- 1Department of Bioinformatics, Putuo People’s Hospital, Tongji University, Shanghai, China
- 2Guangdong Provincial Key Laboratory of Colorectal and Pelvic Floor Diseases, Department of Colorectal Surgery, The Sixth Affiliated Hospital, Guangdong Institute of Gastroenterology, Sun Yat-sen University, Guangzhou, China
- 3National Clinical Research Center for Child Health, The Children’s Hospital, Zhejiang University School of Medicine, Hangzhou, China
- 4State Key Laboratory of Biotherapy, West China Hospital, Sichuan University and Collaborative Innovation Center, Chengdu, China
- 5School of Medicine, Sun Yat-sen University, Shenzhen, China
Background: The pathological differences between Crohn’s disease (CD) and ulcerative colitis (UC) are substantial and unexplained yet. Here, we aimed to identify potential regulators that drive different pathogenesis of CD and UC by causal inference analysis of transcriptome data.
Methods: Kruskal–Wallis and Dunnett’s tests were performed to identify differentially expressed genes (DEGs) among CD patients, UC patients, and controls. Subsequently, differentially expressed pathways (DEPs) between CD and UC were identified and used to construct the interaction network of DEPs. Causal inference was performed to identify IBD subtype-regulators. The expression of the subtype-regulators and their downstream genes was validated by qRT-PCR with an independent cohort.
Results: Compared with the control group, we identified 1,352 and 2,081 DEGs in CD and UC groups, respectively. Multiple DEPs between CD and UC were closely related to inflammation-related pathways, such as NOD-like receptor signaling, IL-17 signaling, and chemokine signaling pathways. Based on the priori interaction network of DEPs, causal inference analysis identified IFNG and GBP5 as IBD subtype-regulators. The results with the discovery cohort showed that the expression level of IFNG, GBP5, and NLRP3 was significantly higher in the CD group than that in the UC group. The regulation relationships among IFNG, GBP5, and NLRP3 were confirmed with transcriptome data from an independent cohort and validated by qRT-PCR.
Conclusion: Our study suggests that IFNG and GBP5 were IBD subtype-regulators that trigger more intense innate immunity and inflammatory responses in CD than those in UC. Our findings reveal pathomechanical differences between CD and UC that may contribute to personalized treatment for CD and UC.
Introduction
Inflammatory bowel disease (IBD) is a chronic, nonspecific, and inflammatory intestinal disease that causes significant morbidity to patients and an enormous burden to the society (Britzen-Laurent et al., 2016). The last decades have seen increased prevalence of IBD, especially in newly industrialized countries, whose number of cases increased from 3.8 million in 1990 to more than 6.8 million in 2017 with a growth rate of 85.1% (GBD 2017 Inflammatory Bowel Disease Collaborators, 2020; Kaplan and Ng, 2017). Though the mechanism still remains elusive, substantial investigations have revealed that multiple factors, including genetic susceptibility, intestinal epithelial barrier deficiency, immunological dysfunction, and nervous system dysregulation and the interactions among them, contribute to the pathogenesis of IBD (Knights et al., 2013).
Crohn’s disease (CD) and ulcerative colitis (UC), two major subtypes of IBD, have significantly different clinical presentations and markedly distinct disease courses. The manifestations of CD are discontinuous transmural asymmetric lesions which can affect the entire alimentary canal, while UC is characterized by the diffuse inflammation that is confined to the superficial mucosa of the colon, with possible involvement of the rectum (Bernstein et al., 2016). Currently, endoscopy is commonly used for the differential diagnosis of CD and UC, with a risk of bowel perforation (Kumar et al., 2019). Moreover, it is nowadays challenging to choose the best diagnostic test and the treatment protocol for the management of either CD or UC, which often results in unsatisfactory patient outcomes. Therefore, it is critical to understand the disease signature for each subtype in order to provide the most appropriate and personalized treatment for IBD patients. Previous studies suggested that the genetic biomarkers, namely, nucleotide-binding oligomerization domain-containing protein 2 (NOD2), immunity-related GTPase M (IRGM), and autophagy-related gene (ATG16L1) are specific for CD while interleukin 10 (IL10) and the human leukocyte antigen (HLA) are specific for UC (Uniken Venema et al., 2017). Importantly, susceptibility genes could cause the dysfunction of the intestinal epithelium and immune system, which leads to the invasion of gut microbiota. For instance, some studies reported that the deficiencies in susceptibility genes NOD2 and ATG16L1 may contribute to the IBD risk, due to the impaired anti-inflammatory responses and the further defects in microbial clearance (Chu et al., 2016). Furthermore, based on colon transcriptome, our previous study revealed that the colonic immunity of CD and UC is differently activated toward various pathogens (Yang et al., 2019). Overall, due to the complex interactions between genes and phenotypes, the driving force that causes the different IBD subtypes was still not identified, which is the goal of the current study.
Here, we took a new approach that integrates the quantitative pathway analysis (QPA) and causal inference. QPA is an approach that retains information of genes in pathway analysis and compares pathways between groups through dimension reduction and mapping of data. QPA has been successfully applied in several studies, demonstrating its advantage over the method of pathway enrichment analysis (Ren et al., 2018; Gao et al., 2020). With QPA, we identified differentially expressed pathways (DEPs) between IBD and control. Based on the priori network constructed with DEPs, causal inference was performed to identify the key factors that drive the different pathogenesis of CD and UC. Figure 1 is the overall workflow of this study.
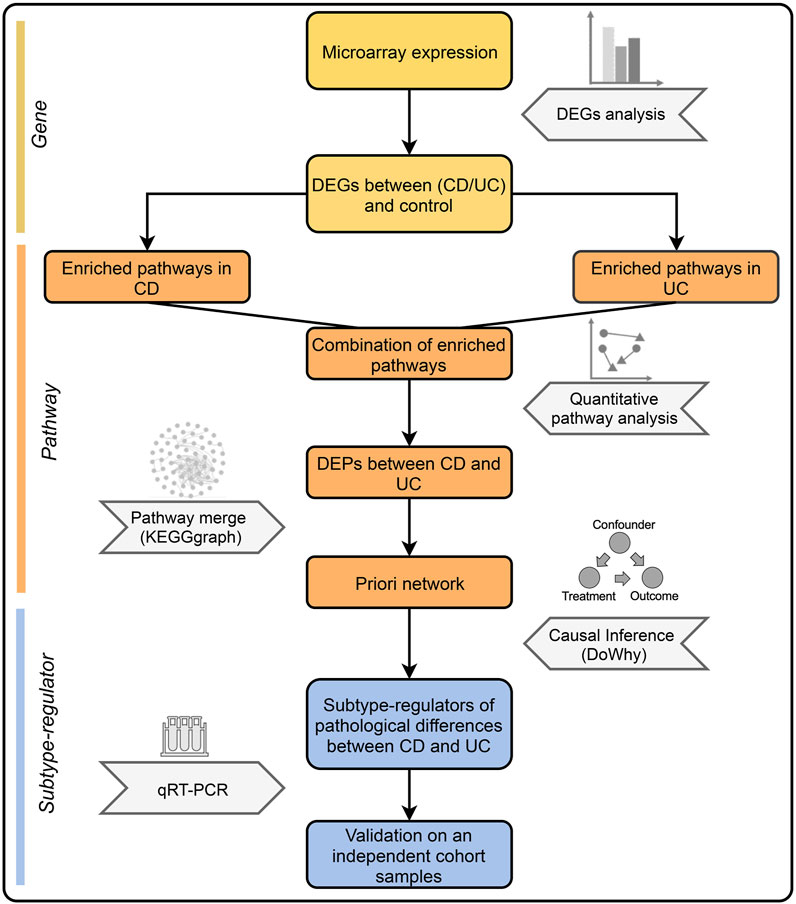
FIGURE 1. Overall workflow of this study. First, DEGs between IBD and control groups were identified. Next, the combination of enriched pathways was compared between CD and UC by QPA to determine DEPs. Subsequently, DEPs were merged into a priori network, which was used to identify the subtype-regulators using causal inference. Finally, validation was performed by qRT-PCR with an independent cohort.
Materials and Methods
Study Design
Datasets GSE38713 (Planell et al., 2013) and GSE52746 (Leal et al., 2015) generated using Affymetrix Human Genome U133 Plus 2.0 Array were selected as a discovery cohort to identify the DEGs between IBD and control groups. The selected datasets were generated in the same laboratory, minimizing potential batch effects due to different experimental conditions. A total of 10 CD, 15 UC, and 13 control samples were selected from the two datasets according to the following inclusion criteria: similar sample sizes of different groups, similar female to male ratio, and age ranges (Supplementary Tables S1, S2). Additionally, we selected transcriptome data of colonic tissues from an independent cohort (GSE111889) as a validation cohort (25 of CD, 22 of UC, and six of control).
