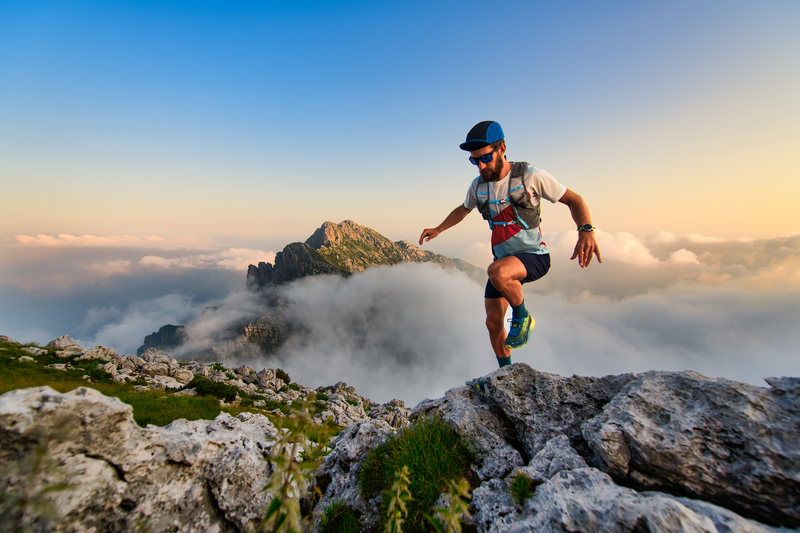
95% of researchers rate our articles as excellent or good
Learn more about the work of our research integrity team to safeguard the quality of each article we publish.
Find out more
SYSTEMATIC REVIEW article
Front. Pharmacol. , 16 March 2022
Sec. Drugs Outcomes Research and Policies
Volume 13 - 2022 | https://doi.org/10.3389/fphar.2022.862640
This article is part of the Research Topic From Clinical Trials to Real-World Data Sciences: Evidence-Based Medicine for Value in Health View all 36 articles
Objective: Due to the initiation of the priority review program in China, many antitumor drugs have been approved for marketing based on phase II clinical trials and short-term surrogate endpoint indicators. This study used approved targeted drugs for the treatment of non-small-cell lung cancer (NSCLC) in China as an example to evaluate the association between short-term surrogate endpoints [objective response rate (ORR) and disease control rate (DCR)] and median progression-free survival (mPFS) and median overall survival (mOS).
Methods: Five databases, i.e., MEDLINE, Embase, Cochrane Library, China National Knowledge Infrastructure (CNKI), and Wanfang Data were searched, for phase II or phase III clinical trials of all molecular targeted drugs that have been marketed in China for the treatment of NSCLC. After screening the literature and extracting information, both univariate and multivariate linear regression were performed on the short-term surrogate indicators and mPFS and mOS to explore the relationship.
Results: A total of 63 studies were included (25 studies with only ORR, DCR, and mPFS and 39 studies with ORR, DCR, mPFS, and mOS). In terms of the targeted drugs for the treatment of NSCLC, in addition to the good but not excellent linear relationship between DCR and mOS (0.4 < R2adj = 0.5653 < 0.6), all other short-term surrogate endpoint indicators had excellent linear relationships with mPFS and mOS (R2adj≥0.6), while mPFS and mOS had the most excellent linear relationships (R2adj = 0.8036).
Conclusion: For targeted drugs for the treatment of NSCLC, short-term surrogate endpoint indicators such as ORR and DCR may be reliable surrogate indicators for mPFS and mOS. However, whether short-term surrogate endpoint indicators can be used to predict final endpoints remains to be verified.
Malignant tumors are a high-risk factor for death and severely hinder increases in the average life expectancy of the population (1). They are the leading cause of death in the urban population. In 2019, approximately 25.73% of urban population deaths in China were caused by malignant tumors, with a mortality rate of approximately 161.56/100,000 people (2). In 2018, there were 3.804 million new cases of malignant tumors in China, accounting for more than 20% of the global cases. The incidence of malignant tumors was 278.07 per 100,000 people, and the mortality rate was 167.89 per 100,000 people (Ma and Yu, 2020). Malignant tumors seriously threaten the lives and health of people. From the perspective of disease burden, malignant tumors have caused a substantial loss of disability-adjusted life years (DALYs). Studies have shown that (3) the proportion of DALYs caused by trachea, bronchus, and lung cancers was 4.1% of the total DALYs, ranking fourth only after stroke (11.9%), ischemic heart disease (8.1%), and chronic obstructive pulmonary disease (5.5%). From the economic burden perspective, the average medical costs for malignant tumor patients are increasing year by year. In 2005, the average cost of a single hospitalization for discharged patients in China was 10,777 yuan (RMB), increasing to 13,322 yuan in 2011, 15,672 yuan in 2013, and 17,567 yuan in 2016 (Wei-jing and xiao-lu, 2019).
To increase patients’ accessibility to new drugs and to improve the quality of life, the National Medical Products Administration (NMPA) in China launched a priority review program to allow more innovative drugs to be approved as soon as possible to bring patients with malignant tumors benefits. The NMPA priority review processes mainly include three policies: one review process for breakthrough therapeutic drugs, one review process for the conditional approval of drugs for marketing, and one priority review process for drug marketing authorization (Adminstration, 2020). The priority review program greatly shortens the time to market for some new anticancer drugs which often focus on the rare targets, and many of them do not have abundant clinical data based on Chinese patients. Many of these clinical studies are often single-arm with a small sample size and short follow-ups, and even primary endpoint indicators such as progression-free survival (PFS) and overall survival (OS) were not reported.
Table 1 summarized the reported status of clinical trial indicators for anticancer drugs approved in China from 2017 to November 2021. An increasing number of drugs were approved using only short-term surrogate endpoint indicators. Among them, only 16 new drugs reported both PFS and OS data. However, the lack of primary endpoint indicators causes challenges in reliably determining the safety and efficacy of anticancer drugs and, likewise, poses a significant challenge for economic evaluations. In the economic evaluation of anticancer drugs, the partitioned survival model (PSM) and the Markov model are most popular model types (Rui et al., 2021). The construction of both the PSM and the Markov model requires the support of mature PFS and OS data (6). Therefore, when only short-term surrogate endpoint indicators available, it is worth investigating whether there is a significant relationship between such indicators and primary endpoint indicators.
TABLE 1. Summary of clinical endpoints of new anti-cancer drugs approved from January 2017 to November 2021.
To explore this issue, this study will use clinical trials related to targeted therapy for the treatment of NSCLC approved in China as an example to explore the correlation between short-term surrogate endpoint indicators and primary endpoint indicators.
Chinese and English databases and platforms were searched for Phase II or phase III clinical trials of molecular targeted drugs for the treatment of NSCLC. The Chinese search included China National Knowledge Infrastructure (CNKI) and Wanfang Data. The English search included MEDLINE through the PubMed search platform, Embase, and the Cochrane Library. The date ranges for the searches were from the establishment of the databases to 20 March 2021. The searches were based on a combination of subject headings and free-text. Chinese search terms and English search terms included non-small-cell lung cancer and clinical trial, among other terms. The English search strategy was shown in the supplementary materials.
