital herpes simplex virus (HSV) infection (Razonable, 2011). Oseltamivir is a licensed medication that specifically targets the neuraminidase protein common to influenza A and B viruses (Long et al., 2018). The antiviral drug molnupiravir for the treatment of mild-to-moderate COVID-19 in adults with at least one risk factor for severe illness has been authorized in UK since November 2021 (Mahase, 2021a). It has been reported that certain small molecules inhibit PEDV proliferation, but commercial drugs have not been developed yet (Wang et al., 2020; Huan et al., 2021; Zhang Y et al., 2021).
Several replicative enzymes responsible for coronavirus replication may be the potential target of antiviral treatment. Papain-like protease (PLpro) plays an important role in virus maturation, dysregulation of host inflammation, and antiviral immune responses (Shin et al., 2020). It can be inhibited by GRL0617, a benzamide resulting from the formal condensation of the carboxy group of 5-amino-2-methylbenzoic acid with the amino group of (1R)-1-(naphthalen-1-yl)ethan-1-amine, which further showed a clear inhibition of SARS-CoV-2 replication in vitro (Fu et al., 2021). Paxlovid is reported in phase III trials for the treatment of COVID-19 by targeting 3C protease (3CLpro), which is essential for processing and maturation of the viral polyprotein (Mahase, 2021b; Zhao et al., 2021). The approved antiviral drug molnupiravir increases the frequency of viral RNA mutations and impairs SARS-CoV-2 replication by disturbing the function of RNA-dependent RNA polymerase (RdRp) (Kabinger et al., 2021). Researchers identified and validated a compound (a bismuth salt) that specifically blocks nucleoside triphosphate hydrolase (NTPase) and RNA helicase activities of SARS-CoV-2 nsp13 (Shu et al., 2020). It is reported that coronavirus uridylate-specific endoribonuclease (EndoU) ensures efficient viral replication (Zhao et al., 2020), and compound NSC95397, also known as 2,3-bis(2-hydroxyethylthio)-1,4-naphthalenedione, was screened as an inhibitor of EndoU (Canal et al., 2021). However, the discovery and screening of small compounds targeting PEDV replicative enzymes have not been systematically conducted up to now.
High-throughput screening has been used for screening of chemical libraries to discover lead compounds for target (Sundberg, 2000). The interactions between compound libraries and target can be evaluated by virtual screening in a rapid and low-consumption manner (Ma et al., 2013). Several antiviral drugs against SARS-CoV-2, Ebola virus, Zika virus, and so on have been found through virtual screening (Capuzzi et al., 2018; Santos et al., 2020; Jang et al., 2021).
To discover the antiviral compound against PEDV, potential PEDV replicative enzyme inhibitors have been identified by virtual screening of 187,119 compounds contained in the ZINC natural products database. It was observed that NTPase had the most hits among the targets. Among the chosen seven compounds, ZINC12899676 was identified to significantly inhibit the NTPase activity. Furthermore, ZINC12899676 was confirmed to significantly inhibit PEDV replication in vitro. These findings offer novel and promising therapeutic possibilities for fighting infections caused by PEDV.
Materials and Methods
Hardware, Software, and Web Service
Virtual screening and molecular dynamics (MD) simulation studies were performed using a 12-core Intel Xeon Platinum 8269CY processor rented from the Aliyun platform. AutoDock Vina (Trott and Olson, 2010) and LeDock (Wang Z et al., 2016) were used in two-round virtual screening. MD simulations were conducted using the GROMACS package and the GROMOS96 43A1 force field, according to the previous description. LigPlot+ is a program for automatic generation of 2D ligand–protein interaction diagrams. Electrostatic potential surfaces were analyzed by Gaussian and visualized by Multiwfn. The webtool ADMETlab2.0 was used to evaluate the drug-like properties of the compounds (Xiong et al., 2021).
3D Model Generation and Verification of Targets
Unknown structure targets were modeled by using three methods: I-TASSER, designed for protein structure modeling by iterative threading assembly simulations (Yang J. et al., 2015; Roy et al., 2010; Zhang, 2008); SWISS-MODEL, dedicated to protein structure homology modeling (Waterhouse et al., 2018); and trRosetta, built for fast and accurate protein structure prediction by deep neural network and homology modeling (Du et al., 2021). C-score of I-TASSER is typically in the range of [−5, 2], where a higher C-score signifies a model with a high confidence and vice versa. A TM-score of I-TASSER greater than 0.5 indicates a model of correct topology. GMQE gives an overall model quality measurement to the SWISS-MODEL between 0 and 1, with higher numbers indicating higher expected quality. A TM-score of trRosetta higher than 0.5 usually indicates a model with correctly predicted topology. Quality assessment of the predicted 3D model of targets was performed using VERIFY 3D (Luthy et al., 1992), PROCHECK (Laskowski et al., 1996), WHATCHECK (Hooft et al., 1996), ERRAT (Colovos and Yeates, 1993), and PROVE (Pontius et al., 1996) integration in SAVES v6.0.
Choice of the Small Compound Library
Natural products library of ZINC15 (Sterling and Irwin, 2015), which is a free database of commercially available compounds provided by the Irwin and Shoichet Laboratories in the Department of Pharmaceutical Chemistry at the University of California comprising 187,119 compounds, was selected for virtual screening. The structure files of ligands were transformed into PDBQT and mol2 files for AutoDock Vina and LeDock by Raccoon and Open Babel software.
Prokaryotic Expression and Purification of PEDV nsp13
The full-length cDNA of PEDV nsp13 was amplified via PCR using the primers, 5′-CGCGAATTCTCTGCAGGGCTTTGTGTTGTT-3′ and 5′-GCGCTCGAGCTGCAAATCAGACAATTTAAG-3′, and then, it was inserted into a pET-28a vector at EcoRI/XhoI restriction sites. The pET-28a-nsp13 was transformed into the E. coli strain BL21, and the cells were cultured at 37°C in the LB medium. When optical density at 600 nm (OD600) reached 0.8, the culture was cooled to 18°C and supplemented with 0.2 mM IPTG. The cells were harvested after incubation at 18°C for 18 h, resuspended in PBS, and then disrupted by ultrasonication. The supernatant was filtered and loaded on Ni-sepharose (GE Healthcare, United States). Finally, the His-tagged protein was eluted using a linear gradient between binding buffer and elution buffer A (20 mM Tris, pH 7.4, 500 mM NaCl, and 250 mM imidazole). A low concentration of imidazole (50 mM) was used to wash impurities, and a high concentration of imidazole (250 mM) was used to elute targeted protein. The target protein was condensed and desalinated using an Amicon Ultra-4 centrifugal filter unit (50 kDa, GE Healthcare, United States). The proteins were analyzed by SDS-PAGE. All the purification procedures were performed at 4°C to avoid unexpected degradation.
ATPase Activity Assay
The Kinase-Glo Plus Luminescent Kinase Assay kit (Promega, United States) was used to detect the ATPase activity of PEDV nsp13. In brief, the purified recombinant proteins in reaction buffer (40 mM Tris-HCl at pH 7.5, 50 mM NaCl, 2 mM Mg2+, 80 μM ATP, and 0.2 μM NTPase) were added to a 96-well black plate in the presence of compounds or DMSO to a total volume of 50 μl. Apyrase from potatoes (Sigma-Aldrich, United States) was used as a positive control enzyme. PEDV N protein was used as a negative control enzyme. The plate was then incubated in a 37°C incubator for 20 min. At the end of the reaction, 50 μl of Kinase-Glo reagent was added to the reaction mixture. After mixing and incubating at room temperature for 2 min, luminescence of each well was measured using a PerkinElmer EnSpire Multimode Plate Reader.
MM-PBSA
The molecular mechanics Poisson–Boltzmann Surface Area (MM-PBSA) approach has been used to estimate the free energy of the binding of small ligands to biological macromolecules. The gmx_mmpbsa shell (https://jerkwin.github.io/gmxtool) together with the APBS program was used to calculate ligand-binding affinities and the corresponding energy decomposition term based on molecular dynamics trajectory (Du et al., 2022). Free binding energy lower than −57 kJ/mol was regarded as very strong affinity.
FEL
The free energy landscape (FEL) is a representation of possible conformations taken by a protein or a ligand–protein complex in molecular dynamics simulation along with Gibbs free energy. Both 2D and 3D graphs of the FEL were plotted using Rg, RMSD, and Gibbs free energy, calculated by using the gmx sham tool.
Cells, Virus, and Reagents
Vero cells and IPEC-J2 cells were maintained in Dulbecco’s modified Eagle’s medium (DMEM) (Gibco, United States) with 10% fetal bovine serum (Lonsera, Uruguay), penicillin (250 U/ml), and streptomycin (250 µg/ml). The cells were incubated at 37°C in a humidified incubator with 5% CO2. The PEDV MS (GenBank accession no. MT683617) strain was maintained in our laboratory and passaged in Vero cells with 6 μg/ml trypsin. Small compounds with purity >90% were used for in vitro experiments (Molport, United States).
Viral Inhibition Assay In Vitro
Vero and IPEC-J2 cells were seeded in 24-well plates at 5 × 105 cells per well. Due to the difference in sensitivity to PEDV, different MOIs of PEDV have been used for Vero and IPEC-J2 cells (Altawaty et al., 2018; Zhang et al., 2018). When approximately 90% confluent, the cells were treated with a compound of different concentrations or DMSO (0.1% v/v) for 1 h and then infected with PEDV (0.01 MOI for Vero and 1 MOI for IPEC-J2) or mock infected for 1 h. The cells were then washed with PBS, and then, the culture medium containing the compound was added back to each well. At 16 h post-infection (hpi), samples were collected to detect the virus quantity. Cell viability was tested using an enhanced Cell Counting Kit-8 (CCK-8) (Beyotime, China), following the manufacturer’s instructions.
Virus Titration
Vero cells grown in 96-well plates were infected with 10-fold serial dilutions of PEDV samples in four replicates. After 1 h at 37°C, the culture medium was replaced with fresh DMEM. The plates were incubated for 48–72 h at 37°C. Virus titers are expressed as TCID50, calculated using the Reed–Muench method.