Quantitative real-time polymerase chain reaction (qRT-PCR) was performed on samples of an independent cohort. Patients with an established diagnosis of CD and UC were included in the study. The subjects excluded from IBD based on endoscopic and histopathologic findings were classified as “non-IBD”controls. Potential participants were excluded if they were unable to or did not consent to provide tissue, were pregnant, were diagnosed with indeterminate colitis, or had an acute gastrointestinal infection. The tissue samples (N = 36 for CD, N = 13 for UC, and N = 33 for control, Supplementary Tables S3, S4) were collected at the time of colonoscopy. Sigmoid colon biopsies were obtained and stored in a freezer at −80°C after soaking with RNAlater (Sigma-Aldrich). The study was approved by the Institutional Review Board at The Sixth Affiliated Hospital of Sun Yat-sen University, Guangzhou, and each participant provided informed consent.
Preprocessing of Microarray Data
MicroArray Suite 5 method was used to process the microarray raw data. “Median values of housekeeping genes adjustment” (Vandesompele et al., 2002) was used to eliminate the batch effects from two datasets. First, analysis of variance (ANOVA) was performed to identify stable reference genes among CD, UC, and control groups from the 3,804 reported housekeeping genes (Eisenberg and Levanon, 2013). Subsequently, according to the descending order of Benjamini and Hochberg adjusted P of ANOVA, top 100 housekeeping genes were defined as correcting reference genes (CRGs), which were used to eliminate batch effects. Afterward, a sample(i) was randomly selected as a reference sample. To correct the expression of sample(j), the ratio of CRG median values of sample(i) and sample(j) was defined as the correcting coefficient for the sample(j). Finally, the expressions of genes in each sample were corrected by multiplying by the corresponding correcting coefficient, as detailed in the following formulas:
Differentially Expressed Gene (DEG) Analysis
Considering the non-normal distribution of the transcriptome data, non-parametric Kruskal–Wallis tests were used to test whether samples were originated from the same distribution. Subsequently, post hoc Dunnett’s tests were performed to determine DEGs between IBD (CD or UC) and control groups (|log2(Fold Change)| > 1 and p < 0.05).
Quantitative Pathway Analysis (QPA)
QPA identifies DEPs among groups by projecting high-dimensional pathway data onto the same dimension for comparison. This approach has been successfully applied in previous studies (Ren et al., 2018; Gao et al., 2020). Here, QPA was used to examine the differences between CD and UC at the pathway level. Briefly, we first performed pathway enrichment via clusterProfiler (Yu et al., 2012) against the KEGG (Kyoto Encyclopedia of Genes and Genomes) database. Then, the combination of those enriched pathways between IBD (CD or UC) and control groups was compared between CD and UC. QPA was then performed as previously described (Gao et al., 2020), including 3 steps: pathway quantification, pathway comparison, and significance evaluation by the permutation test, which generates the list of DEPs between CD and UC.
IBD Subtype-Regulator Analysis Using Causal Inference
We performed causal inference using the DoWhy (https://microsoft.github.io/dowhy/) causal inference framework to identify IBD subtype-regulators that mediate different pathogenesis of CD and UC, following an algorithm described previously (Wu et al., 2020). Based on the priori knowledge of biological interaction relationship from KEGG database, we constructed a priori network by merging all DEPs via KEGGgraph R package (Zhang and Wiemann, 2009). The causal effects of the DEGs between IBD (CD or UC) and control groups in the priori network were assessed with causal estimate values (CEV) of genes on driving different IBD subtypes. CEV represents the amount of change in the outcome value after intervention and the change of the treatment. Furthermore, p values were computed by the permutation test with 1,000 permutations to determine significant causalities. Genes with significant statistical differences between IBD subtypes and significant causal effects (p < 0.05) were considered as IBD subtype-regulators.
Messenger RNA Expression by qRT-PCR
Total RNA was isolated using the Tissue RNA Purification Kit Plus (ES Science). RNA was reverse transcribed to cDNA using the Fast Reverse Transcription kit (ES Science) following the manufacturer’s instruction. qRT-PCR assays were performed using LightCycler 96 (Roche). The reaction volume was 10 μl in total including 1 μl cDNA, 0.5 μl forward and reverse primer pairs, 5 μl FastStart Essential DNA Green Master (Roche), and 3.5 μl RNase free water. The primer sequences of each gene were listed in Supplementary Table S5. β-Actin was used as the housekeeping gene. The relative expressions of these genes were calculated using the 2−ΔΔCT method.
Statistical Analysis
Differences among 3 groups were tested using one-way analysis of variance and post hoc Dunnett’s multiple comparison test. p value less than 0.05 was considered significant.
Results
Microarray Dataset Characterization
To examine the gene expression profiles of IBD, two microarray datasets of colonic biopsies were selected: GSE38713 and GSE52746 (Planell et al., 2013; Leal et al., 2015). To eliminate potential batch effects, we normalized gene expressions using the method of “median values of housekeeping genes adjustment” with CRGs. To validate this approach and to ascertain the quality of the data, we examined the adjusted expression levels of the housekeeping genes and some of the known IBD feature genes. As expected, the expression levels of two housekeeping genes, namely, glyceraldehyde 3-phosphate dehydrogenase (GAPDH) and actin beta (ACTB) were similar between IBD and control groups (Figure 2A), while the expression of four IBD feature genes, namely, lipocalin-2 (LCN2) (Cayatte et al., 2012), matrix metallopeptidase 9 (MMP9) (Farkas et al., 2015), interleukin 6 (IL6) (Atreya and Neurath, 2005), and S100 calcium-binding protein A12 (S100A12) (Nahidi et al., 2011) was highly elevated in CD and UC (Figure 2B).
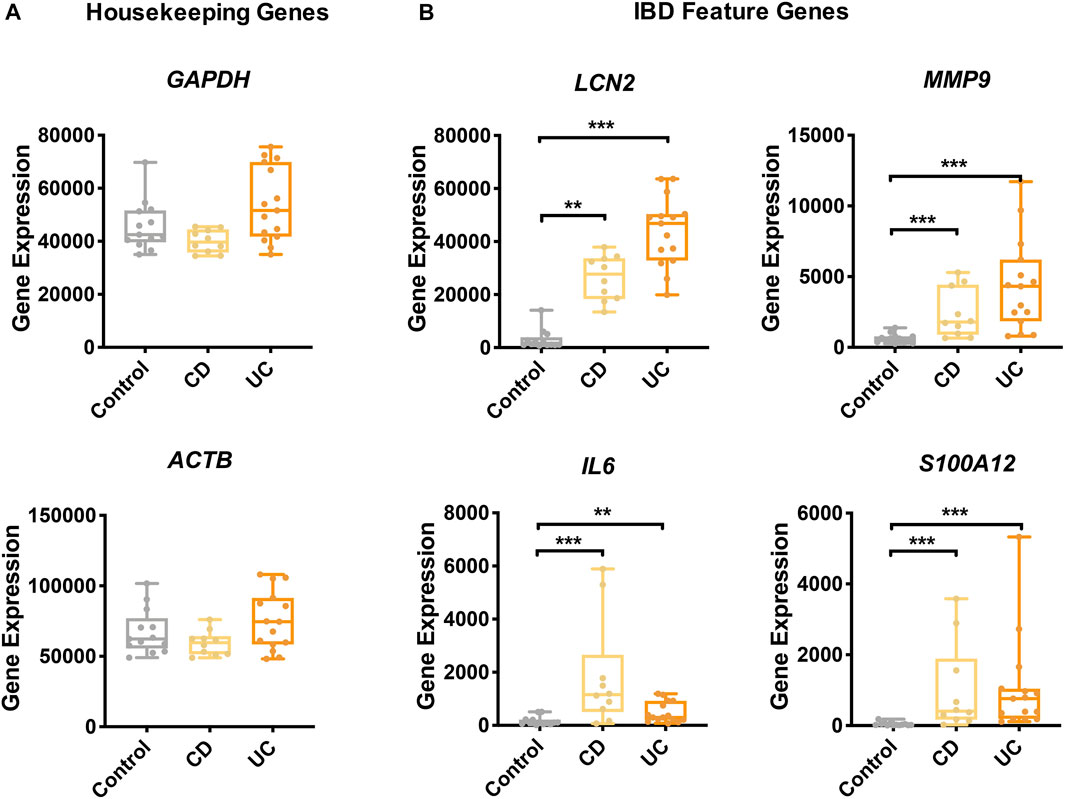
FIGURE 2. Gene expression of IBD feature genes and housekeeping genes. The expression of housekeeping genes (A) and feature genes of IBD (B) was compared among the study groups. Yellow, CD; orange, UC; gray, control. Data are presented as mean ± standard error. *: p < 0.05, **: p < 0.01, ***: p < 0.001, Dunnett’s test.
The DEGs Between IBD and Control Groups and the DEG-Enriched Pathways
To identify the potential pathological mechanisms of IBD, we compared the transcriptome profiles of IBD (CD or UC) and control groups. At the gene level, compared to the control group, there were 1,352 and 2081 genes differentially expressed in CD and UC, respectively. In the CD group, among the 784 up-regulated genes, RCN1 and IFI6 were the most significantly elevated DEGs, while SEPW1 and DTX4 were the most significantly decreased DEGs among the 568 down-regulated genes (Figure 3A). Similarly, in the UC group, DUOX2 and PI3 were the most significantly elevated DEGs out of the 1,421 up-regulated genes, whereas RHOU and OSBPL1A were the most significantly decreased DEGs among the 660 down-regulated genes (Figure 3C). Among all the DEGs, there were a total of 657 DEGs (Supplementary Figure S1A), including 533 up-regulated (Supplementary Figure S1B) and 112 down-regulated genes (Supplementary Figure S1C), that were common in CD and UC groups. Notably, the majority of the DEGs were CD- or UC-specific, suggesting the distinct pathological mechanisms of the two IBD subtypes.