The inclusion criteria for this study were as follows: (Bray et al., 2021) phase II or phase III clinical trials, including single-arm clinical trials and placebo-controlled clinical trials (Ma and Yu, 2020); patients diagnosed with NSCLC by laboratory tests, imaging examinations and clinical signs and symptoms (Wei-jing and xiao-lu, 2019); intervention measures that included molecular targeted drugs for the treatment of NSCLC approved for marketing in China as of March 2021, including gefitinib, erlotinib, icotinib, crizotinib, dacomitinib, afatinib, osimertinib, almonertinib, alectinib, ceritinib, brigatinib, lorlatinib, selpercatinib, entrectinib, dabrafenib + trametinib, erlotinib + linsitinib, erlotinib + pazotinib, erlotinib + sorafenib, and anlotinib (Adminstration, 20202020); short-term surrogate endpoint indicators included ORR or DCR; and (Rui et al., 2021) primary endpoint indicators included median progression-free survival (mPFS) and median overall survival (mOS). The exclusion criteria for this study were as follows: (Bray et al., 2021): duplicate literature (Ma and Yu, 2020); non-Chinese or non-English literature (Wei-jing and xiao-lu, 2019); conference abstract (Adminstration, 20202020); trials other than phase II or phase III clinical trials (Rui et al., 2021); no simultaneous reporting of DCR, ORR, and mPFS; and (Coyle and Coyle, 2014) intervention measures that included molecular targeted drugs combined with other types of therapeutic measures.
Two researchers independently screened the literature, extracted the data, and cross-checked the data. Disagreements were resolved through consultation with a third party. Data extraction mainly included ① basic characteristics of the included studies (title, authors, year, etc.); ② sample size of each group; ③ treatment measures and their usage and dosage; ④ key elements of bias risk assessments; and ⑤ endpoint indicators (ORR, DCR, mPFS and mOS).
Two investigators independently conducted quality evaluations of the included studies and cross-checked the results. For randomized controlled clinical trials (RCTs), the quality of the included studies was evaluated using the risk of bias assessment tool for RCTs recommended by the Cochrane Manual (Higgins et al., 2011). The Newcastle–Ottawa scale (NOS), recommended by the Cochrane Non-Randomized Studies Methods Group (NRSMG), was used to evaluate the quality of single-arm clinical trials (Margulis et al., 2014).
This study used STATA 15.1 to perform both univariate and multivariate linear regression analysis of the relationship between DCR and ORR and mPFS, as well as the relationship between DCR and ORR and mOS. In the case of a poor linear relationship between the shot-term surrogate endpoint indicators and the primary endpoint indicators, ln transformation was performed on the short-term surrogate endpoint indicators to explore the linear relationship between ln (short-term surrogate endpoint indicators) and the primary endpoint indicators. For the different dosage, medication or duration included in the analysis, the treatments were categorized for inclusion in multivariate regression analysis. In addition, some studies showed that the OS is largely affected by the number of previous treatment lines, which means that patients received more lines of treatments often have a worse prognosis (Gisselbrecht et al., 2010; Rule et al., 2017). Therefore, the subgroup analyses were performed for first-line treatment and second-line or post-second-line treatment based on the number of treatment lines in the univariate linear regression analysis to separate patients with different treatment lines to reduce heterogeneity. The scatter plots for DCR and ORR vs. mPFS and mOS were plotted using Microsoft Excel. Adjusted goodness-of-fit R2adj was used to evaluate the degree of fit of the model. According to Lassere et al. (Lassere et al., 2012), R2adj≥0.6 indicates excellent goodness-of-fit, R2adj≥0.4 indicates good goodness-of-fit, R2adj≥0.2 indicates fair goodness-of-fit, and R2adj<0.2 indicates poor goodness-of-fit.
A total of 5,058 articles were obtained in the preliminary searches, and a total of 4,547 articles were included in the preliminary screening after excluding duplicates. After reading the titles and abstracts, 4,019 papers were excluded, and 528 papers were included in the full-text rescreening. After reading the full text of the 528 papers, 63 articles were included in the final sample for the quantitative analysis of DCR, ORR, mPFS and mOS. The literature screening process is shown in Figure 1.
Among the 63 included studies, 25 (Inoue et al., 2006; Wen et al., 2007; Kim et al., 2012; Yamada et al., 2013; Hu et al., 2015; Yoshimura et al., 2015; Park et al., 2016; Yang et al., 2017a; Leighl et al., 2017; Peters et al., 2017; Han et al., 2018a; Akamatsu et al., 2018; Wu et al., 2018a; Nie et al., 2018; Yang et al., 2018; Zhou et al., 2018; Deng et al., 2019; Landi et al., 2019; Michels et al., 2019; Ohe et al., 2019; Saito et al., 2019; Yang et al., 2019; Yokoyama et al., 2019; Yang et al., 2020; de Marinis et al., 2021) only reported ORR, DCR, and mPFS, and 39 (Guan et al., 2005; Zhou et al., 2009; Gaafar et al., 2011; Lee et al., 2011; Spigel et al., 2011; Ciuleanu et al., 2012; Deng et al., 2012; Miller et al., 2012; Pallis et al., 2012; Pérol et al., 2012; Ramalingam et al., 2012; Shi et al., 2013; Wu et al., 2013; Ramalingam et al., 2014; Choi et al., 2015; Juan et al., 2015; Wu et al., 2015; An et al., 2016; Ding-guo and Xiu-li, 2016; Neal et al., 2016; Yang et al., 2017b; Han et al., 2017; Ikezawa et al., 2017; Miyawaki et al., 2017; Han et al., 2018b; Wu et al., 2018b; Garon et al., 2018; Kiura et al., 2018; Spigel et al., 2018; Uchibori et al., 2018; Arrieta et al., 2019; Landi et al., 2019; Xu et al., 2019; Ahn et al., 2020; Eide et al., 2020; Gadgeel et al., 2020; Goldman et al., 2020; Huber et al., 2020; Scagliotti et al., 2020) reported ORR, DCR, mPFS, and mOS concurrently. One paper (19) grouped the patients for whom ORR, DCR, and mPFS were reported and the patients for whom ORR, DCR, mPFS and mOS were reported concurrently. Basic information and the ORR, DCR, mPFS and mOS values reported in the included studies are provided in Tables 2, 3.
The publication dates for all the included articles were concentrated from 2005 to 2021, and the target population was patients with advanced NSCLC. Among the studies, 21 (33.3%) were single-arm clinical trials, 42 (66.7%) were double-arm or multi-arm clinical trials, 34 (54.0%) enrolled patients in the first-line treatment stage, and 29 (46.0%) enrolled patients in the late-line or multi-line treatment stage. The sample sizes ranged from 11 to 479. The targeted therapies included 15 targeted drugs (avapritinib, afatinib, icotinib, alectinib. Anlotinib, osimertinib, brigatinib, dacomitinib, erlotinib, ensatinib, gefitinib, crizotinib, linsitinib, ceritinib, and sorafenib). DCRs ranged from 14.9% to 100.0%; ORRs values ranged from 5.3% to 87.0%; mPFS ranged from 1.5 to 20.0 months; and mOS ranged from 3.2 to 34.0 months.