RNA Extraction and Quantitative Real-Time PCR
Total RNA was extracted from the IPEC-J2 cells using a Total RNA kit (Omega Bio-tek, United States) and reverse transcribed into cDNA using HiScript qRT SuperMix (Vazyme, China), following the manufacturer’s instructions. The detection of mRNA levels of PEDV was performed, as described previously (Wang et al., 2020). Quantitative RT-PCR was performed using the AceQ® qPCR SYBR® Green Master Mix (Vazyme, China). Each reaction was performed in triplicate, and results are expressed as mean ± standard deviation (SD).
Western Blot Assay
The cells were lysed with 100 μlof RIPA lysis buffer (Beyotime, China) on ice for 15 min, then resolved by SDS-PAGE, and transferred to a nitrocellulose membrane. After transfer, the membrane was incubated in blocking buffer (5% non-fat milk in PBST w/v) for 2 h at room temperature, washed three times with PBST, and then probed with the following antibodies: anti-PEDV N-protein (1:1000) prepared in our laboratory; anti-GAPDH (60004-1-Ig, 1:5000; Proteintech Group, United States); and anti-His (1:2000; AF5060, Beyotime, China) for 2 h at room temperature; then, it was washed three times with PBST. The membranes were incubated with HRP-conjugated goat anti-mouse IgG (H−L) secondary antibodies (1:1000; A0216, A0208, Beyotime, China). Bound proteins were exposed with an ECL Kit (Tanon, China).
Construction of the Deep Learning Network
A simple multilayer perceptron (MLP) neural network containing three hidden layers was built to predict the binding energy of ultra-large compound library in-stock. The nodes in the network used a non-linear rectified linear unit (ReLU) function as the activation function. The model was trained using the adaptive moment estimation (Adam) optimizer, which estimates the adaptive learning rate for all parameters involved in the training of gradients. The relationship of the simplified molecular-input line-entry system (SMILES) string and binding energy data from virtual screening was used to train a regression model. We divided the data set into the training set, test set, and validation set with the ratio of 7:2:1. The mean squared error (MSE) was utilized as the loss function and performance metrics:
The hyperparameters, including the learning rate, neuron number in each layer, batch size, and epoch, were tuned.
Statistical Analysis
Statistical analysis was performed by GraphPad Prism 8 software. Results were expressed as mean ± SD. Differences between groups were examined for statistical significance using one-way analysis of variance (ANOVA). The asterisks in the figures indicated significant differences (* = p < 0.05; ** = p < 0.01; *** = p < 0.001; ns, not significant).
Results
Virtual Screening
PEDV replicative enzymes were modeled by I-TASSER, SWISS-MODEL, and trRosetta webtool. All but the 3D structure of nsp12 modeled by trRosetta were credible according to the self-standard (Table 1A). The structures were then subjected to validation by using structure validation tools such as VERIFY 3D, PROCHECK, ERRAT, and PROVE (Table 1B). We selected the structure of nsp12 modeled by using SWISS-MODEL and structures of nsp13 and nsp15 modeled by trRosetta (Table 2). Key amino acid residues were set as pocket for virtual screening.
During the first round of virtual screening, we abandoned the process for nsp3 in advance due to the impracticable binding energy of compounds. Other targets had a lot of ligands whose binding energies were lower than −9 kcal/mol (Figures 1A–D). After the second round of virtual screening, we observed that NTPase possesses the most hits among all targets (Figures 1E–G). It indicated that PEDV NTPase was a potential target by the small compounds, so we focused on the compounds targeting PEDV NTPase for further research (Table 3).
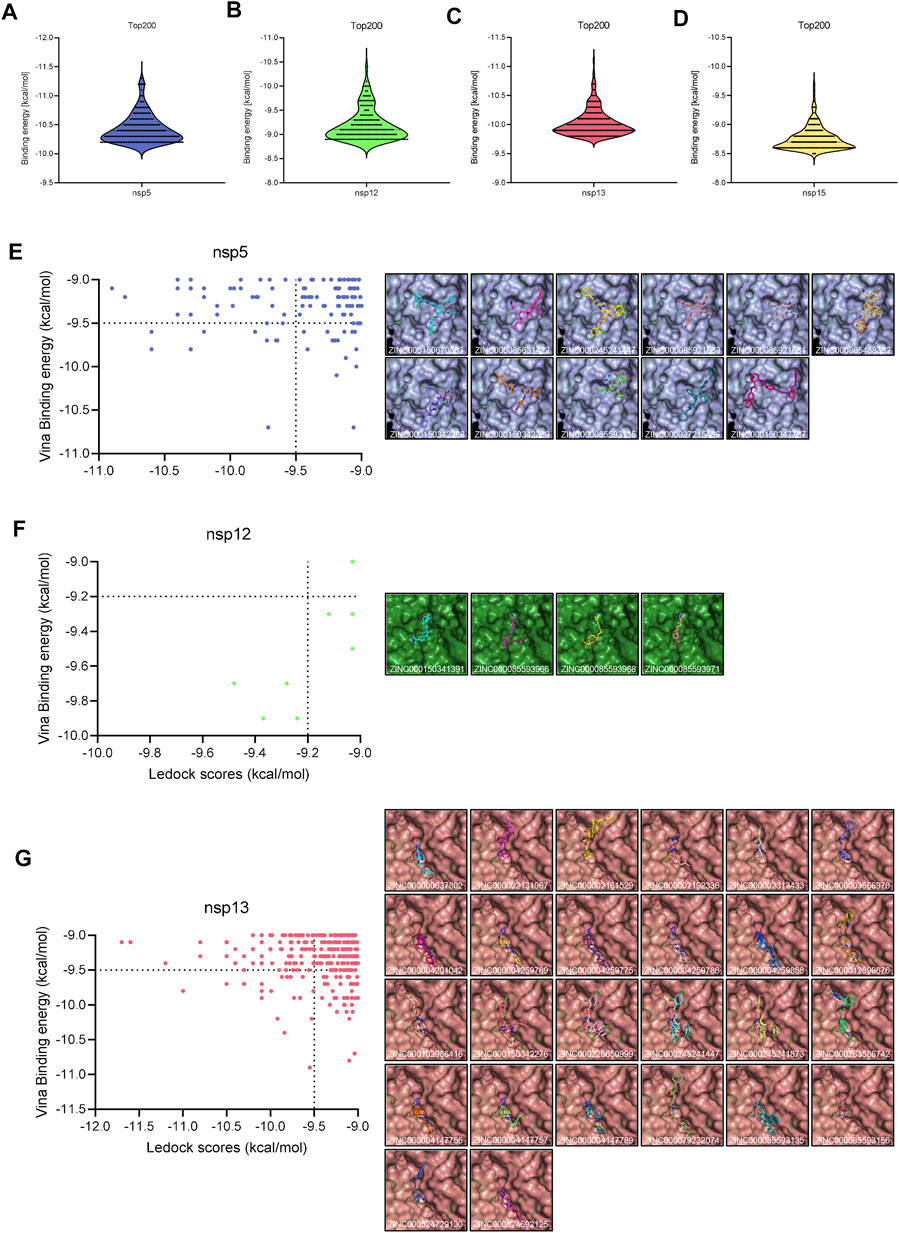
FIGURE 1. Virtual screening. (A–C) Distribution of the docking scores of the top 200 virtual screening hits by AutoDock Vina. (E–G) Left: distribution of high binding energy hits by LeDock; right: overview of the interactions between the ligand and the receptor structure.
ZINC12899676 Inhibits the Activity of PEDV NTPase
Considering binding energy and drug-like characteristic of compounds, seven small compounds, namely, M2 to M8, were selected to ascertain the effect of compounds to PEDV NTPase (Table 4). We first conducted the prokaryotic expression and purification of PEDV nsp13 (Figure 2A) and detected the ATPase activity of recombinant nsp13 proteins by using a Kinase-Glo Plus Luminescent Kinase Assay kit, which measured luminescence caused by the remaining ATP in the reaction mixture. The reaction consisting PEDV N protein as a negative control remained a high luminescent unit same as the mock group. Apyrase from potatoes as a positive control decreased the luminescent unit to little. The amount of ATP remaining in the reaction mixture sharply decreased as the PEDV nsp13 concentration increased (0.1, 0.2, and 1 μM) (Figure 2B). When nsp13 was added, the ATP immediately decreased along with time (Figure 2C). It indicated that recombinant nsp13 possessed the ATP hydrolysis activity.
