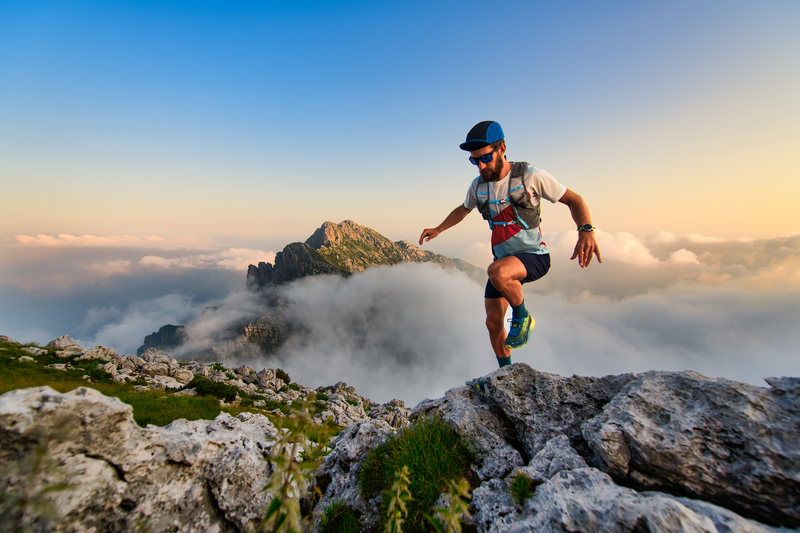
95% of researchers rate our articles as excellent or good
Learn more about the work of our research integrity team to safeguard the quality of each article we publish.
Find out more
ORIGINAL RESEARCH article
Front. Pharmacol. , 09 March 2022
Sec. Ethnopharmacology
Volume 13 - 2022 | https://doi.org/10.3389/fphar.2022.784242
Background: Traditional Chinese medicine (TCM) has been widely used in the treatment of human diseases. However, the synergistic effects of multiple TCM prescriptions in the treatment of stroke have not been thoroughly studied.
Objective of the study: This study aimed to reveal the mechanisms underlying the synergistic effects of these TCM prescriptions in stroke treatment and identify the active compounds.
Methods: Herbs and compounds in the Di-Tan Decoction (DTD), Xue-Fu Zhu-Yu Decoction (XFZYD), and Xiao-Xu-Ming Decoction (XXMD) were acquired from the TCMSP database. SEA, HitPick, and TargetNet web servers were used for target prediction. The compound-target (C-T) networks of three prescriptions were constructed and then filtered using the collaborative filtering algorithm. We combined KEGG enrichment analysis, molecular docking, and network analysis approaches to identify active compounds, followed by verification of these compounds with an oxygen-glucose deprivation and reoxygenation (OGD/R) model.
Results: The filtered DTD network contained 39 compounds and 534 targets, the filtered XFZYD network contained 40 compounds and 508 targets, and the filtered XXMD network contained 55 compounds and 599 targets. The filtered C-T networks retained approximately 80% of the biological functions of the original networks. Based on the enriched pathways, molecular docking, and network analysis results, we constructed a complex network containing 3 prescriptions, 14 botanical drugs, 26 compounds, 13 targets, and 5 pathways. By calculating the synergy score, we identified the top 5 candidate compounds. The experimental results showed that quercetin, baicalin, and ginsenoside Rg1 independently and synergistically increased cell viability.
Conclusion: By integrating pharmacological and chemoinformatic approaches, our study provides a new method for identifying the effective synergistic compounds of TCM prescriptions. The filtered compounds and their synergistic effects on stroke require further research.
Stroke is the second leading cause of death and disability worldwide and can be categorized into two subtypes: ischemic stroke (cerebral infarction) and hemorrhagic stroke (intracerebral hemorrhage and subarachnoid hemorrhage) (Collaborators, 2019). Within a very short time, approximately several hours after stroke, neurological damage needs to be minimized by removing blockages or hemorrhages with surgery and medications. After the most effective therapies are administered in the short term, there is still a lack of specific medicines for stroke. In the clinic, symptomatic and supportive medications, including neurotrophic medicines (e.g., edaravone) (Feng et al., 2011; Yang et al., 2011) and cytidine (Marti-Carvajal et al., 2020)); antiplatelet medicines (e.g., aspirin and clopidogrel (Johnston et al., 2018)); and anti-vasospasm medicines (e.g., nimodipine) (Carlson et al., 2020), are mainly used in stroke treatment. However, currently available therapies, especially medications, are still limited. Therefore, there is an urgent need for more effective treatment of post-stroke neurological damage.