Risk of bias in RCTs: The results of the risk of bias analyses for 42 two-arm or multi-arm RCTs were provided in Figure 2. “Selective reporting,” “incomplete outcome data” and “random sequence generation” had a low risk of bias, and “blinding of outcome assessment” and “blinding of participants and personnel” had a high risk of bias. The risk of bias results for “allocation concealment” and “other bias” were not clear.
Quality evaluation results for single-arm trials: The NOS scores for the 21 included single-arm clinical trials were shown in Figure 3. The NOS scores for all studies ranged from 4 to 6, with an average score of 5.6, indicating that the overall quality of the studies was high. Among them, the NOS score for one paper was four points, the NOS score for six papers was five points, and the NOS score for the remaining 14 papers was six points.
Taking the natural logarithm of mPFS, the adjusted goodness-of-fit of the univariate regression between ORR and ln (mPFS) was excellent (R2adj = 0.7356 > 0.6), which was shown in Figure 4 and Supplementary Table S4. After controlling the treatment factors, the adjusted goodness-of-fit of the multivariate regression between ORR and ln (mPFS) was excellent (R2adj = 0.7772 > 0.6), which was shown in the Supplementary Table S5.
Taking the natural logarithm of mPFS, the adjusted goodness-of-fit of the univariate regression between DCR and ln (mPFS) was excellent (R2adj = 0.7642 > 0.6), which was shown in Figure 5 and Supplementary Table S6. After controlling the treatment factors, the adjusted goodness-of-fit of the multivariate regression between DCR and ln (mPFS) was excellent (R2adj = 0.7806 > 0.6), which was shown in the Supplementary Table S7.
The adjusted goodness-of-fit of the univariate regression between ORR and mOS was excellent (R2adj = 0.7633 > 0.6), which was shown in Figure 6 and Supplementary Table S8. After controlling the treatment factors, the adjusted goodness-of-fit of the multivariate regression between ORR and mOS was excellent (R2adj = 0.7813 > 0.6), which was shown in the Supplementary Table S9.
Taking the natural logarithm of mOS, the adjusted goodness-of-fit of the univariate regression between DCR and ln (mOS) was good (R2adj = 0.5653 > 0.4), which was shown in Figure 7 and Supplementary Table S10. After controlling the treatment factors, the adjusted goodness-of-fit of the multivariate regression between DCR and ln (mOS) was excellent (R2adj = 0.6331 > 0.6), which was shown in the Supplementary Table S11.
The adjusted goodness-of-fit of the univariate regression between mPFS and mOS was excellent (R2adj = 0.7616 > 0.6), which was shown in Figure 8 and Supplementary Table S12. After controlling the treatment factors, the adjusted goodness-of-fit of the multivariate regression between mPFS and mOS was excellent (R2adj = 0.8036 > 0.6), which was shown in the Supplementary Table S13.
Taking the natural logarithm of mPFS, the adjusted goodness-of-fit of the univariate regression between ORR and ln (mPFS) was excellent (R2adj = 0.6188 > 0.6), which was shown in Figure 9.
Taking the natural logarithm of mPFS, the adjusted goodness-of-fit of the univariate regression between DCR and ln (mPFS) was excellent (R2adj = 0.7128 > 0.6), which was shown in Figure 10.
The adjusted goodness-of-fit of the univariate regression between ORR and mOS was excellent (R2adj = 0.7074 > 0.6), which was shown in Figure 11.
The adjusted goodness-of-fit of the univariate regression between mPFS and mOS was excellent (R2adj = 0.7764 > 0.6), which was shown in Figure 12.
Taking the natural logarithm of mPFS, the adjusted goodness-of-fit of the univariate regression between ORR and ln (mPFS) was excellent (R2adj = 0.6926 > 0.6), which was shown in Figure 13.
Taking the natural logarithm of mPFS, the adjusted goodness-of-fit of the univariate regression between mPFS and mOS was excellent (R2adj = 0.7497 > 0.6), which was shown in Figure 14.
The adjusted goodness-of-fit of the univariate regression between ORR and mOS was excellent (R2adj = 0.7324 > 0.6), which was shown in Figure 15.
This study summarized all clinical trials of molecular targeted drugs for the treatment of NSCLC approved for marketing in China as of March 2021. Studies that included DCR, ORR, mPFS concurrently and studies that included DCR, ORR, mPFS, and mOS concurrently were extracted for univariate linear regression analysis. This study included a total of 25 articles that reported DCR, ORR, and mPFS concurrently and 39 articles that reported DCR, ORR, mPFS, and mOS concurrently. In the relationship between DCR and ORR and mPFS, DCR and ORR had an excellent linear relationship with ln (mPFS), and the adjusted goodness-of-fit R2adj was >0.6. However, the linear relationships between DCR and ORR and mPFS were slightly weaker. For the relationships between DCR and ORR vs. mPFS and mOS, the linear relationship between DCR and mOS or ln (mOS) was good but not excellent (0.4 < R2adj<0.6). mPFS and mOS had most excellent linear relationships (R2adj = 0.8036).
Cooper et al. (2020) (75) conducted a systematic review of the literature that explored the relationship between short-term surrogate endpoint indicators and primary endpoint indicators in malignancy clinical trials. A systematic search of relevant literature in five databases (from the establishment of the database to March 2019) was conducted, resulting in the inclusion of 64 articles. The results of the study suggested that short-term surrogate endpoint indicators such as ORR and CR cannot replace primary endpoint indicators such as PFS and OS and that the correlation between the two is weak and unstable. Our result was inconsistent with those reported by Cooper et al. (Cooper et al., 2020) Although Cooper et al. reported that there was no significant correlation between short-term surrogate endpoint indicators and primary endpoint indicators, the conclusion was likely due to the wide selection of disease types and treatment regimens included in the study. Moreover, Cooper et al. did not address whether there was a correlation between short-term surrogate endpoint indicators and primary endpoint indicators in the clinical trials of specific types of anticancer drugs, which might be one of the main reasons for the difference between the results of this study and the study by Cooper et al.