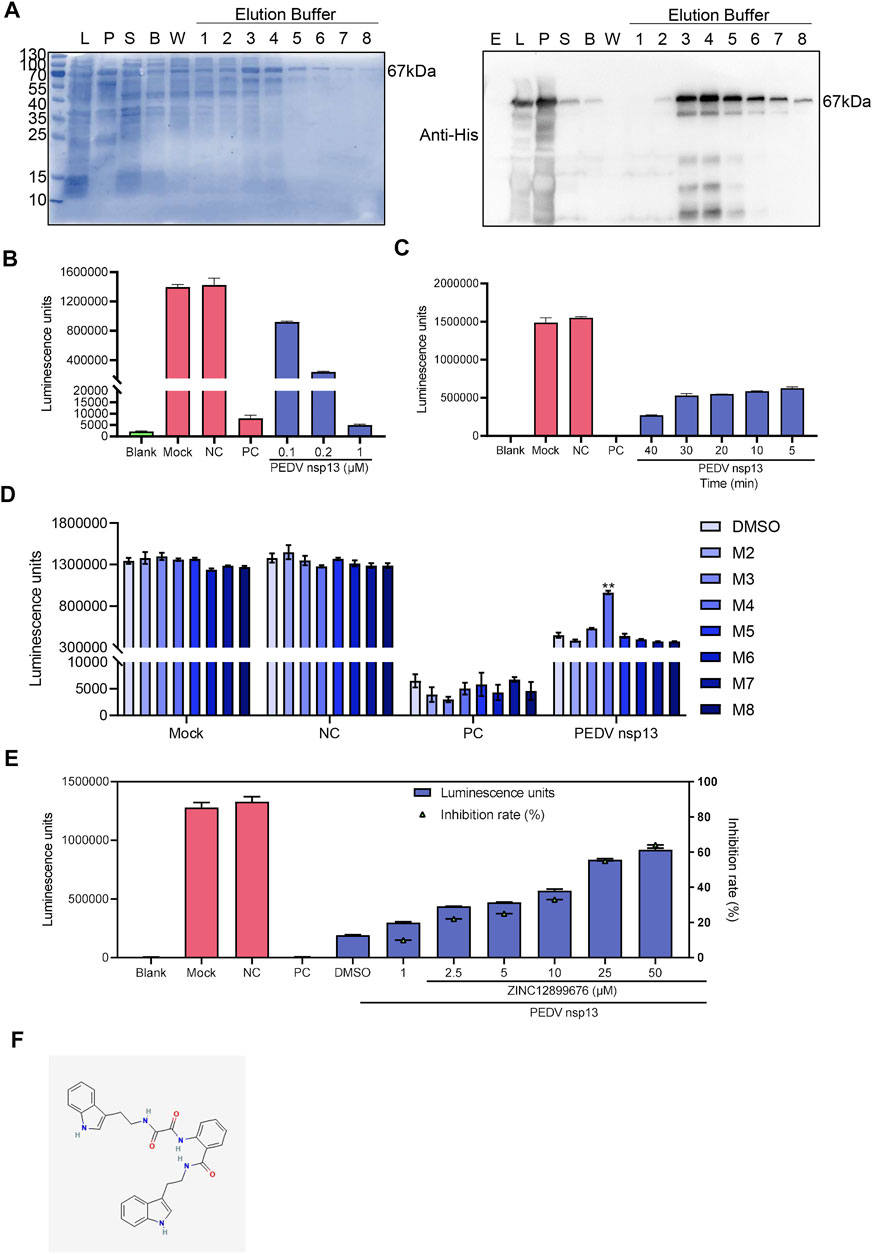
FIGURE 2. ZINC12899676 inhibits the activity of PEDV NTPase. (A) Purification of PEDV nsp13. “E” represents empty vector, “L” represents whole bacteria after induction, “S” represents the supernatant after ultrasonication, “B” represents the liquid collected after washing using binding buffer, “W” represents the liquid collected after washing using washing buffer, and numbers (1–8) represent the liquid collected after washing using elution buffer. (B) Purified nsp13 recombinant proteins (0, 0.1, 0.2, or 0.5 μM) were incubated with ATP in the reaction buffer at 37°C for 20 min, and then, the Kinase-Glo reagent mix was added, and the ATPase activity was measured. (C) PEDV nsp13 (0.2 μM) was reacted with ATP at 37°C for 5, 10, 20, 30, or 40 min, and then, the ATPase activity was measured. (D) Compounds (25 μM) or DMSO were added to the reaction mixture 10 min ahead of ATP, and the ATPase activity was measured. (E) Different concentrations of ZINC12899676 were added to the reaction mixture 10 min ahead of ATP, and the ATPase activity was measured. (F) 2D structure of ZINC12899676.
Compounds (25 μM) or DMSO were added to the reaction mixture 10 min ahead of ATP to measure the inhibition of compounds to the activity of PEDV NTPase, and then, we found that M4 (ZINC12899676) increased the remaining ATP compared with DMSO. In other words, ZINC12899686 significantly inhibited the activity of PEDV NTPase (Figure 2D). PEDV nsp13 was treated with different concentrations of ZINC12899676 and, then the inhibition rate was calculated. The percentage of inhibition was calculated as follows: percentage of inhibition (%) = 100 × [1 − RLU of (negative control–drug treatment)/RLU of (negative control–DMSO treatment)]. ZINC12899676 inhibited the activity of PEDV NTPase in a dose-dependent manner (Figures 2E,F). The IUPAC of ZINC12899676 is N-[2-(1H-indol-3-yl)ethyl]-N'-[2-[2-(1H-indol-3-yl)ethylcarbamoyl]phenyl]oxamide.
Active Pocket of PEDV NTPase Is Targeted by ZINC12899676 Strongly and Its Conformational Change Occurs
To figure out the mechanism of ZINC12899676 against PEDV NTPase, we analyzed the molecular docking between PEDV NTPase and its inhibitor. Hydrogen bonds are generally considered to be important contributors to protein–ligand binding (Chen et al., 2016). LigPlot software visualized the two H-bonds and some residues involving the hydrophobic interaction between the ligand and the receptor (Figure 3A). The H-bond between the compound and the key active site S290 was also visualized by Pymol. These hydrogens bonds and hydrophobic force may contribute to the binding of the ligand and receptor. The various numbers of hydrogen bonds between LigPlot and Pymol may be due to the different judgment standards. In drug discovery, it is widely accepted that electrostatic potential (ESP) surface complementarity between the protein and ligand is critically important in order to obtain optimal affinity and selectivity (Rathi et al., 2020). The ESP surfaces of PEDV NTPase and ZINC12899676 were respectively calculated by APBS plugin integrated into Pymol and Gaussian. The protein ESP surfaces used blue to represent the positive electrostatic potential and red to represent the negative electrostatic potential, and the color representation ligand electrostatic potential surface was the opposite. We could observe that the head and backside of ligand ESP surfaces were fully compatible with the protein ESP surfaces in relevant position, which explained the ZINC12899676 and PEDV NTPase interaction using quantum mechanics (Figure 3B).
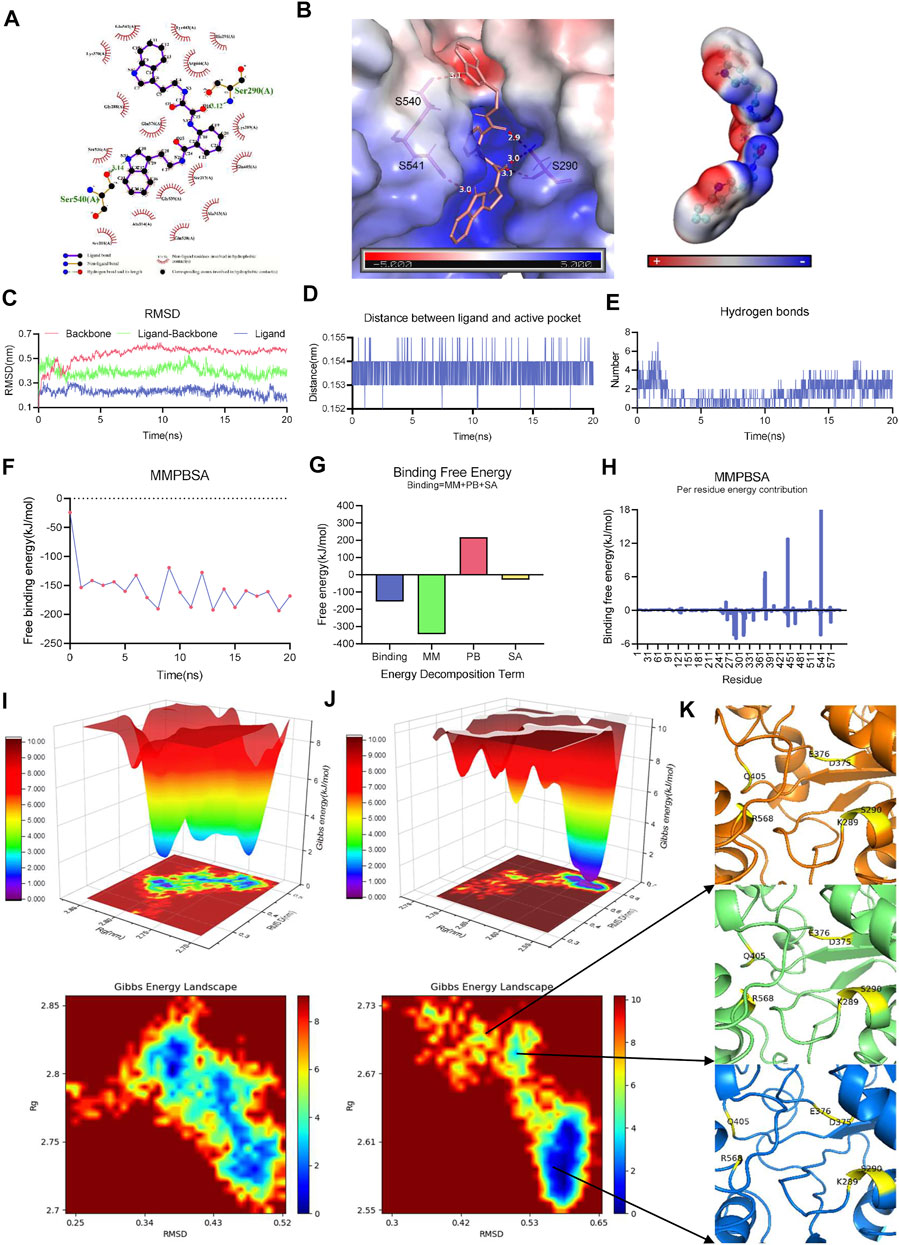
FIGURE 3. Active pocket of PEDV NTPase is targeted by ZINC12899676 strongly, and its conformational change occurs. (A) Interactions between PEDV NTPase and ZINC12899676. Intermolecular hydrogen bonds are shown by green dashed lines with distance in Å. Non-ligand residues in hydrophobic contacts are shown by red gear. (B) Left: H-bond between PEDV NTPase and ZINC12899676 analyzed by Pymol and the electrostatic potential surface representation of PEDV NTPase in complex with ZINC12899676; right: electrostatic potential surface of ZINC12899676. (C) RMSD values of protein backbone, ligand-backbone, and ligand over the simulation time. (D) Distance between the ligand and active pocket of PEDV NTPase over the simulation time. (E) Number of H-bonds involved in the interaction between the protein and compound during the MD simulation. (F) Free binding energy of PEDV NTPase and ZINC12899676 calculated by MM-PBSA. (G) Energy decomposition of the binding energy calculated by MM-PBSA. (H) Decomposition of the energy per residue in the interaction between PEDV NTPase and ZINC12899676 calculated by MM-PBSA. The Gibbs energy landscape obtained during 20 ns MD simulation for (I) free PEDV NTPase and (J) PEDV NTPase-ZINC12899676 complex. (K) Conformation of the active pocket during different periods.
