- Molecular Modelling and Drug Design Lab, Institute for Drug Research and Fraunhofer Project Center for Drug Discovery and Delivery, Faculty of Medicine, The Hebrew University of Jerusalem, Jerusalem, Israel
In recent years, the cannabinoid type 2 receptor (CB2R) has become a major target for treating many disease conditions. The old therapeutic paradigm of “one disease-one target-one drug” is being transformed to “complex disease-many targets-one drug.” Multitargeting, therefore, attracts much attention as a promising approach. We thus focus on designing single multitargeting agents (MTAs), which have many advantages over combined therapies. Using our ligand-based approach, the “Iterative Stochastic Elimination” (ISE) algorithm, we produce activity models of agonists and antagonists for desired therapeutic targets and anti-targets. These models are used for sequential virtual screening and scoring large libraries of molecules in order to pick top-scored candidates for testing in vitro and in vivo. In this study, we built activity models for CB2R and other targets for combinations that could be used for several indications. Those additional targets are the cannabinoid 1 receptor (CB1R), peroxisome proliferator-activated receptor gamma (PPARγ), and 5-Hydroxytryptamine receptor 4 (5-HT4R). All these models have high statistical parameters and are reliable. Many more CB2R/CBIR agonists were found than combined CB2R agonists with CB1R antagonist activity (by 200 fold). CB2R agonism combined with PPARγ or 5-HT4R agonist activity may be used for treating Inflammatory Bowel Disease (IBD). Combining CB2R agonism with 5-HT4R generates more candidates (14,008) than combining CB2R agonism with agonists for the nuclear receptor PPARγ (374 candidates) from an initial set of ∼2.1 million molecules. Improved enrichment of true vs. false positives may be achieved by requiring a better ISE score cutoff or by performing docking. Those candidates can be purchased and tested experimentally to validate their activity. Further, we performed docking to CB2R structures and found lower statistical performance of the docking (“structure-based”) compared to ISE modeling (“ligand-based”). Therefore, ISE modeling may be a better starting point for molecular discovery than docking.
1 Introduction
The cannabinoid receptors (CBRs) consist of cannabinoid receptors 1 (CB1R) and 2 (CB2R), which are members of the lipid class A G protein-coupled receptors (GPCRs) family. The CBRs participate in many physiological processes, including mood regulation, cognitive function, neuroprotection, nociception, cell growth and proliferation, appetite, and lipid metabolism (Stasiulewicz et al., 2020). Both are expressed in the central nervous system (CNS) and in peripheral tissues. CB2Rs have lower expression levels than CB1Rs in the CNS and are primarily expressed in immune cells (Wu, 2019). Their different expression regions in the brain suggest a neuroprotective role of CB2R, avoiding CB1R mediated side-effects (Deng et al., 2015). Moreover, CB2R expression can be upregulated in the brain under some pathological conditions (e.g., addiction, inflammation, anxiety), suggesting CB2R involvement in various psychiatric and neurological disorders (Wu, 2019).
In the brain, CB2R is proposed as a potential target for attenuating inflammation associated with neurodegenerative diseases (Alzheimer’s disease (AD), Parkinson’s disease (PD), and others) (Cassano et al., 2017; Bie et al., 2018; Kelly et al., 2020; Mecha et al., 2020). Several selective CB2R agonists exhibited analgesic activity in preclinical models of acute inflammatory, chronic, and neuropathic pain (Murineddu et al., 2013; Soliman et al., 2021). Its role is also investigated in mental disorders like schizophrenia, depression, anxiety, and addictions (García-Gutiérrez et al., 2010; García-Gutiérrez and Manzanares, 2010; Ortega-Alvaro et al., 2011; ZX et al., 2011; Jordan and Xi, 2019; ME et al., 2019). Other potential therapeutic areas of CB2Rs were explored: anti-cancer (Guzmán, 2003; Fernández-Ruiz et al., 2007), epilepsy (Ji et al., 2021), osteoporosis (Idris et al., 2005; Rossi et al., 2011), atopic dermatitis (Maekawa et al., 2006), (NCT00697710), ischemia/reperfusion injury (Bátkai et al., 2007; Rajesh et al., 2007), atherosclerosis (Mach et al., 2008), gastrointestinal inflammation (Wright et al., 2008) and disorders of reproduction (Maccarrone, 2008).
In the past 2 decades, treating multifactorial illnesses, i.e., infections, cancer, and CNS disorders, shifted towards multitargeting (Csermely et al., 2005; Hopkins et al., 2006; Boran and Iyengar, 2010; L.; Bolognesi, 2013; Bolognesi and Cavalli, 2016; Zhou et al., 2019). Simultaneous modulation of multiple targets may have better efficacy and safety profile than single targeted drugs, and the number of multitargeting new molecular entities is increasing over the years (Ramsay et al., 2018). The design of multitargeting agents (MTAs) assigns desired therapeutic targets and avoids targets associated with side effects (“anti targets”). In principle, MTA can be a single compound or a combination of compounds, each directed to a different target (“cocktails” or as a co-formulated drug-device), and both are used in the clinic. Despite the highly significant therapeutic relevance of combinatorial therapy (Conway and Cohen, 2010; Morphy, 2010; Wright, 2010; Modi et al., 2011; Lu et al., 2012), single MTA has substantial advantages over combination therapy: 1) more predictable pharmacokinetic profile 2) avoiding drug-drug interactions 3) easier dose regimen and higher compliance 4) enabling to overcome mutations in relevant diseases such as cancer, viral and bacterial ailments 5) simultaneous presence of the molecule in tissues where it is expected to affect and 6) an easier regulatory process (Hopkins, 2008; Anighoro et al., 2014).
Targets from different protein superfamilies may challenge the design of such MTAs, lacking shared/similar ligands or common structural motifs, which are sometimes the cause of side-effects (Morphy et al., 2004). Therefore such different targets may be of more interest. Nevertheless, single MTAs have been discovered (Ryckmans et al., 2002; Natesan Murugesan et al., 2004; Omar et al., 2018).
The broad involvement of CB2R in various disorders makes it a valuable target for multitargeting therapies while combining its modulation with affecting other relevant proteins in each disease. Several studies proposed its combination with other targets such as acetylcholinesterase (AChE) and butyrylcholinesterase for AD (Gonzalez-Naranjo et al., 2013; Dolles et al., 2016, 2018; González-Naranjo et al., 2019). Suggestions were also raised to find dual CB2R/histone deacetylases and CB2R/σ receptor compounds for treating cancer and neurodegenerative diseases (Mangiatordi et al., 2020), and to develop multitargeting analgesics (Maione et al., 2013). Here we shall focus on several possibilities of multitargeting CB2R with other targets.
1.1 Combined Effects of CB2 and CB1 Receptors
The CBRs play a critical role in several human physiological and pathological conditions. However, the CNS side effects of CB1R ligands may limit the therapeutic use of such agents if they cross the Blood-Brain Barrier (BBB). That is the case of the CB1R inverse agonists Rimonabant and Taranabant (Moreira and Crippa, 2009; Martín-García et al., 2010). To overcome the central effects, peripheral CB1R antagonists were developed (Chorvat, 2013; El-Atawneh et al., 2019; Quarta and Cota, 2020). Another option is to develop pure antagonists (An et al., 2020; Stasiulewicz et al., 2020). Agonists of the CBRs may be used to treat anxiety (Stasiulewicz et al., 2020) or as analgesics, anti-inflammatory, neuroprotective and anti-emetic compounds (An et al., 2020). Peripheral CB1R antagonists combined with CB2R agonists may be used for treating liver diseases (Mallat et al., 2011) and diabetic complications (Gruden et al., 2016). This dual activity may be useful in treating obesity, abolishing diabetes-induced albuminuria, inflammation, tubular injury, and renal fibrosis (Barutta et al., 2017). Combining CB1R antagonism with CB2R agonism in the brain is shown to have a synergistic effect on reward processing (Gobira et al., 2019). Another option is to design selective CB2R agonists to benefit from their nociception and neuroinflammation role without psychoactive effects (Hollinshead et al., 2013; Verty et al., 2015; Poleszak et al., 2020). CB2R selective agonists are investigated to treat pain, inflammation, arthritis, addictions, cancer besides their neuroprotective role (An et al., 2020).
1.2 Combined Effects at CB2R, PPARγ, and 5-HT4R
CB2R could be targeted with other receptors to attenuate inflammation for several autoimmune and inflammatory conditions. The peroxisome proliferator-activated receptor (PPAR)-γ is a nuclear receptor that plays a crucial role in regulating lipid metabolism and glucose homeostasis. It associated with metabolic disorders, such as atherosclerosis, obesity, metabolic syndrome, dyslipidemias, type 2 diabetes, and cancer (Decara et al., 2020). PPARγ agonists have been shown to prevent inflammation, dermal fibrosis, and lipoatrophy in preclinical models of systemic sclerosis (SSc) (Wei et al., 2010). SSc is an orphan autoimmune multi-organic disease that affects the connective tissue. Dual CB2/PPARγ agonists such as VCE-004.8 and JBT-101 (Ajulemic acid, Lenabasum) have alleviated skin fibrosis and inflammation in SSc models (Rio et al., 2018; García-Martín et al., 2019). JBT-101 is in clinical trials for SSc (NCT03398837), dermatomyositis (NCT03813160), and cystic fibrosis (NCT02465450). Additionally, PPARγ agonists can suppress the pro-inflammatory cytokines associated with chronic diseases such as Inflammatory Bowel Disease (IBD).
