- 1Guangzhou University of Chinese Medicine, Guangzhou, China
- 2Department of Oncology, The First Affiliated Hospital of Guangzhou University of Chinese Medicine, Guangzhou, China
Background: Severe acute respiratory syndrome coronavirus 2 (SARS-CoV-2), the leading cause of coronavirus disease-2019 (COVID-19), is an emerging global health crisis. Lung cancer patients are at a higher risk of COVID-19 infection. With the increasing number of non-small-cell lung cancer (NSCLC) patients with COVID-19, there is an urgent need of efficacious drugs for the treatment of COVID-19/NSCLC.
Methods: Based on a comprehensive bioinformatic and systemic biological analysis, this study investigated COVID-19/NSCLC interactional hub genes, detected common pathways and molecular biomarkers, and predicted potential agents for COVID-19 and NSCLC.
Results: A total of 122 COVID-19/NSCLC interactional genes and 21 interactional hub genes were identified. The enrichment analysis indicated that COVID-19 and NSCLC shared common signaling pathways, including cell cycle, viral carcinogenesis, and p53 signaling pathway. In total, 10 important transcription factors (TFs) and 44 microRNAs (miRNAs) participated in regulations of 21 interactional hub genes. In addition, 23 potential candidates were predicted for the treatment of COVID-19 and NSCLC.
Conclusion: This study increased our understanding of pathophysiology and screened potential drugs for COVID-19 and NSCLC.
Introduction
Severe acute respiratory syndrome coronavirus 2 (SARS-CoV-2), responsible for pandemic coronavirus disease-2019 (COVID-19), is a novel beta-coronavirus belonging to the subgenus Sarbecovirus (Ciotti et al., 2020; Umakanthan et al., 2020). As an emerging global health crisis, SARS-CoV-2 shares a similar transmission mode with other respiratory viruses, mainly through air droplets and close contact (Shang et al., 2021). Due to the rapidly evolving nature of SARS-CoV-2, as on 21 November 2021, there had been 257,788,585 confirmed cases with mortality calculated at 2% (Worldometer, 2021). Recently, the development of Delta and Omicron variants has further complicated the control of the pandemic (Lopez Bernal et al., 2021; Wang and Powell, 2021). About 80% of COVID-19 patients exhibit mild to moderate clinical manifestations, including fever, dyspnea, dry cough, and acute pneumonia (Liu et al., 2020; Mallah et al., 2021; Shang et al., 2021). The case fatality rate for COVID-19 shows a close connection between age, underlying disease status, and immune state (Olloquequi, 2020). A growing body of evidence shows that cancer patients harbor a higher risk of COVID-19 infection, along with severe events and unfavorable outcomes (Passaro et al., 2021; Sinha and Kundu, 2021). A cohort study of 1,590 COVID-19 cases reported that lung cancer was the most frequent type of cancer [5 (28%) of 18 cancer patients] due to the inherent associated pulmonary fragility (Liang et al., 2020). Moreover, lung cancer patients with smoking-related lung damage, significant cardiovascular or respiratory comorbidities, and older age are more likely to develop COVID-19 severity (Berlin et al., 2020; Liang et al., 2020; Passaro et al., 2020). According to the Global Cancer Statistics in 2020, lung cancer remains to be the leading cause of cancer incidence and mortality, representing 11.4% and 18.0% of all cases, respectively (Sung et al., 2021). Accounting for approximately 85% of lung cancers, non-small-cell lung cancer (NSCLC) is comprised of several histological subtypes such as lung adenocarcinoma, squamous-cell carcinoma, and large-cell carcinoma (Zappa and Mousa, 2016). Despite chemotherapy and targeted therapies being widely applied for the treatment of NSCLC, the 5-year survival rate has remained abysmally low (16%) for the last four decades (Suresh et al., 2019).
Cell proliferation is a vital and fundamental mechanism for growth, development, and regeneration of eukaryotic organisms (Diaz-Moralli et al., 2013). Dysregulation of the cell cycle leads to aberrant cell proliferation, which is found in various malignancies (Williams and Stoeber, 2012). Most NSCLCs have detectable cell cycle abnormalities, and the more defective the cell cycle becomes, the more severe the consequences would be (Sterlacci et al., 2012). Driven by complex interactions between host factors, tumorigenesis creates an ideal tumor microenvironment and promotes tumor formation (Dzobo, 2021). Prior studies have pointed out that the tumor microenvironment not only played an important part in tumor development at primary and metastatic sites but also deteriorated viral infection (Tian et al., 2020; Malkani and Rashid, 2021). In lung cancer patients, the tumor microenvironment supports SARS-CoV-2 proteins by activating cytokine storm- and cellular metabolic variation-related pathways, which further accelerate infection and weaken the immune system. Angiotensin-converting enzyme 2 (ACE2), an entry receptor for SARS-CoV-2, almost ubiquitously present in human organs, but primarily expressed in alveolar epithelial type II cells, secretes surfactant and plays a crucial part in pulmonary gas exchange (Zhou P. et al., 2020; Walls et al., 2020). In lung cancer tissues, significantly upregulated ACE2 caused lung parenchyma to become vulnerable to SARS-CoV-2 attack (Zhang L. et al., 2020; Mason, 2020). By triggering associated cascades, SARS-CoV-2 infections increased inflammatory mediators, which could induce paracrine senescence through prolonging cytokine signaling. Accumulative evidence suggests that cellular senescence damages vascular functions persistently by impairing endothelium and decreasing angiogenesis. Vascular dysfunction strikes the balance between anti- and procoagulant pathways and further increases the risk of abnormal coagulation (thrombosis) (Bochenek et al., 2016; Escher et al., 2020). To conclude, persistent inflammation and cellular senescence are associated with pulmonary parenchyma injury, which could potentially restrict blood flow to the lungs, activate coagulation, induce capillary damage, and eventually contribute to hypoxemia and acute respiratory distress in COVID-19 and NSCLC patients.
In the context of the highly contagious COVID-19 pandemic, the number of NSCLC patients with COVID-19 continues to rise (Worldometer, 2021). Although remdesivir (Eastman et al., 2020; Hung et al., 2020), ribavirin, and hydroxychloroquine (Yao et al., 2020) have been approved for COVID-19, none of them have been suggested to be specific. With the widespread application of high-throughput technologies, a large amount of biological data has been generated. We performed a comprehensive bioinformatic and systemic biological analysis to further understand mechanisms and seek potential efficacious drugs to combat COVID-19/NSCLC (Figure 1).
Materials and Methods
Identification of Genetic Interrelations Between COVID-19 and Non-Small-Cell Lung Cancer
To determine genetic interrelations shared by COVID-19 and NSCLC, we searched a variety of databases to collect available data for each disease. We searched six online databases for COVID-19–related genes, including Online Mendelian Inheritance in Man (OMIM, https://www.omim.org/) (Hamosh et al., 2005), Therapeutic Target Database (TTD, http://db.idrblab.net/ttd/) (Wang et al., 2020), PubChem (https://pubchem.ncbi.nlm.nih.gov/) (Kim S. et al., 2019), DisGeNET (https://www.disgenet.org/covid/diseases/summary/) (Piñero et al., 2020), GeneCards (https://www.genecards.org/) (Stelzer et al., 2016), and Comparative Toxicogenomics Database (CTD, http://ctdbase.org/) (Davis et al., 2021). Transcriptomic RNA-sequencing (RNA-seq) datasets of COVID-19 [GSE147507 (Daamen et al., 2021), GSE157103 (Overmyer et al., 2021), and GSE166190 (Vono et al., 2021)] were downloaded from Gene Expression Omnibus (GEO, https://www.ncbi.nlm.nih.gov/geo/) (Clough and Barrett, 2016). Then, we used the R package Deseq2 (Love et al., 2014) to extract differentially expressed genes (DEGs) at the threshold |log2FoldChange| >1 and adjusted the p-value <0.05. COVID-19–related genes were identified by intersecting related genes from online databases and GEO datasets.
In addition, five online platforms were searched for NSCLC-related genes, including OMIM (Hamosh et al., 2005), CTD (Davis et al., 2021), TTD (Wang et al., 2020), DisGeNET (Piñero et al., 2020), and GeneCards (Stelzer et al., 2016). RNA-seq datasets of NSCLC were obtained from The Cancer Genome Atlas (TCGA) data portal on the UCSC Xena database (https://xenabrowser.net/datapages/) (Goldman et al., 2020). Data of 1,135 tissues (1,027 cancer tissues and 108 para-cancerous tissues) from 1,016 NSCLC patients were obtained.
To obtain the robust and biologically significant DEG list, we extracted DEGs from TCGA-NSCLC–related genes by combining data from lung cancers with different pathological classifications, according to the previous studies (Han et al., 2019; Zhang J. et al., 2020; Zhang et al., 2021). We employed the R package Deseq2 (Love et al., 2014), set the threshold |log2FoldChange| at >1, and adjusted the p-value at <0.05. NSCLC-related genes were collected by intersecting related genes from public platforms and TCGA data portal. After intersecting the COVID-19–related genes and NSCLC-related genes, we identified genes shared by COVID-19 and NSCLC as interactional genes. These genes might play important roles in pathophysiological processes of COVID-19 and NSCLC and serve as important clues for screening candidate drugs for NSCLC patients with COVID-19.
Protein–Protein Interaction Network Analysis and Sub-Network Analysis
To interpret associated cellular machinery operations and explore protein mechanisms, PPI network analysis was conducted using STRING (https://string-db.org/) based on proteins derived from COVID-19/NSCLC interactional genes. Active interaction sources of the PPI mainly included text-mining, experiments, databases, co-expression, neighborhood, gene fusion, and co-occurrence. Moreover, to assure the highest confidence of the network, the minimum confidence score was set at 0.90 for network construction. Disconnected nodes were removed from the network. Then Cytoscape 3.9.0 software (Shannon et al., 2003) was applied for network visualization. In the network, the significance of each node was evaluated by betweenness centrality, degree and closeness centrality. In addition, a network analysis module named cytoHubba (Chin et al., 2014) was employed for detecting interactional hub genes. Analytical methods including betweenness, bottleneck, closeness, degree, density of maximum neighborhood component (DMNC), eccentricity, edge-percoalated component (EPC), maximal clique centrality (MCC), maximum neighborhood component (MNC), radiality, and stress were applied to obtain top 10 genes of each method. Based on previous studies, hub genes were defined as genes with a degree value twice the median or more in the whole network (Guo et al., 2015; Yu et al., 2018; Zhuang et al., 2020). Hub genes and genes obtained by the cytoHubba module were intersected, and duplication was removed. By employing these 12 approaches, COVID-19/NSCLC interactional hub genes were identified. Furthermore, sub-network analyses were conducted by the Molecular Complex Detection (MCODE) (Bader and Hogue, 2003) module of Cytoscape 3.9.0 software (Shannon et al., 2003) to identify important gene clusters in the whole PPI network. MCODE is a useful tool for detecting densely connected regions in large protein–protein interaction networks, which helps to identify gene clusters and understand the connectivity and proximity of genes. In the present study, the parameters of MCODE were set as follows: degree cutoff: 2; cluster finding method: haircut; node score cutoff: 0.2; K-Core: 2; and maximal depth: 100.
Gene Ontology and Kyoto Encyclopedia of Genes and Genomes Pathway Analysis
Based on COVID-19/NSCLC interactional genes, GO analysis and KEGG pathway analyses were carried out by the R package clusterProfiler (Wu et al., 2021) to explore potential pathogenic mechanisms of COVID-19 and NSCLC. GO analysis classified associated mechanisms into three categories, including biological processes (BP), cellular components (CC), and molecular functions (MF), and then KEGG pathway analysis further specified related mechanisms. p-value <0.05 was set as a standard metric to quantify the most closely related GO and KEGG terms. Furthermore, to explore the function of interactional genes in the biggest sub-network, GO terms and KEGG pathway terms (p-value < 0.05) relevant to interactional genes were also identified by the R package clusterProfiler (Wu et al., 2021).
Identification of Transcription Factors and MiRNAs Interacting With Interactional Hub Genes
To study underlying regulatory mechanisms at the transcriptional level and identify hub protein’s regulatory molecules, a comprehensive network-based method was employed to decipher regulatory TFs and miRNAs. ChIP-X Enrichment Analysis 3 (ChEA3, https://amp.pharm.mssm.edu/chea3/) (Keenan et al., 2019) is an open-access tool for identifying TFs that are in control of observed alters in gene expression. ChEA3 assembles TFs data from ENCODE (Davis et al., 2018), ReMap (Chèneby et al., 2020), Genotype-Tissue Expression (GTEx) (Carithers and Moore, 2015), ARCHS4 (Lachmann et al., 2018), and Enrichr (Kuleshov et al., 2016). Evidence mainly from ChIP-seq experiments assures the interaction between TFs and customized input genes. The integration method MeanRank performed the best in the ChEA3 benchmark and was recommended as the preferable method to present results from different TFs libraries. Results from each library were sorted in ascending order by score using ChEA3. The lower the score, the closer the connection between a gene and a TF. In the study, TFs of COVID-19/NSCLC interactional hub genes were retrieved from ChEA3. Moreover, top 10 TFs with the lowest scores were selected for each gene after removing duplication. Experimentally supported data about the relation of miRNAs to COVID-19/NSCLC interactional hub genes were obtained from Tarbase (Karagkouni et al., 2018) and miRTarbase databases (Chou et al., 2018). To assure the accuracy and robustness of results, we searched the Tarbase database and selected miRNAs that were identified by low-throughput experimental techniques. In the miRTarbase database, the source of miRNAs included a reporter assay, western blot, and qt-PCR. Species was set to Homo sapiens in these two databases. Subsequently, regulatory networks of TFs, miRNAs, and COVID-19/NSCLC interactional hub genes were also constructed by Cytoscape 3.9.0 software (Shannon et al., 2003).
Evaluation of Applicant Drugs for COVID-19 and Non-Small-Cell Lung Cancer
Evaluation of protein–drug interactions is a crucial strategy to detect structural features and respond to receptor sensitivity of proteins, which can pave the way for drug development. To explore promising drugs for COVID-19/NSCLC, drug–proteins interactions were retrieved from the Enrichr database (https://maayanlab.cloud/Enrichr/) (Kuleshov et al., 2016) and the ShinyGO v0.75 web tool (http://bioinformatics.sdstate.edu/go/) (Ge et al., 2020).
Enrichr, containing 192 gene-set libraries and counting, is a comprehensive web portal for gene-set enrichment analysis (Kuleshov et al., 2016). Two series of Enrichr library were downloaded and analyzed, including drug perturbations from GEO (DPFG) and a collection of Drug Signatures Database (DSigDB). DPFG was built based on experimentally supported data from GEO in which gene expression levels were assessed before and after drug administration. Two types of data were retrieved from DPFG for subsequent analyses, including data on the relation of a drug to upregulated genes and data on the relation of a drug to downregulated genes. Species was set to Homo sapiens for filtering data. DSigDB is a collection of 22,527 drug-related gene sets with 17,389 compounds covering genes (Yoo et al., 2015). ShinyGO v0.75 is a graphical tool for gene-set enrichment analysis, which is accessible to KEGG and STRING. It applies false discovery rate (FDR) as an adjustment method for p-values of enrichment terms. To obtain robust protein–drug interactions, we merely selected drug molecules which targeted more than half of COVID-19/NSCLC interactional hub genes. Enrichment terms from ShinyGO v0.75 with an adjusted p-value <0.05 were included. Finally, drug molecules obtained from Enrichr libraries and ShinyGO v0.75 were carefully searched on PubChem and PubMed databases. Drug molecules with anticancer and/or antivirus effect were finally selected by browsing relevant articles carefully.
Result
Identification of Interactional Genes Between COVID-19 and Non-Small-Cell Lung Cancer
COVID-19–related genes retrieved from OMIM, TTD, PubChem, DisGeNET, GeneCards, and CTD were 2, 78, 628, 1,832, 2,572, and 9,860, respectively (Supplementary File S1). After removing duplication, a total of 11,392 related genes of COVID-19 were obtained from these online databases. COVID-19–related DEGs obtained from the RNA-seq dataset GSE147507, GSE157103, and GSE166190 were 4,825 (3,064 upregulated and 1,761 downregulated), 3,089 (1,422 upregulated and 1,667 downregulated), and 494 (278 upregulated and 216 downregulated), respectively (Table 1; Supplementary File S2). By intersecting COVID-19–related genes from online databases and DEGs from RNA-seq datasets, we obtained 3,795 COVID-19–related genes (Figure 2A).