Traditional Chinese medicine (TCM) has a long history of treating post-stroke neurological damage (Gong and Sucher, 1999). Di-Tan Decoction (DTD), Xue-Fu Zhu-Yu Decoction (XFZYD), and Xiao-Xu-Ming Decoction (XXMD) are three widely used classical prescriptions, and they cover the three most common syndromes in post-stroke patients complementarily, including phlegm obstruction, blood stasis, and Qi deficiency (Fu et al., 2013; Shi et al., 2013; Shen et al., 2015; Yang et al., 2020; Kwon et al., 2021). Previous studies have shown that DTD could promote metabolism and improve blood circulation by resolving phlegm (lei et al., 2020; Kwon et al., 2021), alleviating syncope, and improving movement and speech after stroke (Yan et al., 2017; Xue, 2020). XFZYD was reportedly the most representative prescription for removing blood stasis and promoting blood circulation in TCM prescriptions (Yi and Hongyan, 2018; Shao et al., 2020). It contained a variety of herbs with significant anticoagulation effects, which were important for improving the local blood supply and controlling the recurrence of thrombosis (Lee JJ. et al., 2011; Yi and Hongyan, 2018; Hongqin et al., 2019). XXMD reportedly promoted Qi to activate blood in TCM experience (Fu et al., 2013). It was usually used to treat systemic symptoms after stroke, including mouth and eyes askew, slurred speech, body paralysis, dizziness, phlegm accumulation, drooling, etc. (Fu et al., 2013; Juan et al., 2021).
To explore the efficacy and safety of DTD, XFZYD, and XXMD in stroke treatment with modern clinical studies, we searched both Chinese and English literature databases for RCTs using the three prescriptions. In the Chinese database, DTD, XFZYD, and XXMD respectively have 53, 79, and 25 stroke-related RCT studies. Among which, 37, 62, and 21 are ischemic stroke-related RCTs, respectively, and 16, 17, and 4 are hemorrhagic stroke-related RCTs, respectively. For example, Yan et al. reported that DTD significantly promoted neurological recovery of 32 ischemic stroke patients compared with the control group (Yan et al., 2017). Hong et al. reported that XFZYD improved muscle strength recovery and decreased blood viscosity of 100 patients after ischemic stroke (Hongqin et al., 2019). Juan et al. reported that the application of XXMD based on conventional treatment reduced NIHSS score and the levels of TNF-α, IL-6, and hs-CRP in 43 acute ischemic stroke patients (Juan et al., 2021). We believe that these Chinese RCTs could provide positive evidence on efficacy and safety, which correspond to the previous meta-analysis results (Fu et al., 2013; Shao et al., 2020; Kwon et al., 2021). In the English database, we only collected 2 RCTs related to DTD and XFZYD with other diseases, which showed the efficacy and safety of DTD in treating Alzheimer’s disease and XFZYD in treating unstable angina (Chu et al., 2010; Chua et al., 2015).
In addition, several previous pharmacological studies showed that DTD, XFZYD, and XXMD had neuroprotective effects and therapeutic potential in vitro and in vivo. DTD reportedly decreased inflammation and oxidative stress by inhibiting ERK, Nrf2/HO-1, and NF−kappa B signaling pathways (Kwon et al., 2021). XFZYD could inhibit adenosine diphosphate-induced platelet activation through blocking exposure of the glycoprotein IIb/IIIa complex (Li et al., 1999), and XFZYD mediated neuroprotection via inhibition of HIF-1α and TNF-α, followed by inhibition of inflammatory responses and apoptosis (Lee JJ. et al., 2011). XXMD was revealed to exert neuroprotective effects by downregulating the expression levels of LC3, Beclin1, Lamp1, and mitochondrial p62, thereby inhibiting mitochondrial autophagy (Lan et al., 2018). Although there are some similarities between mice and humans in cerebral vascular anatomy and post-stroke pathophysiology, there are also some important differences. For example, a higher ratio of gray matter to white matter in the brains of mice leads to more intense neurological function after stroke, as the rats’ neurons tolerate hypoxia longer and the astrocytes resist oxidative stress better than humans (Casals et al., 2011; Krafft et al., 2012). Moreover, rodents demonstrated better neuroprotection and a smaller risk of bleeding at thrombin blockade when compared with human coagulation function. Thus, some drugs have been shown to work well in stroke rats, but the results have limited application in treating humans (Hermann et al., 2019).