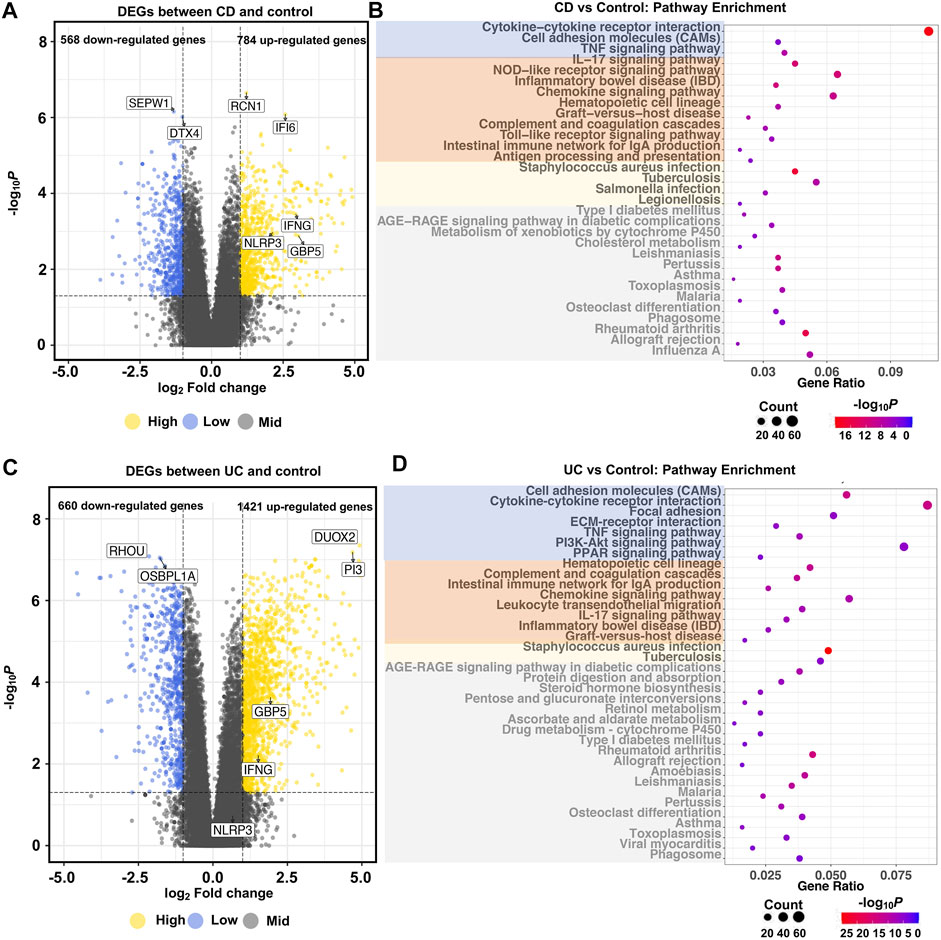
FIGURE 3. Global transcriptional changes of gene profiles and KEGG pathway enrichment in IBD. Volcano plots show the differentially expressed genes between CD (A), UC (C), and control groups (|log2Fold Change| >1 and adjusted p < 0.05). Dot plots show the pathways significantly enriched with DEGs between CD (B), UC (D), and control groups (adjusted p < 1.0e-3). N = 10, 15, and 13 for the CD, the UC, and the control groups, respectively. In the dot plots, signaling molecules interaction and immunity- and bacterial infection-related pathways are marked by blue, orange, and yellow, respectively. Irrelevant enriched pathways are marked by gray.
At the pathway level, pathway enrichment analysis identified 58 (Figure 3B) and 92 KEGG pathways (Figure 3D) based on the CD- and UC-specific DEGs, respectively. In contrast to the DEG results, most of the enriched pathways were common in CD (52 out of 58) and UC (52 out of 92) (Supplementary Figure S1D). These common pathways are related to signaling molecules interaction, or signaling transduction, such as cytokine–cytokine receptor interaction, cell adhesion molecules (CAMs), and bacterial infection-related pathways, such as tuberculosis, legionellosis, and Staphylococcus aureus infection. Importantly, pathways related to immune and inflammatory reactions, including the IL-17 signaling pathway, NOD-like receptor signaling pathway, toll-like receptor signaling pathway, and chemokine signaling pathway were enriched in both CD and UC. These similar biological functions reflected the common inflammatory characteristics of CD and UC. On the other hand, some inflammatory-related pathways exhibited different activities between CD and UC. For example, the NOD2 signaling pathway was only enriched in CD. This is interesting as NOD2 is an important innate immune sensor implicated in host defense in CD (Strober and Watanabe, 2011). Some pathways such as ECM-receptor interaction and PPAR signaling pathway were only enriched in UC. ECM remodeling is a decisive feature of the progression of IBD, and ECM degradation by proteases could participate in the dysfunction of intestinal barrier and inflammation in IBD (Petrey and de la Motte, 2017). PPARγ, a key molecule in the PPAR signaling pathway, is a nuclear receptor that drives bacterial-induced inflammation (Dubuquoy et al., 2006; Dou et al., 2015). These differential pathways between CD and UC suggest that IBD subtypes are different in immune and intestinal barrier-related pathomechanisms.
DEPs Between CD and UC
Next, we investigated the differences at the pathway level between CD and UC via QPA. Here, we began with the pathways identified from the enrichment analysis, and a total of 98 pathways were included, which is the combination of enriched pathways in CD and UC. According to QPA, seven out of 98 pathways were significantly differentially expressed between CD and UC, including the NOD-like receptor signaling pathway, Staphylococcus aureus infection, IL-17 signaling pathway, rheumatoid arthritis, TNF signaling pathway, transcriptional misregulation in cancer, and chemokine signaling pathway (Supplementary Table S6). The DEPs between CD and UC were predominantly related to cytoplasmic recognition, pro-inflammatory cytokines, and cytokine interactions, which play important roles in innate immunity and inflammatory responses. We, then, examined the expression of individual DEGs in the DEPs (Figure 4). The samples were clearly clustered into three clusters consistent with the disease status, which confirmed the correlation of the DEPs with the subtypes of IBD. Compared to the control group, the expression levels of these DEGs were generally higher in IBD (CD and UC). In addition, the gene expressions of some pathways in CD were higher than those in UC, such as genes in the NOD-like receptor signaling pathway, IL-17 signaling pathway, and chemokine signaling pathway. The distinct expression levels of these DEGs in the DEPs between CD and UC suggested that these genes are related to the pathological differences between IBD subtypes.
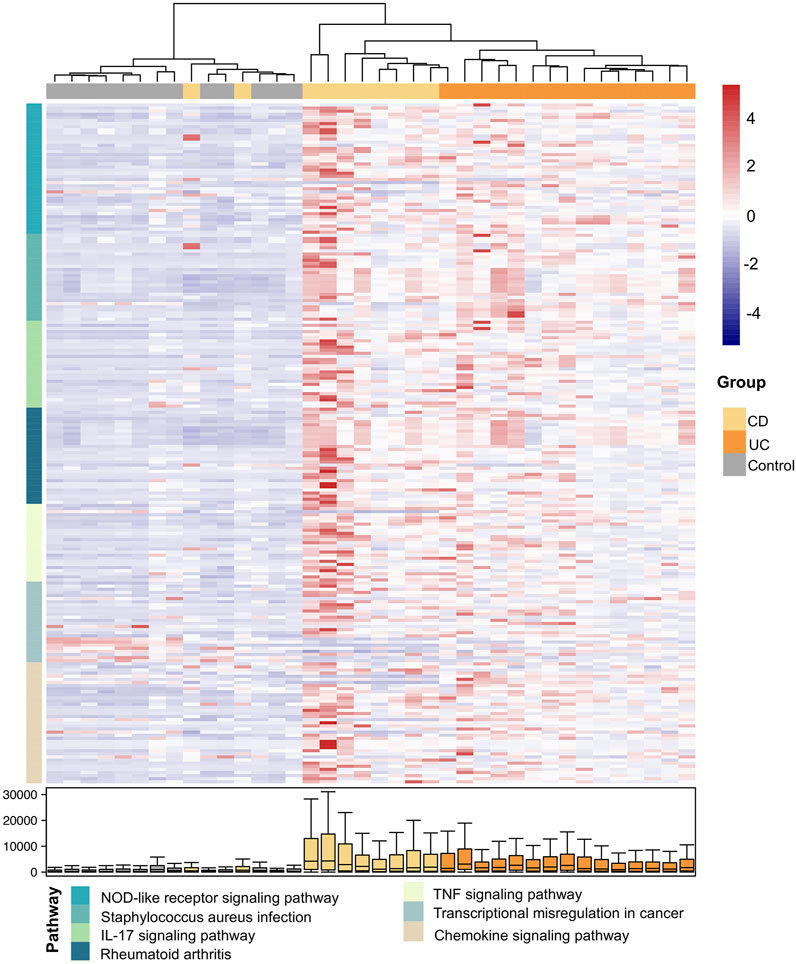
FIGURE 4. Expression heatmap of the DEGs in the DEPs between CD and UC. The samples and the DEGs are presented in columns and rows, respectively. The color of the DEGs in rows indicates different DEPs as specified in the following (pathway). The color bar on the right side indicates the scaled expressions of these genes in each sample. The distribution of the expressions for each DEG is shown by boxplot at the bottom.