IBD, including ulcerative colitis (UC) and Crohn’s disease (CD), has been considered one of the most prevalent GI diseases with accelerating incidence in newly industrialized countries. Yet it lacks effective drug targets and medications (Seyedian et al., 2019). As a lifelong disease, therapy aims to induce remission in the short term and maintain remission in the long term. New drugs have diverse mechanisms of action, targeting mainly the inflammation pathways. The current anti-inflammatory small molecules used to treat IBD are associated with several side effects (5-amino salicylate and its prodrugs such as Olsalazine and Balsalazide), with more severe toxicity (Azathioprine, Mercaptopurine, Methotrexate) or with known long term negative impacts of steroid hormones (glucocorticoids). Biological drugs are expensive, require more intensive medical attention in a clinic or at home (self-injections), and, in the case of TNFalpha antibodies, elicit resistance by immune system response (Torres et al., 2020). Although the mechanism by which PPARγ acts on the pathogenesis of IBD has not been clarified (Decara et al., 2020), natural and chemical PPARγ ligands have ameliorated the fibrotic process in preliminary clinical trials and experimental models of intestinal fibrosis (Vetuschi et al., 2018). Moreover, many studies showed the anti-inflammatory role of PPARγ activation in intestinal tissues in UC and CD (Decara et al., 2020).
Recent investigations suggest that serotonin (5-HT) can influence the development and severity of inflammation within the gut, particularly in the setting of IBD. 5-HT influences every major function inherent to the gut, including motility, secretion, blood flow, and sensation (Coates et al., 2017). Alterations in its receptor activity in disease conditions may result in many problematic symptoms, including abdominal pain, diarrhea, or constipation (Coates et al., 2017). The 5-HT4 receptor (5-HT4R) mediates enteric neuron survival and neurogenesis of adult mice (Liu et al., 2009). It promotes the reconstruction of an enteric neural circuit leading to the recovery of the defecation reflex in the distal gut (Matsuyoshi et al., 2010). 5-HT4R activation maintains motility in healthy colons of mice and guinea pigs and reduces inflammation in colons of mice with colitis (Spohn et al., 2016). PPARγ and 5-HT4R agonists may be combined with CB2R as a potential therapy for IBD (Turcotte et al., 2016). A peripheral CB2R agonist (Olorinab) reached phase II trials for abdominal pain in CD (NCT03155945) and irritable bowel syndrome (NCT04043455).
1.3 Multitargeting in Silico
Computational methods allow us to examine options for designing or discovering multitargeting candidates in a reliable, fast, and low-cost manner (Sliwoski et al., 2014; Zhang et al., 2017). Screening candidates for binding against several targets to find single MTA differs from designing compounds based on conjugated pharmacophores by merging/fusing/linking molecules (Morphy and Rankovic, 2005; Zhou et al., 2019), which could take longer to synthesize and might increase the molecular weight and affect the drug-likeness properties.
Our research combines ligand and structure-based methods. Our algorithm for solving complex combinatorial problems, the 'Iterative stochastic elimination algorithm’ (ISE) (Stern and Goldblum, 2014; El-Atawneh and Goldblum, 2017), has been applied in recent years to molecular discovery (Zatsepin et al., 2016; Da’adoosh et al., 2019; El-Atawneh et al., 2019), including one example of multitargeting modeling: modeling the properties of molecules that may be remotely loaded to nanoliposomes and the properties that enable them to be stable inside the nanoliposomes, in a biological fluid (Cern et al., 2017). Molecules that had high scores in both loading and stability models were chosen. For any discovery of MTAs, virtual screening (VS) by separate ligand-based models is performed in sequential order.
After finding top candidate ligands, it is helpful to examine the structural aspects, since our classifications are based on physicochemical properties and not on structural elements. Molecules with similar properties might have different structures and sizes. Thus, we dock the top candidates to the target protein if such a structure has been reported. Structures of CB2R were deposited recently in the Protein Data Bank (PDB), one with a bound antagonist (PDB code 5ZTY) (Li et al., 2019) and the other with an agonist (PDB code 6KPC) (Hua et al., 2020), which makes structure-based design feasible (Tuccinardi et al., 2006; Cichero et al., 2011). CB2R shares 44% sequence identity and 68% similarity with CB1R in the transmembrane regions (Munro et al., 1993). The antagonist-binding pockets in both receptors are quite distinct, while the agonist-binding pockets in CB1R and CB2R, including side-chain rotamers, of the key residues involved in ligands interactions are almost identical (Li et al., 2019; Hua et al., 2020), which might be the source of cross-reactivity between their ligands and difficulty in attaining selectivity. There are also CB1R and PPARγ structures, with agonists and antagonists in both. Yet, there is no published atomic-level structure of 5-HT4R, but ligand-based modeling for 5-HT4R with ISE is possible due to its many known ligands.
2 Methods
2.1 Data Sets
2.1.1 Learning\Training Sets
Compounds with reported activity, agonists (EC50 values) and antagonists (ki or IC50 values) at the different receptors were taken from the ChEMBL database (http://www.ebi.ac.uk/ChEMBLdb/) (Bento et al., 2014). Duplicates were removed based on their simplified molecular input line entry specification (SMILES notation). Molecules with undefined potency values, error comments, and a confidence score below seven (reported at ChEMBL) were excluded, as well as molecules that are active above 100 µM. The active molecules were diluted with random molecules assumed to be inactive (“decoys”) with a ratio of 1:100 (active: inactive) (Tropsha, 2010). Randoms were picked from the ZINC database (Sterling and Irwin, 2015), based on the “applicability domain” (APD) of the actives (Netzeva et al., 2005). The application of APD for picking randoms imposes to discover differences between active and inactive molecules with some basic similarities, thus making the task of classification more difficult. We apply APD by selecting random molecules for which the values of molecular weight (MW), calculated lipophilic character (clogP), hydrogen bond acceptors (HBA), and hydrogen bond donors (HBD) are within the average ± two standard deviations for these variables of the active molecules.
2.1.2 Screening Set
The Enamine HTS Collection (Enamine HTS Collection 2021), consisting of 2,159,632 compounds was used for VS in both ligand and structure-based methods.
2.2 Datasets Preparation
All molecules were prepared by the “Molecular Database Wash” (v. 2011.10) (Molecular Operating Environment, 2021). This includes hydrogen adjustment, removing minor components, determining the protonation state, enumeration of ionization states, and tautomer forms. Mutagenic and reactive molecules (based on calculated descriptors by MOE) were removed from the learning sets.
2.3 Descriptors Calculation
The standard descriptors we calculated for building the models are the 2-dimensional (2D) descriptors by QuaSAR- MOE (v.2011.10) with 186 descriptors. The complete descriptors list is given at (http://www.cadaster.eu/sites/cadaster.eu/files/challenge/descr.htm). Descriptors with low variance (Smialowski et al., 2010), or highly correlated descriptors (Pearson correlation coefficient > 0.9), were excluded, using the Knime platform (v. 4.0.1) (Berthold et al., 2008) to exclude out of two highly correlated descriptors the one which has greater similarity to other descriptors. We have also tested the performance of 3D descriptors for CB2R (see results and discussion).
2.4 Activity Models Constructed by the Iterative Stochastic Elimination Algorithm
Our generic ISE algorithm has been applied to many problems related to drug discovery and has been presented in reviews, with details of the mathematical and statistical criteria to distinguish between two activities based on physicochemical properties (descriptors) of known active vs. inactive compounds (Stern and Goldblum, 2014; El-Atawneh and Goldblum, 2017). For each model, five cross-validations were performed (James et al., 2013), with 4 out of the five-folds producing the model, and the fifth fold was used as a test set. We include some of the main details of model construction and screening in Supplementary Data section 1.1.
2.5 Tanimoto Fingerprint Similarity
The “Atom-pair” fingerprints for the active molecules were generated using RDKit toolkit (RDKit, 2018) (in Knime platform v. 4.0.1) (Berthold et al., 2008). The “Tanimoto similarity coefficient” (Tc) for the fingerprints is based on the CDK toolkit.
2.6 Docking
The two structures of CB2R were downloaded from the PDB (5ZTY (Li et al., 2019) and 6KPC (Hua et al., 2020)), and prepared by the “Protein Preparation Wizard” (Schrödinger Suit 2019-3) (Madhavi Sastry et al., 2013). For 5ZTY, we allowed C-OH rotations of SER90, THR114, TYR190; for 6KPC, we allowed such rotations of TYR25, SER90, THR114, TYR190, and SER285 for the grid construction. Alanine (ALA) scan was performed to assign the critical residues in the binding site of the two structures for 23 residues detected by PDBsum (Laskowski, 2009). The screened molecules were prepared using “LigPrep” (Schrödinger Release, 2018), with default settings, except the chirality option that was set to “Generate all combinations” for the Enamine database (5,024,833 entries were generated). Molecular docking was performed with Glide HTVS and SP (Richard A. Friesner et al., 2006).
In the docking analysis, we examined the geometric character of binding by requiring the docked molecules to be in contact with residues that were found to be “hot spots” by performing a virtual ALA scan.
3 Results
3.1 Ligand-Based Approach
3.1.1 Iterative Stochastic Elimination Algorithm Activity Models
We constructed several models for each target based on the relevant molecular activity reported by ChEMBL. There are molecules reported as partial agonists and inverse agonists for the CB2R (access date: January/2016), and those were excluded from the present study. Some models were constructed with a subset of highly active molecules (i.e., activity values less than 5 nM or 10 nM) from the larger set of reported activities. We choose the best-performing model based on Matthews Correlation Coefficient (MCC, Supplementary Data S1.1) (Matthews, 1975), Area under the ROC curve (AUC), and the Enrichment Factor (EF, Supplementary Data S1.1) (Table 1). Only ten molecules were reported with IC50 activity for 5-HT4R (access date: December/2017), so we used the reported Ki values for constructing the antagonist models (reported for 227 molecules). For PPARγ (access date: February/2018) and 5-HT4R agonist models, we built only one model based on the available data. The PPARγ antagonist models (access date: October/2021) have similar performance, and we chose the Ki model because it has a better EF value. All models have good mean MCC values > 0.65, AUC > 0.9, and EF values vary from 12 to 71 with a positive (> 0.0) index cutoff. The learning sets’ similarity is low for all chosen models (average Tc ≤ 0.5, Supplementary Table S1).