MD utilizes Newtonian physics to simulate atomic movements in a solvated system and is an accurate computational method for simulating protein–drug interactions (Hollingsworth and Dror, 2018). To assess receptor and ligand stability, a 20 ns molecular dynamic simulation was carried out. The smooth and steady RMSD of the backbone/ligand indicated less structural changes during MD simulation. The protein–compound complex reached its stability throughout the simulation with relative RMSD in acceptable range, with few fluctuations observed (Figure 3C). Distances between compound and the active pocket of NTPase were highly conserved and tight during simulation calculations (Figure 3D). We monitored the number of hydrogen bonds during the MD simulations to better capture the intermolecular polar interactions. The stable existence of H-bonds in the complex began with the 12 ns, which explained the integrity and stable nature of the protein–ligand complex (Figure 3E). In order to ascertain the observed stability, the binding affinities of ZINC12899676 with the NTPase complex were defined by binding free energy based on the molecular mechanics Poisson–Boltzmann surface area (MM-PBSA) approach and measured using the gmx_mmpbsa tool. The estimated MM-PBSA binding energy free energy variation with time is shown in Figure 3F, which suggested the efficient and steady binding of the ligand to NTPase. The average of binding free energy of the protein–ligand complex obtained using MM-PBSA was −154.702 kJ/mol and indicated a very strong affinity (Figure 3G). Residues which provided free binding energy were mainly located in the active pocket of PEDV NTPase, which was consistent with the actual interaction situation (Figure 3H).
The Gibbs free energy landscape (FEL) is analyzed for understanding the conformational transition beneath the protein–compound interaction, using the reaction coordinates of RMSD and Rg, respectively (Efaz et al., 2021). FELs of PEDV NTPase and the complex system of NTPase in presence of ZINC12899676 were displayed in 2D and 3D graphs. The single energy cluster with deep blue plots in the energy distribution indicated strong and stable interaction, and multiple energy minimum clusters with shallow yellow plots reflected weak and unstable interaction of the protein and ligand. The deep blue areas with minimal energy were found to be highly concentrated in NTPase in the presence of the ZINC12899676 complex (Figure 3J) when compared to the NTPase alone (Figure 3I). This result elucidated that the compound had the potential to induce NTPase to enter the local energy minimal state and to form the stable binding of the compound at the protein active pocket. Furthermore, the different stage conformations during the energy minimal state had been extracted from the trajectory. It was obvious that the β-sheet located in R568 had been transformed into α-helix, and Q405 shifting out from the active pocket led to the expansion of pocket (Figure 3K). The conformation change caused by ZINC12899676 may finally lead to the dysfunction of NTPase. In general, the ligand strongly binding to enzyme’s active site and transforming the active pocket conformation contributed to inhibit the activity of PEDV NTPase.
ZINC12899676 Has Antiviral Activity Against PEDV In Vitro
Since the compound inhibited the activity of PEDV NTPase, we further evaluated its anti-PEDV activity in vitro. Some compounds were ruled out because of the visible cytotoxicity (Figures 4A,B), and the remaining compounds were screened by Western blot. Results demonstrated that only ZINC12899676 had anti-PEDV activity coinciding with the inhibition of the NTPase enzymatic activity (Figures 4C,D).
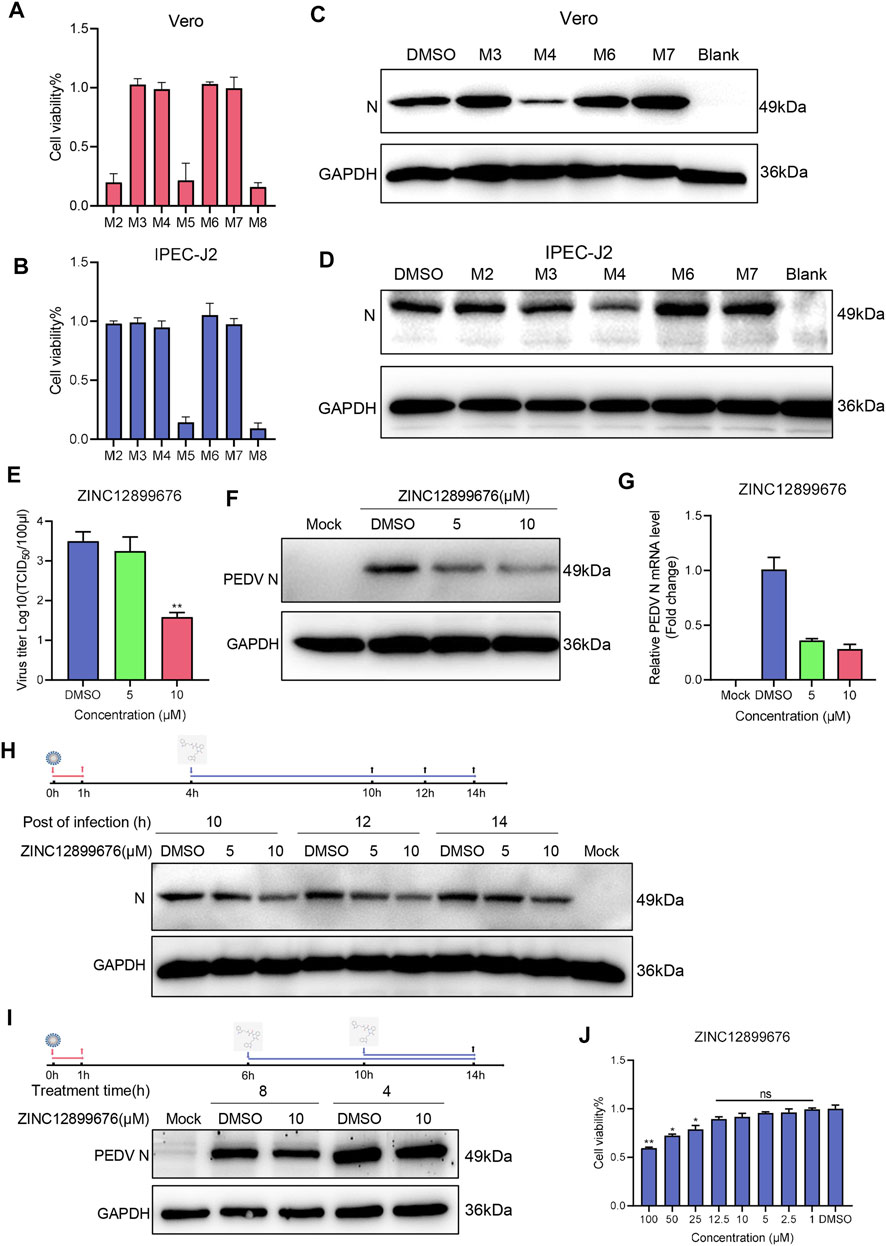
FIGURE 4. ZINC12899676 has antiviral activity against PEDV in vitro. (A,B) Cell viability of Vero and IPEC-J2 cells pretreated with compounds (10 μM) and incubated for 24 h. The results are from one of three independent experiments. (C,D) Vero and IPEC-J2 cells were pretreated with compounds (10 μM) for 1 h and then infected with PEDV for 1 h at 37°C. The cells were washed with PBS and then incubated in a fresh medium containing compounds for 16 h. DMSO served as the treatment control. (E–G) IPEC-J2 cells were pretreated with the indicated concentrations of ZINC12899676 for 1 h and then infected with PEDV for 1 h at 37°C. The cells were washed with PBS and then incubated in fresh medium containing ZINC12899676 for 16 h. DMSO served as the treatment control. (E) Culture supernatants were collected at indicated time points for viral titration. Results are expressed as TCID50. Titers from three independent experiments are shown as means ± SD (error bars). (F) Western blot for the viral N-protein in cells infected with PEDV and treated with indicated concentrations of ZINC12899676 or DMSO, at 16 hpi. (G) Relative PEDV N mRNA levels, determined by qRT-PCR, and expressed relative to that in DMSO-treated cells. The internal loading control was GAPDH. (H) IPEC-J2 cells were incubated with PEDV at 37°C for 1 h and washed three times with PBS to remove free virus. At 4 hpi, the culture medium was replaced with fresh DMEM containing ZINC12899676 or DMSO, and the cultures were incubated at 37°C. PEDV N and GAPDH proteins in the samples collected at 10, 12, and 14 hpi were measured using Western blot. (I) IPEC-J2 cells were incubated with PEDV at 37°C for 1 h and washed three times with PBS to remove free virus. At 6 or 10 hpi, the culture medium was replaced with fresh DMEM containing ZINC12899676 or DMSO, and the cultures were incubated at 37°C. PEDV N and GAPDH proteins in the samples collected at 14 hpi were measured using Western blot. (J) Cell viability of IPEC-J2 cells pretreated with indicated concentrations of ZINC12899676 and incubated for 24 h.
To elucidate the antiviral activity of ZINC12899676 in porcine cells, IPEC-J2 cells were treated with 5 and 10 μM compounds for 1 h and then infected with PEDV (1 MOI). TCID50, Western blot, and qRT-PCR analysis showed that the virus titers, N-protein, and mRNA levels significantly decreased in a dose-dependent manner (Figures 4E–G). We examined the effect of tomatidine on PEDV replication by adding the compound during the replication stage. As shown in Figure 4H, ZINC12899676 treatment significantly decreased PEDV N protein levels compared to treatment with DMSO, suggesting that the compound inhibits PEDV replication. In addition, ZINC12899676 was added in different hours post-infection. As the action time of ZINC12899676 on replication stage was reduced, the antiviral effect of the drug decreased (Figure 4I). Inhibition of the activity of the PEDV NTPase responsible for replication corresponded to the action on the replication stage of PEDV by ZINC12899676. Meanwhile, the concentrations of the compound exerting on IPEC-J2 cells displayed no cytotoxicity through cell viable assay (Figure 4J). In summary, ZINC12899676 had been proved to fight against PEDV in vitro.