The results of this study revealed that short-term surrogate endpoint indicators (ORR and DCR) might have a linear relationship with mPFS and mOS, potentially providing the option to use short-term surrogate endpoint indicators to predict mPFS and mOS. In the pharmacoeconomic evaluation of tumors, PFS and OS are the most important evaluation indicators to verify drug efficacy and determine the success of the construction of pharmacoeconomic models. In the traditional pharmacoeconomic models for advanced cancer, 3-state models are often used to construct Markov models or PSMs for pharmacoeconomic evaluations (5). Markov models indicate the transition probability between health states using PFS and OS curves, and PSMs use PFS and OS curves to divide the area under the survival curve into three regions to calculate the area under the curve. If short-term surrogate endpoint indicators are used to predict mPFS and mOS, only two median values can be obtained, posing a challenge for pharmacoeconomic evaluations. We recommend that when only mPFS and mOS are available, assuming the PFS and OS curves obey an exponential distribution, mPFS and mOS should be used to construct an exponential distribution survival curve, thereby allowing the construction of a Markov model and an economic evaluation (Latimer, 2011). Although this method has strong assumptions, it can also provide a certain reference value in the absence of data.
This study has some limitations. Firstly, the molecular targeted drugs included in this study were limited to targeted drugs for the treatment of NSCLC that were approved for marketing in China as of March 2021; many targeted drugs approved for marketing in the other countries were not included in this study. Therefore, the extrapolation of the results is limited. Secondly, although only molecular targeted drugs approved in China were included, the race distribution of the included patients were not considered in the analyses. For many of these drugs, especially the recently approved drugs, were approved based on the published clinical data of the published international population plus the unpublished clinical data of a small sample of the Chinese population. Thirdly, this study did not use a large amount of real-world data for prediction and validation for the focus was to establish the statistical relationship between short-term surrogate endpoint indicators and primary endpoint indicators. Finally, for mOS, in addition to short-term surrogate endpoint indicators, other factors, such as the choice of subsequent treatment, will have a significant impact on mOS; however, the univariate linear regression used in this study did not include enough influencing factors other than treatments.
The original contributions presented in the study are included in the article/Supplementary Material, further inquiries can be directed to the corresponding author.
Conception of the study: MR, ZW, and HL; literature search: MR, ZF and ZW; data extraction: MR, ZW, ZF, and LS; statistical analysis: MR, YW, and YCW; drafting the manuscript: MR, YCW, and YW; revising and completion of final work: LS, YS, ZF, and HL; all authors reviewed and approved the final manuscript.
The publishing fees were funded by the corresponding author.
The authors declare that the research was conducted in the absence of any commercial or financial relationships that could be construed as a potential conflict of interest.
All claims expressed in this article are solely those of the authors and do not necessarily represent those of their affiliated organizations, or those of the publisher, the editors, and the reviewers. Any product that may be evaluated in this article, or claim that may be made by its manufacturer, is not guaranteed or endorsed by the publisher.
The Supplementary Material for this article can be found online at: https://www.frontiersin.org/articles/10.3389/fphar.2022.862640/full#supplementary-material
Adminstration, N. M. P. (20202020). National Medical Products Adminstration on Issuing Three Documents Including "Breakthrough Therapy Drug Review Work Procedures (Trial). Available at: https://www.nmpa.gov.cn/xxgk/ggtg/qtggtg/20200708151701834.html.
Ahn, M. J., Han, J. Y., Kim, D. W., Cho, B. C., Kang, J. H., Kim, S. W., et al. (2020). Osimertinib in Patients with T790M-Positive Advanced Non-small Cell Lung Cancer: Korean Subgroup Analysis from Phase II Studies. Cancer Res. Treat. 52 (1), 284–291. doi:10.4143/crt.2019.200
Akamatsu, H., Katakami, N., Okamoto, I., Kato, T., Kim, Y. H., Imamura, F., et al. (2018). Osimertinib in Japanese Patients with EGFR T790M Mutation-Positive Advanced Non-small-cell Lung Cancer: AURA3 Trial. Cancer Sci. 109 (6), 1930–1938. doi:10.1111/cas.13623
An, C., Zhang, J., Chu, H., Gu, C., Xiao, F., Zhu, F., et al. (2016). Study of Gefitinib and Pemetrexed as First-Line Treatment in Patients with Advanced Non-small Cell Lung Cancer Harboring EGFR Mutation. Pathol. Oncol. Res. 22 (4), 763–768. doi:10.1007/s12253-016-0067-4
Arrieta, O., Barrón, F., Padilla, M. S., Avilés-Salas, A., Ramírez-Tirado, L. A., Arguelles Jiménez, M. J., et al. (2019). Effect of Metformin Plus Tyrosine Kinase Inhibitors Compared with Tyrosine Kinase Inhibitors Alone in Patients with Epidermal Growth Factor Receptor-Mutated Lung Adenocarcinoma: A Phase 2 Randomized Clinical Trial. JAMA Oncol. 5 (11), e192553. doi:10.1001/jamaoncol.2019.2553
Bray, F., Laversanne, M., Weiderpass, E., and Soerjomataram, I. (2021). The Ever-Increasing Importance of Cancer as a Leading Cause of Premature Death Worldwide. Cancer 127 (16), 3029–3030. doi:10.1002/cncr.33587
Cheng, Y., Wang, Q., Li, K., Shi, J., Liu, Y., Wu, L., et al. (2021). Anlotinib vs. Placebo as Third- or Further-Line Treatment for Patients With Small Cell Lung Cancer: A Randomised, Double-Blind, Placebo-Controlled Phase 2 Study. Br. J. Cancer 125, 366–371. doi:10.1038/s41416-021-01356-3
Choi, Y. J., Lee, D. H., Choi, C. M., Lee, J. S., Lee, S. J., Ahn, J. H., et al. (2015). Randomized Phase II Study of Paclitaxel/carboplatin Intercalated with Gefitinib Compared to Paclitaxel/carboplatin Alone for Chemotherapy-Naïve Non-small Cell Lung Cancer in a Clinically Selected Population Excluding Patients with Non-smoking Adenocarcinoma or Mutated EGFR. BMC Cancer 15, 763. doi:10.1186/s12885-015-1714-y
Ciuleanu, T., Stelmakh, L., Cicenas, S., Miliauskas, S., Grigorescu, A. C., Hillenbach, C., et al. (2012). Efficacy and Safety of Erlotinib versus Chemotherapy in Second-Line Treatment of Patients with Advanced, Non-small-cell Lung Cancer with Poor Prognosis (TITAN): a Randomised Multicentre, Open-Label, Phase 3 Study. Lancet Oncol. 13 (3), 300–308. doi:10.1016/S1470-2045(11)70385-0
Cooper, K., Tappenden, P., Cantrell, A., and Ennis, K. (2020). A Systematic Review of Meta-Analyses Assessing the Validity of Tumour Response Endpoints as Surrogates for Progression-free or Overall Survival in Cancer. Br. J. Cancer 123 (11), 1686–1696. doi:10.1038/s41416-020-01050-w
Coyle, D., and Coyle, K. (2014). The Inherent Bias from Using Partitioned Survival Models in Economic Evaluation. Value in Health 17 (3), A194. doi:10.1016/j.jval.2014.03.1130
de Marinis, F., Laktionov, K. K., Poltoratskiy, A., Egorova, I., Hochmair, M., Passaro, A., et al. (2021). Afatinib in EGFR TKI-Naïve Patients with Locally Advanced or Metastatic EGFR Mutation-Positive Non-small Cell Lung Cancer: Interim Analysis of a Phase 3b Study. Lung Cancer 152, 127–134. doi:10.1016/j.lungcan.2020.12.011
Deng, H., Li, B., Li, L., Peng, J., Lv, T., Liu, Y., et al. (2019). Clinical Observation of Crizotinib in the Treatment of ALK-Positive Advanced Non-small Cell Lung Cancer. Pathol. Res. Pract. 215 (12), 152695. doi:10.1016/j.prp.2019.152695
Deng, J., Fang, W. J., Zhang, X. C., Wu, D. P., Fang, H. M., Chen, J., et al. (2012). Phase II Trial of Gefitinib in Pretreated Chinese Women with Advanced Non-small-cell Lung Cancer. Med. Oncol. 29 (2), 595–599. doi:10.1007/s12032-011-9891-2
Ding-guo, Z., and Xiu-li, R. (2016). A Randomized Clinical Study of Gefitinib and Pemetrexed as Second Line Therapy for Advanced Non-small Cell Lung Cancer. Oncol. Prog. 14 (01), 78–80+6.