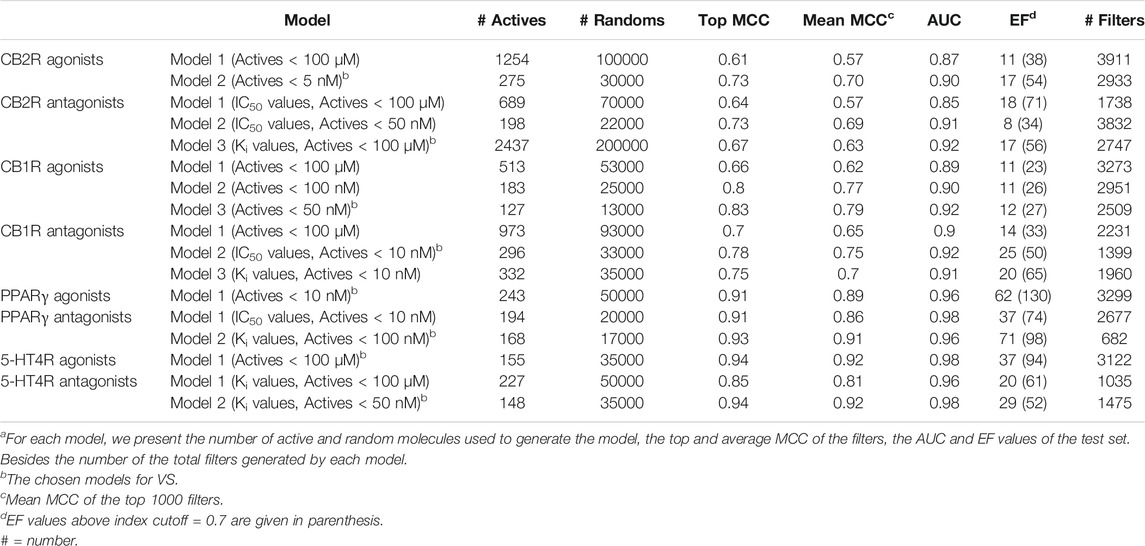
TABLE 1. Models of agonists and antagonists for the four receptorsa.
All constructed Models are presented in Table 1. The models used for screening are marked. Models constructed on the basis of active molecules with highest affinity (Nanmolar range) have better statistical parameters than those constructed on the basis of 100 µM activities, and were thus used for screening. That is the case of CB2R/CB1R/PPARγ agonists and antagonists, and 5HT4R antagonists. Only a single model of actives with lesser activity, of 5HT4R agonists, was used for screening. However the number of molecules with lesser affinity among the 155 used for modeling is small: only 5 molecules have EC50 values between 1 and 100 µM. Also, the 5HT4R model for agonists is the one with best statistical parameters compared to all other GPCR models for actives up to 100 µM.
3.1.1.1 Performance of 3D Descriptors
Taking the learning set of the chosen 2D-based CB2R agonist model (Model 2- with 275 active molecules < 5 nM diluted with 30,000 randoms), we built 3D and the 2D/3D combined descriptors’ based models. The ISE agonist model based on 2D descriptors performed better than the 3D, and the 2D/3D combined descriptors by MCC, AUC, and EF (Supplementary Table S2). The 3D model has a lower mean MCC (0.5) and AUC (0.85) than the combined 2D/3D model.
3.2 Multitargeting Candidates
To find multitargeting candidates for the different indications, we performed hierarchical VS. First, focusing on the CBRs, we screened the Enamine database (DB) through the different CBR activity models, considering desired activity, i.e., of CB2R agonists, and the unwanted activity as anti targets. Molecules with a positive index pass the model, and those with a negative score are considered to fail. We found 241,260 CB2 selective agonists (about 11% of the dataset); those molecules passed the CB2R agonist model and did not pass the CB2R antagonist model. They also did not pass the CB1R agonist and antagonist models. Adding the CB1R agonists or antagonists to CB2 agonists, we found many less candidates (63,735 and 324, respectively), as shown in Figure 1. Raising the index cutoff above 0.0 reduces these numbers.
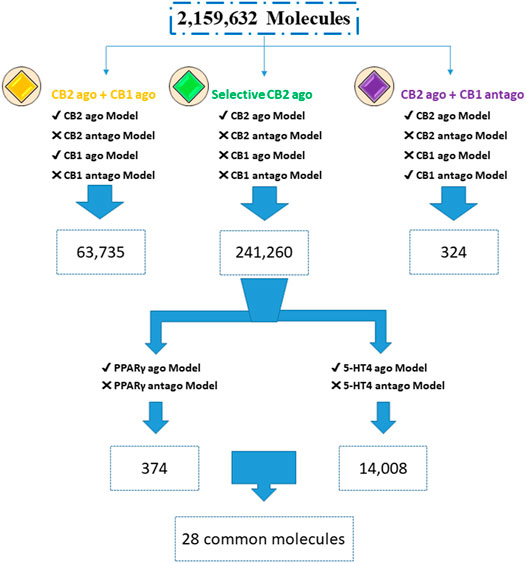
FIGURE 1. Screening for multitargeted candidates. Enamine database (2,159,632 compounds) was screened through agonist (ago) and antagonist (antago) ISE models. Numbers are of molecules with a positive index for models with a “✓” symbol, while failing to pass the models is marked by “X” (due to a negative index).
Looking for additional activities of the selective CB2R agonists, we screened those 241,260 candidates through the PPARγ and 5-HT4R agonist models (Figure 1). To avoid anti-targets we screened the same set by the antagonist models of PPARγ and 5-HT4R. This yielded 374 CB2R and PPARγ agonists, and 14,008 candidates for CB2R and 5-HT4R agonism with no antagonism at any of the three receptors. We found 28 candidate agonists for simultaneously hitting all the three targets of CB2R, PPARγ, and 5-HT4R. All the mentioned hit sets are internally diverse, as well as being diverse (by Tanimoto criteria) towards the actives used for model construction: comparisons yield a low average Tanimoto coefficient of Tc ≤ 0.4 (Supplementary Table S3).
3.2.1 Common Substructures for the Multitargeting Hits
Common substructures could be used to explain why molecules are candidates for binding and activating different receptors. We examined that possibility for each multitargeting set. To perform that task, we used Canvas (v. 4.2.012, Schrödinger Suit 2019-4) to find the maximum common substructure. In Figure 2, we display the major common substructures for five different groups: agonists of all three receptors, CB2R/PPARγ, CB2R/5-HT4R as well as CB2R/CB1R agonists and CB2Ragonists/CB1R antagonists. A larger scope of common substructures is presented in Supplementary Figure S1.
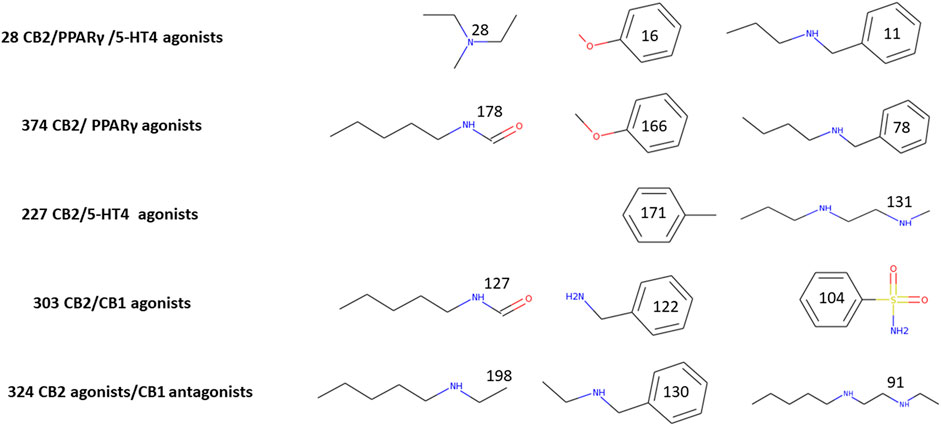
FIGURE 2. Major common multitargeted substructures. The numbers on each substructure indicate the number of molecules that include it. We chose 303 top candidates (with index score >0.7) for assigning substructures to CB2R/CB1R agonists and 227 top candidates (Index > 0.7) for the substructures of CB2/5-HT4 agonists. Other substructures were assigned for sets with an index > 0.0.
Figure 2 presents major substructure elements of top multitargeted screened molecules. It is easy to detect some of the fragments which appear in more than 20% of each multitargeted group: tertiary and secondary amines, benzylamine, anisol, alkyl chains with amines or amide, and benzenesulfonamide. It is noteworthy that all the 28 CB2R/PPARγ/5-HT4R multitargeted candidates have a tertiary amine moiety, which is not abundant in either CB2R/PPARγ or CB2R/5-HT4R. Two fragments of CB2R/PPARγ—anisol and N-butylbenzylamine contribute to the triple multitargeting, while the only fragment of the CB2R/5-HT4R in the triple target is a phenyl ring. All three structures common to CB2R agonists/CB1R antagonists are secondary amines. Only a single secondary amine is among the main fragments of CB2R/CB1R agonists, and the two others are an aromatic sulfonamide and an amide of N-pentylamine.
3.3 Structure-Based Confirmation of CB2R Ligands
The structures of CB2R (6KPC (Hua et al., 2020) with an agonist and 5ZTY (Li et al., 2019) with an antagonist) have similar binding pockets and binding residues (Li et al., 2019; Hua et al., 2020) (Supplementary Table S4). Similarity is also observed between the CB2R and CB1R binding pockets (Li et al., 2019). This creates an obstacle to distinguishing between agonist and antagonist activity for the CB2R if we consider docking alone. We examined the binding residues in both structures by applying a virtual ALA scan (Schrödinger Suit 2019-3) (Madhavi Sastry et al., 2013) for 23 residues in the binding site (Supplementary Table S4). AM12033 (6KPC- CB2R agonist) has 19 interactions, mainly with hydrophobic and aromatic residues and 3 H-bonds, with LEU 182 and SER285. AM10257 (5ZTY- CB2R antagonist) has 16 interactions with no H-bonds (as shown in PDBsum (Laskowski, 2009)).
The calculated stability for the 23 residues (by virtual ALA scan) does not differ dramatically between 6KPC and 5ZTY. The considered contacts in the 6KPC agonist structure in order to suggest more successful docked ligands are: hydrogen bonding with LEU182 and SER285, and Van der Waals (VDW) interactions with the following: TYR25, PHE87, PHE91, PHE94, ILE110, PHE183, TYR190, LEU191, TRP194, LEU262, MET265, PHE281.