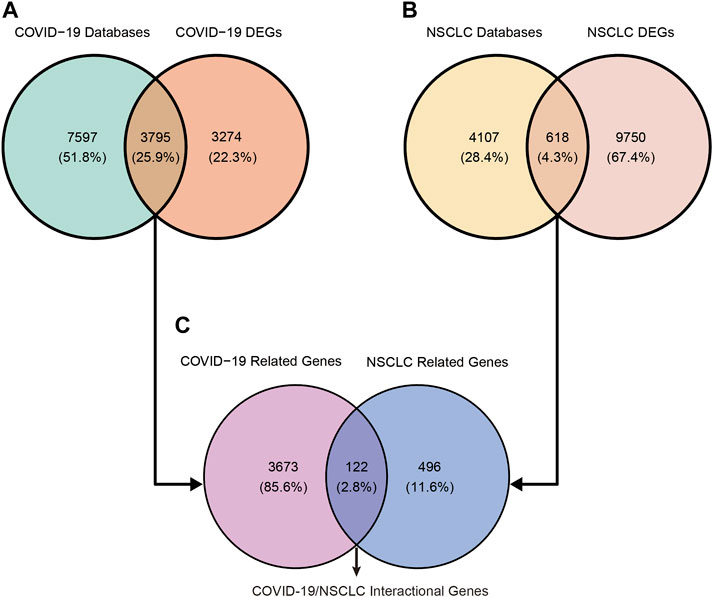
FIGURE 2. Identification of COVID-19/NSCLC interactional genes by intersecting COVID-19–related genes and NSCLC-related genes from public databases and DEGs from RNA-seq datasets. (A) Identification of COVID-19-related genes. (B) Identification of NSCLC-related genes. (C) Identification of COVID-19/NSCLC interactional genes.
NSCLC-related genes collected from OMIM, CTD, TTD, DisGeNET, and GeneCards were 15, 144, 167, 447, and 4,536, respectively (Supplementary File S3). After removing duplication, a total of 4,725 related genes of NSCLC were obtained. A total of 10,368 DEGs (8,229 upregulated and 2,139 downregulated) of NSCLC were identified from the TCGA (Supplementary File S4). By intersecting NSCLC-related genes from online platforms and DEGs from the TCGA, we obtained 618 NSCLC-related target genes (Figure 2B). Finally, a total of 122 COVID-19/NSCLC interactional genes were identified by intersecting COVID-19–related genes and NSCLC-related genes (Figure 2C).
Systemic Biological Significance of Interactional COVID-19/Non-Small-Cell Lung Cancer Genes
Systemic biology provides multivariate approaches to analyze the larger interactive network of biological pathways holistically and identify important players in disease onset and progression (Starchenko and Lauffenburger, 2018). To reveal characteristics shared by COVID-19 and NSCLC at the genetic level based on systemic biology, GO and KEGG pathway enrichment analyses were performed based on 122 COVID-19/NSCLC interactional genes. As a result, 392 GO terms (BP: 352; CC: 26; and MF: 14) and 23 KEGG pathways were highlighted. The top 10 GO terms of each ontology and 23 KEGG pathways are shown in Figure 3. Representative BP terms included cell aging, regulation of mitotic cell cycle phase transition, and regulation of cell cycle phase transition; representative CC terms included condensed chromosome kinetochore, kinetochore, and condensed chromosome; representative MF terms included cytokine activity, receptor ligand activity, and DNA binding and bending. In addition, representative pathways included the Janus kinase/signal transducers and activators of transcription (JAK/STAT) signaling pathway, interleukin (IL)-17 signaling pathway, chemical carcinogenesis receptor activation, cell cycle, and cellular senescence. These GO terms and KEGG pathways may exert a synergistic effect in the morbidity of COVID-19/NSCLC, which could be clues of therapeutic strategies for these two diseases.
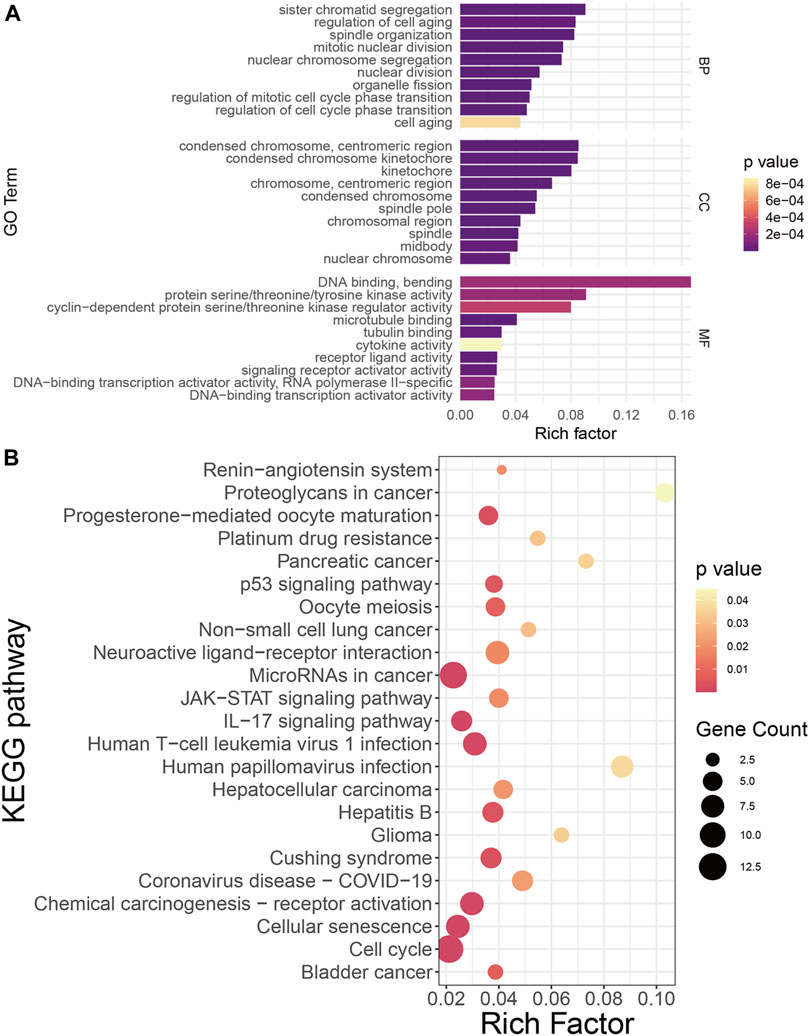
FIGURE 3. Functional annotation of COVID-19/NSCLC interactional genes. (A) GO analysis of COVID-19/NSCLC interactional genes. (B) KEGG pathway analysis of COVID-19/NSCLC interactional genes. Note: rich factor is defined as the ratio of input genes that are annotated in a term to all genes that are annotated in this term. The computational formula of rich factor is as follows: Rich factor = number of input genes under this pathway term/number of all annotated genes under this pathway term. The greater the rich factor, the greater the degree of pathway enrichment.
Identification of Hub Genes and Exploration of Their Interactions
To explore interrelationships of 122 COVID-19/NSCLC interactional genes, a PPI network containing 59 nodes and 347 edges with the highest confidence scores has been visualized (Figures 4A, 5A). Key parameters of the network were as follows: mean betweenness: 0.05, mean degree: 11.7, and mean closeness: 0.59. A total of 25 DEGs with a degree twice the median or more were identified by the PPI network (Figure 4B). In addition, the other 11 analytical network approaches were employed to identify the top 10 genes for each approach (Table 2). After intersecting genes identified by different approaches, a total of 21 COVID-19/NSCLC interactional hub genes were finally obtained for subsequent analyses. The detail information of these 21 genes is shown in Table 3.
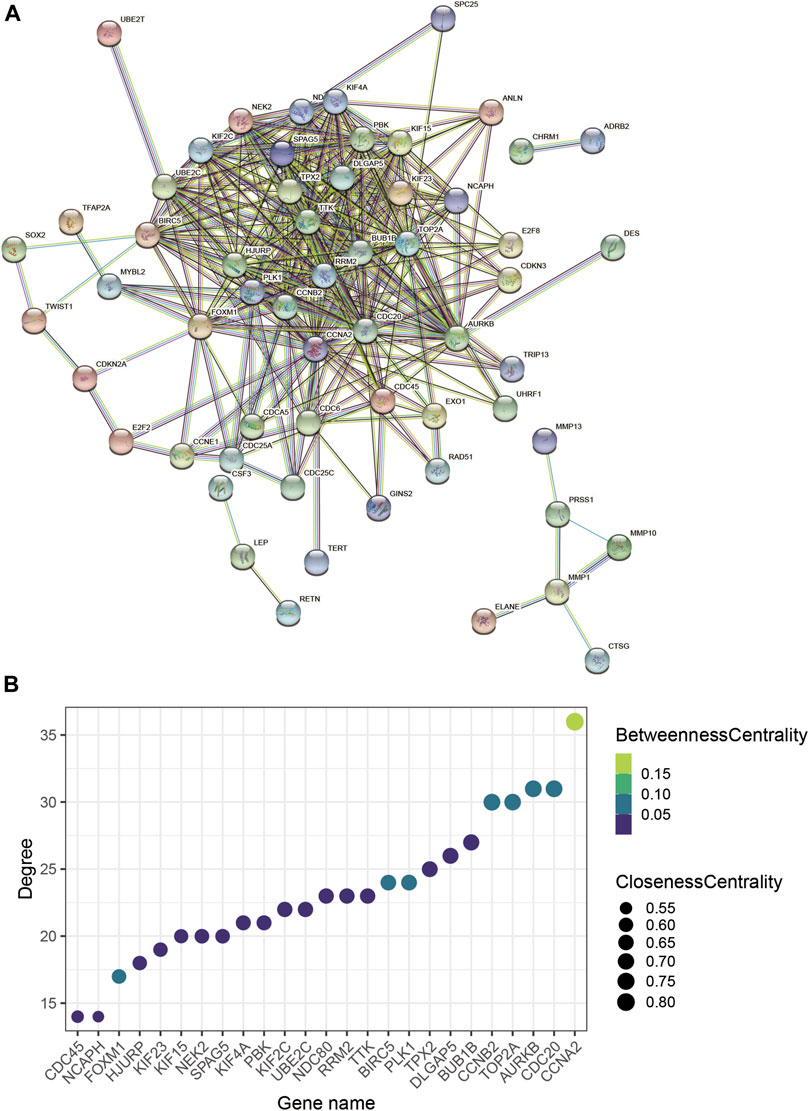
FIGURE 4. PPI network analysis based on COVID-19/NSCLC interactional genes. (A) PPI network containing 59 nodes and 347 edges. (B) Bubble chart of the genes with degree value more than the two-fold median degree value in the whole network. Note: nodes represent interactional genes, and edges represent interaction relationships in panel (A).
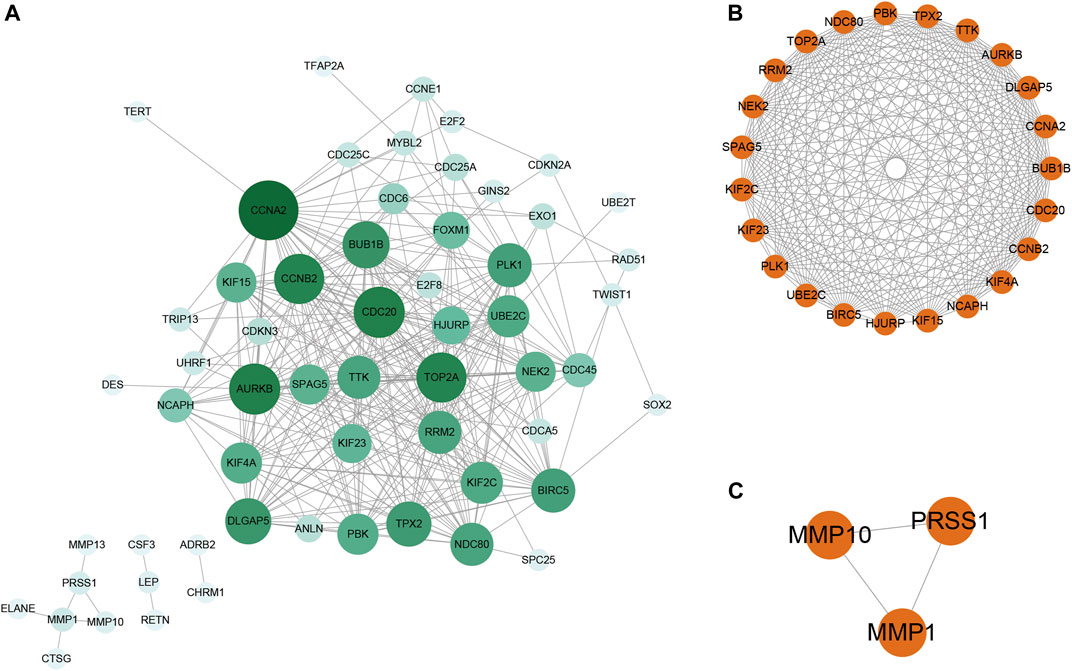
FIGURE 5. PPI network analysis and sub-network analysis based on identified COVID-19/NSCLC interactional genes. (A) PPI network containing 59 nodes and 347 edges. (B) Biggest sub-network of the PPI network. (C) Small sub-network of the PPI network. Note: nodes represent interactional genes, and edges represent interaction relationships. The depth of the color of the node is positively correlated with the degree value in panel (A).
To further understand the main systemic biological significance of the network, a sub-network analysis based on nodes of the whole network was conducted. With the recommended parameters in the molecular complex detection (MCODE) module (degree cutoff: 2, node score cutoff: 0.2, K-Core: 2, and maximum depth = 100), two sub-networks were extracted from the primary one (Figures 5B,C). Based on the biggest sub-network containing 23 nodes and 232 edges (Figure 5B), 283 GO terms (BP: 235; CC: 35; and MF: 13) and 12 KEGG pathways were highlighted (Supplementary File S5). The top 10 terms of each GO and all the KEGG pathways are shown in Figures 6A–D. Typical BP terms mainly involved mitotic spindle organization, organelle fission, and chromosome segregation; typical CC terms mainly involved chromosomal region, midbody, and spindle; typical MF terms mainly involved protein threonine kinase activity, microtubule binding, and kinase regulator activity. In addition, the main KEGG pathways involved cell cycle, viral carcinogenesis, p53 signaling pathway, and cellular senescence. By comparing the KEGG pathways from the whole PPI network and the main sub-network, functions of COVID-19/NSCLC interactional genes in cell cycle, cellular senescence, and p53 signaling pathway were underlined.
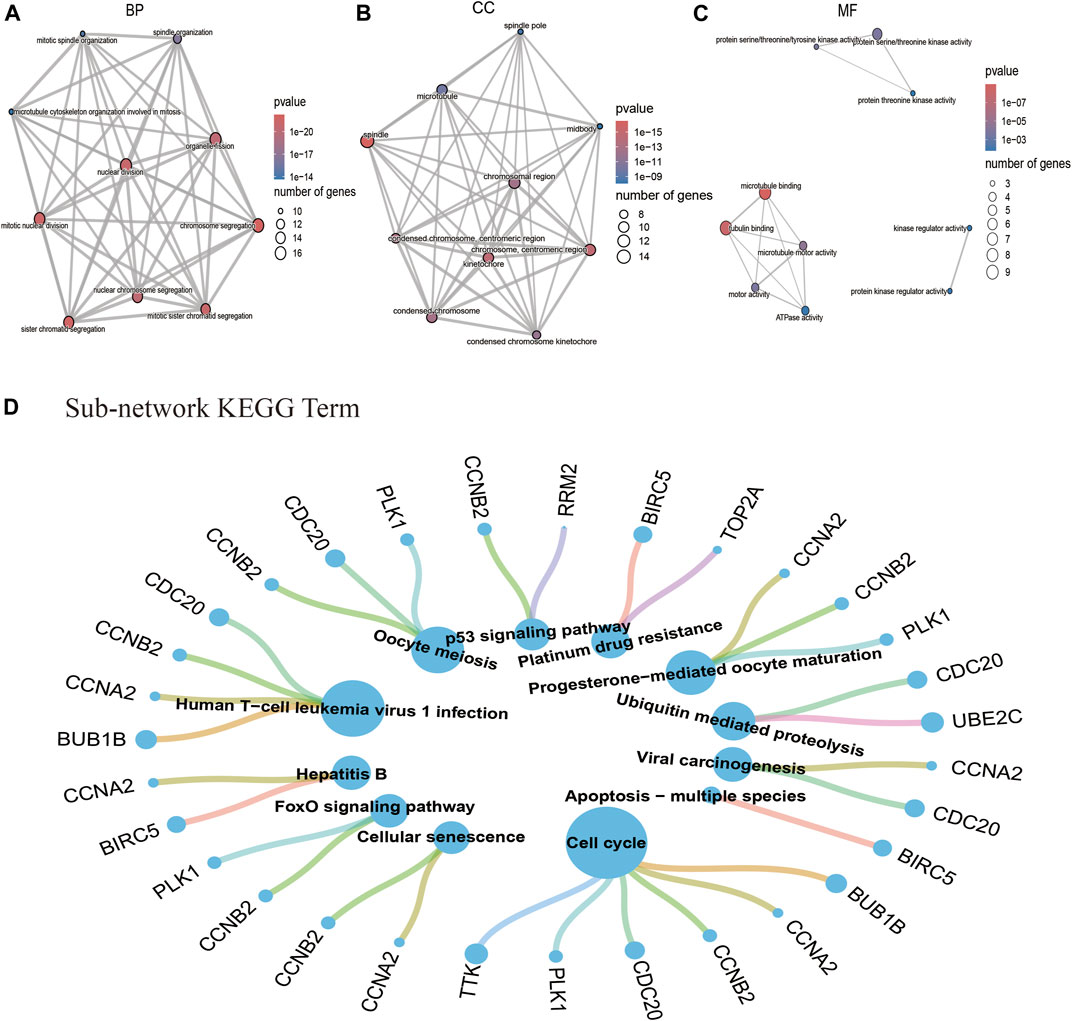
FIGURE 6. GO and KEGG pathway analysis based on the interactional genes in the biggest sub-network. (A) Top 10 BP terms of GO analysis. (B) Top 10 CC terms of GO analysis. (C)Top 10 MF terms of GO analysis. (D) Twelve pathway terms of KEGG analysis. Note: nodes represent genes or pathways, and edges represent enrichment relationships. The size of term nodes is positively correlated with the number of enriched genes. The size of gene nodes is positively correlated with the number of enriched terms.