IBD Subtype-Regulators
To identify the regulators that drive the differential pathogenesis of IBD subtypes, we performed causal inference against a priori network with established regulation relationships among genes. The priori network is composed of 709 nodes and 2,146 edges (Supplementary Figure S2) based on the DEPs generated in QPA. As a result, 27 DEGs were identified as causal DEGs (p < 0.05) responsible for the differences between CD and UC. The causal estimate values (CEVs) of the genes were shown in Supplementary Table S7. We, then, defined eight causal DEGs that were differentially expressed between subtypes as IBD subtype-regulators (Supplementary Table S8), including guanylate-binding protein 5 (GBP5), defensin beta 1 (DEFB1), interferon gamma (IFNG), C–X–C motif chemokine ligand 10 (CXCL10), lymphotoxin beta (LTB), prostaglandin-endoperoxide synthase 2 (PTGS2), suppressor of cytokine signaling 3 (SOCS3), and C–C motif chemokine ligand 3 (CCL3). Combined the results of DEP analysis with those from causal inference analysis, we found that the NOD-like receptor signaling pathway, as the most significant DEPs, was enriched with three subtype-regulators, namely, IFNG, GBP5, and NLRP3 that work together from upstream to downstream in the regulation of immune response, which indicated the important roles of the three subtype-regulators in the pathway. Therefore, we extracted and depicted a subnetwork of the priori DEP network by retrieving IFNG, GBP5, and NLRP3 and their two most adjacent genes in the NOD-like receptor signaling pathway (Figure 5).
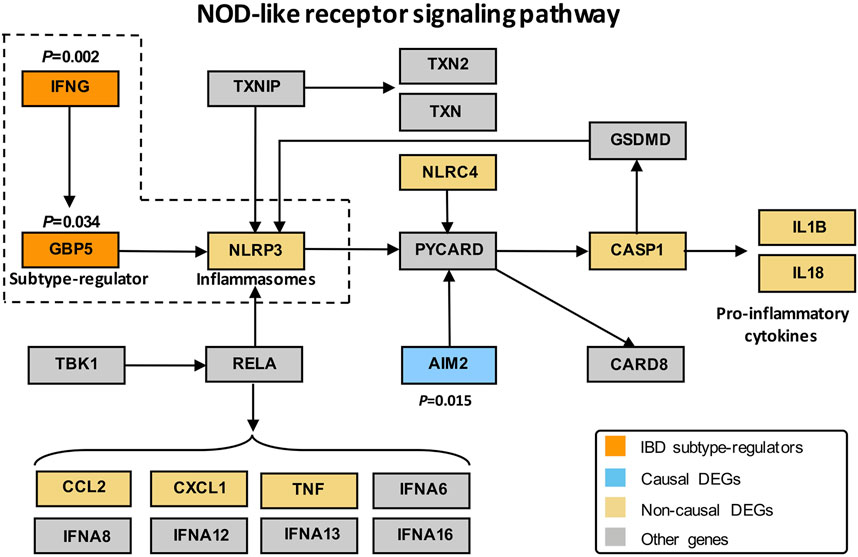
FIGURE 5. Subnetwork of the DEPs KEGG network with IFNG, GBP5, and NLRP3. Orange, blue, yellow, and gray represent subtype-regulators, causal DEGs, non-causal DEGs, and other genes, respectively, in DEPs. DEGs refer to differentially expressed genes between IBD and control groups. Subtype-regulators are DEGs with p < 0.05 in the causal effect significance test (see Method for detail) and showed statistical differences between CD and UC; causal DEGs represent DEGs with p < 0.05 in the causal effect significance test; non-causal DEGs represent DEGs with p > 0.05 in the causal effect significance test.
For a closer examination of the expression of IFNG, GBP5, and NLRP3, our transcriptome data showed that IFNG (Figure 6A), GBP5 (Figure 6B), and NLRP3 (Figure 6C) were significantly up-regulated in CD than those in UC and control groups. Additionally, the expressions of GBP5 were positively correlated with those of IFNG (Figure 6D) and NLRP3 (Figure 6E), respectively (Spearman, p < 0.05). The expressions of IFNG and those of NLRP3 were also positively correlated (Figure 6F). Regression analysis was also performed with IFNG, GBP5, and NLRP3 in CD, UC, and controls, respectively (Supplementary Figure S3). Thus, our data indicated a sequential cause of relationships from IFNG to GBP5, and then to NLRP3. With the validation dataset, the expression of IFNG, GBP5, and NLRP3 was consistent with that in the discovery datasets (Supplementary Table S9), and the regression analysis also demonstrated similar relationships among these three genes (Supplementary Figure S4).
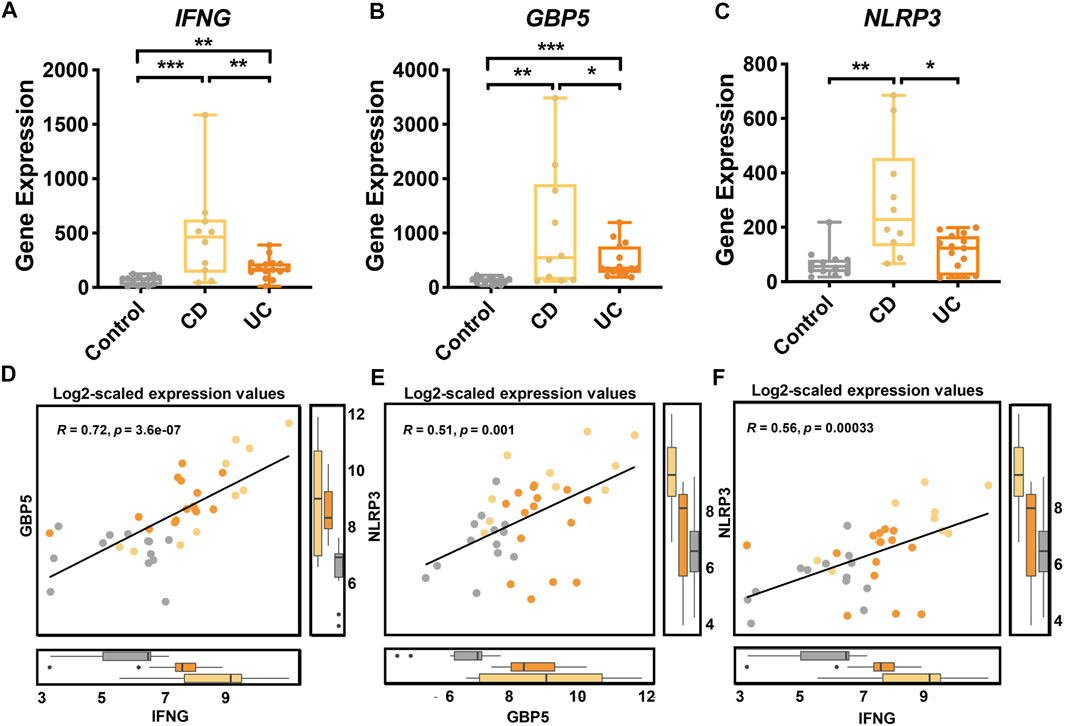
FIGURE 6. Gene expression of IFNG, GBP5, and NLRP3 in healthy controls, CD, and UC patients according to transcriptome analysis. The expression of (A) IFNG, (B) GBP5, and (C) NLRP3 genes in the control, CD, and UC groups. Yellow, CD; orange, UC; gray, control. All data are presented as mean ± standard error. *: p < 0.05; **: p < 0.01; ***: p < 0.001. N = 10, 15, and 13 for the CD, the UC, and the control groups, respectively. (D) Linear regression between IFNG and GBP5 based on log2-scaled expression values (Spearman; R = 0.72, p = 3.6e-07). (E) Linear regression between GBP5 and NLRP3 based on log2-scaled expression values (Spearman; R = 0.51, p = 0.001). (F) Linear regression between IFNG and NLRP3 based on log2-scaled expression values (Spearman; R = 0.56, p = 0.00033).
Furthermore, we examined the expression of pro-inflammatory cytokines interleukin 1 beta (IL1B) and interleukin 18 (IL18) that are at the endpoint of NLRP3 inflammasome activation. According to our transcriptome data, the expression of both IL1B and IL18 was significantly higher in CD than those in UC and control groups, and both IL1B and IL18 were positively correlated with NLRP3 (Supplementary Figure S5). These results support a unique role for NLRP3 inflammasome in CD compared to UC.