3.3.1 Docking Validation
To choose one out of the two structures for detecting agonists and/or antagonists of CB2R, we constructed similar grids for the docking region in both structures, 6KPC and 5ZTY. We then redocked the ligands in both structures and performed cross-docking between the two. For 6KPC, the agonist, AM12033, got a better docking score (−12.2 kcal/mol) than the antagonist AM10257 (−8.7 kcal/mol). However, in 5ZTY, both agonist and antagonist got similar docking scores (−9.8 and −10.8 kcal/mol, respectively). The redocked positions of the agonist and antagonist are shown in Figure 3.
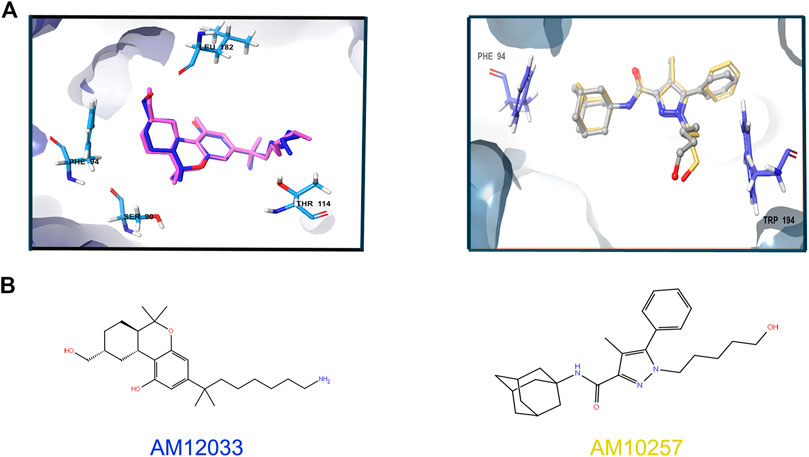
FIGURE 3. Superimposition of the redocked ligands at 6KPC and 5ZTY. (A) Left: relevant residues at 6KPC are shown in azure sticks (SER90, PHE94, LEU182, THR114 and LEU182). The redocked agonist (AM12033, docking score = −12.2 kcal/mol)—blue aligned with the original ligand (pink), with RMSD = 0.94. Right: relevant residues (PHE94 and TRP194) at 5ZTY are shown in blue sticks. The redocked antagonist (AM10257, docking score = −10.8 kcal/mol)—yellow aligned with the original ligand (gray), with RMSD = 1.5. (B) 2D representation of the agonist and antagonist ligands.
To further examine the binding of ligands to both structures, we docked overall 23 known ligands of CB2R and of CB1R with different selectivities (Supplementary Table S5) (An et al., 2020). Docking scores are not correlated with experimental Ki values (An et al., 2020) in Supplementary Table S5. Detailed interactions with binding site residues for the 19 ligands that passed docking to the 6KPC structure are listed in Supplementary Table S6. None of the interactions can be related to a specific activity. This is also seen in Supplementary Figure S2, where the best-docked ligand of each activity type is compared to the 6KPC ligand (AM12033). Finally, we screened the learning set of the CB2R agonist modeling (275 active molecules and 30,000 randoms), resulting in a very low AUC for docking to both 6KPC and 5ZTY: 0.45 and 0.44, respectively. The ISE model, however, got an AUC of 0.9. Due to the success in redocking an agonist, and the need for discovering agonists, we continued all docking experiments with 6KPC.
3.4 Virtual Screening: Ligand-Based vs. Structure-Based Methods
We compared ligand (ISE) and structure-based (docking) methods by performing VS of the Enamine DB (2,159,632 compounds) for CB2R. ISE screening is extremely fast compared to docking (Figure 4). A positive index in screening by the CB2R agonist model was assigned to 241,260 molecules. We pick molecules with higher indexes and better EF values to improve the quality of our candidates, thus resulting in fewer molecules. For example, with a high index cutoff ≥ 0.7, 41,102 molecules pass, and the EF equals 54. That EF is only 17 at a lower index cutoff >0.0 (for 241,260 molecules). Docking was applied to the ISE candidates with a positive index: SP docking to the 6KPC structure found 238,718 molecules with docking scores of 6.6 to −12.8 kcal/mol. Filtration was based on docking scores ≤ −9 kcal/mol and hydrogen bonds with LEU182 and SER285, to a final set of 131 candidates.
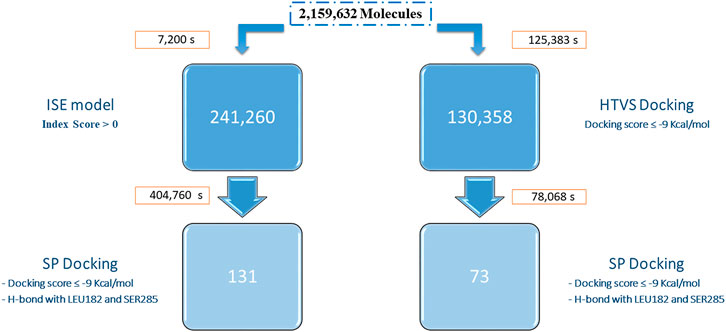
FIGURE 4. Workflows of VS of the Enamine database by ISE (left) and by docking (right). The screening times (in seconds) and the number of candidates are indicated for each step. The SP docking for the ISE hits was performed for 857,546 entries (generated by ligprep from the 241,260 candidates). The docking protocol (HTVS, on the right) was performed for 5,026,503 entries (generated by ligprep). Only 130,358 molecules passed the score filtration, and those continued to SP docking.
Docking to CB2R was performed in two stages with the same 6KPC structure. First, HTVS docking was executed for the whole Enamine DB. The docked poses have a docking score range from 10.4 to −12.5 kcal/mol. Molecules with docking scores of less than −9 kcal/mol were further docked by the SP protocol (130,358 molecules). Most of these molecules (130,080) passed SP with a 5.7 to −12.9 kcal/mol docking score. By picking those with a score better than −9 kcal/mol and hydrogen bonds with LEU182 and SER285, only 73 molecules remain. Ten out of the 73 docking hits have positive ISE index scores. Only nine molecules are shared between the two SP screenings. Both sets are diverse from the known active CB2R agonists, and from each other (average Tc ∼0.3).
4 Discussion
The CBRs exert many physiological functions and are thus considered valuable therapeutic targets. CB2R, in particular, gains more attention due to its protective actions, involved in many pathological conditions such as cancer, CNS disorders, and a variety of disorders in the cardiovascular, gastrointestinal, and reproductive systems (Pacher and Mechoulam, 2011), while being devoid of psychoactive effects associated with the CB1R central activation. Finding single multitargeting agents (Morphy et al., 2004; Morphy and Rankovic, 2005; Zhang et al., 2017) for CB2R combined with other targets such as CB1R, PPARγ, and the 5-HT4R is not a trivial endeavor but one worth pursuing. Searching by virtual screening may suggest candidates in a shorter time than by in vitro screening and allows to test vast numbers of compounds. Our approach is to begin by constructing models for the binding or function of molecules at specific targets based on previously published results (“ligand-based” modeling). Our main tool for modeling is our ISE algorithm. The number of molecules for each model should not be less than a few dozens. Multitargeting requires to construct models for each of the relevant targets and anti-targets. If these models are of good quality, they may be used for VS, scoring, and sorting millions of molecules in a short time.
Here we present activity models built by the ISE algorithm for agonists and antagonists at each target. All models are statistically valid and should be useful (Table 1). The algorithm generates filters based on the ranges of physicochemical properties (computed) of known active molecules and randoms. Those filters are used for scoring by VS. It is noteworthy that the PPARγ and 5-HT4R models perform better than the models of CBRs. Their active sets are more similar (by Tc) than those of the CBRs, as shown in Supplementary Table S1. With an average Tc∼0.5, these sets of agonists may still be considered to be diverse. For VS, we use filters with top MCC values up to 20% below the maximal value or just the best 1,000 filters.
Choosing between 2D and 3D descriptors depends on the problem we want to solve. Even though 3D descriptors are more representative, they don’t yield better results, as have been studied in a large number and diverse range of applications over the past decades (Ekins et al., 2007). Some studies have shown that combining 2D and 3D molecular descriptors may improve models’ performance (Yera et al., 2011; Kombo et al., 2013). But for the CB2R agonist model, both the 3D-based and combined 2D/3D models have lower performance than the 2D-based model as shown in section 3.1.1.1.
Screening through ISE models was performed to find MTAs for several target combinations which reflect different indications (Figure 1). First, we screened through CBR models, which are involved in many pathological disorders. CB2R selective agonists have neuroprotective and anti-inflammatory effects (An et al., 2020). It is possible to reduce the number of molecules by increasing the cutoff index above 0.0. The higher that index, there will be less molecules to test further—but the enrichment factor, with more “true positives” will be greater. By performing SP docking of 241,260 molecules, subsequent to ISE modeling, we got 131 candidates (Figure 4). We got more candidates when combining CB2R agonists with CB1R agonist activity (63,735) rather than with CB1R antagonist activity 324) (Figure 1). That may be due to the high degree of structural similarity in the orthosteric binding pockets between agonist-bound CB2R and CB1R structures (Shahbazi et al., 2020).
Combining CB2R ligands that are active at CB1R might elicit central side effects associated with the CB1R. Therefore, it is important to limit CB1R activity to the periphery and avoid central activities, either agonistic or antagonistic. By applying criteria for peripheral action of CB1R ligands, it is possible to combine with CB2R ligands, particularly the combination of CB2R agonists/CB1R antagonists. Those candidates may be tested for multiple metabolic disorders, such as obesity and renal fibrosis (Barutta et al., 2017).