Determination of Regulatory Signatures
A network-based approach was employed to identify regulatory TFs and miRNAs and to have a glimpse of changes happening at the transcriptional level. A total of 1,632 TFs were filtered from the ChEA3 database and sorted by the MeanRank method for the most robust result (Supplementary File S6). The data source of TFs of each COVID-19/NSCLC interactional hub gene is shown in Figure 7A. The top 10 TFs of the interactional hub gene recommended by the ChEA3 database were retrieved for subsequent analyses (Figure 7B). Next, a TF–gene interaction network containing 30 nodes and 186 edges was constructed (Figure 7C). Centromere protein A (CENPA), DNA methyltransferase 1 (DNMT1), MYB proto-oncogene-like 2 (MYBL2), transcription factor Dp-1 (TFDP1), and zinc finger protein 367 (ZNF367) were identified as the most influential regulatory factors since they targeted all interactional hub genes.
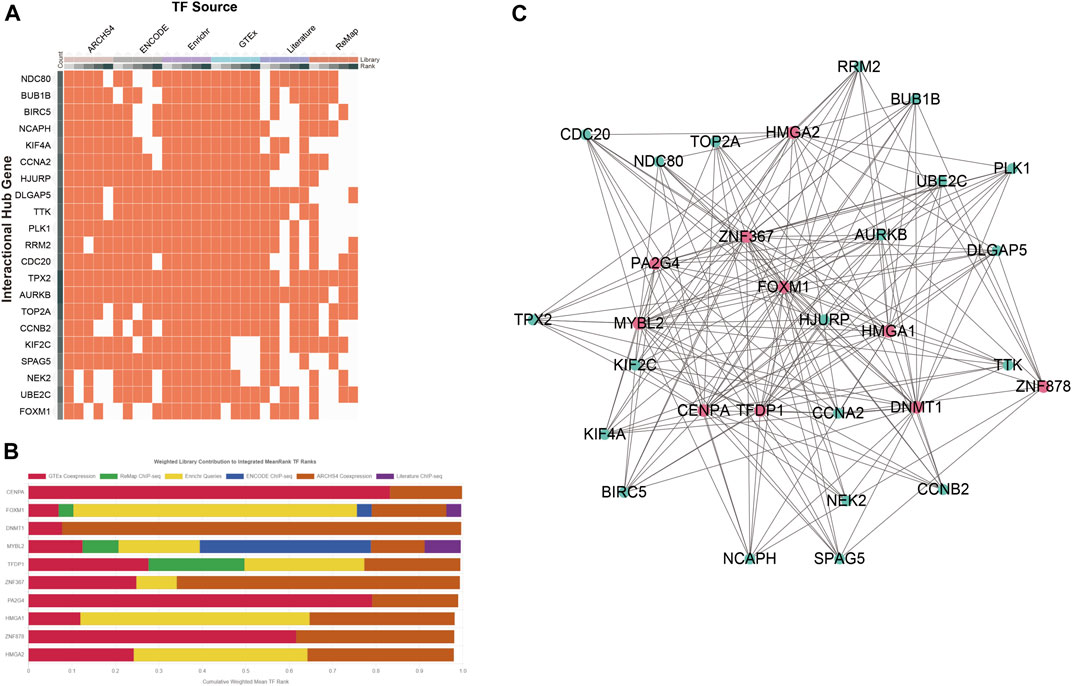
FIGURE 7. Determination of regulatory signatures (TFs). (A) Data source of TFs of the COVID-19/NSCLC interactional hub gene. (B) Top 10 TFs identified by the ChEA3 database. (C) TFs–interactional hub genes interaction network. Note: red nodes represent TFs, green nodes represent genes, and edges represent interaction relationships in panel (C).
Furthermore, we obtained 17 and 40 miRNAs from Tarbase and mirTarbase, respectively (Supplementary File S7). After removing duplicated outcomes, a total of 44 miRNAs engaged with interactional hub genes of COVID-19 and NSCLC were identified. A miRNA–gene interaction network comprising 53 nodes and 51 edges was built (Figure 8). Representative miRNAs included hsa-miR-24-3p (targeting at four interactional hub genes), hsa-miR-16-5p (targeting at two interactional hub genes), hsa-let-7a-5p (targeting at two interactional hub genes), hsa-miR-34a-5p (targeting at two interactional hub genes), and hsa-miR-10b-3p (targeting at two interactional hub genes). Taken together, these two networks indicated that 10 important TFs and 44 post-transcriptional regulatory signatures (miRNAs) provided evidence for exploring regulatory mechanisms of COVID-19 and NSCLC by participating in the regulation of interactional hub genes.
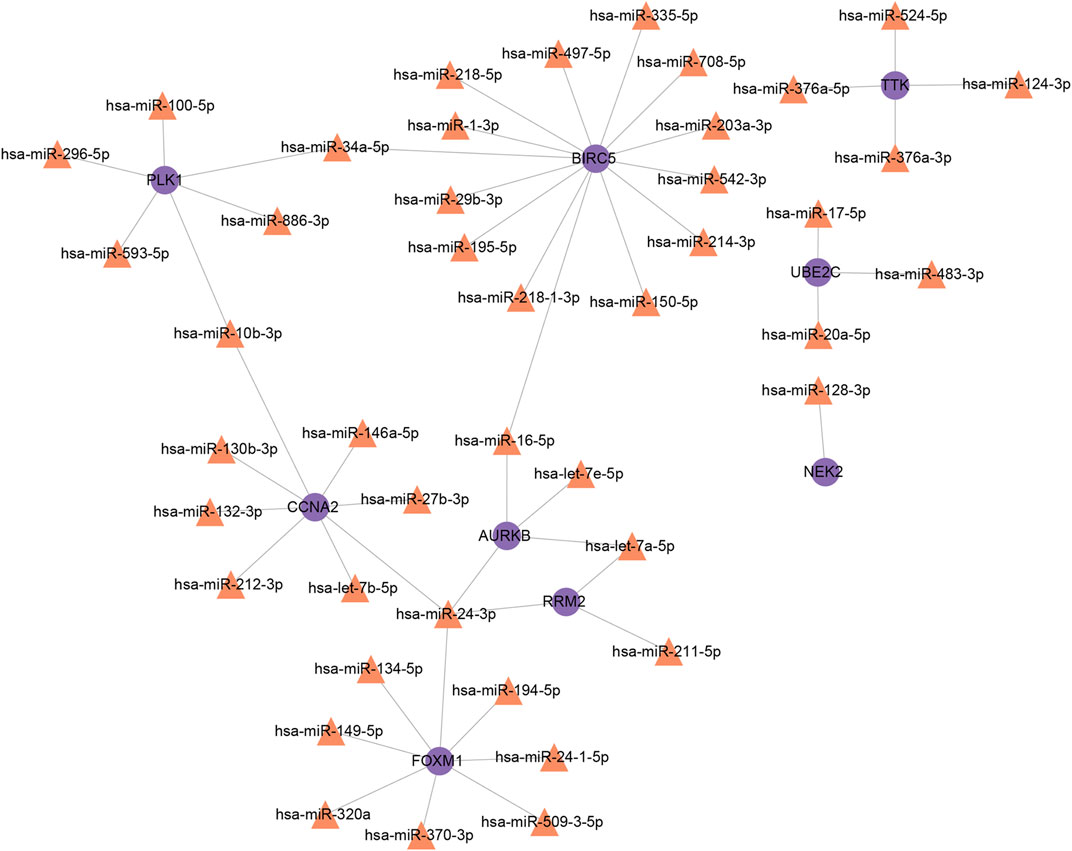
FIGURE 8. MiRNAs–interactional hub genes interaction network. Note: purple nodes represent genes, orange nodes represent MiRNAs, and edges represent the interactions between nodes.
Identification of Candidate Drugs for COVID-19 and Non-Small-Cell Lung Cancer
To identify prospective agents for COVID-19 and NSCLC, we selected potential candidates from the Enrichr database, including DSigDB, DPFG, and ShinyGO v0.75. Studies of drug molecules from these three sources were retrieved from the PubMed database for screening drugs with anticancer and/or antivirus effect (Supplementary Table S1). According to the result, the number of drug molecules obtained from DSigDB, DPFG, and ShinyGO v0.75 was 15, 10, and 3, respectively. A total of 23 candidates were identified after removing duplication. Among them, nine drugs have already been used in NSCLC, while six drugs have been registered to clinical trials for COVID-19. Resveratrol was identified both in DSigDB and DPFG, lucanthone was identified both in DSigDB and ShinyGO v0.75, and vemurafenib was identified both in DPFG and ShinyGO v0.75. Notably, natural small molecules included resveratrol and quercetin worth focus because of their binding affinity with molecular ACE-2.
Discussion
The present outbreak of SARS-CoV-2 has spread to 224 countries, with more than 250 million people infected (Worldometer, 2021). The elderly and those with pre-existing complications or smoking-related lung damage are susceptible to COVID-19, while they are precisely the profile of lung cancer patients (Berlin et al., 2020; Liao et al., 2020). The emergence of COVID-19 has resulted in detrimental alterations including (epi-)genetic alterations and changes at the transcriptional level and related signaling pathways, which have changed lung cancer treatment provision (Alnajeebi et al., 2021). Various candidate drugs and vaccines are being studied for the treatment of COVID-19; nevertheless, no therapy has shown a specific effect (Majumder and Minko, 2021). Therefore, in this study, we conducted a comprehensive systemic biological and bioinformatic analysis to identify potential interactional biomarkers that might help understand co-pathogenic factors of these two diseases and provide clues for the treatment of COVID-19 and NSCLC.
Identified Related Genes Indicated Genetic Interrelationships of COVID-19 and Non-Small-Cell Lung Cancer
Bioinformatic and systemic biological analysis can integrate data of gene expressions and protein interactions, analyze gene-regulatory pathways, and construct protein–protein networks, which is an effective tool for molecular mechanisms (Durmuş et al., 2015; Ahmed, 2020) and drug development (van Driel and Brunner, 2006), especially in the field of cancer treatment (Lee et al., 2017; Wooller et al., 2017) and infectious diseases (Josset et al., 2010; Li J. et al., 2016). NSCLC is a genomic disease which involves a loss of control over vital cellular functions and could be deteriorated by COVID-19 infection due to differential expressions of multiple host genes (Ahmed, 2020). Biomarker identification is helpful in managing NSCLC or COVID-19 (Samprathi and Jayashree, 2020; Wang et al., 2021). Inherent cancer-related changes in NSCLC genome and the aberrant expression of host factors caused by COVID-19 infection could interact with each other and impact the prognosis of patients (Passaro et al., 2021). In this study, we identified 122 COVID-19/NSCLC interactional genes through bioinformatic and systemic biological approach, which would pave the way to reveal common pathophysiological mechanisms shared by COVID-19 and NSCLC (Ahmed, 2020; Samprathi and Jayashree, 2020; Wang et al., 2021).
Gene Ontology and Kyoto Encyclopedia of Genes and Genomes Analyses Highlighted Systemic Biological Significance
To elucidate the roles of the identified 122 COVID-19/NSCLC interactional genes, GO and KEGG pathway analyses were conducted. In GO analysis, biological processes were mostly enriched in sister chromatid segregation, regulation of cell aging, spindle organization, and mitotic nuclear division. Dysregulated cell–cycle machinery, uncontrolled proliferation, and resistance to programmed cell death feature cancers, including NSCLC (Sherr, 1996; Hanahan and Weinberg, 2000; Smolle et al., 2019). Due to the paucity of well-proven therapies or vaccines, inhibiting cell division may be a good way to control COVID-19, which shares a similar goal with NSCLC treatment (Borcherding et al., 2020). Some of interactional genes participated in the BP have been found to be closely associated with the development and prognosis of NSCLC and COVID-19. For example, aurora kinase B (AURKB) has been found among the DEGs of SARS-CoV-2 in Caco-2 cells (Bock and Ortea, 2020). In addition, an in vitro experiment suggested that AURKB inhibition caused cell cycle arrest and polyploidy, followed by extensive cell death in the NSCLC model (Bertran-Alamillo et al., 2019). During SARS-CoV-2 infection, the PLK1 gene and its translational products performed significant tasks in the normal cell division cycle, promoting the spread of virus (Chen et al., 2021). The inhibition of PLK1 could alter the immune microenvironment of NSCLC by increasing dendritic cell maturation and enriching T-cell infiltration (Zhou et al., 2021).
KEGG links a group of genes in the genome with the higher-order functional information via computerizing current knowledge about cellular processes and standardizing gene annotations (Kanehisa and Goto, 2000). Based on KEGG pathway analysis, we found pathways shared by COVID-19 and NSCLC, including cell cycle, celluar senescence, IL-17 signaling pathway, chemical carcinogenesis-receptor activation, p53 signaling pathway, and Janus kinase/signal transducer and activator of the transcription (JAK-STAT) signaling pathway. Previous studies suggested that JAK-STAT, IL-17, and p53 signaling pathways were not only closely associated with the tumorigenesis of NSCLC via regulating cell proliferation, differentiation, and apoptosis but also involved in the promotion of cytokine storm which deteriorated conditions in COVID-19 patients (Shibabaw, 2020; Hu H. et al., 2021; Satarker et al., 2021). Regulated by interferon (IFN) signaling, the endocytosis of SARS-CoV-2 in lung epithelial cells is initiated by the ACE2 receptor. A cross-sectional study showed that in severe COVID-19 patients, dysregulated type I/III IFNs and JAK/STAT signaling caused impaired antiviral responses (Hadjadj et al., 2020). During the development of COVID-19, SARS-CoV-2 infection induced an excessive immune response and released a variety of pro-inflammatory cytokines through the JAK/STAT pathway, such as IL-2, IL-6, and granulocyte colony-stimulating factor signaling (Solimani et al., 2021). In addition, high levels of pro-inflammatory cytokines and chemokines accelerated disease progression, thus becoming the main risk factor of cellular senescence and age-related diseases (Bruunsgaard et al., 2003; Hu X. et al., 2021). Previous studies showed that the severity of COVID-19 and NSCLC was closely associated with hyperinflammation that could drive lung and multiorgan injury and increase mortality via cytokine storm and sepsis (Gomes et al., 2014; Tay et al., 2020). Thus, targeting inflammation-related signaling pathways may be a good option for the treatment of COVID-19 and NSCLC.
Among the interactional genes involved in the aforementioned pathways, cyclin A2 (CCNA2) and cyclin B2 (CCNB2) are members of the cyclin protein family, which play critical roles in controlling cell cycle, cell senescence, and viral infection (Nam and van Deursen, 2014). Overexpressed CCNA2 and CCNB2 are correlated with a poor prognosis in NSCLC (Cooper et al., 2009; Takashima et al., 2014; Qian et al., 2015). Meanwhile, during SARS-CoV-2 infection, the increasing level of CCNA2 not only provides favorable conditions for virus transmission but also hints at chromosomal abnormalities and other genetic material damage in the host cells (Chen et al., 2021). Cellular senescence, an irreversible state of cell cycle arrest in response to damaging stimuli, secretes factors known as senescence-associated secretory phenotype or SASP (González-Gualda et al., 2021; Kumari and Jat, 2021). In this case, cells maintain active metabolism without responses of mitogenic and apoptotic signals (González-Gualda et al., 2021). In the elderly or patients upon consistent and chronic damages, cellular senescence may impair regenerative ability and accelerate the progression of inflammation and lung cancer (Hernandez-Segura et al., 2018; Kuźnar-Kamińska et al., 2018). In lung cancer tumorigenesis, senescent cells produced soluble signaling factors [including interleukins (Rabinovich et al., 2007), chemokines (Coppé et al., 2010), and growth factors (Yang et al., 2006)], proteases (Finkel et al., 2007), insoluble proteins, and extracellular matrix components (Acosta et al., 2008a; Acosta et al., 2008b), which mediated cell proliferation, invasion, and migration (Wald et al., 2011; Han et al., 2015; Kuźnar-Kamińska et al., 2016). Furthermore, a strong connection between cellular senescence and SARS-CoV-2 has been found, as cellular senescence increased the risk of developing severe COVID-19 (Nehme et al., 2020). SARS-CoV-2 infection could induce paracrine senescence by increasing IFN secretion in infected cells, and danger-associated molecular patterns released in cells undergoing pyroptosis or necroptosis further accelerated senescence in the environment via SASP factors (Kim et al., 2009; Acosta et al., 2013; Kandhaya-Pillai et al., 2017; Nehme et al., 2020). Cellular senescence, in turn, 1) led to a weak adaptive immunity by increasing senescent-like T cells and B lymphocytes (Frasca et al., 2017; De Biasi et al., 2020); 2) enhanced aberrant healing response and tissue fibrosis in the respiratory system (Mason, 2020); 3) resulted in vascular dysfunction via decreasing angiogenesis and increasing thrombosis and inflammatory responses in COVID-19 patients (Ungvari et al., 2018; Escher et al., 2020). Dysregulation of the cell cycle and cellular senescence has been observed in both COVID-19 and lung cancer. Thus, the importance of understanding the homeostasis maintenance and pathological alteration of these biological processes should be emphasized.