Eide, I. J. Z., Helland, Å., Ekman, S., Mellemgaard, A., Hansen, K. H., Cicenas, S., et al. (2020). Osimertinib in T790M-Positive and -negative Patients with EGFR-Mutated Advanced Non-small Cell Lung Cancer (The TREM-Study). Lung Cancer 143, 27–35. doi:10.1016/j.lungcan.2020.03.009
Gaafar, R. M., Surmont, V. F., Scagliotti, G. V., Van Klaveren, R. J., Papamichael, D., Welch, J. J., et al. (2011). A Double-Blind, Randomised, Placebo-Controlled Phase III Intergroup Study of Gefitinib in Patients with Advanced NSCLC, Non-progressing after First Line Platinum-Based Chemotherapy (EORTC 08021/ILCP 01/03). Eur. J. Cancer 47 (15), 2331–2340. doi:10.1016/j.ejca.2011.06.045
Gadgeel, S., Rodríguez-Abreu, D., Speranza, G., Esteban, E., Felip, E., Dómine, M., et al. (2020). Updated Analysis from KEYNOTE-189: Pembrolizumab or Placebo Plus Pemetrexed and Platinum for Previously Untreated Metastatic Nonsquamous Non-small-cell Lung Cancer. J. Clin. Oncol. 38 (14), 1505–1517. doi:10.1200/jco.19.03136
Garon, E. B., Siegfried, J. M., Stabile, L. P., Young, P. A., Marquez-Garban, D. C., Park, D. J., et al. (2018). Randomized Phase II Study of Fulvestrant and Erlotinib Compared with Erlotinib Alone in Patients with Advanced or Metastatic Non-small Cell Lung Cancer. Lung Cancer 123, 91–98. doi:10.1016/j.lungcan.2018.06.013
Gisselbrecht, C., Glass, B., Mounier, N., Singh Gill, D., Linch, D. C., Trneny, M., et al. (2010). Salvage Regimens with Autologous Transplantation for Relapsed Large B-Cell Lymphoma in the Rituximab Era. J. Clin. Oncol. 28 (27), 4184–4190. doi:10.1200/JCO.2010.28.1618
Goldman, J. W., Mazieres, J., Barlesi, F., Dragnev, K. H., Koczywas, M., Göskel, T., et al. (2020). A Randomized Phase III Study of Abemaciclib versus Erlotinib in Patients with Stage IV Non-small Cell Lung Cancer with a Detectable KRAS Mutation Who Failed Prior Platinum-Based Therapy: JUNIPER. Front. Oncol. 10, 578756. doi:10.3389/fonc.2020.578756
Guan, Z. Z., Zhang, L., Li, L. Y., Jiang, G. L., Liu, X. Y., Chu, D. T., et al. (2005). Efficacy of Gefitinib on Chinese Patients with Locally Advanced or Metastatic Non-small Cell Lung Cancer: a Clinical Trial. Ai Zheng 24 (8), 980–984.
Han, B., Jin, B., Chu, T., Niu, Y., Dong, Y., Xu, J., et al. (2017). Combination of Chemotherapy and Gefitinib as First-Line Treatment for Patients with Advanced Lung Adenocarcinoma and Sensitive EGFR Mutations: A Randomized Controlled Trial. Int. J. Cancer 141 (6), 1249–1256. doi:10.1002/ijc.30806
Han, B., Li, K., Wang, Q., Zhang, L., Shi, J., Wang, Z., et al. (2018). Effect of Anlotinib as a Third-Line or Further Treatment on Overall Survival of Patients with Advanced Non-small Cell Lung Cancer: The ALTER 0303 Phase 3 Randomized Clinical Trial. JAMA Oncol. 4 (11), 1569–1575. doi:10.1001/jamaoncol.2018.3039
Han, B., Li, K., Zhao, Y., Li, B., Cheng, Y., Zhou, J., et al. (2018). Anlotinib as a Third-Line Therapy in Patients with Refractory Advanced Non-small-cell Lung Cancer: a Multicentre, Randomised Phase II Trial (ALTER0302). Br. J. Cancer 118 (5), 654–661. doi:10.1038/bjc.2017.478
Higgins, J. P., Altman, D. G., Gøtzsche, P. C., Jüni, P., Moher, D., Oxman, A. D., et al. (2011). The Cochrane Collaboration's Tool for Assessing Risk of Bias in Randomised Trials. BMJ 343, d5928. doi:10.1136/bmj.d5928
Hu, X., Zhang, L., Shi, Y., Zhou, C., Liu, X., Wang, D., et al. (2015). The Efficacy and Safety of Icotinib in Patients with Advanced Non-small Cell Lung Cancer Previously Treated with Chemotherapy: A Single-Arm, Multi-Center, Prospective Study. PLoS One 10 (11), e0142500. doi:10.1371/journal.pone.0142500
Huber, R. M., Hansen, K. H., Paz-Ares Rodríguez, L., West, H. L., Reckamp, K. L., Leighl, N. B., et al. (2020). Brigatinib in Crizotinib-Refractory ALK+ NSCLC: 2-Year Follow-Up on Systemic and Intracranial Outcomes in the Phase 2 ALTA Trial. J. Thorac. Oncol. 15 (3), 404–415. doi:10.1016/j.jtho.2019.11.004
Ikezawa, Y., Asahina, H., Oizumi, S., Watanabe, M., Takamura, K., Kawai, Y., et al. (2017). A Randomized Phase II Trial of Erlotinib vs. S-1 as a Third- or Fourth-Line Therapy for Patients with Wild-type EGFR Non-small Cell Lung Cancer (HOT1002). Cancer Chemother. Pharmacol. 80 (5), 955–963. doi:10.1007/s00280-017-3432-4
Inoue, A., Suzuki, T., Fukuhara, T., Maemondo, M., Kimura, Y., Morikawa, N., et al. (2006). Prospective Phase II Study of Gefitinib for Chemotherapy-Naive Patients with Advanced Non-small-cell Lung Cancer with Epidermal Growth Factor Receptor Gene Mutations. J. Clin. Oncol. 24 (21), 3340–3346. doi:10.1200/JCO.2005.05.4692
Juan, Ó., Aparisi, F., Sánchez-Hernández, A., Muñoz-Langa, J., Esquerdo, G., García-Sánchez, J., et al. (2015). Intercalated Dosing Schedule of Erlotinib and Docetaxel as a Therapeutic Strategy to Avoid Antagonism and Optimize its Benefits in Advanced Non-small-cell Lung Cancer. A Randomized Phase II Clinical Trial. Clin. Lung Cancer 16 (3), 193–199. doi:10.1016/j.cllc.2014.11.006
Kim, S. T., Uhm, J. E., Lee, J., Sun, J. M., Sohn, I., Kim, S. W., et al. (2012). Randomized Phase II Study of Gefitinib versus Erlotinib in Patients with Advanced Non-small Cell Lung Cancer Who Failed Previous Chemotherapy. Lung Cancer 75 (1), 82–88. doi:10.1016/j.lungcan.2011.05.022