4.1 Some Implications of Ligand-Based Multitargeting
Multitargeting by ISE could be based on molecules with known activities on two or more targets. One publication mentions the construction of such a database, but it is not accessible (Chen et al., 2017). It is highly unlikely that enough molecules will be found to enable ISE modeling. Therefore, in the main spirit of ISE, each “variable” (in that case, a target, with many ligands as its “values”) requires separate model construction. Screening and scoring through any single model reduce the molecular library size by 10-fold or more. In HTS, it is common to discover 1 out of 1,000 molecules tested for activity. However, that is a real activity in vitro, while we only suggest candidates for in vitro testing, which may include false positives. Therefore their numbers are much larger.
As we add more targets and anti-targets, the number of candidates decreases: we found, among our ∼2.1 million screened molecules, only 374 candidates for combined (simultaneous) CB2R and PPARγ agonism, which may be tested for SSc (Wei et al., 2010), dermatomyositis, cystic fibrosis, and IBD (Decara et al., 2020). Adding 5-HT4R agonists reduces that number to 28, while CB2R and 5-HT4R agonists that could be valuable for IBD have 14,008 candidates. The much larger number of shared molecules that could hit CB2R and 5-HT4R (compared to sharing between CB2R and PPARγ) reflects the fact that both are aminergic GPCRs of the A family with 27% sequence similarity, as calculated by blastp (McGinnis and Madden, 2004), and may have a greater chance for ligand cross-reactivity (Yang et al., 2021). PPARγ belongs to a different family of cytoplasmic nuclear receptors. Moreover, only 60 molecules are shared between PPARγ and 5-HT4R agonists (without screening through CB2R models).
Screening by ISE models has already succeeded in achieving “scaffold hopping” (Zatsepin et al., 2016; Da’adoosh et al., 2019; El-Atawneh et al., 2019) due to the use of physicochemical properties rather than of structures. Even in those cases of greater similarity among the actives (agonists of PPARγ (0.52) and of 5-HT4R (0.5), Supplementary Table S1), the top screened candidates are varied among themselves, i.e., Tc = 0.4 for the 28 multitargeted agonists of CB2R/PPARγ/5-HT4R. That is also the case of screened molecules vs. actives in the learning sets (all results in Supplementary Table S3).
The main substructure elements presented in Figure 2 may help to understand how it is possible that a single molecule binds to different binding sites: the amine moieties—frequently two amines in a molecule—are singly charged, and the first protonation reduces the pKa of the other amine. Amine protonation prevails in four out of the five multitargeted sets, except for CB2R/CB1R agonists in which a negative charge on the oxygen of the amides may have a leading role. It is also clear from the difference between the coupling of CB2R agonists with either CB1R agonists or antagonists, that it is possible to separate between these multitarget pairs. It would still be impossible to suggest a synthesis of multitargeted compounds based on these major fragments, but it is easy to pick molecules that contain these fragments for each multitargeted alternative by requiring to include these substructures with their statistical weight as in Figure 2 or even better, as in Supplementary Figure S1. None of these moieties resemble the structures of known cannabinoid ligands (classical, non-classical, amino-alkylindoles, and those with the eicosanoid group).
4.2 The Impact of Structure-Based Modeling
Structures of CB2R have been recently deposited in the PDB (Li et al., 2019; Hua et al., 2020) and enable to perform structure-based studies—docking, pharmacophore, and molecular dynamics. The similarity between CB2R agonist/antagonist complexes and CB1R and CB2R structures make it challenging to design ligands with high selectivity (Hua et al., 2020). Docking is considered a time-consuming approach, as shown in Figure 4. Screening by docking has been shown to be much less reliable statistically than our ligand-based approach for CB2R agonism. Our ISE models screen molecules based on their properties and not on structural elements. That may result in top screened molecules having similar properties but different sizes and volumes, which may or may not be accommodated by the targets. Some of these molecules might not fit into binding sites and will be rejected. The results of our CB2R modeling confirm our preferable sequence of actions: ligand-based modeling should be followed by structure-based testing, which is better than structure-based docking alone.
Virtual ALA scan was used in this and other of our studies for picking “hot spots”—the main residues that contribute to the binding of smaller or larger ligands (i.e., including protein-protein interactions). Those “hot spots” determine the region of the grids for screening by docking and provide the initial geometric criteria that are applied prior to considering the docking scores. In ALA scan, we replace a larger side chain (of 18 amino acids, except for GLY and ALA) with a shorter one. We do not however apply any minimization or dynamics to that change, which positions a methyl group in the Cβ position, with tetrahedral angles vis-à-vis Cα, in place of a longer side chain, leaving some “void”. No other side chain position is modified around the virtually mutated one. This protocol is due to our wish to discover molecules that replace an existing ligand/protein with an exact similar conformation of side chains in the protein target, as in the PDB, in order to promote competition. That is clearly not the case with genetically mutated ALA scan. In that in vitro experiment, other side chains could change their conformations in the vicinity and more remote from the ALA mutated position. In vitro ALA scan may even change conformations of the main protein chain. Therefore, it is rewarding if mutagenesis studies support some of our results such as for PHE87, PHE91, PHE94, HIS95 (Li et al., 2019), and TRP194 (Zhang et al., 2011). TYR190 mutation to Ile resulted in a loss of ligand recognition and function (McAllister et al., 2002).
This is a theoretical study, which includes statistics (AUC, EF) that clarify what are the chances for discovering multitargeted actives. Naturally, the next step is to pick top candidates from each set for biochemical experiments. Our multitargeting results also suggest which multitargeting sets have a greater chance to be experimentally confirmed. Previously, we published our theoretical predictions and experimental validations of the binding of 8 molecules out of 15 predicted candidates (picked by ISE modeling from a library of 1.8 million) (El-Atawneh et al., 2019). Finally, only in vitro testing of candidates predicted by each method in silico will confirm or refute the VS results conducted by ISE and docking approaches.
Data Availability Statement
The original contributions presented in the study are included in the article/Supplementary Material, further inquiries can be directed to the corresponding author.
Author Contributions
SE-A performed the research and wrote the first draft of this article. AG revised the article and developed the ISE algorithm.
Conflict of Interest
The authors declare that the research was conducted in the absence of any commercial or financial relationships that could be construed as a potential conflict of interest.
Publisher’s Note
All claims expressed in this article are solely those of the authors and do not necessarily represent those of their affiliated organizations, or those of the publisher, the editors, and the reviewers. Any product that may be evaluated in this article, or claim that may be made by its manufacturer, is not guaranteed or endorsed by the publisher.
Supplementary Material
The Supplementary Material for this article can be found online at: https://www.frontiersin.org/articles/10.3389/fphar.2022.812745/full#supplementary-material
References
An, D., Peigneur, S., Hendrickx, L. A., and Tytgat, J. (2020). Targeting Cannabinoid Receptors: Current Status and Prospects of Natural Products. Int. J. Mol. Sci. 21 (21), 5064. doi:10.3390/IJMS21145064
Anighoro, A., Bajorath, J., and Rastelli, G. (2014). Polypharmacology: Challenges and Opportunities in Drug Discovery. J. Med. Chem. 57, 7874–7887. doi:10.1021/jm5006463
Barutta, F., Grimaldi, S., Gambino, R., Vemuri, K., Makriyannis, A., Annaratone, L., et al. (2017). Dual Therapy Targeting the Endocannabinoid System Prevents Experimental Diabetic Nephropathy. Nephrol. Dial. Transpl. 32, 1655–1665. doi:10.1093/ndt/gfx010
Bátkai, S., Osei-Hyiaman, D., Pan, H., El-Assal, O., Rajesh, M., Mukhopadhyay, P., et al. (2007). Cannabinoid-2 Receptor Mediates protection against Hepatic Ischemia/reperfusion Injury. FASEB J. 21, 1788–1800. doi:10.1096/fj.06-7451com
Bento, A. P., Gaulton, A., Hersey, A., Bellis, L. J., Chambers, J., Davies, M., et al. (2014). The ChEMBL Bioactivity Database: an Update. Nucleic Acids Res. 42, D1083–D1090. doi:10.1093/nar/gkt1031
Berthold, M. R., Cebron, N., Dill, F., Gabriel, T. R., Kötter, T., Meinl, T., et al. (2008). KNIME: The Konstanz Information Miner. Berlin, Heidelberg: Springer, 319–326. doi:10.1007/978-3-540-78246-9_38
Bie, B., Wu, J., Foss, J. F., and Naguib, M. (2018). An Overview of the Cannabinoid Type 2 Receptor System and its Therapeutic Potential. Curr. Opin. Anaesthesiol. 31, 407–414. doi:10.1097/ACO.0000000000000616
Bolognesi, M. L., and Cavalli, A. (2016). Multitarget Drug Discovery and Polypharmacology. ChemMedChem 11, 1190–1192. doi:10.1002/CMDC.201600161
Bolognesi, M. L. (2013). Polypharmacology in a Single Drug: Multitarget Drugs. Curr. Med. Chem. 20, 1639–1645. doi:10.2174/0929867311320130004
Boran, A. D., and Iyengar, R. (2010). Systems Approaches to Polypharmacology and Drug Discovery. Curr. Opin. Drug Discov. Devel. 13, 297–309.