In summary, the aforementioned genes and pathways are commonly and significantly altered in both COVID-19 and NSCLC, preparing the ground for pathophysiological studies and drug development.
Protein–Protein Interaction and Sub-Network Analysis Explored In-Depth Interrelationships of Interactional Hub Genes
On the basis of interactional genes, we built a PPI network presenting in-depth biological characteristics. In the biggest sub-network, CCNA2, CCNB2, AURKB, DNA topoisomerase II alpha (TOP2A), and baculoviral IAP repeat containing 5 (BIRC5) were closely connected with other genes. TOP2A, a target for cytotoxic drugs (etoposide, anthracyclines) and a key regulator of chromosome condensation and chromatid separation, prevents DNA replication and transcription (Nicoś et al., 2021). It is generally believed that TOP2A is a prognostic indicator of NSCLC, and its level is negatively correlated with the prognosis (Hou et al., 2017; Ma et al., 2019). In consistence with the result of our study, TOP2A has been identified as a hub gene that could govern many cellular processes by protein–protein interactions in COVID-19 patients (Hasan et al., 2022).
Comparing to the result of KEGG analysis based on 122 interactional genes, the biggest sub-network identified additional pathways, such as forkhead box, sub-group O (FoxO) signaling pathway, ubiquitin-mediated proteolysis, viral carcinogenesis, and apoptosis. As tumor suppressors, FoxO proteins increased the expression level of death receptor ligands such as Fas ligand and tumor necrosis factor (TNF) apoptosis ligand, engaged with pro-apoptotic pathways, and blocked cell cycle progression (Farhan et al., 2017). According to the result, viral carcinogenesis was one of the most important KEGG pathways, indicating that co-pathogenic factors shared by COVID-19 and NSCLC could be the basis of finding potential drugs for synergistic treatment.
Interaction of Transcription Factors and MicroRNAs With Interactional Hub Genes
Genetic alterations have long been blamed for malignancy but cannot fully explain tumor development. Epigenetic dysregulations give new insights into how heritable changes in gene expression happen without involving changes in nucleotide sequence, and finally promote carcinogenesis (Yuan et al., 2016). MiRNAs and TFs are the largest families of trans-acting gene regulatory species and pivotal players in a complex regulatory network (Sharma et al., 2020). Herein, we investigated TF–gene and miRNA–genes interaction that might help in learning more about disease development.
Transcription factors are key cellular components that regulate multiple genes over a long distance by maintaining proliferation status, driving cellular differentiation, and determining cell fate (Essebier et al., 2017). The identified top 10 TFs included CENPA, DNMT1, MYBL2, TFDP1, ZNF367, high mobility group AT-hook 1 (HMGA1), proliferation-associated 2G4 (PA2G4), high mobility group AT-hook 2 (HMGA2), zinc finger protein 878 (ZNF878), and FOXM1. Among them, CENPA (Mullen et al., 2020), DNMT1 (Wu et al., 2020), MYBL2 (Mullen et al., 2020), TFDP1 (Zhan et al., 2017), ZNF367 (Liu Z. et al., 2018), HMGA1 (Zhang Z. et al., 2015), HMGA2 (Gao et al., 2018), and FOXM1 (Mullen et al., 2020) were previously shown to be highly associated with NSCLC progression. It has been demonstrated that CENPA, MYBL2, and FOXM1 were linked to numerous cancer-specific enhancers, and their elevated expression levels were associated with a poor survival rate of NSCLC patients (Mullen et al., 2020). Meanwhile, the level of DNMT1 was found significantly downregulated in SARS-COV-2–infected epithelial cells (Muhammad et al., 2021). SARS-COV-2 infection mainly affects the molecular mechanisms of aging centered on HMGA1 and HMGA2 proteins, and their interactions may impair or trigger inflammatory pathways, leading to various responses in different age groups (Mercatelli et al., 2021). To conclude, DNMT1, HMGA1, and HMGA2 might be central TFs in the TF–gene regulatory network in COVID-19/NSCLC.
MiRNAs are endogenous small non-coding RNA molecules that can regulate the expression of non-coding sequences and genes involved in oncogenesis (Liu et al., 2014). Various studies have identified miRNAs as key players in the pathogenesis and therapeutics of viral diseases. Moreover, as part of host–pathogen interactions, miRNA can scan target SARS-CoV-2 genes as well as host inflammatory machinery to counter-act the impairing effects of infection (Ghosh et al., 2009; Khokhar et al., 2022). The top 10 most significant miRNAs mainly involved in respiratory diseases (Table 4), including NSCLC [hsa-miR-24-3p (Wei et al., 2021), hsa-miR-34a-5p (Zhou X. et al., 2020), hsa-miR-10b-3p (Liu et al., 2017), hsa-miR-20a-5p, and hsa-miR-17-5p (Leidinger et al., 2016)], idiopathic pulmonary fibrosis [hsa-miR-524-5p (Li Q. et al., 2020), COVID-19 (hsa-miR-20a-5p, hsa-miR-17-5p (Li C. et al., 2020)), and hsa-miR-16-5p (Kim et al., 2020)], pneumoconiosis [hsa-let-7a-5p (Zhang et al., 2018)], and asthma [(hsa-let-7a-5p (Huang et al., 2019)]. Interestingly, compared with the healthy controls, the levels of hsa-miR-20a-5p and hsa-miR-17-5p were significantly downregulated in patients with COVID-19; therefore, they were considered as essential modulators of viral replication (Li C. et al., 2020). In addition, according to a study based on high-throughput qRT-PCR validation, hsa-miR-20a-5p and hsa-miR-17-5p were the top markers that could distinguish NSCLC patients from unaffected controls with 94.5% accuracy (Leidinger et al., 2016).
Notably, hsa-miR-24-3p, hsa-miR-16-5p, and hsa-let-7a-5p targeted AURKB, central regulator of cell division (Bertran-Alamillo et al., 2019), indicating that these miRNAs may be potential targets to control cell cycle and cellular senescence. In addition, miR-34a-5p regulated the G1/S checkpoint in NSCLC cells (Gupta et al., 2020). Forced expression of miR-34a-5p enhanced p21 expression and promoted cellular senescence, whereas downregulated miR-34a-5p decreased senescence and increased apoptosis by targeting B-cell lymphoma-2 (BCL2), myelocytomatosis oncogene (MYC), mesenchymal-epithelial transition (MET), and p53 (Gallardo et al., 2009; Kasinski and Slack, 2012). According to the result, BIRC5, FOXM1, CCNA2, and PLK1 showed a higher degree of interaction with miRNAs, which may play crucial roles in the interaction networks.
To sum up, TF–gene intereactions are reactors that regulate gene expression by binding with target genes and miRNAs (Zhang HM. et al., 2015). The constructed TF–gene and miRNA–gene interaction networks help further understand the direct regulatory relationship of miRNA and TFs in NSCLC and COVID-19, while interactional hub genes may be crucial biomarkers and therapeutic targets.
Potential Drugs Provided Possible Treatments for COVID-19 and Non-Small-Cell Lung Cancer
In this study, a total of 23 candidates containing 18 drugs and 5 natural compounds were identified. Among them, nine drugs have already been administered to NSCLC patients, including irinotecan (Murakami et al., 2018), dasatinib (Xu et al., 2015), 5-fluorouracil (Nokihara et al., 2017), etoposide (Liang et al., 2017), carboplatin (Hasegawa et al., 2020), ascorbic acid (Pathak et al., 2005), azacitidine (Cheng et al., 2021), decitabine (Chu et al., 2013), and palbociclib (Nie et al., 2019). According to the ClinicalTrials database (https://clinicaltrials.gov/), cyclosporin A (NCT04540926), dasatinib (NCT04830735), quercetin (NCT04851821), etoposide (NCT04356690), ascorbic acid (NCT04401150), and decitabine (NCT04482621) have been registered to clinical trials for evaluating their efficacy in COVID-19 patients. Therefore, it can be inferred that dasatinib, etoposide, ascorbic acid, and decitabine are likely to be the promising agents for NSCLC patients with COVID-19, which provides support for our predictions.
Quercetin and resveratrol are two natural compounds which have both antiviral and anti-NSCLC effects (Zhao et al., 2010; Wu et al., 2015; Lin et al., 2017; Chai et al., 2021). Quercetin, a well-known natural polyphenol with anti-inflammatory, antioxidant, and immunomodulatory properties, is involved in a variety of diseases, such as viral infections, respiratory diseases, allergies, asthma, and cancer (Li Y. et al., 2016; Xu et al., 2019). Previous network pharmacology and molecular docking studies anticipated that quercetin could interfere with SARS-CoV-2 replication by interacting with 3-chymotrypsin-like protease (3CLpro), papain-like protease (PLpro), and S proteins (Derosa et al., 2021; DI Pierro et al., 2021b). An open-label, randomized controlled trial included 152 outpatients with confirmed SARS-CoV-2 infection but without severe COVID-19 symptoms found that formulated quercetin treatments reduced the frequency and length of hospitalization, the need of non-invasive oxygen therapy, and the number of deaths (DI Pierro et al., 2021a). In addition, available experimental studies suggested that quercetin could directly modulate multiple lung cancer-relevant miRNAs and DNA methylation (Kim DH. et al., 2019; Kedhari Sundaram et al., 2019). Another ingredient resveratrol is a potent antioxidant that could inhibit platelet aggregation and vasodilation, reduce blood viscosity, and maintain blood flow (Olas and Wachowicz, 2005; de la Lastra and Villegas, 2007). In vitro and in vivo studies indicated that resveratrol induced cell apoptosis and inhibited proliferation, growth, and metastasis in NSCLC (Yousef et al., 2017; Tang et al., 2020). Given the thrombin-inhibitory and anti-inflammatory effects, resveratrol deserves further study for the treatment of COVID-19 and NSCLC.
It is noteworthy that a computational structure-based study identified trichostatin A as a potential SARS-CoV-2 Mpro inhibitor. The result has been further validated by an essay which has suggested that trichostatin A could reduce the viral RNA load, viral antigen expression, and infectious virus particle formation (Wen et al., 2021). Other identified ingredients also had an anti-respiratory virus effect. For instance, cyclosporine at non-cytotoxic concentrations could induce a strong inhibition of the replication of specific coronaviruses in vitro, including SARS-CoV, MERS-CoV, and human coronavirus 229E (HCoV-229E) (Poulsen et al., 2020). A retrospective observational study recently found that in COVID-19 hospitalized patients, cyclosporine was significantly associated with a decrease in mortality, probably due to its combined activity of immunosuppression and antiviral activity (Guisado-Vasco et al., 2020). Dyall et al. (2014) found that dasatinib was active against both MERS-CoV and SARS-CoV in vitro, and it might minimize immunotoxicity as it blocked viral replication. Vemurafenib interfered the cellular Raf/MEK/ERK signaling cascade by binding to the ATP-binding site of BRAF (V600E) kinase and inhibiting its function (Pleschka et al., 2001). Interestingly, since Raf/MEK/ERK signaling pathways mediated the increasing SARS-CoV-1 replication (Dyall et al., 2014), it may be a therapeutic target for host-directed SARS-CoV-2 antivirals. Thus, vemurafenib may also be a potential anti-COVID-19 drug. To conclude, according to available literature and the result of our study, identified molecules may be prospective agents in the treatment of COVID-19 and NSCLC.
Based on the comprehensive bioinformatic and systemic biological analysis, the present study carried out a study framework to reveal interaction networks and therapeutic implications for NSCLC patients with COVID-19, the findings of which would provide evidence and shed light for the further research on COVID-19/NSCLC. However, there were still some limitations in the present study. First, the sample size of certain disease studies might be insufficient to capture all of the critical disease-related genes for identifying the common DEGs. Second, incompleteness of available interactome data and limitation of computational methods might make the conclusions less dependable and accurate. Finally, the results of the present study were derived from multiple computational approaches, and future in vivo and in vitro experiments are required to fully assess the biological relevance of candidates.
Conclusion
In summary, based on bioinformatic analyses, we predicted promising therapeutic ingredients and drugs for NSCLC patients with COVID-19. We detected interactional hub genes enriched in regulating biological processes and signaling pathways, which were mainly relevant to cell division, cell aging, cell cycle, and cellular senescence. This study has identified 1) potential therapeutic targets, including CCNA2, CCNB2, AURKB, TOP2A, and BIRC5; 2) signaling pathways primarily related to cell cycle, cell aging, viral carcinogenesis, and p53 signaling pathway; 3) potential agents for the treatment of COVID-19 and NSCLC, including quercetin, resveratrol, cyclosporine, dasatinib, etoposide, ascorbic acid, and decitabine. Future experimental and clinical studies should be carried out with predicted agents to explore pharmacological mechanisms and to inform possible interventions for COVID-19 and NSCLC.
Data Availability Statement
The datasets presented in this study can be found in online repositories. The names of the repository/repositories and accession number(s) can be found in the article/Supplementary Material.
Author Contributions
ZL and ZZ conceived and designed the study. ZZ and QC performed data analysis and data interpretation. ZZ, QC, and XZ conducted the bioinformatic and statistical analyses. ZZ and QC plotted the figures. QC, XZ, and HC prepared the manuscript.
Funding
This work was supported by the “Double First-Class” and High-level University Discipline Collaborative Innovation Team Project of Guangzhou University of Chinese Medicine (Grant No. 2021xk23).
Conflict of Interest
The authors declare that the research was conducted in the absence of any commercial or financial relationships that could be construed as a potential conflict of interest.
Publisher’s Note
All claims expressed in this article are solely those of the authors and do not necessarily represent those of their affiliated organizations, or those of the publisher, the editors, and the reviewers. Any product that may be evaluated in this article, or claim that may be made by its manufacturer, is not guaranteed or endorsed by the publisher.
Supplementary Material
The Supplementary Material for this article can be found online at: https://www.frontiersin.org/articles/10.3389/fphar.2022.857730/full#supplementary-material
Supplementary File S1 | COVID-19–related genes obtained from public databases.
Supplementary File S2 | COVID-19–related DEGs obtained from the RNA-seq dataset.
Supplementary File S3 | NSCLC-related genes obtained from public databases.
Supplementary File S4 | DEGs of NSCLC obtained from TCGA database.
Supplementary File S5 | GO and KEGG terms based on the biggest sub-network with the associated statistics.
Supplementary File S6 | TFs filtered from the ChEA3 database.
Supplementary File S7 | MiRNAs obtained from Tarbase and mirTarbase.
Supplementary Table S1 | Antiviral and/or anticancer activities of candidate drugs for COVID-19/NSCLC from Enrichr database and ShinyGO v0.75 database.