Neural Network Is Built to Accelerate the Discovery of Potential Compounds Targeting PEDV NTPase
To improve the structure diversity of lead compounds, we had meant to conduct an ultra-large virtual screening to discover more lead compounds. Structure-based virtual screening costs too many computes. Recently, deep learning (DL) models show promising performance for drug property prediction. We built a simple drug property prediction multilayer perceptron (MLP) neural network to exclude the low binding energy compounds, thereby narrowing the scope of virtual screening (Figure 5A). The remaining high binding energy compounds through AI prediction were carried out by two-round virtual screening. After the tunning of hyperparameters, the MSE finally reached to 0.23 (Figures 5B–E). The parameters and hyperparameters are shown in Figure 5F. The speed of neuron network prediction was more than 600 times than virtual screening (Figure 5G); it indicated neuron network predicting had the capacity to screen millions of compounds. We finally quickly discovered 768 compounds with high binding energy (<−9.5 kcal/mol) from 13,651,290 compounds through a workflow: AI predicting-AutoDock Vina-LeDock virtual screening (Figure 5H). Cluster analysis based on the structures was performed on these compounds by ChemMine software (Backman et al., 2011). We can observe that the molecules were grouped into several clusters (Figure 5I). The top item from each cluster should be selected as the representative of the related cluster and used for more perspective of PEDV NTPase inhibitors. This result may be beneficial to enhance the structure diversity of lead compounds targeting PEDV NTPase.
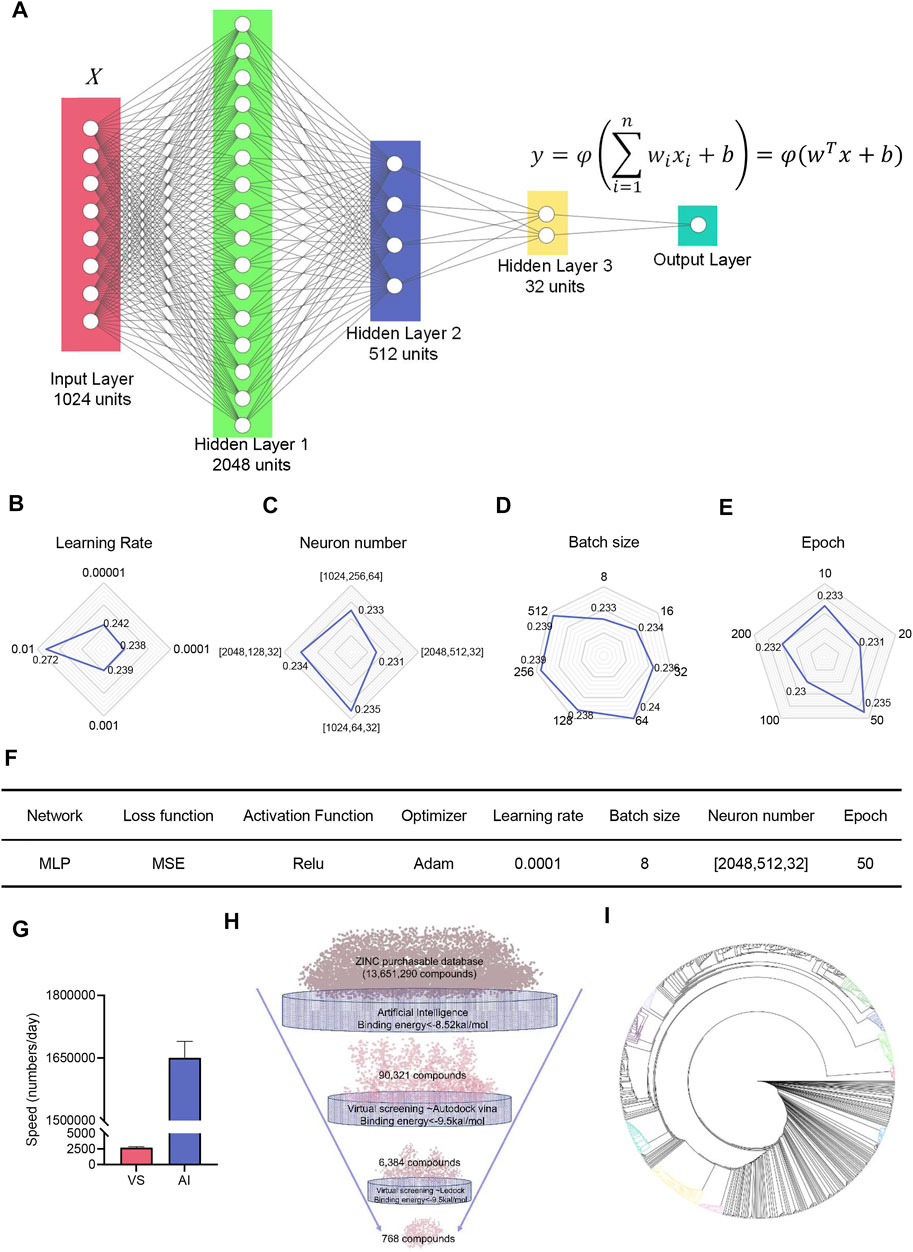
FIGURE 5. Neural network built to accelerate the discovery of potential compounds targeting PEDV NTPase. (A) Structure of the deep neural network. Neurons are represented by circles. (B–E) Tunning of hyperparameters. (F) Parameter and hyperparameter settings for the deep neural network. (G) Comparison of the speed of virtual screening with artificial intelligence. (H) Combination application of AI and VS to find compounds with high binding energy to PEDV NTPase. (I) Clusters of compounds targeting PEDV NTPase with high binding energy.
Discussion
Researchers have found several antiviral small compounds against PEDV in vitro. There are some compounds such as salinomycin (Yuan et al., 2021), homoharringtonine (Dong et al., 2018), and A77 1726 (Li X. et al., 2020) preventing PEDV proliferation by affecting the host defense system. Many compounds inhibit PEDV replication by targeting PEDV 3CLpro, such as tomatidine (Wang et al., 2020), hypericin (Zhang Y et al., 2021), quercetin (Li Z. et al., 2020), and GC376 (Ye et al., 2020). PEDV RdRp also can be the target of antiviral drugs (Xie et al., 2021). In this study, a natural compound ZINC12899676 was first identified to significantly inhibit the NTPase activity, and it significantly inhibited PEDV replication in vitro.
The nsp13s from representative α- and β-coronavirus shared conserved helicase and NTPase. It possesses NTPase and helicase activities (Jia et al., 2019; Ren et al., 2021). Coronavirus NTPase can hydrolyze nucleoside triphosphate in the presence of Mg2+ and Mn2+ to produce energy, which can be utilized by helicase for unwinding dsDNA and dsRNA (Ivanov et al., 2004). Thus, we speculated ZINC12899676 can also inhibit PEDV nsp13 helicase activity by limiting energy production (Yang et al., 2007). Coronavirus nsp13 can make an impact on host defense. Researchers reported that the host interferon signaling pathway can be suppressed by SARS-CoV-2 nsp13 (Lei et al., 2020; Yuen et al., 2020; Feng et al., 2021). In addition, SARS-CoV-2 nsp13 hijacks host deubiquitinase UPS13 and confronts host antiviral immune response (Guo et al., 2021). Inflammasome complexes have both positive and negative effects on the host. SARS-CoV-2 nsp13 domains responsible for helicase activity significantly reduced NLRP3-inflammasome-induced caspase-1 activity and IL-1β secretion (Kim et al., 2021). Many DNA and RNA viruses can cause disturbance of cell cycle regulation; researchers demonstrated that the interaction between nsp13 and DNA polymerase δ induced DNA replication stress in IBV-infected cells (Xu et al., 2011). Therefore, it is extremely meaningful to seek small compounds targeting PEDV NTPase.
So far, a variety of docking programs are available for the scientific community. These programs have been systematically evaluated by analyzing the accuracies of binding pose prediction and binding affinity estimation, which are two pillars for a successful docking experiment (Saikia and Bordoloi, 2019). Results showed that LeDock had the best sampling power among the five academic programs, and AutoDock Vina had the best scoring power among the commercial and academic programs (Wang Z et al., 2016). Therefore, we carried out virtual screening by AutoDock Vina and LeDock programs. According to the report, it is inevitable to generate false-negatives and positives during virtual screening (Ferreira et al., 2010). Furthermore, expectation of identifying high-affinity compounds by VS and subjectivity of post-VS compound selection are two pitfalls in virtual screening. Avoiding these two problems may better optimize the efficiency and accuracy of virtual screening (Scior et al., 2012). Structure-based virtual screening costs too many computes. The speed of virtual screening is not sufficient to face the billion drugs in the database. Artificial intelligence (AI) has recently demonstrated its superior performance than classic methods to assist computational drug discovery, owing to its expressive power in extracting, processing, and extrapolating patterns in molecular data (Ekins et al., 2019; Zhavoronkov et al., 2019; Stokes et al., 2020).
The 3D structure of targets is the fundamental of virtual screening. We determined protein structures through comparing various modeling approaches and assessing the reliability of modeling structures. We predicted protein structures by I-TASSER, SWISS-MODEL, and trRosetta, which have been widely used and granted numerous awards. Benefits from the determination of the crystal structure of SARS-CoV-2 (Malone et al., 2021; Newman et al., 2021), SWISS-MODEL, and trRosetta-based homology modeling have better performance. During our preparation of this article, Alphafold2 (Jumper et al., 2021), and RoseTTAFold (Baek et al., 2021), delivering a revolutionary advance for protein structure predictions, have been published (Mullard, 2021). The combination of these tools can create 3D models of the eukaryotic protein complex which may help accelerate the development of future novel therapeutics and drug discoveries (Humphreys et al., 2021). However, there is lack of online AlphaFold service to utilize, and it demands ultra-high hardware to deploy locally. Reassuringly, we selected the PEDV NTPase structure modeled by trRosetta, which showed performance similar to AlphaFold (Pakhrin et al., 2021). In addition, other researchers revealed the enzyme catalytic center of several coronavirus replicative enzymes. The enzyme catalytic centers of nsp3 are C1729, H1888, D1901, and W1730 (Durie et al., 2021). Residues H41 and C144 are key amino acids of the activity of nsp5 (Wang D et al., 2016). Amino acid residues G608, W609, D610, Y611, L750, S751, D752, D753, K790, C791, W792, E803, F804, C805, and S806 form the active pocket of nsp12 (Aftab et al., 2020). The active pocket of nsp13 NTPase is constituted by K289, S290, D375, E376, Q405, and R568 (Mirza and Froeyen, 2020). The active pocket of nsp15 is composed of H226, H241, and K282 (Wu et al., 2020). These findings contributed to the selection of the PEDV enzyme active pocket.