Validation of the Differential Expression of IBD Subtype-Regulators by qRT-PCR
We validated the differential expression of IFNG, GBP5, and NLRP3 using qRT-PCR on an independent cohort of healthy controls, CD and UC patients (Supplementary Table S9). Compared to the UC and the control groups, the expression of the IFNG was significantly higher in the CD group (Figure 7A; p < 0.05 and p < 0.001, respectively). The expression of GBP5 showed a similar pattern in three groups, and was significantly higher in the CD group than that in the UC and the control group (Figure 7B; p < 0.01 and p < 0.001, respectively). The expression of NLRP3 was significantly higher in the CD group than that in the control group (Figure 7C; p < 0.05). Overall, the PCR results were consistent with the transcriptome data that the up-regulation of IFNG, GBP5, and NLRP3 was more significant in CD than that in UC. Again, significant correlations in gene expression levels were observed among IFNG, GBP5, and NLRP3 (Figures 7D–F). With qRT-PCR data, regression analysis showed that correlations among IFNG, GBP5, and NLRP3 in CD were more significant than those in UC (Supplementary Figure S6).
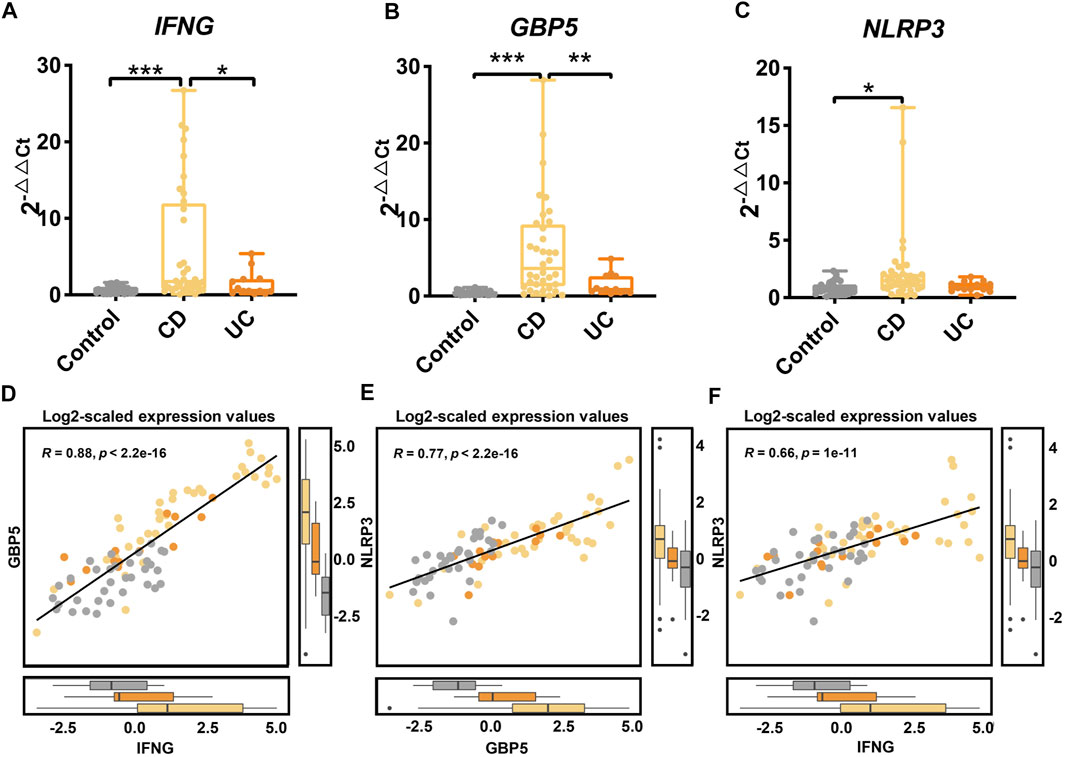
FIGURE 7. Gene expression of IFNG, GBP5, and NLRP3 in an independent cohort of healthy controls, CD, and UC patients as determined by qRT-PCR. The gene expressions of (A) IFNG, (B) GBP5, and (C) NLRP3 in the control, the CD, and the UC patients. Data are presented as mean ± standard error. *p < 0.05, **p < 0.01, and ***p < 0.001. N = 36, 13, and 33 for the CD, the UC, and the control groups, respectively. (D) Linear regression between IFNG and GBP5 based on log2-scaled expression values (Spearman, R = 0.88, p < 2.2e-16). (E) Linear regression between GBP5 and NLRP3 based on log2-scaled expression values (Spearman, R = 0.77, p < 2.2e-16). (F) Linear regression between IFNG and NLRP3 based on log2-scaled expression values (Spearman, R = 0.66, P = 1e-11).
Discussion
In the present study, we identified IFNG and GBP5 as IBD subtype-regulators that play key roles at the early steps of the pathogenesis of CD and UC using causal inference. IFNG and GBP5 may drive the pathological differences between CD and UC by up-regulating NLRP3. That is, NLRP3 is more intensely induced by IFNG/GBP5 in CD than that in UC. These findings facilitate our understanding of the pathological mechanisms of CD and UC and provide a foundation for the personalized medicine for IBD patients. We identified IFNG and GBP5 as IBD subtype-regulators.
Many studies have investigated possible pathological mechanisms of CD and UC (Ramos and Papadakis, 2019; Kaur and Goggolidou, 2020). However, it is unclear what triggers the phenotypic differences between CD and UC. In transcriptome studies, the biological relationships could be masked by irrelevant impacts (confounders) and indirect effects (mediators) (Vansteelandt and Vanderweele, 2012). Thus, it is difficult to identify IBD subtype-regulators through simple statistical analysis such as correlation analysis. Causal inference is a technique used for extracting causal estimates from observational data with strong exactness and interpretability, providing key insights by studying how behaviors or treatments affect the outcomes of interest, and has been increasingly employed to investigate the relationship between microbiota and host diseases (Goren et al., 2021; Lv et al., 2021). To better explore the complex mechanisms within IBD, we applied causal inference to analyze transcriptome data and unveil the potential regulators of IBD subtypes in this study.
In order to obtain disease-related priori knowledge for causal inference, we used QPA to identify latent differences between CD and UC at the pathway level. The NOD-like receptor signaling pathway, IL-17 signaling pathway, TNF signaling pathway, and chemokine signaling pathway were demonstrated as DEPs between CD and UC. These results indicate that differences between CD and UC indeed exist and they may contribute to the different clinical presentations between CD and UC. Among the DEPs, the most significant DEP, the NOD-like receptor signaling pathway (p = 0.020) plays a crucial role in maintaining the innate immune response to microbiota in the intestine by inducing inflammatory and chemotactic molecules such as IL1B and IL18 (Franchi et al., 2009). Many studies have demonstrated that NOD-like receptors (NLRs) are closely related to the the intestinal mucosal barrier which prevents the invasion of gut microbiota (Zhong et al., 2013). Particularly, NLRP3, a member of NLRs family, has received much attention in the studies of the pathological mechanism of IBD (Wang et al., 2020). NLRP3 is a critical gene in the NOD-like receptor signaling pathway that contributes to the formation of the NLRP3 inflammasome in immune cells, which could cause CASP1-mediated proteolytic activation of IL1B and IL18, and induces inflammation, and pyroptotic cell death in IBD (Tourkochristou et al., 2019). Our transcriptome data also indicated that both IL1B and IL18 were highly expressed in CD. In contrast, IL18, but not IL18 was highly expressed in UC. The positive correlations of the NLRP3 gene with its downstream pro-inflammatory cytokines (IL1B and IL18) provide further evidence for the up-regulation of NLRP3 inflammasome activity in CD. Thus, these results demonstrated that the NLRP3 inflammasome was more activated in CD than that in UC. In line with our observations, a previous study reported the higher activation of NLRP3 inflammasome in CD patients than in UC and control (Lazaridis et al., 2017). These observations support a role for NLRP3 in distinct inflammatory activities between CD and UC. Moreover, many studies have revealed that microbiome composition could be impacted by NLRP3 inflammasome (Yao et al., 2017; Zhang et al., 2019), which demonstrated its important role in regulating microbiome balance in the intestine.
However, NLRP3 was not identified as an IBD subtype-regulator with a CEV = 4.78e-6 and a p = 0.45 after eliminating the irrelevant impacts and indirect effects from other genes in the regulation network. Instead, the upstream genes IFNG (CEV = 0.004, p = 0.002) and GBP5 (CEV = 0.0009, p = 0.034) showed strong causal effects on IBD subtypes. Our result is supported by the reports that IFNG induces GBP5 (Fujiwara et al., 2016), which in turn activates the NLRP3 inflammasome with key roles in innate immunity and overall inflammation (Bergantz et al., 2019).