References
Acosta, J. C., Banito, A., Wuestefeld, T., Georgilis, A., Janich, P., Morton, J. P., et al. (2013). A Complex Secretory Program Orchestrated by the Inflammasome Controls Paracrine Senescence. Nat. Cel. Biol. 15 (8), 978–990. doi:10.1038/ncb2784
Acosta, J. C., O'Loghlen, A., Banito, A., Guijarro, M. V., Augert, A., Raguz, S., et al. (2008a). Chemokine Signaling via the CXCR2 Receptor Reinforces Senescence. Cell 133 (6), 1006–1018. doi:10.1016/j.cell.2008.03.038
Acosta, J. C., O'Loghlen, A., Banito, A., Raguz, S., and Gil, J. (2008b). Control of Senescence by CXCR2 and its Ligands. Cell Cycle 7 (19), 2956–2959. doi:10.4161/cc.7.19.6780
Afrasiabi, E., Ahlgren, J., Bergelin, N., and Törnquist, K. (2008). Phorbol 12-Myristate 13-Acetate Inhibits FRO Anaplastic Human Thyroid Cancer Cell Proliferation by Inducing Cell Cycle Arrest in G1/S Phase: Evidence for an Effect Mediated by PKCdelta. Mol. Cel. Endocrinol. 292 (1-2), 26–35. doi:10.1016/j.mce.2008.04.018
Ahmed, F. (2020). A Network-Based Analysis Reveals the Mechanism Underlying Vitamin D in Suppressing Cytokine Storm and Virus in SARS-CoV-2 Infection. Front. Immunol. 11, 590459. doi:10.3389/fimmu.2020.590459
Alnajeebi, A. M., Alharbi, H. F. H., Alelwani, W., Babteen, N. A., Alansari, W., Shamlan, G., et al. (2021). COVID-19 Candidate Genes and Pathways Potentially Share the Association with Lung Cancer. Cchts 25, 1–10. doi:10.2174/1386207324666210712092649
Argenta, D. F., Silva, I. T., Bassani, V. L., Koester, L. S., Teixeira, H. F., and Simões, C. M. (2015). Antiherpes Evaluation of Soybean Isoflavonoids. Arch. Virol. 160 (9), 2335–2342. doi:10.1007/s00705-015-2514-z
Bader, G. D., and Hogue, C. W. (2003). An Automated Method for Finding Molecular Complexes in Large Protein Interaction Networks. BMC Bioinformatics 4, 2. doi:10.1186/1471-2105-4-2
Badia, R., Angulo, G., Riveira-Muñoz, E., Pujantell, M., Puig, T., Ramirez, C., et al. (2016). Inhibition of Herpes Simplex Virus Type 1 by the CDK6 Inhibitor PD-0332991 (Palbociclib) through the Control of SAMHD1. J. Antimicrob. Chemother. 71 (2), 387–394. doi:10.1093/jac/dkv363
Beach, L. B., Rawson, J. M., Kim, B., Patterson, S. E., and Mansky, L. M. (2014). Novel Inhibitors of Human Immunodeficiency Virus Type 2 Infectivity. J. Gen. Virol. 95 (Pt 12), 2778–2783. doi:10.1099/vir.0.069864-0
Bergmann, S., and Elbahesh, H. (2019). Targeting the Proviral Host Kinase, FAK, Limits Influenza a Virus Pathogenesis and NFkB-Regulated Pro-Inflammatory Responses. Virology 534, 54–63. doi:10.1016/j.virol.2019.05.020
Berlin, I., Thomas, D., Le Faou, A. L., and Cornuz, J. (2020). COVID-19 and Smoking. Nicotine Tob. Res. 22 (9), 1650–1652. doi:10.1093/ntr/ntaa059
Bertran-Alamillo, J., Cattan, V., Schoumacher, M., Codony-Servat, J., Giménez-Capitán, A., Cantero, F., et al. (2019). AURKB as a Target in Non-Small Cell Lung Cancer with Acquired Resistance to Anti-EGFR Therapy. Nat. Commun. 10 (1), 1812. doi:10.1038/s41467-019-09734-5
Bochenek, M. L., Schütz, E., and Schäfer, K. (2016). Endothelial Cell Senescence and Thrombosis: Ageing Clots. Thromb. Res. 147, 36–45. doi:10.1016/j.thromres.2016.09.019
Bock, J. O., and Ortea, I. (2020). Re-analysis of SARS-CoV-2-Infected Host Cell Proteomics Time-Course Data by Impact Pathway Analysis and Network Analysis: A Potential Link with Inflammatory Response. Aging (Albany NY) 12 (12), 11277–11286. doi:10.18632/aging.103524
Borcherding, N., Jethava, Y., and Vikas, P. (2020). Repurposing Anti-Cancer Drugs for COVID-19 Treatment. Drug Des. Devel. Ther. 14, 5045–5058. doi:10.2147/dddt.S282252
Bruunsgaard, H., Andersen-Ranberg, K., Hjelmborg, Jv., Pedersen, B. K., and Jeune, B. (2003). Elevated Levels of Tumor Necrosis Factor Alpha and Mortality in Centenarians. Am. J. Med. 115 (4), 278–283. doi:10.1016/s0002-9343(03)00329-2
Carew, J. S., Espitia, C. M., Esquivel, J. A., Mahalingam, D., Kelly, K. R., Reddy, G., et al. (2011). Lucanthone Is a Novel Inhibitor of Autophagy that Induces Cathepsin D-Mediated Apoptosis. J. Biol. Chem. 286 (8), 6602–6613. doi:10.1074/jbc.M110.151324
Carithers, L. J., and Moore, H. M. (2015). The Genotype-Tissue Expression (GTEx) Project. Biopreserv. Biobank 13 (5), 307–308. doi:10.1089/bio.2015.29031.hmm
Chai, R., Xu, C., Lu, L., Liu, X., and Ma, Z. (2021). Quercetin Inhibits Proliferation of and Induces Apoptosis in Non-Small-Cell Lung Carcinoma via the lncRNA SNHG7/miR-34a-5p Pathway. Immunopharmacol. Immunotoxicol. 43 (6), 693–703. doi:10.1080/08923973.2021.1966032
Chan, K. K. L., Siu, M. K. Y., Jiang, Y. X., Wang, J. J., Leung, T. H. Y., and Ngan, H. Y. S. (2018). Estrogen Receptor Modulators Genistein, Daidzein and ERB-041 Inhibit Cell Migration, Invasion, Proliferation and Sphere Formation via Modulation of FAK and PI3K/AKT Signaling in Ovarian Cancer. Cancer Cel. Int. 18, 65. doi:10.1186/s12935-018-0559-2
Chen, J.-C., Xie, T.-A., Lin, Z.-Z., Li, Y.-Q., Xie, Y.-F., Li, Z.-W., et al. (2021). Identification of Key Pathways and Genes in SARS-CoV-2 Infecting Human Intestines by Bioinformatics Analysis. Biochem. Genet. 1–19. doi:10.1007/s10528-021-10144-w
Chèneby, J., Ménétrier, Z., Mestdagh, M., Rosnet, T., Douida, A., Rhalloussi, W., et al. (2020). ReMap 2020: A Database of Regulatory Regions from an Integrative Analysis of Human and Arabidopsis DNA-Binding Sequencing Experiments. Nucleic Acids Res. 48 (D1), D180–d188. doi:10.1093/nar/gkz945
Cheng, H., Zou, Y., Shah, C. D., Fan, N., Bhagat, T. D., Gucalp, R., et al. (2021). First-in-Human Study of Inhaled Azacitidine in Patients with Advanced Non-Small Cell Lung Cancer. Lung Cancer 154, 99–104. doi:10.1016/j.lungcan.2021.02.015
Chin, C. H., Chen, S. H., Wu, H. H., Ho, C. W., Ko, M. T., and Lin, C. Y. (2014). cytoHubba: Identifying Hub Objects and Sub-Networks from Complex Interactome. BMC Syst. Biol. 8 (Suppl. 4), S11. doi:10.1186/1752-0509-8-s4-s11
Chou, C. H., Shrestha, S., Yang, C. D., Chang, N. W., Lin, Y. L., Liao, K. W., et al. (2018). miRTarBase Update 2018: A Resource for Experimentally Validated microRNA-Target Interactions. Nucleic Acids Res. 46 (D1), D296–d302. doi:10.1093/nar/gkx1067
Chu, B. F., Karpenko, M. J., Liu, Z., Aimiuwu, J., Villalona-Calero, M. A., Chan, K. K., et al. (2013). Phase I Study of 5-Aza-2'-Deoxycytidine in Combination with Valproic Acid in Non-Small-Cell Lung Cancer. Cancer Chemother. Pharmacol. 71 (1), 115–121. doi:10.1007/s00280-012-1986-8
Ciotti, M., Ciccozzi, M., Terrinoni, A., Jiang, W. C., Wang, C. B., and Bernardini, S. (2020). The COVID-19 Pandemic. Crit. Rev. Clin. Lab. Sci. 57 (6), 365–388. doi:10.1080/10408363.2020.1783198
Clough, E., and Barrett, T. (2016). “The Gene Expression Omnibus Database,” in Statistical Genomics (New York, NY: Springer), 93–110. doi:10.1007/978-1-4939-3578-9_5
Colunga Biancatelli, R. M. L., Berrill, M., and Marik, P. E. (2020). The Antiviral Properties of Vitamin C. Expert Rev. Anti Infect. Ther. 18 (2), 99–101. doi:10.1080/14787210.2020.1706483
Cooper, W. A., Kohonen-Corish, M. R., McCaughan, B., Kennedy, C., Sutherland, R. L., and Lee, C. S. (2009). Expression and Prognostic Significance of Cyclin B1 and Cyclin A in Non-Small Cell Lung Cancer. Histopathology 55 (1), 28–36. doi:10.1111/j.1365-2559.2009.03331.x
Coppé, J. P., Desprez, P. Y., Krtolica, A., and Campisi, J. (2010). The Senescence-Associated Secretory Phenotype: The Dark Side of Tumor Suppression. Annu. Rev. Pathol. 5, 99–118. doi:10.1146/annurev-pathol-121808-102144
Daamen, A. R., Bachali, P., Owen, K. A., Kingsmore, K. M., Hubbard, E. L., Labonte, A. C., et al. (2021). Comprehensive Transcriptomic Analysis of COVID-19 Blood, Lung, and Airway. Sci. Rep. 11 (1), 7052. doi:10.1038/s41598-021-86002-x
Davis, A. P., Grondin, C. J., Johnson, R. J., Sciaky, D., Wiegers, J., Wiegers, T. C., et al. (2021). Comparative Toxicogenomics Database (CTD): Update 2021. Nucleic Acids Res. 49 (D1), D1138–d1143. doi:10.1093/nar/gkaa891
Davis, C. A., Hitz, B. C., Sloan, C. A., Chan, E. T., Davidson, J. M., Gabdank, I., et al. (2018). The Encyclopedia of DNA Elements (ENCODE): Data portal Update. Nucleic Acids Res. 46 (D1), D794–d801. doi:10.1093/nar/gkx1081
De Biasi, S., Meschiari, M., Gibellini, L., Bellinazzi, C., Borella, R., Fidanza, L., et al. (2020). Marked T Cell Activation, Senescence, Exhaustion and Skewing towards TH17 in Patients with COVID-19 Pneumonia. Nat. Commun. 11 (1), 3434. doi:10.1038/s41467-020-17292-4
de Carvalho Scharf Santana, N., Lima, N. A., Desoti, V. C., Bidóia, D. L., de Souza Bonfim Mendonça, P., Ratti, B. A., et al. (2016). Vitamin K3 Induces Antiproliferative Effect in Cervical Epithelial Cells Transformed by HPV 16 (SiHa Cells) through the Increase in Reactive Oxygen Species Production. Arch. Gynecol. Obstet. 294 (4), 797–804. doi:10.1007/s00404-016-4097-7
de la Lastra, C. A., and Villegas, I. (2007). Resveratrol as an Antioxidant and Pro-Oxidant Agent: Mechanisms and Clinical Implications. Biochem. Soc. Trans. 35 (Pt 5), 1156–1160. doi:10.1042/bst0351156
Derosa, G., Maffioli, P., D'Angelo, A., and Di Pierro, F. (2021). A Role for Quercetin in Coronavirus Disease 2019 (COVID-19). Phytother. Res. 35 (3), 1230–1236. doi:10.1002/ptr.6887
DI Pierro, F., Derosa, G., Maffioli, P., Bertuccioli, A., Togni, S., Riva, A., et al. (2021a). Possible Therapeutic Effects of Adjuvant Quercetin Supplementation Against Early-Stage COVID-19 Infection: A Prospective, Randomized, Controlled, and Open-Label Study. Int. J. Gen. Med. 14, 2359–2366. doi:10.2147/ijgm.S318720
DI Pierro, F., Khan, A., Bertuccioli, A., Maffioli, P., Derosa, G., Khan, B. A., et al. (2021b). Quercetin Phytosome® as a Potential Candidate for Managing COVID-19. Minerva Gastroenterol. (Torino) 67 (2), 190–195. doi:10.23736/s2724-5985.20.02771-3
Diaz-Moralli, S., Tarrado-Castellarnau, M., Miranda, A., and Cascante, M. (2013). Targeting Cell Cycle Regulation in Cancer Therapy. Pharmacol. Ther. 138 (2), 255–271. doi:10.1016/j.pharmthera.2013.01.011
Didelot, C., Mirjolet, J. F., Barberi-Heyob, M., Ramacci, C., Teiten, M. H., and Merlin, J. L. (2003). Oncoprotein Expression of E6 and E7 Does Not Prevent 5-Fluorouracil (5FU) Mediated G1/S Arrest and Apoptosis in 5FU Resistant Carcinoma Cell Lines. Int. J. Oncol. 23 (1), 81–87. doi:10.3892/ijo.23.1.81
Du, J., Cullen, J. J., and Buettner, G. R. (2012). Ascorbic Acid: Chemistry, Biology and the Treatment of Cancer. Biochim. Biophys. Acta 1826 (2), 443–457. doi:10.1016/j.bbcan.2012.06.003
Durmuş, S., Çakır, T., Özgür, A., and Guthke, R. (2015). A Review on Computational Systems Biology of Pathogen-Host Interactions. Front. Microbiol. 6, 235. doi:10.3389/fmicb.2015.00235
Dyall, J., Coleman, C. M., Hart, B. J., Venkataraman, T., Holbrook, M. R., Kindrachuk, J., et al. (2014). Repurposing of Clinically Developed Drugs for Treatment of Middle East Respiratory Syndrome Coronavirus Infection. Antimicrob. Agents Chemother. 58 (8), 4885–4893. doi:10.1128/AAC.03036-14
Dzobo, K. (2021). The Role of Viruses in Carcinogenesis and Molecular Targeting: From Infection to Being a Component of the Tumor Microenvironment. OMICS: A J. Integr. Biol. 25 (6), 358–371. doi:10.1089/omi.2021.0052
Eastman, R. T., Roth, J. S., Brimacombe, K. R., Simeonov, A., Shen, M., Patnaik, S., et al. (2020). Remdesivir: A Review of its Discovery and Development Leading to Emergency Use Authorization for Treatment of COVID-19. ACS Cent. Sci. 6 (5), 672–683. doi:10.1021/acscentsci.0c00489
Escher, R., Breakey, N., and Lämmle, B. (2020). Severe COVID-19 Infection Associated with Endothelial Activation. Thromb. Res. 190, 62. doi:10.1016/j.thromres.2020.04.014
Essebier, A., Lamprecht, M., Piper, M., and Bodén, M. (2017). Bioinformatics Approaches to Predict Target Genes from Transcription Factor Binding Data. Methods 131, 111–119. doi:10.1016/j.ymeth.2017.09.001
Farhan, M., Wang, H., Gaur, U., Little, P. J., Xu, J., and Zheng, W. (2017). FOXO Signaling Pathways as Therapeutic Targets in Cancer. Int. J. Biol. Sci. 13 (7), 815–827. doi:10.7150/ijbs.20052
Finkel, T., Serrano, M., and Blasco, M. A. (2007). The Common Biology of Cancer and Ageing. Nature 448 (7155), 767–774. doi:10.1038/nature05985
Frasca, D., Diaz, A., Romero, M., and Blomberg, B. B. (2017). Human Peripheral Late/Exhausted Memory B Cells Express a Senescent-Associated Secretory Phenotype and Preferentially Utilize Metabolic Signaling Pathways. Exp. Gerontol. 87 (Pt A), 113–120. doi:10.1016/j.exger.2016.12.001
Fujita, M., Hasegawa, A., Yamamori, M., and Okamura, N. (2017). In Vitro and In Vivo Cytotoxicity of Troglitazone in Pancreatic Cancer. J. Exp. Clin. Cancer Res. 36 (1), 91. doi:10.1186/s13046-017-0557-6
Fukano, K., Tsukuda, S., Oshima, M., Suzuki, R., Aizaki, H., Ohki, M., et al. (2018). Troglitazone Impedes the Oligomerization of Sodium Taurocholate Cotransporting Polypeptide and Entry of Hepatitis B Virus into Hepatocytes. Front. Microbiol. 9, 3257. doi:10.3389/fmicb.2018.03257
Gallardo, E., Navarro, A., Viñolas, N., Marrades, R. M., Diaz, T., Gel, B., et al. (2009). miR-34a as a Prognostic Marker of Relapse in Surgically Resected Non-Small-Cell Lung Cancer. Carcinogenesis 30 (11), 1903–1909. doi:10.1093/carcin/bgp219
Gao, N., Wang, F. X., Wang, G., and Zhao, Q. S. (2018). Targeting the HMGA2 Oncogene by miR-498 Inhibits Non-Small Cell Lung Cancer Biological Behaviors. Eur. Rev. Med. Pharmacol. Sci. 22 (6), 1693–1699. doi:10.26355/eurrev_201803_14582
Garbe, C., and Eigentler, T. K. (2018). Vemurafenib. Recent Results Cancer Res. 211, 77–89. doi:10.1007/978-3-319-91442-8_6
Ge, S. X., Jung, D., and Yao, R. (2020). ShinyGO: A Graphical Gene-Set Enrichment Tool for Animals and Plants. Bioinformatics 36 (8), 2628–2629. doi:10.1093/bioinformatics/btz931
Gencer, E. B., Ural, A. U., Avcu, F., and Baran, Y. (2011). A Novel Mechanism of Dasatinib-Induced Apoptosis in Chronic Myeloid Leukemia; Ceramide Synthase and Ceramide Clearance Genes. Ann. Hematol. 90 (11), 1265–1275. doi:10.1007/s00277-011-1212-5