Cassano, T., Calcagnini, S., Pace, L., De Marco, F., Romano, A., and Gaetani, S. (2017). Cannabinoid Receptor 2 Signaling in Neurodegenerative Disorders: From Pathogenesis to a Promising Therapeutic Target. Front. Neurosci. 11, 30. doi:10.3389/FNINS.2017.00030
Cern, A., Marcus, D., Tropsha, A., Barenholz, Y., and Goldblum, A. (2017). New Drug Candidates for Liposomal Delivery Identified by Computer Modeling of Liposomes' Remote Loading and Leakage. J. Control Release 252, 18–27. doi:10.1016/j.jconrel.2017.02.015
Chen, C., Wu, M., Cen, S., Wu, J., and Zhou, J. (2017). MTLD, a Database of Multiple Target Ligands, the Updated Version. Molecules 22. doi:10.3390/molecules22091375
Chorvat, R. J. (2013). Peripherally Restricted CB1 Receptor Blockers. Bioorg. Med. Chem. Lett. 23, 4751–4760. doi:10.1016/j.bmcl.2013.06.066
Cichero, E., Ligresti, A., Allarà, M., Di Marzo, V., Lazzati, Z., D'Ursi, P., et al. (2011). Homology Modeling in Tandem with 3D-QSAR Analyses: A Computational Approach to Depict the Agonist Binding Site of the Human CB2 Receptor. Eur. J. Med. Chem. 46, 4489–4505. doi:10.1016/J.EJMECH.2011.07.023
Coates, M. D., Tekin, I., Vrana, K. E., and Mawe, G. M. (2017). Review Article: the many Potential Roles of Intestinal Serotonin (5-hydroxytryptamine, 5-HT) Signalling in Inflammatory Bowel Disease. Aliment. Pharmacol. Ther. 46, 569–580. doi:10.1111/APT.14226
Conway, D., and Cohen, J. A. (2010). Combination Therapy in Multiple Sclerosis. Lancet Neurol. 9, 299–308. doi:10.1016/S1474-4422(10)70007-7
Csermely, P., Agoston, V., Pongor, S., Ágoston, V., and Pongor, S. (2005). The Efficiency of Multi-Target Drugs: the Network Approach Might Help Drug Design. Trends Pharmacol. Sci. 26, 178–182. doi:10.1016/j.tips.2005.02.007
Da'adoosh, B., Marcus, D., Rayan, A., King, F., Che, J., and Goldblum, A. (2019). Discovering Highly Selective and Diverse PPAR-delta Agonists by Ligand Based Machine Learning and Structural Modeling. Sci. Rep. 9, 1106. doi:10.1038/s41598-019-38508-8
Decara, J., Rivera, P., López-Gambero, A. J., Serrano, A., Pavón, F. J., Baixeras, E., et al. (2020). Peroxisome Proliferator-Activated Receptors: Experimental Targeting for the Treatment of Inflammatory Bowel Diseases. Front. Pharmacol. 11, 730. doi:10.3389/FPHAR.2020.00730
Del Rio, C., Cantarero, I., Palomares, B., Gómez-Cañas, M., Fernández-Ruiz, J., Pavicic, C., et al. (2018). VCE-004.3, a Cannabidiol Aminoquinone Derivative, Prevents Bleomycin-Induced Skin Fibrosis and Inflammation through PPARγ- and CB2 Receptor-dependent Pathways. Br. J. Pharmacol. 175, 3813–3831. doi:10.1111/BPH.14450
Deng, L., Guindon, J., Cornett, B. L., Makriyannis, A., Mackie, K., and Hohmann, A. G. (2015). Chronic Cannabinoid Receptor 2 Activation Reverses Paclitaxel Neuropathy without Tolerance or Cannabinoid Receptor 1-dependent Withdrawal. Biol. Psychiatry 77, 475–487. doi:10.1016/J.BIOPSYCH.2014.04.009
Dolles, D., Hoffmann, M., Gunesch, S., Marinelli, O., Möller, J., Santoni, G., et al. (2018). Structure-Activity Relationships and Computational Investigations into the Development of Potent and Balanced Dual-Acting Butyrylcholinesterase Inhibitors and Human Cannabinoid Receptor 2 Ligands with Pro-cognitive In Vivo Profiles. J. Med. Chem. 61, 1646–1663. doi:10.1021/ACS.JMEDCHEM.7B01760
Dolles, D., Nimczick, M., Scheiner, M., Ramler, J., Stadtmüller, P., Sawatzky, E., et al. (2016). Aminobenzimidazoles and Structural Isomers as Templates for Dual-Acting Butyrylcholinesterase Inhibitors and hCB2 R Ligands to Combat Neurodegenerative Disorders. ChemMedChem 11, 1270–1283. doi:10.1002/CMDC.201500418
Ekins, S., Mestres, J., and Testa, B. (2007). In Silico pharmacology for Drug Discovery: Methods for Virtual Ligand Screening and Profiling. Br. J. Pharmacol. 152, 9–20. doi:10.1038/sj.bjp.0707305
El-Atawneh, S., Hirsch, S., Hadar, R., Tam, J., and Goldblum, A. (2019). Prediction and Experimental Confirmation of Novel Peripheral Cannabinoid-1 Receptor Antagonists. J. Chem. Inf. Model. 59, 3996–4006. doi:10.1021/acs.jcim.9b00577
El-Atawneh, S., and Goldblum, A. (2017). Iterative Stochastic Elimination for Discovering Hits and Leads. Chim. Oggi-chemistry TODAY 35, 41–46.
Enamine HTS Collection (2021). HTS Collection. Available at: https://enamine.net/ (Accessed December 15, 2021).
Fernández-Ruiz, J., Romero, J., Velasco, G., Tolón, R. M., Ramos, J. A., and Guzmán, M. (2007). Cannabinoid CB2 Receptor: a New Target for Controlling Neural Cell Survival? Trends Pharmacol. Sci. 28, 39–45. doi:10.1016/J.TIPS.2006.11.001
Friesner, R. A., Murphy, R. B., Repasky, M. P., Frye, L. L., Greenwood, J. R., Halgren, T. A., et al. (2006). Extra Precision glide: Docking and Scoring Incorporating a Model of Hydrophobic Enclosure for Protein-Ligand Complexes. J. Med. Chem. 49 (21), 6177–6196. doi:10.1021/JM051256O
García-Gutiérrez, M., Pérez-Ortiz, J., Gutiérrez-Adán, A., and Manzanares, J. (2010). Depression-resistant Endophenotype in Mice Overexpressing Cannabinoid CB2 Receptors. Br. J. Pharmacol. 160, 1773–1784. doi:10.1111/J.1476-5381.2010.00819.X
García-Gutiérrez, M. S., and Manzanares, J. (2010). Overexpression of CB2 Cannabinoid Receptors Decreased Vulnerability to Anxiety and Impaired Anxiolytic Action of Alprazolam in Mice. J. Psychopharmacol. 25, 111–120. doi:10.1177/0269881110379507
García-Martín, A., Garrido-Rodríguez, M., Navarrete, C., Caprioglio, D., Palomares, B., DeMesa, J., et al. (2019). Cannabinoid Derivatives Acting as Dual PPARγ/CB2 Agonists as Therapeutic Agents for Systemic Sclerosis. Biochem. Pharmacol. 163, 321–334. doi:10.1016/J.BCP.2019.02.029
Gobira, P. H., Oliveira, A. C., Gomes, J. S., da Silveira, V. T., Asth, L., Bastos, J. R., et al. (2019). Opposing Roles of CB1 and CB2 Cannabinoid Receptors in the Stimulant and Rewarding Effects of Cocaine. Br. J. Pharmacol. 176, 1541–1551. doi:10.1111/BPH.14473
González-Naranjo, P., Campillo, N. E., Páez, J. A., and Paez, A. (2013). Multitarget Cannabinoids as Novel Strategy for Alzheimer Disease. Curr. Alzheimer Res. 10, 229–239. doi:10.2174/1567205011310030002
González-Naranjo, P., Pérez-Macias, N., Pérez, C., Roca, C., Vaca, G., Girón, R., et al. (2019). Indazolylketones as New Multitarget Cannabinoid Drugs. Eur. J. Med. Chem. 166, 90–107. doi:10.1016/J.EJMECH.2019.01.030
Gruden, G., Barutta, F., Kunos, G., and Pacher, P. (2016). Role of the Endocannabinoid System in Diabetes and Diabetic Complications. Br. J. Pharmacol. 173, 1116–1127. doi:10.1111/BPH.13226
Guzmán, M. (2003). Cannabinoids: Potential Anticancer Agents. Nat. Rev. Cancer 3, 745–755. doi:10.1038/nrc1188
Hollinshead, S. P., Tidwell, M. W., Palmer, J., Guidetti, R., Sanderson, A., Johnson, M. P., et al. (2013). Selective Cannabinoid Receptor Type 2 (CB2) Agonists: Optimization of a Series of Purines Leading to the Identification of a Clinical Candidate for the Treatment of Osteoarthritic Pain. J. Med. Chem. 56, 5722–5733. doi:10.1021/JM400305D
Hopkins, A. L., Mason, J. S., and Overington, J. P. (2006). Can We Rationally Design Promiscuous Drugs? Curr. Opin. Struct. Biol. 16, 127–136. doi:10.1016/j.sbi.2006.01.013
Hopkins, A. L. (2008). Network Pharmacology: the Next Paradigm in Drug Discovery. Nat. Chem. Biol. 4, 682–690. doi:10.1038/nchembio.118
Hua, T., Li, X., Wu, L., Iliopoulos-Tsoutsouvas, C., Wang, Y., Wu, M., et al. (2020). Activation and Signaling Mechanism Revealed by Cannabinoid Receptor-Gi Complex Structures. Cell 180, 655–e18. e18. doi:10.1016/J.CELL.2020.01.008
Idris, A. I., van 't Hof, R. J., Greig, I. R., Ridge, S. A., Baker, D., Ross, R. A., et al. (2005). Regulation of Bone Mass, Bone Loss and Osteoclast Activity by Cannabinoid Receptors. Nat. Med. 11, 774–779. doi:10.1038/nm1255
James, G., Witten, D., Hastie, T., and Tibshirani, R. (2013). An Introduction to Statistical Learning with Applications in R. Springer, 1–14. doi:10.1007/978-1-4614-7138-7_1
Ji, X., Zeng, Y., and Wu, J. (2021). The CB2 Receptor as a Novel Therapeutic Target for Epilepsy Treatment. Int. J. Mol. Sci. 22, 8961. doi:10.3390/IJMS22168961