The scale of natural products with numerous structural and chemical diversities is much larger than any synthetic libraries of small molecules (Shen, 2015). The utilization of natural products and/or synthetic variations using their novel structures from 1981 to 2019 is still alive and well (Newman and Cragg, 2020). At the same time, researchers found PEDV replication can be inhibited by natural compounds, such as griffithsin (Li et al., 2019), coumarin (Yang J. L. et al., 2015), and so on. Thus, natural products library of ZINC15 was selected for virtual screening. Delightfully, ZINC12899676 showed drug-like properties in accordance with Lipinski’s rule of five and Pfizer’s rule. In addition, ZINC12899676 was previously reported to inhibit TLR1-TLR2 heterodimerization as an immunomodulatory agent (Zhong et al., 2015).
In conclusion, we observed that PEDV NTPase had the most hits among the five targets (PLP2, 3CLpro, RdRp, NTPase, and NendoU) through two-round virtual screening. ZINC12899676 was identified to significantly inhibit the NTPase activity. Computational biology analysis indicated that the active pocket of PEDV NTPase was targeted by ZINC12899676 strongly, and its conformational change occurred. Furthermore, ZINC12899676 significantly inhibited PEDV replication in vitro. Our findings first demonstrated that ZINC12899676 inhibits PEDV replication by targeting NTPase, and then, NTPase may serve as a novel target for anti-PEDV.
Data Availability Statement
The original contributions presented in the study are included in the article/Supplementary Material, further inquiries can be directed to the corresponding author.
Author Contributions
Conception of the work: PJ and PW; cellular experiment: PW, JB, MS, and XaL; analysis and interpretation of data: PJ, XnL, and PW; preparation of the manuscript: PJ and PW. All authors read and approved the final manuscript.
Funding
This work was supported by the China Agriculture Research System (CARS-35), the National Key Research and Development Program of China (2021YFD1801102), and the Priority Academic Program Development of Jiangsu Higher Education Institutions (PAPD).
Conflict of Interest
The authors declare that the research was conducted in the absence of any commercial or financial relationships that could be construed as a potential conflict of interest.
Publisher’s Note
All claims expressed in this article are solely those of the authors and do not necessarily represent those of their affiliated organizations, or those of the publisher, the editors, and the reviewers. Any product that may be evaluated in this article, or claim that may be made by its manufacturer, is not guaranteed or endorsed by the publisher.
Supplementary Material
The Supplementary Material for this article can be found online at: https://www.frontiersin.org/articles/10.3389/fphar.2022.879733/full#supplementary-material
References
Aftab, S. O., Ghouri, M. Z., Masood, M. U., Haider, Z., Khan, Z., Ahmad, A., et al. (2020). Analysis of SARS-CoV-2 RNA-dependent RNA Polymerase as a Potential Therapeutic Drug Target Using a Computational Approach. J. Transl. Med. 18 (1), 275. doi:10.1186/s12967-020-02439-0
Altawaty, T., Liu, L., Zhang, H., Tao, C., Hou, S., Li, K., et al. (2018). Lack of LTβR Increases Susceptibility of IPEC-J2 Cells to Porcine Epidemic Diarrhea Virus. Cells 7 (11). doi:10.3390/cells7110222
Backman, T. W., Cao, Y., and Girke, T. (2011). ChemMine Tools: an Online Service for Analyzing and Clustering Small Molecules. Nucleic Acids Res. 39, W486–W491. doi:10.1093/nar/gkr320
Baek, M., Dimaio, F., Anishchenko, I., Dauparas, J., Ovchinnikov, S., Lee, G. R., et al. (2021). Accurate Prediction of Protein Structures and Interactions Using a Three-Track Neural Network. Science 373 (6557), 871–876. doi:10.1126/science.abj8754
Bevins, S. N., Lutman, M., Pedersen, K., Barrett, N., Gidlewski, T., Deliberto, T. J., et al. (2018). Spillover of Swine Coronaviruses, United States. Emerg. Infect. Dis. 24 (7), 1390–1392. doi:10.3201/eid2407.172077
Canal, B., Fujisawa, R., Mcclure, A. W., Deegan, T. D., Wu, M., Ulferts, R., et al. (2021). Identifying SARS-CoV-2 Antiviral Compounds by Screening for Small Molecule Inhibitors of Nsp15 Endoribonuclease. Biochem. J. 478 (13), 2465–2479. doi:10.1042/BCJ20210199
Capuzzi, S. J., Sun, W., Muratov, E. N., Martínez-Romero, C., He, S., Zhu, W., et al. (2018). Computer-Aided Discovery and Characterization of Novel Ebola Virus Inhibitors. J. Med. Chem. 61 (8), 3582–3594. doi:10.1021/acs.jmedchem.8b00035
Chen, D., Oezguen, N., Urvil, P., Ferguson, C., Dann, S. M., and Savidge, T. C. (2016). Regulation of Protein-Ligand Binding Affinity by Hydrogen Bond Pairing. Sci. Adv. 2 (3), e1501240. doi:10.1126/sciadv.1501240
Chen, Q., Wang, L., Zheng, Y., Zhang, J., Guo, B., Yoon, K. J., et al. (2018). Metagenomic Analysis of the RNA Fraction of the Fecal Virome Indicates High Diversity in Pigs Infected by Porcine Endemic Diarrhea Virus in the United States. Virol. J. 15 (1), 95. doi:10.1186/s12985-018-1001-z
Colovos, C., and Yeates, T. O. (1993). Verification of Protein Structures: Patterns of Nonbonded Atomic Interactions. Protein Sci. 2 (9), 1511–1519. doi:10.1002/pro.5560020916
Dong, H. J., Wang, Z. H., Meng, W., Li, C. C., Hu, Y. X., Zhou, L., et al. (2018). The Natural Compound Homoharringtonine Presents Broad Antiviral Activity In Vitro and In Vivo. Viruses 10 (11). doi:10.3390/v10110601
Du, J., Yang, C., Ma, X., and Li, Q. (2022). Insights into the Conformation Changes of SARS-CoV-2 Spike Receptor-Binding Domain on Graphene. Appl. Surf. Sci. 578, 151934. doi:10.1016/j.apsusc.2021.151934
Du, Z., Su, H., Wang, W., Ye, L., Wei, H., Peng, Z., et al. (2021). The trRosetta Server for Fast and Accurate Protein Structure Prediction. Nat. Protoc. 16 (12), 5634–5651. doi:10.1038/s41596-021-00628-9
Durie, I. A., Dzimianski, J. V., Daczkowski, C. M., Mcguire, J., Faaberg, K., and Pegan, S. D. (2021). Structural Insights into the Interaction of Papain-like Protease 2 from the Alphacoronavirus Porcine Epidemic Diarrhea Virus and Ubiquitin. Acta Crystallogr. D Struct. Biol. 77 (Pt 7), 943–953. doi:10.1107/S205979832100509X
Efaz, F. M., Islam, S., Talukder, S. A., Akter, S., Tashrif, M. Z., Ali, M. A., et al. (2021). Repurposing Fusion Inhibitor Peptide against SARS-CoV-2. J. Comput. Chem. 42 (32), 2283–2293. doi:10.1002/jcc.26758
Ekins, S., Puhl, A. C., Zorn, K. M., Lane, T. R., Russo, D. P., Klein, J. J., et al. (2019). Exploiting Machine Learning for End-To-End Drug Discovery and Development. Nat. Mater. 18 (5), 435–441. doi:10.1038/s41563-019-0338-z
Feng, K., Min, Y.-Q., Sun, X., Deng, F., Li, P., Wang, H., et al. (2021). Interactome Profiling Reveals Interaction of SARS-CoV-2 NSP13 with Host Factor STAT1 to Suppress Interferon Signaling. J. Mol. Cel Biol. 13, 760–762. doi:10.1093/jmcb/mjab068
Ferreira, R. S., Simeonov, A., Jadhav, A., Eidam, O., Mott, B. T., Keiser, M. J., et al. (2010). Complementarity between a Docking and a High-Throughput Screen in Discovering New Cruzain Inhibitors. J. Med. Chem. 53 (13), 4891–4905. doi:10.1021/jm100488w
Fu, Z., Huang, B., Tang, J., Liu, S., Liu, M., Ye, Y., et al. (2021). The Complex Structure of GRL0617 and SARS-CoV-2 PLpro Reveals a Hot Spot for Antiviral Drug Discovery. Nat. Commun. 12 (1), 488. doi:10.1038/s41467-020-20718-8
Guo, G., Gao, M., Gao, X., Zhu, B., Huang, J., Luo, K., et al. (2021). SARS-CoV-2 Non-structural Protein 13 (Nsp13) Hijacks Host Deubiquitinase USP13 and Counteracts Host Antiviral Immune Response. Signal. Transduct Target. Ther. 6 (1), 119. doi:10.1038/s41392-021-00509-3
Guo, Z., Ruan, H., Qiao, S., Deng, R., and Zhang, G. (2020). Co-infection Status of Porcine Circoviruses (PCV2 and PCV3) and Porcine Epidemic Diarrhea Virus (PEDV) in Pigs with Watery Diarrhea in Henan Province, central China. Microb. Pathog. 142, 104047. doi:10.1016/j.micpath.2020.104047