Ghosh, Z., Mallick, B., and Chakrabarti, J. (2009). Cellular versus Viral microRNAs in Host-Virus Interaction. Nucleic Acids Res. 37 (4), 1035–1048. doi:10.1093/nar/gkn1004
Goldman, M. J., Craft, B., Hastie, M., Repečka, K., McDade, F., Kamath, A., et al. (2020). Visualizing and Interpreting Cancer Genomics Data via the Xena Platform. Nat. Biotechnol. 38 (6), 675–678. doi:10.1038/s41587-020-0546-8
Gomes, M., Teixeira, A. L., Coelho, A., Araújo, A., and Medeiros, R. (2014). The Role of Inflammation in Lung Cancer. Adv. Exp. Med. Biol. 816, 1–23. doi:10.1007/978-3-0348-0837-8_1
González‐Gualda, E., Baker, A. G., Fruk, L., and Muñoz‐Espín, D. (2021). A Guide to Assessing Cellular Senescence In Vitro and In Vivo. Febs j 288 (1), 56–80. doi:10.1111/febs.15570
Guisado-Vasco, P., Valderas-Ortega, S., Carralón-González, M. M., Roda-Santacruz, A., González-Cortijo, L., Sotres-Fernández, G., et al. (2020). Clinical Characteristics and Outcomes Among Hospitalized Adults with Severe COVID-19 Admitted to a Tertiary Medical Center and Receiving Antiviral, Antimalarials, Glucocorticoids, or Immunomodulation with Tocilizumab or Cyclosporine: A Retrospective Observational Study (COQUIMA Cohort). EClinicalMedicine 28, 100591. doi:10.1016/j.eclinm.2020.100591
Guo, Q., Zhong, M., Xu, H., Mao, X., Zhang, Y., and Lin, N. (2015). A Systems Biology Perspective on the Molecular Mechanisms Underlying the Therapeutic Effects of Buyang Huanwu Decoction on Ischemic Stroke. Rejuvenation Res. 18 (4), 313–325. doi:10.1089/rej.2014.1635
Gupta, S., Silveira, D. A., and Mombach, J. C. M. (2020). ATM/miR-34a-5p Axis Regulates a P21-dependent Senescence-Apoptosis Switch in Non-small Cell Lung Cancer: A Boolean Model of G1/S Checkpoint Regulation. FEBS Lett. 594 (2), 227–239. doi:10.1002/1873-3468.13615
Hadjadj, J., Yatim, N., Barnabei, L., Corneau, A., Boussier, J., Smith, N., et al. (2020). Impaired Type I Interferon Activity and Inflammatory Responses in Severe COVID-19 Patients. Science 369 (6504), 718–724. doi:10.1126/science.abc6027
Hamamoto, I., Harazaki, K., Inase, N., Takaku, H., Tashiro, M., and Yamamoto, N. (2013). Cyclosporin A Inhibits the Propagation of Influenza Virus by Interfering with a Late Event in the Virus Life Cycle. Jpn. J. Infect. Dis. 66 (4), 276–283. doi:10.7883/yoken.66.276
Hamosh, A., Scott, A. F., Amberger, J. S., Bocchini, C. A., and McKusick, V. A. (2005). Online Mendelian Inheritance in Man (OMIM), a Knowledgebase of Human Genes and Genetic Disorders. Nucleic Acids Res. 33 (Database Issue), D514–D517. doi:10.1093/nar/gki033
Han, N., Yuan, X., Wu, H., Xu, H., Chu, Q., Guo, M., et al. (2015). DACH1 Inhibits Lung Adenocarcinoma Invasion and Tumor Growth by Repressing CXCL5 Signaling. Oncotarget 6 (8), 5877–5888. doi:10.18632/oncotarget.3463
Han, X., Huang, C., Qu, X., Liu, S., Yang, X., Wang, Y., et al. (2019). Tripartite Motif-Containing 15 Overexpression in Non-Small Cell Lung Cancer Is Associated with Poor Patient Prognoses. J. Cancer 10 (4), 843–852. doi:10.7150/jca.27856
Hanahan, D., and Weinberg, R. A. (2000). The Hallmarks of Cancer. Cell 100 (1), 57–70. doi:10.1016/s0092-8674(00)81683-9
Hasan, M. I., Rahman, M. H., Islam, M. B., Islam, M. Z., Hossain, M. A., and Moni, M. A. (2022). Systems Biology and Bioinformatics Approach to Identify Blood Based Signatures Molecules and Drug Targets of Patient with COVID-19. Inform. Med. Unlocked 28, 100840. doi:10.1016/j.imu.2021.100840
Hasegawa, T., Suzuki, H., Abe, J., Sakurada, A., Endo, C., Sato, N., et al. (2020). A Phase 2 Study of Adjuvant Carboplatin Plus S-1 Followed by Maintenance S-1 Therapy for Patients with Completely Resected Stage II/IIIA Non-small Cell Lung Cancer-Japanese Northern East Area Thoracic Surgery Study Group JNETS1302 Study. J. Thorac. Dis. 12 (7), 3591–3601. doi:10.21037/jtd-20-715
Hernandez-Segura, A., Nehme, J., and Demaria, M. (2018). Hallmarks of Cellular Senescence. Trends Cel. Biol. 28 (6), 436–453. doi:10.1016/j.tcb.2018.02.001
Ho, G. Y., Woodward, N., and Coward, J. I. (2016). Cisplatin versus Carboplatin: Comparative Review of Therapeutic Management in Solid Malignancies. Crit. Rev. Oncol. Hematol. 102, 37–46. doi:10.1016/j.critrevonc.2016.03.014
Holzberg, M., Boergeling, Y., Schräder, T., Ludwig, S., and Ehrhardt, C. (2017). Vemurafenib Limits Influenza A Virus Propagation by Targeting Multiple Signaling Pathways. Front. Microbiol. 8, 2426. doi:10.3389/fmicb.2017.02426
Hou, G. X., Liu, P., Yang, J., and Wen, S. (2017). Mining Expression and Prognosis of Topoisomerase Isoforms in Non-Small-Cell Lung Cancer by Using Oncomine and Kaplan-Meier Plotter. PLoS One 12 (3), e0174515. doi:10.1371/journal.pone.0174515
Hu, H., Wang, K., Wang, L., Du, Y., Chen, J., Li, Y., et al. (2021). He-Jie-Shen-Shi Decoction as an Adjuvant Therapy on Severe Coronavirus Disease 2019: A Retrospective Cohort and Potential Mechanistic Study. Front. Pharmacol. 12, 700498. doi:10.3389/fphar.2021.700498
Hu, X., Li, J., Fu, M., Zhao, X., and Wang, W. (2021). The JAK/STAT Signaling Pathway: From Bench to Clinic. Signal. Transduct. Target. Ther. 6 (1), 402. doi:10.1038/s41392-021-00791-1
Huang, Z., Cao, Y., Zhou, M., Qi, X., Fu, B., Mou, Y., et al. (2019). Hsa_circ_0005519 Increases IL-13/IL-6 by Regulating Hsa-Let-7a-5p in CD4+ T Cells to Affect Asthma. Clin. Exp. Allergy 49 (8), 1116–1127. doi:10.1111/cea.13445
Hung, I. F., Lung, K. C., Tso, E. Y., Liu, R., Chung, T. W., Chu, M. Y., et al. (2020). Triple Combination of Interferon Beta-1b, Lopinavir-Ritonavir, and Ribavirin in the Treatment of Patients Admitted to Hospital with COVID-19: An Open-Label, Randomised, Phase 2 Trial. Lancet 395 (10238), 1695–1704. doi:10.1016/s0140-6736(20)31042-4
Josset, L., Textoris, J., Loriod, B., Ferraris, O., Moules, V., Lina, B., et al. (2010). Gene Expression Signature-Based Screening Identifies New Broadly Effective Influenza a Antivirals. PLoS One 5 (10), e13169. doi:10.1371/journal.pone.0013169
Kandhaya-Pillai, R., Miro-Mur, F., Alijotas-Reig, J., Tchkonia, T., Kirkland, J. L., and Schwartz, S. (2017). Tnfα-Senescence Initiates a STAT-Dependent Positive Feedback Loop, Leading to a Sustained Interferon Signature, DNA Damage, and Cytokine Secretion. Aging (Albany NY) 9 (11), 2411–2435. doi:10.18632/aging.101328
Kanehisa, M., and Goto, S. (2000). KEGG: Kyoto Encyclopedia of Genes and Genomes. Nucleic Acids Res. 28 (1), 27–30. doi:10.1093/nar/28.1.27
Karagkouni, D., Paraskevopoulou, M. D., Chatzopoulos, S., Vlachos, I. S., Tastsoglou, S., Kanellos, I., et al. (2018). DIANA-TarBase V8: A Decade-Long Collection of Experimentally Supported miRNA-Gene Interactions. Nucleic Acids Res. 46 (D1), D239–d245. doi:10.1093/nar/gkx1141
Kasinski, A. L., and Slack, F. J. (2012). miRNA-34 Prevents Cancer Initiation and Progression in a Therapeutically Resistant K-Ras and P53-Induced Mouse Model of Lung Adenocarcinoma. Cancer Res. 72 (21), 5576–5587. doi:10.1158/0008-5472.Can-12-2001
Kedhari Sundaram, M., Hussain, A., Haque, S., Raina, R., and Afroze, N. (2019). Quercetin Modifies 5'CpG Promoter Methylation and Reactivates Various Tumor Suppressor Genes by Modulating Epigenetic Marks in Human Cervical Cancer Cells. J. Cel. Biochem. 120 (10), 18357–18369. doi:10.1002/jcb.29147
Keenan, A. B., Torre, D., Lachmann, A., Leong, A. K., Wojciechowicz, M. L., Utti, V., et al. (2019). ChEA3: Transcription Factor Enrichment Analysis by Orthogonal Omics Integration. Nucleic Acids Res. 47 (W1), W212–w224. doi:10.1093/nar/gkz446
Khokhar, M., Tomo, S., and Purohit, P. (2022). MicroRNAs Based Regulation of Cytokine Regulating Immune Expressed Genes and Their Transcription Factors in COVID-19. Meta Gene 31, 100990. doi:10.1016/j.mgene.2021.100990
Kim, D. H., Khan, H., Ullah, H., Hassan, S. T. S., Šmejkal, K., Efferth, T., et al. (2019). MicroRNA Targeting by Quercetin in Cancer Treatment and Chemoprotection. Pharmacol. Res. 147, 104346. doi:10.1016/j.phrs.2019.104346
Kim, K. S., Kang, K. W., Seu, Y. B., Baek, S. H., and Kim, J. R. (2009). Interferon-Gamma Induces Cellular Senescence Through P53-Dependent DNA Damage Signaling in Human Endothelial Cells. Mech. Ageing Dev. 130 (3), 179–188. doi:10.1016/j.mad.2008.11.004
Kim, S., Chen, J., Cheng, T., Gindulyte, A., He, J., He, S., et al. (2019). PubChem 2019 Update: Improved Access to Chemical Data. Nucleic Acids Res. 47 (D1), D1102–d1109. doi:10.1093/nar/gky1033
Kim, W. R., Park, E. G., Kang, K. W., Lee, S. M., Kim, B., and Kim, H. S. (2020). Expression Analyses of MicroRNAs in Hamster Lung Tissues Infected by SARS-CoV-2. Mol. Cell 43 (11), 953–963. doi:10.14348/molcells.2020.0177
Kimura, S., Kuramoto, K., Homan, J., Naruoka, H., Ego, T., Nogawa, M., et al. (2012). Antiproliferative and Antitumor Effects of Azacitidine against the Human Myelodysplastic Syndrome Cell Line SKM-1. Anticancer Res. 32 (3), 795–798.
Kishore, C., Sundaram, S., and Karunagaran, D. (2019). Vitamin K3 (Menadione) Suppresses Epithelial-Mesenchymal-Transition and Wnt Signaling Pathway in Human Colorectal Cancer Cells. Chem. Biol. Interact 309, 108725. doi:10.1016/j.cbi.2019.108725
Kuleshov, M. V., Jones, M. R., Rouillard, A. D., Fernandez, N. F., Duan, Q., Wang, Z., et al. (2016). Enrichr: a Comprehensive Gene Set Enrichment Analysis Web Server 2016 Update. Nucleic Acids Res. 44 (W1), W90–W97. doi:10.1093/nar/gkw377
Kumari, R., and Jat, P. (2021). Mechanisms of Cellular Senescence: Cell Cycle Arrest and Senescence Associated Secretory Phenotype. Front. Cel. Dev. Biol. 9, 645593. doi:10.3389/fcell.2021.645593
Kuźnar-Kamińska, B., Mikuła-Pietrasik, J., Książek, K., Tykarski, A., and Batura-Gabryel, H. (2018). Lung Cancer in Chronic Obstructive Pulmonary Disease Patients: Importance of Cellular Senescence. Pol. Arch. Intern. Med. 128 (7-8), 462–468. doi:10.20452/pamw.4297
Kuźnar-Kamińska, B., Mikuła-Pietrasik, J., Sosińska, P., Książek, K., and Batura-Gabryel, H. (2016). COPD Promotes Migration of A549 Lung Cancer Cells: the Role of Chemokine CCL21. Int. J. Chron. Obstruct. Pulmon. Dis. 11, 1061–1066. doi:10.2147/copd.S96490
Lachmann, A., Torre, D., Keenan, A. B., Jagodnik, K. M., Lee, H. J., Wang, L., et al. (2018). Massive Mining of Publicly Available RNA-Seq Data from Human and Mouse. Nat. Commun. 9 (1), 1366. doi:10.1038/s41467-018-03751-6
Lee, B., Min, J. A., Nashed, A., Lee, S. O., Yoo, J. C., Chi, S. W., et al. (2019). A Novel Mechanism of Irinotecan Targeting MDM2 and Bcl-xL. Biochem. Biophys. Res. Commun. 514 (2), 518–523. doi:10.1016/j.bbrc.2019.04.009
Lee, H., Yoon, N. E., and Jung, B. H. (2017). Metabolomics Study of Cancer Targeting Small Molecule Kinase Inhibitors in Cell Culture. Drug Metab. Pharmacokinet. 32 (1), S77. doi:10.1016/j.dmpk.2016.10.304
Leidinger, P., Brefort, T., Backes, C., Krapp, M., Galata, V., Beier, M., et al. (2016). High-throughput qRT-PCR Validation of Blood microRNAs in Non-small Cell Lung Cancer. Oncotarget 7 (4), 4611–4623. doi:10.18632/oncotarget.6566
Li, C., Hu, X., Li, L., and Li, J. H. (2020). Differential microRNA Expression in the Peripheral Blood from Human Patients with COVID-19. J. Clin. Lab. Anal. 34 (10), e23590. doi:10.1002/jcla.23590
Li, J., Zheng, S., Chen, B., Butte, A. J., Swamidass, S. J., and Lu, Z. (2016). A Survey of Current Trends in Computational Drug Repositioning. Brief Bioinform 17 (1), 2–12. doi:10.1093/bib/bbv020
Li, Q., Li, M., Zheng, K., Li, H., Yang, H., Ma, S., et al. (2020). Detection of microRNA Expression Levels Based on Microarray Analysis for Classification of Idiopathic Pulmonary Fibrosis. Exp. Ther. Med. 20 (4), 3096–3103. doi:10.3892/etm.2020.9068
Li, X., Mei, Q., Nie, J., Fu, X., and Han, W. (2015). Decitabine: a Promising Epi-Immunotherapeutic Agent in Solid Tumors. Expert Rev. Clin. Immunol. 11 (3), 363–375. doi:10.1586/1744666x.2015.1002397
Li, Y., Yao, J., Han, C., Yang, J., Chaudhry, M. T., Wang, S., et al. (2016). Quercetin, Inflammation and Immunity. Nutrients 8 (3), 167. doi:10.3390/nu8030167
Liang, J., Bi, N., Wu, S., Chen, M., Lv, C., Zhao, L., et al. (2017). Etoposide and Cisplatin versus Paclitaxel and Carboplatin with Concurrent Thoracic Radiotherapy in Unresectable Stage III Non-small Cell Lung Cancer: a Multicenter Randomized Phase III Trial. Ann. Oncol. 28 (4), 777–783. doi:10.1093/annonc/mdx009
Liang, W., Guan, W., Chen, R., Wang, W., Li, J., Xu, K., et al. (2020). Cancer Patients in SARS-CoV-2 Infection: a Nationwide Analysis in China. Lancet Oncol. 21 (3), 335–337. doi:10.1016/s1470-2045(20)30096-6
Liao, Z., Rivin Del Campo, E., Salem, A., Pang, Q., Liu, H., and Lopez Guerra, J. L. (2020). Optimizing Lung Cancer Radiation Treatment Worldwide in COVID-19 Outbreak. Lung Cancer 146, 230–235. doi:10.1016/j.lungcan.2020.05.029
Lim, W., Jeong, M., Bazer, F. W., and Song, G. (2017). Coumestrol Inhibits Proliferation and Migration of Prostate Cancer Cells by Regulating AKT, ERK1/2, and JNK MAPK Cell Signaling Cascades. J. Cel. Physiol. 232 (4), 862–871. doi:10.1002/jcp.25494
Lin, S. C., Ho, C. T., Chuo, W. H., Li, S., Wang, T. T., and Lin, C. C. (2017). Effective Inhibition of MERS-CoV Infection by Resveratrol. BMC Infect. Dis. 17 (1), 144. doi:10.1186/s12879-017-2253-8
Liu, B., Li, J., and Cairns, M. J. (2014). Identifying miRNAs, Targets and Functions. Brief Bioinform. 15 (1), 1–19. doi:10.1093/bib/bbs075
Liu, M., Liu, H., and Chen, J. (2018). Mechanisms of the CDK4/6 Inhibitor Palbociclib (PD 0332991) and its Future Application in Cancer Treatment (Review). Oncol. Rep. 39 (3), 901–911. doi:10.3892/or.2018.6221
Liu, Q., Yu, Z., Yuan, S., Xie, W., Li, C., Hu, Z., et al. (2017). Circulating Exosomal microRNAs as Prognostic Biomarkers for Non-small-cell Lung Cancer. Oncotarget 8 (8), 13048–13058. doi:10.18632/oncotarget.14369
Liu, Y., Wang, K., Massoud, T. F., and Paulmurugan, R. (2020). SARS-CoV-2 Vaccine Development: An Overview and Perspectives. ACS Pharmacol. Transl. Sci. 3 (5), 844–858. doi:10.1021/acsptsci.0c00109
Liu, Z., Jiang, L., Zhang, G., Li, S., and Jiang, X. (2018). MiR-24 Promotes Migration and Invasion of Non-small Cell Lung Cancer by Targeting ZNF367. J. Buon. 23 (5), 1413–1419.