Kiura, K., Imamura, F., Kagamu, H., Matsumoto, S., Hida, T., Nakagawa, K., et al. (2018). Phase 3 Study of Ceritinib vs Chemotherapy in ALK-Rearranged NSCLC Patients Previously Treated with Chemotherapy and Crizotinib (ASCEND-5): Japanese Subset. Jpn. J. Clin. Oncol. 48 (4), 367–375. doi:10.1093/jjco/hyy016
Landi, L., Chiari, R., Tiseo, M., D'Incà, F., Dazzi, C., Chella, A., et al. (2019). Crizotinib in MET-Deregulated or ROS1-Rearranged Pretreated Non-small Cell Lung Cancer (METROS): A Phase II, Prospective, Multicenter, Two-Arms Trial. Clin. Cancer Res. 25 (24), 7312–7319. doi:10.1158/1078-0432.CCR-19-0994
Lassere, M. N., Johnson, K. R., Schiff, M., and Rees, D. (2012). Is Blood Pressure Reduction a Valid Surrogate Endpoint for Stroke Prevention? an Analysis Incorporating a Systematic Review of Randomised Controlled Trials, a By-Trial Weighted Errors-In-Variables Regression, the Surrogate Threshold Effect (STE) and the Biomarker-Surrogacy (BioSurrogate) Evaluation Schema (BSES). BMC Med. Res. Methodol. 12 (1), 27. doi:10.1186/1471-2288-12-27
Latimer, N. (2011). NICE DSU Technical Support Document 14: Undertaking Survival Analysis for Economic Evaluations Alongside Clinical Trials - Extrapolation with Patient-Level Data. London, United Kingdom: The National Institute for Health and Care Excellence.
Lee, D. H., Kim, S. W., Suh, C., Han, Y. H., and Lee, J. S. (2011). Phase II Study of Erlotinib for Chemotherapy-Naïve Patients with Advanced or Metastatic Non-small Cell Lung Cancer Who Are Ineligible for Platinum Doublets. Cancer Chemother. Pharmacol. 67 (1), 35–39. doi:10.1007/s00280-010-1280-6
Leighl, N. B., Rizvi, N. A., de Lima, L. G., Arpornwirat, W., Rudin, C. M., Chiappori, A. A., et al. (2017). Phase 2 Study of Erlotinib in Combination with Linsitinib (OSI-906) or Placebo in Chemotherapy-Naive Patients with Non-small-cell Lung Cancer and Activating Epidermal Growth Factor Receptor Mutations. Clin. Lung Cancer 18 (1), 34–e2. doi:10.1016/j.cllc.2016.07.007
Ma, W., and Yu, J.Commission NH (2020). China Health Statistical Yearbook 2020. Beijing: Peking Union Medical College Press.
Margulis, A. V., Pladevall, M., Riera-Guardia, N., Varas-Lorenzo, C., Hazell, L., Berkman, N. D., et al. (2014). Quality Assessment of Observational Studies in a Drug-Safety Systematic Review, Comparison of Two Tools: the Newcastle-Ottawa Scale and the RTI Item Bank. Clin. Epidemiol. 6, 359–368. doi:10.2147/CLEP.S66677
Michels, S., Massutí, B., Schildhaus, H. U., Franklin, J., Sebastian, M., Felip, E., et al. (2019). Safety and Efficacy of Crizotinib in Patients with Advanced or Metastatic ROS1-Rearranged Lung Cancer (EUCROSS): A European Phase II Clinical Trial. J. Thorac. Oncol. 14 (7), 1266–1276. doi:10.1016/j.jtho.2019.03.020
Miller, V. A., Hirsh, V., Cadranel, J., Chen, Y. M., Park, K., Kim, S. W., et al. (2012). Afatinib versus Placebo for Patients with Advanced, Metastatic Non-small-cell Lung Cancer after Failure of Erlotinib, Gefitinib, or Both, and One or Two Lines of Chemotherapy (LUX-Lung 1): a Phase 2b/3 Randomised Trial. Lancet Oncol. 13 (5), 528–538. doi:10.1016/S1470-2045(12)70087-6
Miyawaki, M., Naoki, K., Yoda, S., Nakayama, S., Satomi, R., Sato, T., et al. (2017). Erlotinib as Second- or Third-Line Treatment in Elderly Patients with Advanced Non-small Cell Lung Cancer: Keio Lung Oncology Group Study 001 (KLOG001). Mol. Clin. Oncol. 6 (3), 409–414. doi:10.3892/mco.2017.1154
Neal, J. W., Dahlberg, S. E., Wakelee, H. A., Aisner, S. C., Bowden, M., Huang, Y., et al. (2016). Erlotinib, Cabozantinib, or Erlotinib Plus Cabozantinib as Second-Line or Third-Line Treatment of Patients with EGFR Wild-type Advanced Non-small-cell Lung Cancer (ECOG-ACRIN 1512): a Randomised, Controlled, Open-Label, Multicentre, Phase 2 Trial. Lancet Oncol. 17 (12), 1661–1671. doi:10.1016/S1470-2045(16)30561-7
Nie, K., Zhang, Z., Zhang, C., Geng, C., Zhang, L., Xu, X., et al. (2018). Osimertinib Compared Docetaxel-Bevacizumab as Third-Line Treatment in EGFR T790M Mutated Non-small-cell Lung Cancer. Lung Cancer 121, 5–11. doi:10.1016/j.lungcan.2018.04.012
Ohe, Y., Imamura, F., Nogami, N., Okamoto, I., Kurata, T., Kato, T., et al. (2019). Osimertinib versus Standard-Of-Care EGFR-TKI as First-Line Treatment for EGFRm Advanced NSCLC: FLAURA Japanese Subset. Jpn. J. Clin. Oncol. 49 (1), 29–36. doi:10.1093/jjco/hyy179
Pallis, A. G., Voutsina, A., Kentepozidis, N., Giassas, S., Papakotoulas, P., Agelaki, S., et al. (2012). A Phase II Trial of Erlotinib as Front-Line Treatment in Clinically Selected Patients with Non-small-cell Lung Cancer. Clin. Lung Cancer 13 (2), 129–135. doi:10.1016/j.cllc.2011.08.004
Park, K., Tan, E. H., O'Byrne, K., Zhang, L., Boyer, M., Mok, T., et al. (2016). Afatinib versus Gefitinib as First-Line Treatment of Patients with EGFR Mutation-Positive Non-small-cell Lung Cancer (LUX-Lung 7): a Phase 2B, Open-Label, Randomised Controlled Trial. Lancet Oncol. 17 (5), 577–589. doi:10.1016/S1470-2045(16)30033-X
Pérol, M., Chouaid, C., Pérol, D., Barlési, F., Gervais, R., Westeel, V., et al. (2012). Randomized, Phase III Study of Gemcitabine or Erlotinib Maintenance Therapy versus Observation, with Predefined Second-Line Treatment, after Cisplatin-Gemcitabine Induction Chemotherapy in Advanced Non-small-cell Lung Cancer. J. Clin. Oncol. 30 (28), 3516–3524.