Hollingsworth, S. A., and Dror, R. O. (2018). Molecular Dynamics Simulation for All. Neuron 99 (6), 1129–1143. doi:10.1016/j.neuron.2018.08.011
Hooft, R. W., Vriend, G., Sander, C., and Abola, E. E. (1996). Errors in Protein Structures. Nature 381 (6580), 272. doi:10.1038/381272a0
Huan, C., Xu, W., Ni, B., Guo, T., Pan, H., Jiang, L., et al. (2021). Epigallocatechin-3-Gallate, the Main Polyphenol in Green Tea, Inhibits Porcine Epidemic Diarrhea Virus In Vitro. Front. Pharmacol. 12, 628526. doi:10.3389/fphar.2021.628526
Humphreys, I. R., Pei, J., Baek, M., Krishnakumar, A., Anishchenko, I., Ovchinnikov, S., et al. (2021). Computed Structures of Core Eukaryotic Protein Complexes. Science 374, eabm4805. doi:10.1126/science.abm4805
Ivanov, K. A., Dobbe, J. C., van der Meer, Y., Snijder, E. J., Ziebuhr, J., and Ziebuhr, J. (2004). Multiple Enzymatic Activities Associated with Severe Acute Respiratory Syndrome Coronavirus Helicase. J. Virol. 78 (11), 5619–5632. doi:10.1128/JVI.78.11.5619-5632.2004
Jang, W. D., Jeon, S., Kim, S., and Lee, S. Y. (2021). Drugs Repurposed for COVID-19 by Virtual Screening of 6,218 Drugs and Cell-Based Assay. Proc. Natl. Acad. Sci. U S A. 118 (30). doi:10.1073/pnas.2024302118
Jia, Z., Yan, L., Ren, Z., Wu, L., Wang, J., Guo, J., et al. (2019). Delicate Structural Coordination of the Severe Acute Respiratory Syndrome Coronavirus Nsp13 upon ATP Hydrolysis. Nucleic Acids Res. 47 (12), 6538–6550. doi:10.1093/nar/gkz409
Jumper, J., Evans, R., Pritzel, A., Green, T., Figurnov, M., Ronneberger, O., et al. (2021). Highly Accurate Protein Structure Prediction with AlphaFold. Nature 596 (7873), 583–589. doi:10.1038/s41586-021-03819-2
Jung, K., and Saif, L. J. (2015). Porcine Epidemic Diarrhea Virus Infection: Etiology, Epidemiology, Pathogenesis and Immunoprophylaxis. Vet. J. 204 (2), 134–143. doi:10.1016/j.tvjl.2015.02.017
Kabinger, F., Stiller, C., Schmitzová, J., Dienemann, C., Kokic, G., Hillen, H. S., et al. (2021). Mechanism of Molnupiravir-Induced SARS-CoV-2 Mutagenesis. Nat. Struct. Mol. Biol. 28 (9), 740–746. doi:10.1038/s41594-021-00651-0
Kim, N. E., Kim, D. K., and Song, Y. J. (2021). SARS-CoV-2 Nonstructural Proteins 1 and 13 Suppress Caspase-1 and the NLRP3 Inflammasome Activation. Microorganisms 9 (3). doi:10.3390/microorganisms9030494
Kweon, C. H., Kwon, B. J., Lee, J. G., Kwon, G. O., and Kang, Y. B. (1999). Derivation of Attenuated Porcine Epidemic Diarrhea Virus (PEDV) as Vaccine Candidate. Vaccine 17 (20-21), 2546–2553. doi:10.1016/s0264-410x(99)00059-6
Laskowski, R. A., Rullmannn, J. A., Macarthur, M. W., Kaptein, R., and Thornton, J. M. (1996). AQUA and PROCHECK-NMR: Programs for Checking the Quality of Protein Structures Solved by NMR. J. Biomol. NMR. 8 (4), 477–486. doi:10.1007/BF00228148
Lee, C. (2015). Porcine Epidemic Diarrhea Virus: An Emerging and Re-emerging Epizootic Swine Virus. Virol. J. 12 (1), 193. doi:10.1186/s12985-015-0421-2
Lee, D. U., Kwon, T., Je, S. H., Yoo, S. J., Seo, S. W., Sunwoo, S. Y., et al. (2016). Wild Boars Harboring Porcine Epidemic Diarrhea Virus (PEDV) May Play an Important Role as a PEDV Reservoir. Vet. Microbiol. 192, 90–94. doi:10.1016/j.vetmic.2016.07.003
Lei, X., Dong, X., Ma, R., Wang, W., Xiao, X., Tian, Z., et al. (2020). Activation and Evasion of Type I Interferon Responses by SARS-CoV-2. Nat. Commun. 11 (1), 3810. doi:10.1038/s41467-020-17665-9
Li, L., Yu, X., Zhang, H., Cheng, H., Hou, L., Zheng, Q., et al. (2019). In Vitro Antiviral Activity of Griffithsin Against Porcine Epidemic Diarrhea Virus. Virus Genes 55 (2), 174–181. doi:10.1007/s11262-019-01633-7
Li X, X., Sun, J., Prinz, R. A., Liu, X., and Xu, X. (2020). Inhibition of Porcine Epidemic Diarrhea Virus (PEDV) Replication by A77 1726 through Targeting JAK and Src Tyrosine Kinases. Virology 551, 75–83. doi:10.1016/j.virol.2020.06.009
Li Z, Z., Cao, H., Cheng, Y., Zhang, X., Zeng, W., Sun, Y., et al. (2020). Inhibition of Porcine Epidemic Diarrhea Virus Replication and Viral 3C-like Protease by Quercetin. Int. J. Mol. Sci. 21 (21), 1. doi:10.3390/ijms21218095
Lin, C. M., Saif, L. J., Marthaler, D., and Wang, Q. (2016). Evolution, Antigenicity and Pathogenicity of Global Porcine Epidemic Diarrhea Virus Strains. Virus. Res. 226, 20–39. doi:10.1016/j.virusres.2016.05.023
Long, S. S., Prober, C. G., and Fischer, M. (2018). Pediatric Infectious Diseases :principles and Practice. Fifth Edition. Norwalk, Conn: Appleton & Lange, 1593.
Lüthy, R., Bowie, J. U., and Eisenberg, D. (1992). Assessment of Protein Models with Three-Dimensional Profiles. Nature 356 (6364), 83–85. doi:10.1038/356083a0
Ma, D. L., Chan, D. S., and Leung, C. H. (2013). Drug Repositioning by Structure-Based Virtual Screening. Chem. Soc. Rev. 42 (5), 2130–2141. doi:10.1039/c2cs35357a
Mahase, E. (2021b). Covid-19: Pfizer's Paxlovid Is 89% Effective in Patients at Risk of Serious Illness, Company Reports. BMJ 375, n2713. doi:10.1136/bmj.n2713
Mahase, E. (2021a). Covid-19: UK Becomes First Country to Authorise Antiviral Molnupiravir. BMJ 375, n2697. doi:10.1136/bmj.n2697
Malone, B., Chen, J., Wang, Q., Llewellyn, E., Choi, Y. J., Olinares, P. D. B., et al. (2021). Structural Basis for Backtracking by the SARS-CoV-2 Replication-Transcription Complex. Proc. Natl. Acad. Sci. U.S.A. 118 (19). doi:10.1073/pnas.2102516118
Mirza, M. U., and Froeyen, M. (2020). Structural Elucidation of SARS-CoV-2 Vital Proteins: Computational Methods Reveal Potential Drug Candidates against Main Protease, Nsp12 Polymerase and Nsp13 Helicase. J. Pharm. Anal. 10 (4), 320–328. doi:10.1016/j.jpha.2020.04.008
Mullard, A. (2021). What Does AlphaFold Mean for Drug Discovery? Nat. Rev. Drug Discov. 20 (10), 725–727. doi:10.1038/d41573-021-00161-0
Newman, D. J., and Cragg, G. M. (2020). Natural Products as Sources of New Drugs over the Nearly Four Decades from 01/1981 to 09/2019. J. Nat. Prod. 83 (3), 770–803. doi:10.1021/acs.jnatprod.9b01285
Newman, J. A., Douangamath, A., Yadzani, S., Yosaatmadja, Y., Aimon, A., Brandão-Neto, J., et al. (2021). Structure, Mechanism and Crystallographic Fragment Screening of the SARS-CoV-2 NSP13 Helicase. Nat. Commun. 12 (1), 4848. doi:10.1038/s41467-021-25166-6
Pakhrin, S. C., Shrestha, B., Adhikari, B., and Kc, D. B. (2021). Deep Learning-Based Advances in Protein Structure Prediction. Int. J. Mol. Sci. 22 (11). doi:10.3390/ijms22115553
Pontius, J., Richelle, J., and Wodak, S. J. (1996). Deviations from Standard Atomic Volumes as a Quality Measure for Protein crystal Structures. J. Mol. Biol. 264 (1), 121–136. doi:10.1006/jmbi.1996.0628
Rathi, P. C., Ludlow, R. F., and Verdonk, M. L. (2020). Practical High-Quality Electrostatic Potential Surfaces for Drug Discovery Using a Graph-Convolutional Deep Neural Network. J. Med. Chem. 63 (16), 8778–8790. doi:10.1021/acs.jmedchem.9b01129
Razonable, R. R. (2011). Antiviral Drugs for Viruses Other Than Human Immunodeficiency Virus. Mayo Clin. Proc. 86 (10), 1009–1026. doi:10.4065/mcp.2011.0309
Ren, J., Ding, Z., Fang, P., Xiao, S., and Fang, L. (2021). ATPase and Helicase Activities of Porcine Epidemic Diarrhea Virus Nsp13. Vet. Microbiol. 257, 109074. doi:10.1016/j.vetmic.2021.109074
Roy, A., Kucukural, A., and Zhang, Y. (2010). I-TASSER: a Unified Platform for Automated Protein Structure and Function Prediction. Nat. Protoc. 5 (4), 725–738. doi:10.1038/nprot.2010.5
Saikia, S., and Bordoloi, M. (2019). Molecular Docking: Challenges, Advances and its Use in Drug Discovery Perspective. Curr. Drug Targets 20 (5), 501–521. doi:10.2174/1389450119666181022153016