IFNG is a signature pro-inflammatory cytokine secreted by infected cells. It activates the innate immune response that promotes not only cytokine production but also natural killer cell functions and antigen presentation (Kopitar-Jerala, 2017). The previous study has demonstrated that IFNG plays key roles in the initiation of IBD, and the IFNG deficient mice did not develop DSS-induced colitis (Ito et al., 2006). Importantly, the increased levels of IFNG were found in the inflamed mucosa of CD patients but a normal level of IFNG was found in UC (Neurath et al., 2002). On the other hand, GBP5 is highly inducible by pro-inflammatory cytokines (Fujiwara et al., 2016), including IFNG, and is a marker of IFNG-induced activated macrophages. Our results showed that IFNG and its downstream gene GBP5 were highly expressed in CD than in UC and control. Therefore, the different expression levels of IFNG and GBP5 between CD and UC could be the key factor driving different disease manifestations of CD and UC. Moreover, many studies have revealed that the microbiome composition was different between CD and UC (Sankarasubramanian et al., 2020), which might be the consequence of distinct host immunity. When recognizing microbial antigens, different intensities of host immune responses might lead to the differences of immune cells activation, cytokines secretion, and severity of inflammation, which further impact the environment of gut microorganisms. Therefore, the differences in immune responses caused by IFNG, GBP5, and NLRP3 may contribute to the differences in microbiome composition between IBD subtypes.
Interestingly, DEFB1, CCL3, CXCL10, LTB, PTGS2, and SOCS3 were also identified as IBD subtype-regulators. In our transcriptome data, the expression of CCL3, CXCL10, LTB, PTGS2, and SOCS3 were up-regulated, whereas the expression of DEFB1 was down-regulated in CD and UC compared to the control. DEFB1 is known as a multifunctional mediator in infection and inflammation. The expression of DEFB1 can be up-regulated by LPS, IL-1β, IFN-γ, and arginine. However, a study has demonstrated that the impaired DEFB1 expression in colonic CD is not linked to inflammation-associated tissue damage (Peyrin-Biroulet et al., 2010). CCL3 is a chemokine related to neutrophils. The early release of CCL3 by neutrophils plays roles in the development of a protective immune response (Charmoy et al., 2010). Similarly, CXCL10 is a CXC chemokine in the DEP chemokine signaling pathway that is highly expressed in both CD and UC (Banks et al., 2003). CXCL10 contributes to systemic inflammation, colonic Th1 recruitment, and adaptive immunity in colitis. Our transcriptome data showed that the expression levels of both CCL3 and CXCL10 were increased significantly in CD compared to UC, suggesting that the differential expressions of these genes have strong causal relationships with different innate immune responses between CD and UC. LTB is a pro-inflammatory lipid mediator that could recruit leukocytes to the injury site and plays a pathogenic role in inflammation. PTGS2, also known as COX2, plays a critical role in regulating inflammatory responses (Hellmann et al., 2015). However, the roles of LTB and PTSG2 in IBD still remain undefined. Moreover, we found that SOCS3 increased in both CD and UC, although the over-expression of SOCS3 has been reported to be important for limiting inflammatory responses (Li et al., 2012). The increased expression of SOCS3 might be a response to the excessive inflammation in the intestine instead of a cause for different pathogenesis of CD and UC. In summary, these IBD subtype-regulators provide new insight into the diagnosis of IBD subtypes and facilitate our understanding of the pathological mechanisms of CD and UC.
We acknowledge the limitation that the sample size of this study is relatively small due to the lack of data with sufficient quality. Although it would not affect our conclusions, further evidence from larger IBD cohorts is needed. Nevertheless, with the application of QPA and causal inference, this study provides a paradigm of identifying key regulators of disease based on a quantitative causal analysis of transcriptome data, enabling and promoting future work that aims to uncover causal mechanisms of disease.
Conclusion
Our study suggests that IFNG and GBP5 were IBD subtype-regulators and trigger more intense innate immunity and inflammatory responses in CD than those in UC. Our findings reveal pathomechanical differences between CD and UC that may contribute to personalized treatment for CD and UC. Additionally, the protocol of causal inference analysis proposed in this study could be generalized for other transcriptome studies.
Data Availability Statement
The datasets presented in this study can be found in online repositories. The names of the repository/repositories and accession number(s) can be found in the article/Supplementary Material.
Ethics Statement
The studies involving human participants were reviewed and approved by The Sixth Affiliated Hospital of Sun Yat-sen University, Guangzhou. The patients/participants provided their written informed consent to participate in this study.
Author Contributions
LZ, PL, and RxZ conceived and designed the project. Each author has contributed significantly to the submitted work. SG drafted the manuscript. YL, DW, NJ, LY, RZ, ZX, WC, XL, SC, LZ, PL, and RxZ revised the manuscript. All authors read and approved the final manuscript.
Funding
This work was supported by the National Natural Science Foundation of China (grant number 82170542 to RxZ), (grant number 81770571 to LZ), (grant number 82000536 to NJ), National Key Research and Development Program of China (grant number 2021YFF0703700/2021YFF0703702 to RxZ), Guangdong Province “Pearl River Talent Plan” Innovation and Entrepreneurship Team Project (grant number 2019ZT08Y464 to LZ), National Postdoctoral Program for Innovative Talents of China (grant number BX20190393 to NJ), China Postdoctoral Science Foundation (grant number 2019M663252 to NJ), Fundamental Research Funds for the Central Universities (grant number 19ykzd01 to LZ) and (grant number 20kypy07 to NJ).
Conflict of Interest
Author RxZ serves as an advisory board member for Research Institute, GloriousMed Clinical Laboratory, GloriousMed Technology Co., Ltd., China.
The remaining authors declare that the research was conducted in the absence of any commercial or financial relationships that could be construed as a potential conflict of interest.
Publisher’s Note
All claims expressed in this article are solely those of the authors and do not necessarily represent those of their affiliated organizations, or those of the publisher, the editors, and the reviewers. Any product that may be evaluated in this article, or claim that may be made by its manufacturer, is not guaranteed or endorsed by the publisher.
Supplementary Material
The Supplementary Material for this article can be found online at: https://www.frontiersin.org/articles/10.3389/fphar.2022.869200/full#supplementary-material
Supplementary Figure S1 | DEGs and pathways with altered activities in IBD. (A) All DEGs in IBD; (B) up-regulated DEGs in IBD; (C) down-regulated DEGs in IBD; and (D) enriched pathways in IBD.
Supplementary Figure S2 | Network of DEPs KEGG. Blue, yellow, and gray represent causal DEGs, non-causal DEGs, and other genes, respectively, in DEPs. The node size represents the significance of causal effects.
Supplementary Figure S3 | Regression analysis of IFNG, GBP5, and NLRP3 in healthy controls, CD, and UC patients, respectively, according to transcriptome analysis. N = 10, 15, and 13 for CD, UC, and control groups, respectively. (A–C) Linear regression between IFNG and GBP5 based on log2-scaled expression values in CD (Spearman; R = 0.89, p = 0.00066), UC (Spearman; R = 0.74, p = 0.0039), and control (Spearman; R = −0.022, p = 0.94). (D–F) Linear regression between GBP5 and NLRP3 based on log2-scaled expression values in CD (Spearman; R = 0.69, p = 0.026), UC (Spearman; R = −0.019, p = 0.95), and control (Spearman; R = 0.46, p = 0.12). (G–J) Linear regression between IFNG and NLRP3 based on log2-scaled expression values in CD (Spearman; R = 0.76, p = 0.011), UC (Spearman; R = −0.19, p = 0.51), and control (Spearman; R = 0.45, p = 0.12).
Supplementary Figure S4 | Gene expression of IFNG, GBP5, and NLRP3 in healthy controls, CD, and UC patients in an independent cohort. The expression of (A) IFNG, (B) GBP5, and (C) NLRP3 genes in the control, CD, and UC groups. Yellow, CD; orange, UC; gray, control. All data are presented as mean ± standard error. *: p < 0.05;**: p < 0.01;***: p < 0.001. N = 10, 15, and 13 for CD, UC, and control groups, respectively. (D) Linear regression between IFNG and GBP5 based on log2-scaled expression values (Spearman; R = 0.9, p < 2.2e-16). (E) Linear regression between GBP5 and NLRP3 based on log2-scaled expression values (Spearman; R = 0.85, p = 4.5e-16). (F) Linear regression between IFNG and NLRP3 based on log2-scaled expression values (Spearman; R = 0.81, p = 4.9e-13).
Supplementary Figure S5 | Gene expression of IL1B and IL18 in healthy controls, CD, and UC patients according to transcriptome analysis. The expression of (A) IL1B and (B) IL18 genes in the control, CD, and UC groups. Yellow, CD; orange, UC; gray, control. All data are presented as mean ± standard error. *: p < 0.05; **: p < 0.01; ***: p < 0.001. N = 10, 15, and 13 for CD, UC, and control groups, respectively. (C) Linear regression between NLRP3 and IL1B based on log2-scaled expression values (Spearman; R = 0.7, p = 7.8e-07). (D) Linear regression between NLRP3 and IL18 based on log2-scaled expression values (Spearman; R = 0.4, p = 0.014).