Jordan, C. J., and Xi, Z. X. (2019). Progress in Brain Cannabinoid CB2 Receptor Research: From Genes to Behavior. Neurosci. Biobehav. Rev. 98, 208–220. doi:10.1016/J.NEUBIOREV.2018.12.026
Kelly, R., Joers, V., Tansey, M. G., McKernan, D. P., and Dowd, E. (2020). Microglial Phenotypes and Their Relationship to the Cannabinoid System: Therapeutic Implications for Parkinson's Disease. Molecules 25, 453. doi:10.3390/MOLECULES25030453
Kombo, D. C., Tallapragada, K., Jain, R., Chewning, J., Mazurov, A. A., Speake, J. D., et al. (2013). 3D Molecular Descriptors Important for Clinical success. J. Chem. Inf. Model. 53, 327–342. doi:10.1021/CI300445E/SUPPL_FILE/CI300445E_SI_005.PDF
Laskowski, R. A. (2009). PDBsum New Things. Nucleic Acids Res. 37, D355–D359. doi:10.1093/nar/gkn860
Li, X., Hua, T., Vemuri, K., Ho, J. H., Wu, Y., Wu, L., et al. (2019). Crystal Structure of the Human Cannabinoid Receptor CB2. Cell 176, 459–e13. e13. doi:10.1016/j.cell.2018.12.011
Liu, M. T., Kuan, Y. H., Wang, J., Hen, R., and Gershon, M. D. (2009). 5-HT4 Receptor-Mediated Neuroprotection and Neurogenesis in the Enteric Nervous System of Adult Mice. J. Neurosci. 29, 9683–9699. doi:10.1523/JNEUROSCI.1145-09.2009
Lu, X., Xiao, L., Wang, L., and Ruden, D. M. (2012). Hsp90 Inhibitors and Drug Resistance in Cancer: the Potential Benefits of Combination Therapies of Hsp90 Inhibitors and Other Anti-cancer Drugs. Biochem. Pharmacol. 83, 995–1004. doi:10.1016/j.bcp.2011.11.011
Maccarrone, M. (2008). CB2 Receptors in Reproduction. Br. J. Pharmacol. 153, 189–198. doi:10.1038/sj.bjp.0707444
Mach, F., Montecucco, F., and Steffens, S. (2008). Cannabinoid Receptors in Acute and Chronic Complications of Atherosclerosis. Br. J. Pharmacol. 153, 290–298. doi:10.1038/sj.bjp.0707517
Maekawa, T., Nojima, H., Kuraishi, Y., and Aisaka, K. (2006). The Cannabinoid CB2 Receptor Inverse Agonist JTE-907 Suppresses Spontaneous Itch-Associated Responses of NC Mice, a Model of Atopic Dermatitis. Eur. J. Pharmacol. 542, 179–183. doi:10.1016/J.EJPHAR.2006.05.040
Maione, S., Costa, B., and Di Marzo, V. (2013). Endocannabinoids: A Unique Opportunity to Develop Multitarget Analgesics. Pain 154, S87–S93. doi:10.1016/J.PAIN.2013.03.023
Mallat, A., Teixeira-Clerc, F., Deveaux, V., Manin, S., and Lotersztajn, S. (2011). The Endocannabinoid System as a Key Mediator during Liver Diseases: New Insights and Therapeutic Openings. Br. J. Pharmacol. 163, 1432–1440. doi:10.1111/J.1476-5381.2011.01397.X
Mangiatordi, G. F., Intranuovo, F., Delre, P., Abatematteo, F. S., Abate, C., Niso, M., et al. (2020). Cannabinoid Receptor Subtype 2 (CB2R) in a Multitarget Approach: Perspective of an Innovative Strategy in Cancer and Neurodegeneration. J. Med. Chem. 63, 14448–14469. doi:10.1021/ACS.JMEDCHEM.0C01357
Martín-García, E., Burokas, A., Martín, M., Berrendero, F., Rubí, B., Kiesselbach, C., et al. (2010). Central and Peripheral Consequences of the Chronic Blockade of CB1cannabinoid Receptor with Rimonabant or Taranabant. J. Neurochem. 112, 1338–13351. doi:10.1111/J.1471-4159.2009.06549.X
Matsuyoshi, H., Kuniyasu, H., Okumura, M., Misawa, H., Katsui, R., Zhang, G. X., et al. (2010). A 5-Ht(4)-Receptor Activation-Induced Neural Plasticity Enhances In Vivo Reconstructs of Enteric Nerve Circuit Insult. Neurogastroenterol. Motil. 22, 806–e226. doi:10.1111/J.1365-2982.2010.01474.X
Matthews, B. W. (1975). Comparison of the Predicted and Observed Secondary Structure of T4 Phage Lysozyme. Biochim. Biophys. Acta 405, 442–451. doi:10.1016/0005-2795(75)90109-9
McAllister, S. D., Tao, Q., Barnett-Norris, J., Buehner, K., Hurst, D. P., Guarnieri, F., et al. (2002). A Critical Role for a Tyrosine Residue in the Cannabinoid Receptors for Ligand Recognition. Biochem. Pharmacol. 63, 2121–2136. doi:10.1016/S0006-2952(02)01031-6
McGinnis, S., and Madden, T. L. (2004). BLAST: at the Core of a Powerful and Diverse Set of Sequence Analysis Tools. Nucleic Acids Res. 32, W20–W25. doi:10.1093/NAR/GKH435
Mecha, M., Carrillo-Salinas, F. J., Feliú, A., Mestre, L., and Guaza, C. (2020). Perspectives on Cannabis-Based Therapy of Multiple Sclerosis: A Mini-Review. Front. Cell. Neurosci. 14, 34. doi:10.3389/FNCEL.2020.00034
Modi, S., Stopeck, A., Linden, H., Solit, D., Chandarlapaty, S., Rosen, N., et al. (2011). HSP90 Inhibition Is Effective in Breast Cancer: a Phase II Trial of Tanespimycin (17-AAG) Plus Trastuzumab in Patients with HER2-Positive Metastatic Breast Cancer Progressing on Trastuzumab. Clin. Cancer Res. 17, 5132–5139. doi:10.1158/1078-0432.CCR-11-0072
Molecular Operating Environment (MOE) (2021). Molecular Operating Environment. Montreal, QC, Canada: Chemical Computing Group Inc.
Moreira, F. A., and Crippa, J. A. (2009). The Psychiatric Side-Effects of Rimonabant. Braz. J. Psychiatry 31, 145–153. doi:10.1590/S1516-44462009000200012
Morphy, R., Kay, C., and Rankovic, Z. (2004). From Magic Bullets to Designed Multiple Ligands. Drug Discov. Today 9, 641–651. doi:10.1016/S1359-6446(04)03163-0
Morphy, R., and Rankovic, Z. (2005). Designed Multiple Ligands. An Emerging Drug Discovery Paradigm. J. Med. Chem. 48, 6523–6543. doi:10.1021/jm058225d
Morphy, R. (2010). Selectively Nonselective Kinase Inhibition: Striking the Right Balance. J. Med. Chem. 53, 1413–1437. doi:10.1021/jm901132v
Munro, S., Thomas, K. L., and Abu-Shaar, M. (1993). Molecular Characterization of a Peripheral Receptor for Cannabinoids. Nature 365, 61–65. doi:10.1038/365061a0
Murineddu, G., Deligia, F., Dore, A., Pinna, G., Asproni, B., and Pinna, G. A. (2013). Different Classes of CB2 Ligands Potentially Useful in the Treatment of Pain. Recent Pat. CNS Drug Discov. 8, 42–69. doi:10.2174/15748898112079990016
Murugesan, N., Gu, Z., Fadnis, L., Tellew, J. E., Baska, R. A., Yang, Y., et al. (2004). Dual Angiotensin II and Endothelin A Receptor Antagonists: Synthesis of 2'-substituted N-3-Isoxazolyl Biphenylsulfonamides with Improved Potency and Pharmacokinetics. J. Med. Chem. 48, 171–179. doi:10.1021/JM049548X
Netzeva, T. I., Worth, A., Aldenberg, T., Benigni, R., Cronin, M. T., Gramatica, P., et al. (2005). Current Status of Methods for Defining the Applicability Domain of (Quantitative) Structure-Activity Relationships. The Report and Recommendations of ECVAM Workshop 52. Altern. Lab. Anim. 33, 155–173. doi:10.1177/026119290503300209
Omar, Y. M., Abdu-Allah, H. H. M., and Abdel-Moty, S. G. (2018). Synthesis, Biological Evaluation and Docking Study of 1,3,4-Thiadiazole-Thiazolidinone Hybrids as Anti-inflammatory Agents with Dual Inhibition of COX-2 and 15-LOX. Bioorg. Chem. 80, 461–471. doi:10.1016/J.BIOORG.2018.06.036
Ortega-Alvaro, A., Aracil-Fernández, A., García-Gutiérrez, M. S., Navarrete, F., and Manzanares, J. (2011). Deletion of CB2 Cannabinoid Receptor Induces Schizophrenia-Related Behaviors in Mice. Neuropsychopharmacology 36, 1489–1504. doi:10.1038/npp.2011.34
Pacher, P., and Mechoulam, R. (2011). Is Lipid Signaling through Cannabinoid 2 Receptors Part of a Protective System? Prog. Lipid Res. 50, 193–211. doi:10.1016/J.PLIPRES.2011.01.001
Poleszak, E., Wośko, S., Sławińska, K., Wyska, E., Szopa, A., Sobczyński, J., et al. (2020). Ligands of the CB2 Cannabinoid Receptors Augment Activity of the Conventional Antidepressant Drugs in the Behavioural Tests in Mice. Behav. Brain Res. 378, 112297. doi:10.1016/J.BBR.2019.112297
Quarta, C., and Cota, D. (2020). Anti-obesity Therapy with Peripheral CB1 Blockers: from Promise to Safe(?) Practice. Int. J. Obes. (Lond) 44, 2179–2193. doi:10.1038/s41366-020-0577-8
Rajesh, M., Pan, H., Mukhopadhyay, P., Bátkai, S., Osei-Hyiaman, D., Haskó, G., et al. (2007). Cannabinoid-2 Receptor Agonist HU-308 Protects against Hepatic Ischemia/reperfusion Injury by Attenuating Oxidative Stress, Inflammatory Response, and Apoptosis. J. Leukoc. Biol. 82, 1382–1389. doi:10.1189/jlb.0307180
Ramsay, R. R., Popovic-Nikolic, M. R., Nikolic, K., Uliassi, E., and Bolognesi, M. L. (2018). A Perspective on Multi-Target Drug Discovery and Design for Complex Diseases. Clin. Transl. Med. 7, 3. doi:10.1186/s40169-017-0181-2
RDKit (2018). Open-Source Cheminformatics Software. Available at: http://www.rdkit.org/ (Accessed March 27, 2018).