Santos, F. R. S., Lima, W. G., Maia, E. H. B., Assis, L. C., Davyt, D., Taranto, A. G., et al. (2020). Identification of a Potential Zika Virus Inhibitor Targeting NS5 Methyltransferase Using Virtual Screening and Molecular Dynamics Simulations. J. Chem. Inf. Model. 60 (2), 562–568. doi:10.1021/acs.jcim.9b00809
Scior, T., Bender, A., Tresadern, G., Medina-Franco, J. L., Martínez-Mayorga, K., Langer, T., et al. (2012). Recognizing Pitfalls in Virtual Screening: A Critical Review. J. Chem. Inf. Model. 52 (4), 867–881. doi:10.1021/ci200528d
Shen, B. (2015). A New Golden Age of Natural Products Drug Discovery. Cell 163 (6), 1297–1300. doi:10.1016/j.cell.2015.11.031
Shin, D., Mukherjee, R., Grewe, D., Bojkova, D., Baek, K., Bhattacharya, A., et al. (2020). Papain-like Protease Regulates SARS-CoV-2 Viral Spread and Innate Immunity. Nature 587 (7835), 657–662. doi:10.1038/s41586-020-2601-5
Shu, T., Huang, M., Wu, D., Ren, Y., Zhang, X., Han, Y., et al. (2020). SARS-Coronavirus-2 Nsp13 Possesses NTPase and RNA Helicase Activities that Can Be Inhibited by Bismuth Salts. Virol. Sin 35 (3), 321–329. doi:10.1007/s12250-020-00242-1
Sterling, T., and Irwin, J. J. (2015). ZINC 15--Ligand Discovery for Everyone. J. Chem. Inf. Model. 55 (11), 2324–2337. doi:10.1021/acs.jcim.5b00559
Stokes, J. M., Yang, K., Swanson, K., Jin, W., Cubillos-Ruiz, A., Donghia, N. M., et al. (2020). A Deep Learning Approach to Antibiotic Discovery. Cell 180 (4), 688–702. e13. doi:10.1016/j.cell.2020.01.021
Sundberg, S. A. (2000). High-throughput and Ultra-high-throughput Screening: Solution- and Cell-Based Approaches. Curr. Opin. Biotechnol. 11 (1), 47–53. doi:10.1016/s0958-1669(99)00051-8
Trott, O., and Olson, A. J. (2010). AutoDock Vina: Improving the Speed and Accuracy of Docking with a New Scoring Function, Efficient Optimization, and Multithreading. J. Comput. Chem. 31 (2), 455–461. doi:10.1002/jcc.21334
Wang D, D., Fang, L., Shi, Y., Zhang, H., Gao, L., Peng, G., et al. (2016). Porcine Epidemic Diarrhea Virus 3C-like Protease Regulates its Interferon Antagonism by Cleaving NEMO. J. Virol. 90 (4), 2090–2101. doi:10.1128/JVI.02514-15
Wang, P., Bai, J., Liu, X., Wang, M., Wang, X., and Jiang, P. (2020). Tomatidine Inhibits Porcine Epidemic Diarrhea Virus Replication by Targeting 3CL Protease. Vet. Res. 51 (1), 136. doi:10.1186/s13567-020-00865-y
Wang Z, Z., Sun, H., Yao, X., Li, D., Xu, L., Li, Y., et al. (2016). Comprehensive Evaluation of Ten Docking Programs on a Diverse Set of Protein-Ligand Complexes: the Prediction Accuracy of Sampling Power and Scoring Power. Phys. Chem. Chem. Phys. 18 (18), 12964–12975. doi:10.1039/c6cp01555g
Waterhouse, A., Bertoni, M., Bienert, S., Studer, G., Tauriello, G., Gumienny, R., et al. (2018). SWISS-MODEL: Homology Modelling of Protein Structures and Complexes. Nucleic Acids Res. 46 (W1), W296–W303. doi:10.1093/nar/gky427
Wu, Y., Zhang, H., Shi, Z., Chen, J., Li, M., Shi, H., et al. (2020). Porcine Epidemic Diarrhea Virus Nsp15 Antagonizes Interferon Signaling by RNA Degradation of TBK1 and IRF3. Viruses 12 (6). doi:10.3390/v12060599
Xie, Y., Guo, X., Hu, T., Wei, D., Ma, X., Wu, J., et al. (2021). Significant Inhibition of Porcine Epidemic Diarrhea Virus In Vitro by Remdesivir, its Parent Nucleoside and β-D-N4-hydroxycytidine. Virol. Sin 36 (5), 997–1005. doi:10.1007/s12250-021-00362-2
Xiong, G., Wu, Z., Yi, J., Fu, L., Yang, Z., Hsieh, C., et al. (2021). ADMETlab 2.0: an Integrated Online Platform for Accurate and Comprehensive Predictions of ADMET Properties. Nucleic Acids Res. 49 (W1), W5–W14. doi:10.1093/nar/gkab255
Xu, L. H., Huang, M., Fang, S. G., and Liu, D. X. (2011). Coronavirus Infection Induces DNA Replication Stress Partly through Interaction of its Nonstructural Protein 13 with the P125 Subunit of DNA Polymerase δ. J. Biol. Chem. 286 (45), 39546–39559. doi:10.1074/jbc.M111.242206
Yang J, J., Yan, R., Roy, A., Xu, D., Poisson, J., and Zhang, Y. (2015). The I-TASSER Suite: Protein Structure and Function Prediction. Nat. Methods 12 (1), 7–8. doi:10.1038/nmeth.3213
Yang Jl, J. L., Dhodary, B., Quy Ha, T. K., Kim, J., Kim, E., and Oh, W. K. (2015). Three New Coumarins from Saposhnikovia Divaricata and Their Porcine Epidemic Diarrhea Virus (PEDV) Inhibitory Activity. Tetrahedron 71 (28), 4651–4658. doi:10.1016/j.tet.2015.04.092
Yang, N., Tanner, J. A., Wang, Z., Huang, J. D., Zheng, B. J., Zhu, N., et al. (2007). Inhibition of SARS Coronavirus Helicase by Bismuth Complexes. Chem. Commun. (Camb) 42, 4413–4415. doi:10.1039/b709515e
Ye, G., Wang, X., Tong, X., Shi, Y., Fu, Z. F., and Peng, G. (2020). Structural Basis for Inhibiting Porcine Epidemic Diarrhea Virus Replication with the 3C-like Protease Inhibitor GC376. Viruses 12 (2). doi:10.3390/v12020240
Yuan, C., Huang, X., Zhai, R., Ma, Y., Xu, A., Zhang, P., et al. (2021). In Vitro Antiviral Activities of Salinomycin on Porcine Epidemic Diarrhea Virus. Viruses 13 (4), 580. doi:10.3390/v13040580
Yuen, C. K., Lam, J. Y., Wong, W. M., Mak, L. F., Wang, X., Chu, H., et al. (2020). SARS-CoV-2 Nsp13, Nsp14, Nsp15 and Orf6 Function as Potent Interferon Antagonists. Emerg. Microbes Infect. 9 (1), 1418–1428. doi:10.1080/22221751.2020.1780953
Zhang H, H., Han, F., Shu, X., Li, Q., Ding, Q., Hao, C., et al. (2021). Co‐infection of Porcine Epidemic Diarrhoea Virus and Porcine Deltacoronavirus Enhances the Disease Severity in Piglets. Transbounding Emerging Dis. doi:10.1111/tbed.14144
Zhang, Q., Ke, H., Blikslager, A., Fujita, T., and Yoo, D. (2018). Type III Interferon Restriction by Porcine Epidemic Diarrhea Virus and the Role of Viral Protein Nsp1 in IRF1 Signaling. J. Virol. 92 (4). doi:10.1128/JVI.01677-17
Zhang, Y. (2008). I-TASSER Server for Protein 3D Structure Prediction. BMC Bioinformatics 9, 40. doi:10.1186/1471-2105-9-40
Zhang Y, Y., Chen, H., Zou, M., Oerlemans, R., Shao, C., Ren, Y., et al. (2021). Hypericin Inhibit Alpha-Coronavirus Replication by Targeting 3CL Protease. Viruses 13 (9), 1825. doi:10.3390/v13091825
Zhao, J., Sun, L., Zhao, Y., Feng, D., Cheng, J., and Zhang, G. (2021). Coronavirus Endoribonuclease Ensures Efficient Viral Replication and Prevents Protein Kinase R Activation. J. Virol. 95. doi:10.1128/JVI.02103-20
Zhao, Y., Fang, C., Zhang, Q., Zhang, R., Zhao, X., Duan, Y., et al. (2021). Crystal Structure of SARS-CoV-2 Main Protease in Complex with Protease Inhibitor PF-07321332. Protein Cell 22, 1–5. doi:10.1007/s13238-021-00883-2
Zhavoronkov, A., Ivanenkov, Y. A., Aliper, A., Veselov, M. S., Aladinskiy, V. A., Aladinskaya, A. V., et al. (2019). Deep Learning Enables Rapid Identification of Potent DDR1 Kinase Inhibitors. Nat. Biotechnol. 37 (9), 1038–1040. doi:10.1038/s41587-019-0224-x
Keywords: ZINC12899676, PEDV replication, virtual screening, nsp13, NTPase, deep learning
Citation: Wang P, Wang X, Liu X, Sun M, Liang X, Bai J and Jiang P (2022) Natural Compound ZINC12899676 Reduces Porcine Epidemic Diarrhea Virus Replication by Inhibiting the Viral NTPase Activity. Front. Pharmacol. 13:879733. doi: 10.3389/fphar.2022.879733
Received: 28 February 2022; Accepted: 06 April 2022;
Published: 04 May 2022.
Edited by:
Salvatore Salomone, University of Catania, ItalyReviewed by:
Puxian Fang, Huazhong Agricultural University, ChinaAlex Malogolovkin, University of London, United Kingdom
Copyright © 2022 Wang, Wang, Liu, Sun, Liang, Bai and Jiang. This is an open-access article distributed under the terms of the Creative Commons Attribution License (CC BY). The use, distribution or reproduction in other forums is permitted, provided the original author(s) and the copyright owner(s) are credited and that the original publication in this journal is cited, in accordance with accepted academic practice. No use, distribution or reproduction is permitted which does not comply with these terms.
*Correspondence: Ping Jiang, jiangp@njau.edu.cn