Supplementary Figure S6 | Regression analysis of IFNG, GBP5, and NLRP3 in healthy controls, CD, and UC patients, respectively, according to qRT-PCR data. N = 36, 13, and 33 for CD, UC, and control groups, respectively. (A–C) Linear regression between IFNG and GBP5 based on log2-scaled expression values in CD (Spearman; R = 0.9, p = 1.1e-13), UC (Spearman; R = 0.88, p = 6.6e-05), and control (Spearman; R = 0.63, p = 8.6e-05). (D–F) Linear regression between GBP5 and NLRP3 based on log2-scaled expression values in CD (Spearman; R = 0.78, p = 2e-08), UC (Spearman; R = 0.74, p = 0.0039), and control (Spearman; R = 0.69, p = 7.6e-06). (G–J) Linear regression between IFNG and NLRP3 based on log2-scaled expression values in CD (Spearman; R = 0.61, p =7.2e-05), UC (Spearman; R = 0.67, p = 0.013), and control (Spearman; R = 0.48, p = 0.0048).
Abbreviations
ACTB, actin beta; CASP1, caspase 1; CCL3, C–C motif chemokine ligand 3; CD, Crohn’s disease; CEV, causal estimate values; CRG, correcting reference genes; CXCL10, C–X–C motif chemokine ligand 10; DEFB1, defensin beta 1; DEGs, differentially expressed genes; DEPs, differentially expressed pathways; GAPDH, glyceraldehyde 3-phosphate dehydrogenase; GBP5, guanylate-binding protein 5; IBD, inflammatory bowel disease; IFNG, interferon gamma; IL-17, interleukin 17; IL1B, interleukin 1 beta; IL18, interleukin 18; IL6, interleukin 6; LCN2, lipocalin-2; LTB, lymphotoxin beta; MMP9, matrix metallopeptidase 9; NLRP3, NLR family pyrin domain-containing 3; PTGS2, prostaglandin-endoperoxide synthase 2; PYCARD, PYD and CARD domain containing; qRT-PCR, quantitative real-time polymerase chain reaction; S100A12, S100 calcium binding protein A12; SOCS3, suppressor of cytokine signaling 3; UC, ulcerative colitis.
References
Atreya, R., and Neurath, M. F. (2005). Involvement of IL-6 in the Pathogenesis of Inflammatory Bowel Disease and colon Cancer. Clin. Rev. Allergy Immunol. 28 (3), 187–196. doi:10.1385/CRIAI:28:3:187
Banks, C., Bateman, A., Payne, R., Johnson, P., and Sheron, N. (2003). Chemokine Expression in IBD. Mucosal Chemokine Expression Is Unselectively Increased in Both Ulcerative Colitis and Crohn's Disease. J. Pathol. 199 (1), 28–35. doi:10.1002/path.1245
Bergantz, L., Subra, F., Deprez, E., Delelis, O., and Richetta, C. (2019). Interplay between Intrinsic and Innate Immunity during HIV Infection. Cells 8 (8). doi:10.3390/cells8080922
Bernstein, C. N., Eliakim, A., Fedail, S., Fried, M., Gearry, R., Goh, K. L., et al. (2016). World Gastroenterology Organisation Global Guidelines Inflammatory Bowel Disease: Update August 2015. J. Clin. Gastroenterol. 50 (10), 803–818. doi:10.1097/MCG.0000000000000660
Britzen-Laurent, N., Herrmann, C., Naschberger, E., Croner, R. S., and Stürzl, M. (2016). Pathophysiological Role of Guanylate-Binding Proteins in Gastrointestinal Diseases. World J. Gastroenterol. 22 (28), 6434–6443. doi:10.3748/wjg.v22.i28.6434
Cayatte, C., Joyce-Shaikh, B., Vega, F., Boniface, K., Grein, J., Murphy, E., et al. (2012). Biomarkers of Therapeutic Response in the IL-23 Pathway in Inflammatory Bowel Disease. Clin. Transl Gastroenterol. 3 (2), e10. doi:10.1038/ctg.2012.2
Charmoy, M., Brunner-Agten, S., Aebischer, D., Auderset, F., Launois, P., Milon, G., et al. (2010). Neutrophil-derived CCL3 Is Essential for the Rapid Recruitment of Dendritic Cells to the Site of Leishmania Major Inoculation in Resistant Mice. Plos Pathog. 6 (2), e1000755. doi:10.1371/journal.ppat.1000755
Chu, H., Khosravi, A., Kusumawardhani, I. P., Kwon, A. H., Vasconcelos, A. C., Cunha, L. D., et al. (2016). Gene-microbiota Interactions Contribute to the Pathogenesis of Inflammatory Bowel Disease. Science 352 (6289), 1116–1120. doi:10.1126/science.aad9948
Dou, X., Xiao, J., Jin, Z., and Zheng, P. (2015). Peroxisome Proliferator-Activated Receptor-γ Is Downregulated in Ulcerative Colitis and Is Involved in Experimental Colitis-Associated Neoplasia. Oncol. Lett. 10 (3), 1259–1266. doi:10.3892/ol.2015.3397
Dubuquoy, L., Rousseaux, C., Thuru, X., Peyrin-Biroulet, L., Romano, O., Chavatte, P., et al. (2006). PPARgamma as a New Therapeutic Target in Inflammatory Bowel Diseases. Gut 55 (9), 1341–1349. doi:10.1136/gut.2006.093484
Eisenberg, E., and Levanon, E. Y. (2013). Human Housekeeping Genes, Revisited. Trends Genet. 29 (10), 569–574. doi:10.1016/j.tig.2013.05.010
Farkas, K., Saródi, Z., Bálint, A., Földesi, I., Tiszlavicz, L., Szűcs, M., et al. (2015). The Diagnostic Value of a New Fecal Marker, Matrix Metalloprotease-9, in Different Types of Inflammatory Bowel Diseases. J. Crohns Colitis 9 (3), 231–237. doi:10.1093/ecco-jcc/jjv005
Franchi, L., Warner, N., Viani, K., and Nuñez, G. (2009). Function of Nod-like Receptors in Microbial Recognition and Host Defense. Immunol. Rev. 227 (1), 106–128. doi:10.1111/j.1600-065X.2008.00734.x
Fujiwara, Y., Hizukuri, Y., Yamashiro, K., Makita, N., Ohnishi, K., Takeya, M., et al. (2016). Guanylate-binding Protein 5 Is a Marker of Interferon-γ-Induced Classically Activated Macrophages. Clin. Transl Immunol. 5 (11), e111. doi:10.1038/cti.2016.59
Gao, S., Zhang, Q., Tian, C., Li, C., Lin, Y., Gao, W., et al. (2020). The Roles of Qishen Granules Recipes, Qingre Jiedu, Wenyang Yiqi and Huo Xue, in the Treatment of Heart Failure. J. Ethnopharmacol 249, 112372. doi:10.1016/j.jep.2019.112372
GBD 2017 Inflammatory Bowel Disease Collaborators, (2020). The Global, Regional, and National burden of Inflammatory Bowel Disease in 195 Countries and Territories, 1990-2017: a Systematic Analysis for the Global Burden of Disease Study 2017. Lancet Gastroenterol. Hepatol. 5 (1), 17–30. doi:10.1016/S2468-1253(19)30333-4
Goren, E., Wang, C., He, Z., Sheflin, A. M., Chiniquy, D., Prenni, J. E., et al. (2021). Feature Selection and Causal Analysis for Microbiome Studies in the Presence of Confounding Using Standardization. BMC Bioinformatics 22 (1), 362. doi:10.1186/s12859-021-04232-2
Hellmann, J., Tang, Y., Zhang, M. J., Hai, T., Bhatnagar, A., Srivastava, S., et al. (2015). Atf3 Negatively Regulates Ptgs2/Cox2 Expression during Acute Inflammation. Prostaglandins Other Lipid Mediat 116-117, 49–56. doi:10.1016/j.prostaglandins.2015.01.001
Ito, R., Shin-Ya, M., Kishida, T., Urano, A., Takada, R., Sakagami, J., et al. (2006). Interferon-gamma Is Causatively Involved in Experimental Inflammatory Bowel Disease in Mice. Clin. Exp. Immunol. 146 (2), 330–338. doi:10.1111/j.1365-2249.2006.03214.x
Kaplan, G. G., and Ng, S. C. (2017). Understanding and Preventing the Global Increase of Inflammatory Bowel Disease. Gastroenterology 152 (2), 313–e2. doi:10.1053/j.gastro.2016.10.020
Kaur, A., and Goggolidou, P. (2020). Ulcerative Colitis: Understanding its Cellular Pathology Could Provide Insights into Novel Therapies. J. Inflamm. (Lond) 17, 15. doi:10.1186/s12950-020-00246-4
Knights, D., Lassen, K. G., and Xavier, R. J. (2013). Advances in Inflammatory Bowel Disease Pathogenesis: Linking Host Genetics and the Microbiome. Gut 62 (10), 1505–1510. doi:10.1136/gutjnl-2012-303954
Kopitar-Jerala, N. (2017). The Role of Interferons in Inflammation and Inflammasome Activation. Front. Immunol. 8, 873. doi:10.3389/fimmu.2017.00873