Rossi, F., Bellini, G., Luongo, L., Torella, M., Mancusi, S., De Petrocellis, L., et al. (2011). The Endovanilloid/endocannabinoid System: A New Potential Target for Osteoporosis Therapy. Bone 48, 997–1007. doi:10.1016/J.BONE.2011.01.001
Ryckmans, T., Balançon, L., Berton, O., Genicot, C., Lamberty, Y., Lallemand, B., et al. (2002). First Dual NK(1) Antagonists-Serotonin Reuptake Inhibitors: Synthesis and SAR of a New Class of Potential Antidepressants. Bioorg. Med. Chem. Lett. 12, 261–264. doi:10.1016/S0960-894X(01)00727-2
Sastry, G. M., Adzhigirey, M., Day, T., Annabhimoju, R., and Sherman, W. (2013). Protein and Ligand Preparation: Parameters, Protocols, and Influence on Virtual Screening Enrichments. J. Comput. Aided. Mol. Des. 27, 221–234. doi:10.1007/s10822-013-9644-8
Schrödinger Release (2018). Schrödinger Release 2018-2. New York, NY: LigPrep, Schrödinger, LLCLigPrep.
Seyedian, S. S., Nokhostin, F., and Malamir, M. D. (2019). A Review of the Diagnosis, Prevention, and Treatment Methods of Inflammatory Bowel Disease. J. Med. Life 12, 113–122. doi:10.25122/JML-2018-0075
Shahbazi, F., Grandi, V., Banerjee, A., and Trant, J. F. (2020). Cannabinoids and Cannabinoid Receptors: The Story So Far. iScience 23, 101301. doi:10.1016/J.ISCI.2020.101301
Sliwoski, G., Kothiwale, S., Meiler, J., and Lowe, E. W. (2014). Computational Methods in Drug Discovery. Pharmacol. Rev. 66, 334–395. doi:10.1124/pr.112.007336
Sloan, M. E., Grant, C. W., Gowin, J. L., Ramchandani, V. A., and Le Foll, B. (2019). Endocannabinoid Signaling in Psychiatric Disorders: a Review of Positron Emission Tomography Studies. Acta Pharmacol. Sin. 40, 342–350. doi:10.1038/S41401-018-0081-Z
Smialowski, P., Frishman, D., and Kramer, S. (2010). Pitfalls of Supervised Feature Selection. Bioinformatics 26, 440–443. doi:10.1093/bioinformatics/btp621
Soliman, N., Haroutounian, S., Hohmann, A. G., Krane, E., Liao, J., Macleod, M., et al. (2021). Systematic Review and Meta-Analysis of Cannabinoids, Cannabis-Based Medicines, and Endocannabinoid System Modulators Tested for Antinociceptive Effects in Animal Models of Injury-Related or Pathological Persistent Pain. Pain 162, S26–S44. doi:10.1097/J.PAIN.0000000000002269
Spohn, S. N., Bianco, F., Scott, R. B., Keenan, C. M., Linton, A. A., O'Neill, C. H., et al. (2016). Protective Actions of Epithelial 5-Hydroxytryptamine 4 Receptors in Normal and Inflamed Colon. Gastroenterology 151, 933–e3. e3. doi:10.1053/J.GASTRO.2016.07.032
Stasiulewicz, A., Znajdek, K., Grudzień, M., Pawiński, T., and Sulkowska, A. J. I. (2020). A Guide to Targeting the Endocannabinoid System in Drug Design. Int. J. Mol. Sci. 21, 1. doi:10.3390/IJMS21082778
Sterling, T., and Irwin, J. J. (2015). ZINC 15--Ligand Discovery for Everyone. J. Chem. Inf. Model. 55, 2324–2337. doi:10.1021/acs.jcim.5b00559
Stern, N., and Goldblum, A. (2014). Iterative Stochastic Elimination for Solving Complex Combinatorial Problems in Drug Discovery. Isr. J. Chem. 54, 1338–1357. doi:10.1002/ijch.201400072
Torres, J., Bonovas, S., Doherty, G., Kucharzik, T., Gisbert, J. P., Raine, T., et al. (2020). ECCO Guidelines on Therapeutics in Crohn's Disease: Medical Treatment. J. Crohns Colitis 14, 4–22. doi:10.1093/ecco-jcc/jjz180
Tropsha, A. (2010). Best Practices for QSAR Model Development, Validation, and Exploitation. Mol. Inform. 29, 476–488. doi:10.1002/minf.201000061
Tuccinardi, T., Ferrarini, P. L., Manera, C., Ortore, G., Saccomanni, G., and Martinelli, A. (2006). Cannabinoid CB2/CB1 Selectivity. Receptor Modeling and Automated Docking Analysis. J. Med. Chem. 49, 984–994. doi:10.1021/JM050875U
Turcotte, C., Blanchet, M. R., Laviolette, M., and Flamand, N. (2016). The CB2 Receptor and its Role as a Regulator of Inflammation. Cell. Mol. Life Sci. 73, 4449–4470. doi:10.1007/s00018-016-2300-4
Verty, A. N., Stefanidis, A., McAinch, A. J., Hryciw, D. H., and Oldfield, B. (2015). Anti-Obesity Effect of the CB2 Receptor Agonist JWH-015 in Diet-Induced Obese Mice. PLoS One 10, e0140592. doi:10.1371/JOURNAL.PONE.0140592
Vetuschi, A., Pompili, S., Gaudio, E., Latella, G., and Sferra, R. (2018). PPAR-γ with its Anti-inflammatory and Anti-fibrotic Action Could Be an Effective Therapeutic Target in IBD. Eur. Rev. Med. Pharmacol. Sci. 22, 8839–8848. doi:10.26355/EURREV_201812_16652
Wei, J., Bhattacharyya, S., and Varga, J. (2010). Peroxisome Proliferator-Activated Receptor γ: Innate protection from Excessive Fibrogenesis and Potential Therapeutic Target in Systemic Sclerosis. Curr. Opin. Rheumatol. 22, 671–676. doi:10.1097/BOR.0B013E32833DE1A7
Wright, J. J. (2010). Combination Therapy of Bortezomib with Novel Targeted Agents: an Emerging Treatment Strategy. Clin. Cancer Res. 16, 4094–4104. doi:10.1158/1078-0432.CCR-09-2882
Wright, K. L., Duncan, M., and Sharkey, K. A. (2008). Cannabinoid CB2 Receptors in the Gastrointestinal Tract: a Regulatory System in States of Inflammation. Br. J. Pharmacol. 153, 263–270. doi:10.1038/sj.bjp.0707486
Wu, J. (2019). Cannabis, Cannabinoid Receptors, and Endocannabinoid System: Yesterday, Today, and Tomorrow. Acta Pharmacol. Sin. 40, 297–299. doi:10.1038/s41401-019-0210-3
Xi, Z.-X., Peng, X.-Q., Song, R., Zhang, H.-Y., Liu, Q.-R., Yang, H.-J., et al. (2011). Brain Cannabinoid CB2 Receptors Modulate Cocaine's Actions in Mice. Nat. Neurosci. 14, 1160–1166. doi:10.1038/NN.2874
Yang, D., Zhou, Q., Labroska, V., Qin, S., Darbalaei, S., Wu, Y., et al. (2021). G Protein-Coupled Receptors: Structure- and Function-Based Drug Discovery. Sig Transduct Target. Ther. 6, 1–27. doi:10.1038/s41392-020-00435-w
Yera, E. R., Cleves, A. E., and Jain, A. N. (2011). Chemical Structural Novelty: On-Targets and Off-Targets. J. Med. Chem. 54, 6771–6785. doi:10.1021/jm200666a
Zatsepin, M., Mattes, A., Rupp, S., Finkelmeier, D., Basu, A., Burger-Kentischer, A., et al. (2016). Computational Discovery and Experimental Confirmation of TLR9 Receptor Antagonist Leads. J. Chem. Inf. Model. 56, 1835–1846. doi:10.1021/acs.jcim.6b00070
Zhang, W., Pei, J., and Lai, L. (2017). Computational Multitarget Drug Design. J. Chem. Inf. Model. 57, 403–412. doi:10.1021/acs.jcim.6b00491
Zhang, Y., Xie, Z., Wang, L., Schreiter, B., Lazo, J. S., Gertsch, J., et al. (2011). Mutagenesis and Computer Modeling Studies of a GPCR Conserved Residue W5.43(194) in Ligand Recognition and Signal Transduction for CB2 Receptor. Int. Immunopharmacol. 11, 1303–1310. doi:10.1016/J.INTIMP.2011.04.013
Keywords: cannabinoid receptors 2 (CB2R), multitargeting, ISE, virtual screening, inflammation, neuroprotective, IBD—inflammatory bowel diseases
Citation: El-Atawneh S and Goldblum A (2022) Candidate Therapeutics by Screening for Multitargeting Ligands: Combining the CB2 Receptor With CB1, PPARγ and 5-HT4 Receptors. Front. Pharmacol. 13:812745. doi: 10.3389/fphar.2022.812745
Received: 10 November 2021; Accepted: 13 January 2022;
Published: 28 February 2022.
Edited by:
Frank Park, University of Tennessee Health Science Center (UTHSC), United StatesReviewed by:
Nadine Jagerovic, Spanish National Research Council (CSIC), SpainKirk Hevener, University of Tennessee Health Science Center (UTHSC), United States
Copyright © 2022 El-Atawneh and Goldblum. This is an open-access article distributed under the terms of the Creative Commons Attribution License (CC BY). The use, distribution or reproduction in other forums is permitted, provided the original author(s) and the copyright owner(s) are credited and that the original publication in this journal is cited, in accordance with accepted academic practice. No use, distribution or reproduction is permitted which does not comply with these terms.
*Correspondence: Amiram Goldblum, amiramg@ekmd.huji.ac.il