Peters, S., Camidge, D. R., Shaw, A. T., Gadgeel, S., Ahn, J. S., Kim, D. W., et al. (2017). Alectinib versus Crizotinib in Untreated ALK-Positive Non-small-cell Lung Cancer. N. Engl. J. Med. 377 (9), 829–838. doi:10.1056/NEJMoa1704795
Ramalingam, S. S., Blackhall, F., Krzakowski, M., Barrios, C. H., Park, K., Bover, I., et al. (2012). Randomized Phase II Study of Dacomitinib (PF-00299804), an Irreversible Pan-Human Epidermal Growth Factor Receptor Inhibitor, versus Erlotinib in Patients with Advanced Non-small-cell Lung Cancer. J. Clin. Oncol. 30 (27), 3337–3344. doi:10.1200/JCO.2011.40.9433
Ramalingam, S. S., Jänne, P. A., Mok, T., O'Byrne, K., Boyer, M. J., Von Pawel, J., et al. (2014). Dacomitinib versus Erlotinib in Patients with Advanced-Stage, Previously Treated Non-small-cell Lung Cancer (ARCHER 1009): a Randomised, Double-Blind, Phase 3 Trial. Lancet Oncol. 15 (12), 1369–1378. doi:10.1016/S1470-2045(14)70452-8
Rui, M., Wang, Y., Fei, Z., Zhang, X., Shang, Y., and Li, H. (2021). Will the Markov Model and Partitioned Survival Model lead to Different Results? A Review of Recent Economic Evidence of Cancer Treatments. Expert Rev. Pharmacoeconomics Outcomes Res. 21 (3), 373–380. doi:10.1080/14737167.2021.1893167
Rule, S., Dreyling, M., Goy, A., Hess, G., Auer, R., Kahl, B., et al. (2017). Outcomes in 370 Patients with Mantle Cell Lymphoma Treated with Ibrutinib: a Pooled Analysis from Three Open-Label Studies. Br. J. Haematol. 179 (3), 430–438. doi:10.1111/bjh.14870
Saito, H., Fukuhara, T., Furuya, N., Watanabe, K., Sugawara, S., Iwasawa, S., et al. (2019). Erlotinib Plus Bevacizumab versus Erlotinib Alone in Patients with EGFR-Positive Advanced Non-squamous Non-small-cell Lung Cancer (NEJ026): Interim Analysis of an Open-Label, Randomised, Multicentre, Phase 3 Trial. Lancet Oncol. 20 (5), 625–635. doi:10.1016/S1470-2045(19)30035-X
Scagliotti, G., Moro-Sibilot, D., Kollmeier, J., Favaretto, A., Cho, E. K., Grosch, H., et al. (2020). A Randomized-Controlled Phase 2 Study of the MET Antibody Emibetuzumab in Combination with Erlotinib as First-Line Treatment for EGFR Mutation-Positive NSCLC Patients. J. Thorac. Oncol. 15 (1), 80–90. doi:10.1016/j.jtho.2019.10.003
Shi, Y., Zhang, L., Liu, X., Zhou, C., Zhang, L., Zhang, S., et al. (2013). Icotinib versus Gefitinib in Previously Treated Advanced Non-small-cell Lung Cancer (ICOGEN): a Randomised, Double-Blind Phase 3 Non-inferiority Trial. Lancet Oncol. 14 (10), 953–961. doi:10.1016/S1470-2045(13)70355-3
Spigel, D. R., Burris, H. A., Greco, F. A., Shih, K. C., Gian, V. G., Lipman, A. J., et al. (2018). Erlotinib Plus Either Pazopanib or Placebo in Patients with Previously Treated Advanced Non-small Cell Lung Cancer: A Randomized, Placebo-Controlled Phase 2 Trial with Correlated Serum Proteomic Signatures. Cancer 124 (11), 2355–2364. doi:10.1002/cncr.31290
Spigel, D. R., Burris, H. A., Greco, F. A., Shipley, D. L., Friedman, E. K., Waterhouse, D. M., et al. (2011). Randomized, Double-Blind, Placebo-Controlled, Phase II Trial of Sorafenib and Erlotinib or Erlotinib Alone in Previously Treated Advanced Non-small-cell Lung Cancer. J. Clin. Oncol. 29 (18), 2582–2589. doi:10.1200/JCO.2010.30.7678
Uchibori, K., Satouchi, M., Sueoka-Aragane, N., Urata, Y., Sato, A., Imamura, F., et al. (2018). Phase II Trial of Gefitinib Plus Pemetrexed after Relapse Using First-Line Gefitinib in Patients with Non-small Cell Lung Cancer Harboring EGFR Gene Mutations. Lung Cancer 124, 65–70. doi:10.1016/j.lungcan.2018.07.031
Wei-jing, W., and xiao-lu, Z. (2019). Current Status and Disease burden of Cancer in China. China Econ. Rep. 2019 (04), 63–73.
Wen, Z. S., Chen, X. Q., Wu, H. Y., Wei, W. D., and Rong, T. H. (2007). Efficacy of Gefitinib on Advanced Non-small Cell Lung Cancer of Bilateral Diffuse or Unilateral Giant Mass Type. Ai Zheng 26 (4), 415–417.