Kumar, M., Garand, M., and Al Khodor, S. (2019). Integrating Omics for a Better Understanding of Inflammatory Bowel Disease: a Step towards Personalized Medicine. J. Transl Med. 17 (1), 419. doi:10.1186/s12967-019-02174-1
Lazaridis, L. D., Pistiki, A., Giamarellos-Bourboulis, E. J., Georgitsi, M., Damoraki, G., Polymeros, D., et al. (2017). Activation of NLRP3 Inflammasome in Inflammatory Bowel Disease: Differences between Crohn's Disease and Ulcerative Colitis. Dig. Dis. Sci. 62 (9), 2348–2356. doi:10.1007/s10620-017-4609-8
Leal, R. F., Planell, N., Kajekar, R., Lozano, J. J., Ordás, I., Dotti, I., et al. (2015). Identification of Inflammatory Mediators in Patients with Crohn's Disease Unresponsive to Anti-tnfα Therapy. Gut 64 (2), 233–242. doi:10.1136/gutjnl-2013-306518
Li, Y., de Haar, C., Peppelenbosch, M. P., and van der Woude, C. J. (2012). SOCS3 in Immune Regulation of Inflammatory Bowel Disease and Inflammatory Bowel Disease-Related Cancer. Cytokine Growth Factor. Rev. 23 (3), 127–138. doi:10.1016/j.cytogfr.2012.04.005
Lv, B. M., Quan, Y., and Zhang, H. Y. (2021). Causal Inference in Microbiome Medicine: Principles and Applications. Trends Microbiol. 29 (8), 736–746. doi:10.1016/j.tim.2021.03.015
Nahidi, L., Leach, S. T., Sidler, M. A., Levin, A., Lemberg, D. A., and Day, A. S. (2011). Osteoprotegerin in Pediatric Crohn's Disease and the Effects of Exclusive Enteral Nutrition. Inflamm. Bowel Dis. 17 (2), 516–523. doi:10.1002/ibd.21361
Neurath, M. F., Weigmann, B., Finotto, S., Glickman, J., Nieuwenhuis, E., Iijima, H., et al. (2002). The Transcription Factor T-Bet Regulates Mucosal T Cell Activation in Experimental Colitis and Crohn's Disease. J. Exp. Med. 195 (9), 1129–1143. doi:10.1084/jem.20011956
Petrey, A. C., and de la Motte, C. A. (2017). The Extracellular Matrix in IBD: a Dynamic Mediator of Inflammation. Curr. Opin. Gastroenterol. 33 (4), 234–238. doi:10.1097/MOG.0000000000000368
Peyrin-Biroulet, L., Beisner, J., Wang, G., Nuding, S., Oommen, S. T., Kelly, D., et al. (2010). Peroxisome Proliferator-Activated Receptor Gamma Activation Is Required for Maintenance of Innate Antimicrobial Immunity in the colon. Proc. Natl. Acad. Sci. U S A. 107 (19), 8772–8777. doi:10.1073/pnas.0905745107
Planell, N., Lozano, J. J., Mora-Buch, R., Masamunt, M. C., Jimeno, M., Ordás, I., et al. (2013). Transcriptional Analysis of the Intestinal Mucosa of Patients with Ulcerative Colitis in Remission Reveals Lasting Epithelial Cell Alterations. Gut 62 (7), 967–976. doi:10.1136/gutjnl-2012-303333
Ramos, G. P., and Papadakis, K. A. (2019). Mechanisms of Disease: Inflammatory Bowel Diseases. Mayo Clin. Proc. 94 (1), 155–165. doi:10.1016/j.mayocp.2018.09.013
Ren, W., Gao, S., Zhang, H., Ren, Y., Yu, X., Lin, W., et al. (2018). Decomposing the Mechanism of Qishen Granules in the Treatment of Heart Failure by a Quantitative Pathway Analysis Method. Molecules 23 (7). doi:10.3390/molecules23071829
Sankarasubramanian, J., Ahmad, R., Avuthu, N., Singh, A. B., and Guda, C. (2020). Gut Microbiota and Metabolic Specificity in Ulcerative Colitis and Crohn's Disease. Front. Med. (Lausanne) 7, 606298. doi:10.3389/fmed.2020.606298
Strober, W., and Watanabe, T. (2011). NOD2, an Intracellular Innate Immune Sensor Involved in Host Defense and Crohn's Disease. Mucosal Immunol. 4 (5), 484–495. doi:10.1038/mi.2011.29
Tourkochristou, E., Aggeletopoulou, I., Konstantakis, C., and Triantos, C. (2019). Role of NLRP3 Inflammasome in Inflammatory Bowel Diseases. World J. Gastroenterol. 25 (33), 4796–4804. doi:10.3748/wjg.v25.i33.4796
Uniken Venema, W. T., Voskuil, M. D., Dijkstra, G., Weersma, R. K., and Festen, E. A. (2017). The Genetic Background of Inflammatory Bowel Disease: from Correlation to Causality. J. Pathol. 241 (2), 146–158. doi:10.1002/path.4817
Vandesompele, J., De Preter, K., Pattyn, F., Poppe, B., Van Roy, N., De Paepe, A., et al. (2002). Accurate Normalization of Real-Time Quantitative RT-PCR Data by Geometric Averaging of Multiple Internal Control Genes. Genome Biol. 3 (7), Research0034. doi:10.1186/gb-2002-3-7-research0034
Vansteelandt, S., and Vanderweele, T. J. (2012). Natural Direct and Indirect Effects on the Exposed: Effect Decomposition under Weaker Assumptions. Biometrics 68 (4), 1019–1027. doi:10.1111/j.1541-0420.2012.01777.x
Wang, Z., Zhang, S., Xiao, Y., Zhang, W., Wu, S., Qin, T., et al. (2020). NLRP3 Inflammasome and Inflammatory Diseases. Oxid Med. Cel Longev 2020, 4063562. doi:10.1155/2020/4063562
Wu, D., Jiao, N., Zhu, R., Zhang, Y., Gao, W., Fang, S., et al. (2020). Identification of the keystone Species in Non-alcoholic Fatty Liver Disease by Causal Inference and Dynamic Intervention Modeling. bioRxiv.
Yang, L., Tang, S., Baker, S. S., Arijs, I., Liu, W., Alkhouri, R., et al. (2019). Difference in Pathomechanism between Crohn's Disease and Ulcerative Colitis Revealed by Colon Transcriptome. Inflamm. Bowel Dis. 25 (4), 722–731. doi:10.1093/ibd/izy359
Yao, X., Zhang, C., Xing, Y., Xue, G., Zhang, Q., Pan, F., et al. (2017). Remodelling of the Gut Microbiota by Hyperactive NLRP3 Induces Regulatory T Cells to Maintain Homeostasis. Nat. Commun. 8 (1), 1896. doi:10.1038/s41467-017-01917-2
Yu, G., Wang, L. G., Han, Y., and He, Q. Y. (2012). clusterProfiler: an R Package for Comparing Biological Themes Among Gene Clusters. OMICS 16 (5), 284–287. doi:10.1089/omi.2011.0118
Zhang, J. D., and Wiemann, S. (2009). KEGGgraph: a Graph Approach to KEGG PATHWAY in R and Bioconductor. Bioinformatics 25 (11), 1470–1471. doi:10.1093/bioinformatics/btp167
Zhang, Y., Huang, R., Cheng, M., Wang, L., Chao, J., Li, J., et al. (2019). Gut Microbiota from NLRP3-Deficient Mice Ameliorates Depressive-like Behaviors by Regulating Astrocyte Dysfunction via circHIPK2. Microbiome 7 (1), 116. doi:10.1186/s40168-019-0733-3
Keywords: inflammatory bowel disease, Crohn’s disease, ulcerative colitis, causal inference, IBD subtype-regulator
Citation: Gao S, Li Y, Wu D, Jiao N, Yang L, Zhao R, Xu Z, Chen W, Lin X, Cheng S, Zhu L, Lan P and Zhu R (2022) IBD Subtype-Regulators IFNG and GBP5 Identified by Causal Inference Drive More Intense Innate Immunity and Inflammatory Responses in CD Than Those in UC. Front. Pharmacol. 13:869200. doi: 10.3389/fphar.2022.869200
Received: 04 February 2022; Accepted: 10 March 2022;
Published: 06 April 2022.
Edited by:
Guoxun Chen, The University of Tennessee, United StatesCopyright © 2022 Gao, Li, Wu, Jiao, Yang, Zhao, Xu, Chen, Lin, Cheng, Zhu, Lan and Zhu. This is an open-access article distributed under the terms of the Creative Commons Attribution License (CC BY). The use, distribution or reproduction in other forums is permitted, provided the original author(s) and the copyright owner(s) are credited and that the original publication in this journal is cited, in accordance with accepted academic practice. No use, distribution or reproduction is permitted which does not comply with these terms.
*Correspondence: Lixin Zhu, emh1bHg2QG1haWwuc3lzdS5lZHUuY24=; Ping Lan, bGFucGluZ0BtYWlsLnN5c3UuZWR1LmNu; Ruixin Zhu, cnh6aHVAdG9uZ2ppLmVkdS5jbg==