Longley, D. B., Harkin, D. P., and Johnston, P. G. (2003). 5-fluorouracil: Mechanisms of Action and Clinical Strategies. Nat. Rev. Cancer 3 (5), 330–338. doi:10.1038/nrc1074
Lopez Bernal, J., Andrews, N., Gower, C., Gallagher, E., Simmons, R., Thelwall, S., et al. (2021). Effectiveness of Covid-19 Vaccines against the B.1.617.2 (Delta) Variant. N. Engl. J. Med. 385 (7), 585–594. doi:10.1056/NEJMoa2108891
Love, M. I., Huber, W., and Anders, S. (2014). Moderated Estimation of Fold Change and Dispersion for RNA-Seq Data with DESeq2. Genome Biol. 15 (12), 550. doi:10.1186/s13059-014-0550-8
Ma, W., Wang, B., Zhang, Y., Wang, Z., Niu, D., Chen, S., et al. (2019). Prognostic Significance of TOP2A in Non-small Cell Lung Cancer Revealed by Bioinformatic Analysis. Cancer Cel. Int. 19, 239. doi:10.1186/s12935-019-0956-1
Majumder, J., and Minko, T. (2021). Recent Developments on Therapeutic and Diagnostic Approaches for COVID-19. Aaps j 23 (1), 14. doi:10.1208/s12248-020-00532-2
Malkani, N., and Rashid, M. U. (2021). SARS-COV-2 Infection and Lung Tumor Microenvironment. Mol. Biol. Rep. 48 (2), 1925–1934. doi:10.1007/s11033-021-06149-8
Mallah, S. I., Ghorab, O. K., Al-Salmi, S., Abdellatif, O. S., Tharmaratnam, T., Iskandar, M. A., et al. (2021). COVID-19: Breaking Down a Global Health Crisis. Ann. Clin. Microbiol. Antimicrob. 20 (1), 35. doi:10.1186/s12941-021-00438-7
Mason, R. J. (2020). Pathogenesis of COVID-19 from a Cell Biology Perspective. Eur. Respir. J. 55 (4), 2000607. doi:10.1183/13993003.00607-2020
Mercatelli, D., Pedace, E., Veltri, P., Giorgi, F. M., and Guzzi, P. H. (2021). Exploiting the Molecular Basis of Age and Gender Differences in Outcomes of SARS-CoV-2 Infections. Comput. Struct. Biotechnol. J. 19, 4092–4100. doi:10.1016/j.csbj.2021.07.002
Montecucco, A., Zanetta, F., and Biamonti, G. (2015). Molecular Mechanisms of Etoposide. Excli j 14, 95–108. doi:10.17179/excli2015-561
Muhammad, J. S., Saheb Sharif-Askari, N., Cui, Z. G., Hamad, M., and Halwani, R. (2021). SARS-CoV-2 Infection-Induced Promoter Hypomethylation as an Epigenetic Modulator of Heat Shock Protein A1L (HSPA1L) Gene. Front. Genet. 12, 622271. doi:10.3389/fgene.2021.622271
Mullen, D. J., Yan, C., Kang, D. S., Zhou, B., Borok, Z., Marconett, C. N., et al. (2020). TENET 2.0: Identification of Key Transcriptional Regulators and Enhancers in Lung Adenocarcinoma. Plos Genet. 16 (9), e1009023. doi:10.1371/journal.pgen.1009023
Murakami, S., Saito, H., Kondo, T., Ito, H., Oshita, F., and Yamada, K. (2018). Phase II Study of Nedaplatin and Irinotecan as Adjuvant Chemotherapy for Completely Resected Non-small Cell Lung Cancer. Cancer Chemother. Pharmacol. 81 (1), 81–87. doi:10.1007/s00280-017-3460-0
Nam, H. J., and van Deursen, J. M. (2014). Cyclin B2 and P53 Control Proper Timing of Centrosome Separation. Nat. Cel. Biol. 16 (6), 538–549. doi:10.1038/ncb2952
Nehme, J., Borghesan, M., Mackedenski, S., Bird, T. G., and Demaria, M. (2020). Cellular Senescence as a Potential Mediator of COVID-19 Severity in the Elderly. Aging Cell 19 (10), e13237. doi:10.1111/acel.13237
Nicoś, M., Rolska-Kopińska, A., Krawczyk, P., Grenda, A., Bożyk, A., Szczyrek, M., et al. (2021). Effect of TOP2A and ERCC1 Gene Polymorphisms on the Efficacy and Toxicity of Cisplatin and Etoposide-Based Chemotherapy in Small Cell Lung Cancer Patients. Arch. Med. Sci. 17 (2), 474–480. doi:10.5114/aoms.2020.92572
Nie, H., Zhou, X., Shuzhang, D., Nie, C., Zhang, X., and Huang, J. (2019). Palbociclib Overcomes Afatinib Resistance in Non-small Cell Lung Cancer. Biomed. Pharmacother. 109, 1750–1757. doi:10.1016/j.biopha.2018.10.170
Nokihara, H., Lu, S., Mok, T. S. K., Nakagawa, K., Yamamoto, N., Shi, Y. K., et al. (2017). Randomized Controlled Trial of S-1 versus Docetaxel in Patients with Non-small-cell Lung Cancer Previously Treated with Platinum-Based Chemotherapy (East Asia S-1 Trial in Lung Cancer). Ann. Oncol. 28 (11), 2698–2706. doi:10.1093/annonc/mdx419
Olas, B., and Wachowicz, B. (2005). Resveratrol, a Phenolic Antioxidant with Effects on Blood Platelet Functions. Platelets 16 (5), 251–260. doi:10.1080/09537100400020591
Olloquequi, J. (2020). COVID-19 Susceptibility in Chronic Obstructive Pulmonary Disease. Eur. J. Clin. Invest. 50 (10), e13382. doi:10.1111/eci.13382
Overmyer, K. A., Shishkova, E., Miller, I. J., Balnis, J., Bernstein, M. N., Peters-Clarke, T. M., et al. (2021). Large-Scale Multi-Omic Analysis of COVID-19 Severity. Cell Syst. 12 (1), 23–e7. e27. doi:10.1016/j.cels.2020.10.003
Parsons, P. G., Lean, J., Khoo, S. K., and Lark, J. (1989). Effects of Adriamycin and Etoposide on the Replication of Adenovirus 5 in Sensitive and Resistant Human Tumour Cells. Biochem. Pharmacol. 38 (1), 31–37. doi:10.1016/0006-2952(89)90145-7
Passaro, A., Bestvina, C., Velez Velez, M., Garassino, M. C., Garon, E., and Peters, S. (2021). Severity of COVID-19 in Patients with Lung Cancer: Evidence and Challenges. J. Immunother. Cancer 9 (3), e002266. doi:10.1136/jitc-2020-002266
Passaro, A., Peters, S., Mok, T. S. K., Attili, I., Mitsudomi, T., and de Marinis, F. (2020). Testing for COVID-19 in Lung Cancer Patients. Ann. Oncol. 31 (7), 832–834. doi:10.1016/j.annonc.2020.04.002
Pathak, A. K., Bhutani, M., Guleria, R., Bal, S., Mohan, A., Mohanti, B. K., et al. (2005). Chemotherapy Alone vs. Chemotherapy Plus High Dose Multiple Antioxidants in Patients with Advanced Non Small Cell Lung Cancer. J. Am. Coll. Nutr. 24 (1), 16–21. doi:10.1080/07315724.2005.10719438
Piñero, J., Ramírez-Anguita, J. M., Saüch-Pitarch, J., Ronzano, F., Centeno, E., Sanz, F., et al. (2020). The DisGeNET Knowledge Platform for Disease Genomics: 2019 Update. Nucleic Acids Res. 48 (D1), D845–d855. doi:10.1093/nar/gkz1021
Pleschka, S., Wolff, T., Ehrhardt, C., Hobom, G., Planz, O., Rapp, U. R., et al. (2001). Influenza Virus Propagation Is Impaired by Inhibition of the Raf/MEK/ERK Signalling cascade. Nat. Cel. Biol. 3 (3), 301–305. doi:10.1038/35060098
Poulsen, N. N., von Brunn, A., Hornum, M., and Blomberg Jensen, M. (2020). Cyclosporine and COVID-19: Risk or Favorable? Am. J. Transpl. 20 (11), 2975–2982. doi:10.1111/ajt.16250
Qian, X., Song, X., He, Y., Yang, Z., Sun, T., Wang, J., et al. (2015). CCNB2 Overexpression Is a Poor Prognostic Biomarker in Chinese NSCLC Patients. Biomed. Pharmacother. 74, 222–227. doi:10.1016/j.biopha.2015.08.004
Qiao, C., Qian, H., Wang, J., Zhao, T., Ma, P., Wang, S., et al. (2020). PD173074 Blocks G1/S Transition via CUL3-Mediated Ubiquitin Protease in HepG2 and Hep3B Cells. PLoS One 15 (6), e0234708. doi:10.1371/journal.pone.0234708
Rabinovich, A., Medina, L., Piura, B., Segal, S., and Huleihel, M. (2007). Regulation of Ovarian Carcinoma SKOV-3 Cell Proliferation and Secretion of MMPs by Autocrine IL-6. Anticancer Res. 27 (1a), 267–272.
Rawson, J. M., Landman, S. R., Reilly, C. S., Bonnac, L., Patterson, S. E., and Mansky, L. M. (2015). Lack of Mutational Hot Spots during Decitabine-Mediated HIV-1 Mutagenesis. Antimicrob. Agents Chemother. 59 (11), 6834–6843. doi:10.1128/aac.01644-15
Rawson, J. M., Roth, M. E., Xie, J., Daly, M. B., Clouser, C. L., Landman, S. R., et al. (2016). Synergistic Reduction of HIV-1 Infectivity by 5-azacytidine and Inhibitors of Ribonucleotide Reductase. Bioorg. Med. Chem. 24 (11), 2410–2422. doi:10.1016/j.bmc.2016.03.052
Samprathi, M., and Jayashree, M. (2020). Biomarkers in COVID-19: An Up-To-Date Review. Front. Pediatr. 8, 607647. doi:10.3389/fped.2020.607647
Satarker, S., Tom, A. A., Shaji, R. A., Alosious, A., Luvis, M., and Nampoothiri, M. (2021). JAK-STAT Pathway Inhibition and Their Implications in COVID-19 Therapy. Postgrad. Med. 133 (5), 489–507. doi:10.1080/00325481.2020.1855921
Shang, Z., Chan, S. Y., Liu, W. J., Li, P., and Huang, W. (2021). Recent Insights into Emerging Coronavirus: SARS-CoV-2. ACS Infect. Dis. 7 (6), 1369–1388. doi:10.1021/acsinfecdis.0c00646
Shannon, P., Markiel, A., Ozier, O., Baliga, N. S., Wang, J. T., Ramage, D., et al. (2003). Cytoscape: a Software Environment for Integrated Models of Biomolecular Interaction Networks. Genome Res. 13 (11), 2498–2504. doi:10.1101/gr.1239303
Sharma, R., Upadhyay, S., Bhat, B., Singh, G., Bhattacharya, S., and Singh, A. (2020). Abiotic Stress Induced miRNA-TF-Gene Regulatory Network: A Structural Perspective. Genomics 112 (1), 412–422. doi:10.1016/j.ygeno.2019.03.004
Sherr, C. J. (1996). Cancer Cell Cycles. Science 274 (5293), 1672–1677. doi:10.1126/science.274.5293.1672
Shibabaw, T. (2020). Inflammatory Cytokine: IL-17A Signaling Pathway in Patients Present with COVID-19 and Current Treatment Strategy. J. Inflamm. Res. 13, 673–680. doi:10.2147/jir.S278335
Sinha, S., and Kundu, C. N. (2021). Cancer and COVID-19: Why Are Cancer Patients More Susceptible to COVID-19? Med. Oncol. 38 (9), 101. doi:10.1007/s12032-021-01553-3
Smolle, E., Fink-Neuboeck, N., Lindenmann, J., Smolle-Juettner, F., and Pichler, M. (2019). The Biological and Clinical Relevance of Inhibitor of Growth (ING) Genes in Non-small Cell Lung Cancer. Cancers (Basel) 11 (8), 1118. doi:10.3390/cancers11081118
Solimani, F., Meier, K., and Ghoreschi, K. (2021). Janus Kinase Signaling as Risk Factor and Therapeutic Target for Severe SARS-CoV-2 Infection. Eur. J. Immunol. 51 (5), 1071–1075. doi:10.1002/eji.202149173
Song, X., Wu, J. Q., Yu, X. F., Yang, X. S., and Yang, Y. (2018). Trichostatin A Inhibits Proliferation of Triple Negative Breast Cancer Cells by Inducing Cell Cycle Arrest and Apoptosis. Neoplasma 65 (6), 898–906. doi:10.4149/neo_2018_181212N476
Stantchev, T. S., Markovic, I., Telford, W. G., Clouse, K. A., and Broder, C. C. (2007). The Tyrosine Kinase Inhibitor Genistein Blocks HIV-1 Infection in Primary Human Macrophages. Virus. Res. 123 (2), 178–189. doi:10.1016/j.virusres.2006.09.004
Starchenko, A., and Lauffenburger, D. A. (2018). In Vivo systems Biology Approaches to Chronic Immune/inflammatory Pathophysiology. Curr. Opin. Biotechnol. 52, 9–16. doi:10.1016/j.copbio.2018.02.006
Stelzer, G., Rosen, N., Plaschkes, I., Zimmerman, S., Twik, M., Fishilevich, S., et al. (2016). The GeneCards Suite: From Gene Data Mining to Disease Genome Sequence Analyses. Curr. Protoc. Bioinformatics 54, 1.30.1–1.30.33. doi:10.1002/cpbi.5
Sterlacci, W., Fiegl, M., and Tzankov, A. (2012). Prognostic and Predictive Value of Cell Cycle Deregulation in Non-small-cell Lung Cancer. Pathobiology 79 (4), 175–194. doi:10.1159/000336462
Sung, H., Ferlay, J., Siegel, R. L., Laversanne, M., Soerjomataram, I., Jemal, A., et al. (2021). Global Cancer Statistics 2020: GLOBOCAN Estimates of Incidence and Mortality Worldwide for 36 Cancers in 185 Countries. CA Cancer J. Clin. 71 (3), 209–249. doi:10.3322/caac.21660
Suresh, D., Zambre, A., Mukherjee, S., Ghoshdastidar, S., Jiang, Y., Joshi, T., et al. (2019). Silencing AXL by Covalent siRNA-Gelatin-Antibody Nanoconjugate Inactivates mTOR/EMT Pathway and Stimulates P53 for TKI Sensitization in NSCLC. Nanomedicine 20, 102007. doi:10.1016/j.nano.2019.04.010
Takashima, S., Saito, H., Takahashi, N., Imai, K., Kudo, S., Atari, M., et al. (2014). Strong Expression of Cyclin B2 mRNA Correlates with a Poor Prognosis in Patients with Non-small Cell Lung Cancer. Tumour Biol. 35 (5), 4257–4265. doi:10.1007/s13277-013-1556-7
Tang, S. M., Deng, X. T., Zhou, J., Li, Q. P., Ge, X. X., and Miao, L. (2020). Pharmacological Basis and New Insights of Quercetin Action in Respect to its Anti-cancer Effects. Biomed. Pharmacother. 121, 109604. doi:10.1016/j.biopha.2019.109604
Tay, M. Z., Poh, C. M., Rénia, L., MacAry, P. A., and Ng, L. F. P. (2020). The trinity of COVID-19: Immunity, Inflammation and Intervention. Nat. Rev. Immunol. 20 (6), 363–374. doi:10.1038/s41577-020-0311-8
Tian, S., Hu, W., Niu, L., Liu, H., Xu, H., and Xiao, S. Y. (2020). Pulmonary Pathology of Early-phase 2019 Novel Coronavirus (COVID-19) Pneumonia in Two Patients with Lung Cancer. J. Thorac. Oncol. 15 (5), 700–704. doi:10.1016/j.jtho.2020.02.010