Wu, Y. L., Ahn, M. J., Garassino, M. C., Han, J. Y., Katakami, N., Kim, H. R., et al. (2018). CNS Efficacy of Osimertinib in Patients with T790M-Positive Advanced Non-small-cell Lung Cancer: Data from a Randomized Phase III Trial (AURA3). J. Clin. Oncol. 36 (26), 2702–2709. doi:10.1200/JCO.2018.77.9363
Wu, Y. L., Lee, J. S., Thongprasert, S., Yu, C. J., Zhang, L., Ladrera, G., et al. (2013). Intercalated Combination of Chemotherapy and Erlotinib for Patients with Advanced Stage Non-small-cell Lung Cancer (FASTACT-2): a Randomised, Double-Blind Trial. Lancet Oncol. 14 (8), 777–786. doi:10.1016/S1470-2045(13)70254-7
Wu, Y. L., Xu, C. R., Hu, C. P., Feng, J., Lu, S., Huang, Y., et al. (2018). Afatinib versus Gemcitabine/cisplatin for First-Line Treatment of Chinese Patients with Advanced Non-small-cell Lung Cancer Harboring EGFR Mutations: Subgroup Analysis of the LUX-Lung 6 Trial. Onco Targets Ther. 11, 8575–8587. doi:10.2147/OTT.S160358
Wu, Y. L., Zhou, C., Liam, C. K., Wu, G., Liu, X., Zhong, Z., et al. (2015). First-line Erlotinib versus Gemcitabine/cisplatin in Patients with Advanced EGFR Mutation-Positive Non-small-cell Lung Cancer: Analyses from the Phase III, Randomized, Open-Label, ENSURE Study. Ann. Oncol. 26 (9), 1883–1889. doi:10.1093/annonc/mdv270
Xu, L., Qi, Q., Zhang, Y., Cui, J., Liu, R., and Li, Y. (2019). Combination of Icotinib and Chemotherapy as First-Line Treatment for Advanced Lung Adenocarcinoma in Patients with Sensitive EGFR Mutations: A Randomized Controlled Study. Lung Cancer 133, 23–31. doi:10.1016/j.lungcan.2019.05.008
Yamada, K., Takayama, K., Kawakami, S., Saruwatari, K., Morinaga, R., Harada, T., et al. (2013). Phase II Trial of Erlotinib for Japanese Patients with Previously Treated Non-small-cell Lung Cancer Harboring EGFR Mutations: Results of Lung Oncology Group in Kyushu (LOGiK0803). Jpn. J. Clin. Oncol. 43 (6), 629–635. doi:10.1093/jjco/hyt056
Yang, J. C., Ahn, M. J., Kim, D. W., Ramalingam, S. S., Sequist, L. V., Su, W. C., et al. (2017). Osimertinib in Pretreated T790M-Positive Advanced Non-small-cell Lung Cancer: AURA Study Phase II Extension Component. J. Clin. Oncol. 35 (12), 1288–1296. doi:10.1200/JCO.2016.70.3223
Yang, J. C., Ou, S. I., De Petris, L., Gadgeel, S., Gandhi, L., Kim, D. W., et al. (2017). Pooled Systemic Efficacy and Safety Data from the Pivotal Phase II Studies (NP28673 and NP28761) of Alectinib in ALK-Positive Non-small Cell Lung Cancer. J. Thorac. Oncol. 12 (10), 1552–1560. doi:10.1016/j.jtho.2017.06.070
Yang, J. C., Shepherd, F. A., Kim, D. W., Lee, G. W., Lee, J. S., Chang, G. C., et al. (2019). Osimertinib Plus Durvalumab versus Osimertinib Monotherapy in EGFR T790M-Positive NSCLC Following Previous EGFR TKI Therapy: CAURAL Brief Report. J. Thorac. Oncol. 14 (5), 933–939. doi:10.1016/j.jtho.2019.02.001
Yang, X. B., Chai, X. S., Wu, W. Y., Long, S. Q., Deng, H., Pan, Z. Q., et al. (2018). Gefitinib Plus Fuzheng Kang'ai Formula () in Patients with Advanced Non-small Cell Lung Cancer with Epidermal Growth Factor Receptor Mutation: A Randomized Controlled Trial. Chin. J. Integr. Med. 24 (10), 734–740. doi:10.1007/s11655-017-2819-8
Yang, Y., Zhou, J., Zhou, J., Feng, J., Zhuang, W., Chen, J., et al. (2020). Efficacy, Safety, and Biomarker Analysis of Ensartinib in Crizotinib-Resistant, ALK-Positive Non-small-cell Lung Cancer: a Multicentre, Phase 2 Trial. Lancet Respir. Med. 8 (1), 45–53. doi:10.1016/S2213-2600(19)30252-8
Yokoyama, T., Yoshioka, H., Fujimoto, D., Demura, Y., Hirano, K., Kawai, T., et al. (2019). A Phase II Study of Low Starting Dose of Afatinib as First-Line Treatment in Patients with EGFR Mutation-Positive Non-small-cell Lung Cancer (KTORG1402). Lung Cancer 135, 175–180. doi:10.1016/j.lungcan.2019.03.030
Yoshimura, N., Kudoh, S., Mitsuoka, S., Yoshimoto, N., Oka, T., Nakai, T., et al. (2015). Phase II Study of a Combination Regimen of Gefitinib and Pemetrexed as First-Line Treatment in Patients with Advanced Non-small Cell Lung Cancer Harboring a Sensitive EGFR Mutation. Lung Cancer 90 (1), 65–70. doi:10.1016/j.lungcan.2015.06.002
Zhou, J., Zheng, J., Zhang, X., Zhao, J., Zhu, Y., Shen, Q., et al. (2018). Crizotinib in Patients with Anaplastic Lymphoma Kinase-Positive Advanced Non-small Cell Lung Cancer versus Chemotherapy as a First-Line Treatment. BMC Cancer 18 (1), 10. doi:10.1186/s12885-017-3720-8
Keywords: malignant tumor, short-term surrogate endpoint, median progression-free survival, median overall survival, non-small-cell lung cancer
Citation: Rui M, Wang Z, Fei Z, Wu Y, Wang Y, Sun L, Shang Y and Li H (2022) The Relationship Between Short-Term Surrogate Endpoint Indicators and mPFS and mOS in Clinical Trials of Malignant Tumors: A Case Study of Approved Molecular Targeted Drugs for Non-Small-Cell Lung Cancer in China. Front. Pharmacol. 13:862640. doi: 10.3389/fphar.2022.862640
Received: 26 January 2022; Accepted: 28 February 2022;
Published: 16 March 2022.
Edited by:
Kevin Lu, University of South Carolina, United StatesCopyright © 2022 Rui, Wang, Fei, Wu, Wang, Sun, Shang and Li. This is an open-access article distributed under the terms of the Creative Commons Attribution License (CC BY). The use, distribution or reproduction in other forums is permitted, provided the original author(s) and the copyright owner(s) are credited and that the original publication in this journal is cited, in accordance with accepted academic practice. No use, distribution or reproduction is permitted which does not comply with these terms.
*Correspondence: Hongchao Li, bGlob25nY2hhb0BjcHUuZWR1LmNu
Disclaimer: All claims expressed in this article are solely those of the authors and do not necessarily represent those of their affiliated organizations, or those of the publisher, the editors and the reviewers. Any product that may be evaluated in this article or claim that may be made by its manufacturer is not guaranteed or endorsed by the publisher.
Research integrity at Frontiers
Learn more about the work of our research integrity team to safeguard the quality of each article we publish.