Tianqin, G., Chunlei, C., and Jingjing, W. (2016). Synergistic Anti-glioma Effects In Vitro and In Vivo of Enediyne Antibiotic Neocarzinostatin and Paclitaxel via Enhanced Growth Delay and Apoptosis-Induction. Biol. Pharm. Bull. 39 (10), 1623–1630. doi:10.1248/bpb.b16-00285
Umakanthan, S., Sahu, P., Ranade, A. V., Bukelo, M. M., Rao, J. S., Abrahao-Machado, L. F., et al. (2020). Origin, Transmission, Diagnosis and Management of Coronavirus Disease 2019 (COVID-19). Postgrad. Med. J. 96 (1142), 753–758. doi:10.1136/postgradmedj-2020-138234
Ungvari, Z., Tarantini, S., Kiss, T., Wren, J. D., Giles, C. B., Griffin, C. T., et al. (2018). Endothelial Dysfunction and Angiogenesis Impairment in the Ageing Vasculature. Nat. Rev. Cardiol. 15 (9), 555–565. doi:10.1038/s41569-018-0030-z
van Driel, M. A., and Brunner, H. G. (2006). Bioinformatics Methods for Identifying Candidate Disease Genes. Hum. Genomics 2 (6), 429–432. doi:10.1186/1479-7364-2-6-429
Vono, M., Huttner, A., Lemeille, S., Martinez-Murillo, P., Meyer, B., Baggio, S., et al. (2021). Robust Innate Responses to SARS-CoV-2 in Children Resolve Faster Than in Adults without Compromising Adaptive Immunity. Cell Rep. 37 (1), 109773. doi:10.1016/j.celrep.2021.109773
Wald, O., Izhar, U., Amir, G., Kirshberg, S., Shlomai, Z., Zamir, G., et al. (2011). Interaction between Neoplastic Cells and Cancer-Associated Fibroblasts through the CXCL12/CXCR4 axis: Role in Non-small Cell Lung Cancer Tumor Proliferation. J. Thorac. Cardiovasc. Surg. 141 (6), 1503–1512. doi:10.1016/j.jtcvs.2010.11.056
Walls, A. C., Park, Y. J., Tortorici, M. A., Wall, A., McGuire, A. T., and Veesler, D. (2020). Structure, Function, and Antigenicity of the SARS-CoV-2 Spike Glycoprotein. Cell 181 (2), 281–e6. e286. doi:10.1016/j.cell.2020.02.058
Wang, M., Herbst, R. S., and Boshoff, C. (2021). Toward Personalized Treatment Approaches for Non-small-cell Lung Cancer. Nat. Med. 27 (8), 1345–1356. doi:10.1038/s41591-021-01450-2
Wang, X., and Powell, C. A. (2021). How to Translate the Knowledge of COVID‐19 into the Prevention of Omicron Variants. Clin. Translational Med 11 (12), e680. doi:10.1002/ctm2.680
Wang, Y., Zhang, S., Li, F., Zhou, Y., Zhang, Y., Wang, Z., et al. (2020). Therapeutic Target Database 2020: Enriched Resource for Facilitating Research and Early Development of Targeted Therapeutics. Nucleic Acids Res. 48 (D1), D1031–d1041. doi:10.1093/nar/gkz981
Wei, D., Sun, L., and Feng, W. (2021). hsa_circ_0058357 Acts as a ceRNA to Promote Non-small C-ell L-ung C-ancer P-rogression via the hsa-miR-24-3p/AVL9 axis. Mol. Med. Rep. 23 (6), 470. doi:10.3892/mmr.2021.12109
Wen, L., Tang, K., Chik, K. K., Chan, C. C., Tsang, J. O., Liang, R., et al. (2021). In Silico structure-based Discovery of a SARS-CoV-2 Main Protease Inhibitor. Int. J. Biol. Sci. 17 (6), 1555–1564. doi:10.7150/ijbs.59191
Williams, G. H., and Stoeber, K. (2012). The Cell Cycle and Cancer. J. Pathol. 226 (2), 352–364. doi:10.1002/path.3022
Wooller, S. K., Benstead-Hume, G., Chen, X., Ali, Y., and Pearl, F. M. G. (2017). Bioinformatics in Translational Drug Discovery. Biosci. Rep. 37 (4), BSR20160180. doi:10.1042/bsr20160180
Worldometer (2021). COVID 19 Coronavirus Pandemic. [Online]. Available: https://www.worldometers.info/coronavirus/ (Accessed November 21, 2021).
Wu, T., Hu, E., Xu, S., Chen, M., Guo, P., Dai, Z., et al. (2021). clusterProfiler 4.0: A Universal Enrichment Tool for Interpreting Omics Data. The Innovation 2 (3), 100141. doi:10.1016/j.xinn.2021.100141
Wu, W., Li, R., Li, X., He, J., Jiang, S., Liu, S., et al. (2015). Quercetin as an Antiviral Agent Inhibits Influenza A Virus (IAV) Entry. Viruses 8 (1), 6. doi:10.3390/v8010006
Wu, X. Y., Chen, H. C., Li, W. W., Yan, J. D., and Lv, R. Y. (2020). DNMT1 Promotes Cell Proliferation via Methylating hMLH1 and hMSH2 Promoters in EGFR-Mutated Non-small Cell Lung Cancer. J. Biochem. 168 (2), 151–157. doi:10.1093/jb/mvaa034
Xing, X. L., Lu, Y., and Qiu, H. L. (2016). Effect of Cyclosporin A Particles of Varying Diameters on Gastric Cancer Cell Apoptosis. Genet. Mol. Res. 15 (2), gmr8085. doi:10.4238/gmr.15028085
Xu, C., Buczkowski, K. A., Zhang, Y., Asahina, H., Beauchamp, E. M., Terai, H., et al. (2015). NSCLC Driven by DDR2 Mutation Is Sensitive to Dasatinib and JQ1 Combination Therapy. Mol. Cancer Ther. 14 (10), 2382–2389. doi:10.1158/1535-7163.Mct-15-0077
Xu, D., Hu, M. J., Wang, Y. Q., and Cui, Y. L. (2019). Antioxidant Activities of Quercetin and its Complexes for Medicinal Application. Molecules 24 (6), 1123. doi:10.3390/molecules24061123
Yang, G., Rosen, D. G., Zhang, Z., Bast, R. C., Mills, G. B., Colacino, J. A., et al. (2006). The Chemokine Growth-Regulated Oncogene 1 (Gro-1) Links RAS Signaling to the Senescence of Stromal Fibroblasts and Ovarian Tumorigenesis. Proc. Natl. Acad. Sci. U S A. 103 (44), 16472–16477. doi:10.1073/pnas.0605752103
Yao, X., Ye, F., Zhang, M., Cui, C., Huang, B., Niu, P., et al. (2020). In Vitro Antiviral Activity and Projection of Optimized Dosing Design of Hydroxychloroquine for the Treatment of Severe Acute Respiratory Syndrome Coronavirus 2 (SARS-CoV-2). Clin. Infect. Dis. 71 (15), 732–739. doi:10.1093/cid/ciaa237
Yoo, M., Shin, J., Kim, J., Ryall, K. A., Lee, K., Lee, S., et al. (2015). DSigDB: Drug Signatures Database for Gene Set Analysis. Bioinformatics 31 (18), 3069–3071. doi:10.1093/bioinformatics/btv313
Yousef, M., Vlachogiannis, I. A., and Tsiani, E. (2017). Effects of Resveratrol against Lung Cancer: In Vitro and In Vivo Studies. Nutrients 9 (11), 1231. doi:10.3390/nu9111231
Yu, G., Wang, W., Wang, X., Xu, M., Zhang, L., Ding, L., et al. (2018). Network Pharmacology-Based Strategy to Investigate Pharmacological Mechanisms of Zuojinwan for Treatment of Gastritis. BMC Complement. Altern. Med. 18 (1), 292. doi:10.1186/s12906-018-2356-9
Yuan, S., Yu, Z., Liu, Q., Zhang, M., Xiang, Y., Wu, N., et al. (2016). GPC5, a Novel Epigenetically Silenced Tumor Suppressor, Inhibits Tumor Growth by Suppressing Wnt/β-Catenin Signaling in Lung Adenocarcinoma. Oncogene 35 (47), 6120–6131. doi:10.1038/onc.2016.149
Zappa, C., and Mousa, S. A. (2016). Non-small Cell Lung Cancer: Current Treatment and Future Advances. Transl. Lung Cancer Res. 5 (3), 288–300. doi:10.21037/tlcr.2016.06.07
Zhan, W., Wang, W., Han, T., Xie, C., Zhang, T., Gan, M., et al. (2017). COMMD9 Promotes TFDP1/E2F1 Transcriptional Activity via Interaction with TFDP1 in Non-small Cell Lung Cancer. Cell Signal 30, 59–66. doi:10.1016/j.cellsig.2016.11.016
Zhang, H., Shao, H., Golubovskaya, V. M., Chen, H., Cance, W., Adjei, A. A., et al. (2016). Efficacy of Focal Adhesion Kinase Inhibition in Non-small Cell Lung Cancer with Oncogenically Activated MAPK Pathways. Br. J. Cancer 115 (2), 203–211. doi:10.1038/bjc.2016.190
Zhang, H. M., Kuang, S., Xiong, X., Gao, T., Liu, C., and Guo, A. Y. (2015). Transcription Factor and microRNA Co-regulatory Loops: Important Regulatory Motifs in Biological Processes and Diseases. Brief Bioinform 16 (1), 45–58. doi:10.1093/bib/bbt085
Zhang, J., Li, D., Zhang, Y., Ding, Z., Zheng, Y., Chen, S., et al. (2020). Integrative Analysis of mRNA and miRNA Expression Profiles Reveals Seven Potential Diagnostic Biomarkers for Non-small C-ell L-ung C-ancer. Oncol. Rep. 43 (1), 99–112. doi:10.3892/or.2019.7407
Zhang, J. J., Hong, J., Ma, Y. S., Shi, Y., Zhang, D. D., Yang, X. L., et al. (2021). Identified GNGT1 and NMU as Combined Diagnosis Biomarker of Non-small-cell Lung Cancer Utilizing Bioinformatics and Logistic Regression. Dis. Markers 2021, 6696198. doi:10.1155/2021/6696198
Zhang, L., Han, X., and Shi, Y. (2020). Comparative Analysis of SARS-CoV-2 Receptor ACE2 Expression in Multiple Solid Tumors and Matched Non-diseased Tissues. Infect. Genet. Evol. 85, 104428. doi:10.1016/j.meegid.2020.104428
Zhang, L., Hao, C., Yao, S., Tang, R., Guo, W., Cong, H., et al. (2018). Exosomal miRNA Profiling to Identify Nanoparticle Phagocytic Mechanisms. Small 14 (15), e1704008. doi:10.1002/smll.201704008
Zhang, Z., Wang, Q., Chen, F., and Liu, J. (2015b). Elevated Expression of HMGA1 Correlates with the Malignant Status and Prognosis of Non-small Cell Lung Cancer. Tumour Biol. 36 (2), 1213–1219. doi:10.1007/s13277-014-2749-4
Zhao, W., Bao, P., Qi, H., and You, H. (2010). Resveratrol Down-Regulates Survivin and Induces Apoptosis in Human Multidrug-Resistant SPC-A-1/CDDP Cells. Oncol. Rep. 23 (1), 279–286.
Zhong, W., Yan, T., Webber, M. M., and Oberley, T. D. (2004). Alteration of Cellular Phenotype and Responses to Oxidative Stress by Manganese Superoxide Dismutase and a Superoxide Dismutase Mimic in RWPE-2 Human Prostate Adenocarcinoma Cells. Antioxid. Redox Signal. 6 (3), 513–522. doi:10.1089/152308604773934279
Zhou, J., Yang, Q., Lu, L., Tuo, Z., Shou, Z., and Cheng, J. (2021). PLK1 Inhibition Induces Immunogenic Cell Death and Enhances Immunity against NSCLC. Int. J. Med. Sci. 18 (15), 3516–3525. doi:10.7150/ijms.60135
Zhou, P., Yang, X. L., Wang, X. G., Hu, B., Zhang, L., Zhang, W., et al. (2020). A Pneumonia Outbreak Associated with a New Coronavirus of Probable Bat Origin. Nature 579 (7798), 270–273. doi:10.1038/s41586-020-2012-7
Zhou, X., Zhang, Z., and Liang, X. (2020). Regulatory Network Analysis to Reveal Important miRNAs and Genes in Non-small Cell Lung Cancer. Cell J 21 (4), 459–466. doi:10.22074/cellj.2020.6281
Zhuang, Z., Chen, Q., Huang, C., Wen, J., Huang, H., and Liu, Z. (2020). A Comprehensive Network Pharmacology-Based Strategy to Investigate Multiple Mechanisms of HeChan Tablet on Lung Cancer. Evid. Based Complement. Alternat. Med. 2020, 7658342. doi:10.1155/2020/7658342
Glossary
AURKB Aurora kinase B
BIRC5 Baculoviral IAP repeat-containing 5
BP Biological processes
BUB1B BUB1 mitotic checkpoint serine/threonine kinase B
CC Cellular components
CCNA2 Cyclin A2
CCNB2 Cyclin B2
CDC20 Cell division cycle 20
CENPA Centromere protein A
ChEA3 ChIP-X Enrichment Analysis 3
COVID-19 Coronavirus disease-2019
CTD Comparative Toxicogenomics Database
DEGs Differentially expressed genes
DLGAP5 DLG-associated protein 5
DNMT1 DNA methyltransferase 1
DPFG Drug Perturbations from GEO
DSigDB Drug Signatures Database
FoxO Forkhead box, sub-group O
GEO Gene Expression Omnibus
GO Gene Ontology
IFN Interferon
IL Interleukin
JAK STAT Janus kinase/signal transducer and activator of transcription
KEGG Kyoto Encyclopedia of Genes and Genomes
MERS-CoV Middle East respiratory syndrome coronavirus
MF Molecular functions
MYBL2 MYB proto-oncogene-like 2
NSCLC Non-small-cell lung cancer ACE2 angiotensin-converting enzyme 2
OMIM Online Mendelian Inheritance in Man
PLK1 Polo-like kinase 1
PPI Protein–protein interaction
RNA-seq Transcriptomic RNA-sequencing
SARS-CoV-2 Severe acute respiratory syndrome coronavirus 2
SASP Senescence-associated secretory phenotype
TCGA The Cancer Genome Atlas
TFDP1 Transcription factor Dp-1
TFs Transcription factors
TOP2A DNA topoisomerase II alpha
TTD Therapeutic Target Database
ZNF367 Zinc finger protein 367
Keywords: COVID-19, non-small-cell lung cancer, interaction network, bioinformatic analysis, systemic biological analysis
Citation: Zhuang Z, Zhong X, Chen Q, Chen H and Liu Z (2022) Bioinformatics and System Biology Approach to Reveal the Interaction Network and the Therapeutic Implications for Non-Small Cell Lung Cancer Patients With COVID-19. Front. Pharmacol. 13:857730. doi: 10.3389/fphar.2022.857730
Received: 19 January 2022; Accepted: 28 March 2022;
Published: 02 June 2022.
Edited by:
Francesca Caccuri, University of Brescia, ItalyReviewed by:
Ettore Mosca, National Research Council, ItalySucheendra K. Palaniappan, The Systems Biology Institute, Japan
Copyright © 2022 Zhuang, Zhong, Chen, Chen and Liu. This is an open-access article distributed under the terms of the Creative Commons Attribution License (CC BY). The use, distribution or reproduction in other forums is permitted, provided the original author(s) and the copyright owner(s) are credited and that the original publication in this journal is cited, in accordance with accepted academic practice. No use, distribution or reproduction is permitted which does not comply with these terms.
*Correspondence: Zhanhua Liu, lzh065@163.com