These prescriptions are multi-component and multi-targeted prescriptions, and they formed complex compound-target (C-T) networks. As is widely known, Network pharmacology is a commonly used approach for revealing the mechanisms of TCM based on the C-T network (Jiang, 2005; Hopkins, 2008; Lee et al., 2019; Yang L. et al., 2021). It is a common phenomenon that multiple prescriptions like DTD, XFZYD, and XXMD are used for reference or in conjunction with each other in TCM clinical practice. However, the synergetic relationship in C-T networks of multiple prescriptions remains unclear. An important reason is the lack of a suitable model based on network pharmacology. In this study, we propose a new model via integrating network pharmacology and chemoinformatic approaches to elucidate the synergistic effects of DTD, XFZYD, and XXMD in the treatment of post-stroke neurological damage. The workflow our our study was showed with Figure 1.
Botanical drugs with origin and dosage in DTD, XFZYD, and XXMD were retrieved from the Chinese pharmacopeia 2020 (National Pharmacopoeia Commission, 2020) and the Traditional Chinese Medicine Systems Pharmacology (TCMSP) database (https://tcmsp-e.com/) (Ru et al., 2014), and then proofread the Latin names of herbs by The Plant List database (https://www.theplantlist.org) (List, 2013). The name, origin, and dosage of botanical drugs in these prescriptions are listed in Table 1. Information concerning the chemical components of these botanical drugs was obtained from the TCMSP database and a literature search. Chemical structures were prepared and converted into canonical SMILES using OpenBabel Toolkit (version 3.1.1) (O’Boyle et al., 2008).
Genes related to stroke pathology were collected from multiple public databases. We used “stroke” as the keyword to search for genes in the Clarivate Analytics Integrity database (https://integrity.clarivate.com/integrity/xmlxsl/), therapeutic target database (TTD, http://db.idrblab.net/ttd/) (Wang Y. et al., 2020), DisGeNet database (https://www.disgenet.org/) (Pinero et al., 2020), and GeneCards (https://www.genecards.org/) (Safran et al., 2010). The pathological genes were defined as the intersection of the genes searched by GeneCards and the union set of the genes searched by Integrity, TTD, and DisGeNet.
The computed physicochemical properties and other parameters of all compounds were obtained from the TCMSP database. According to Lipinski’s rule of five (Pollastri, 2010), active compounds met the following conditions: 1) molecular weight (MW) ≤ 500, 2) hydrogen bond donors (Hdon) ≤ 5, 3) hydrogen bond acceptors (Hacc) ≤ 10, 4) octanol-water partition coefficient (AlogP) ≤ 5, and 5) rotation bond number (RBN) ≤ 10. Furthermore, based on the suggested drug screening criteria listed in the TCMSP, active compounds also met the following additional conditions: 6) oral bioavailability (OB) ≥ 30%, 7) drug likeness (DL) ≥ 0.1, 8) blood–brain barrier (BBB) penetration ≥0.3, 9) Caco-2 permeability (Caco-2) ≥ 0.4, and topological polar surface area (TPSA) ≤ 60 (Wang et al., 2020a; Wang et al., 2020b).
Potential targets of the active compounds were predicted by using the similarity ensemble approach (SEA) search server (https://sea.bkslab.org/) (Keiser et al., 2007), HitPickV2 (http://www.hitpickv2.com/) (Hamad et al., 2019), and TargetNet (http://targetnet.scbdd.com) (Yao et al., 2016). The union set of the prediction results of these three tools is defined as the potential targets. The C-T network was constructed and visualized using Cytoscape version 3.8.2 (Kohl et al., 2011) and yFiles layouts (https://www.yworks.com/blog/yfiles-cytoscape-app).
The constructed C-T networks were simplified by using the collaborative filtering algorithm. First, the ECFP6 molecular fingerprints for all the compounds were calculated by using the “rcdk” package (Voicu et al., 2020), and then the Tanimoto coefficient (TC) of all paired compounds was calculated. The following is the formula of the algorithm:
Assume that the total number of compounds is
Assume that the total number of targets is
Matrix
GO terms of biological process, cellular component, molecular function, and human gene information were downloaded from the QuickGO database (https://www.ebi.ac.uk/QuickGO/) (Binns et al., 2009). The reference human genes and pathways were obtained from the Kyoto Encyclopedia of Genes and Genomes (KEGG) database (http://www.kegg.jp/) (Ogata et al., 1999). GO terms and KEGG pathways with fewer than 10 genes were removed. The enrichment analysis was performed using the hypergeometric test and the formula shown in a previous report (Liu HY. et al., 2019; Gao et al., 2020). An FDR-corrected p-value ≤ 0.05 was considered significantly enriched.
The crystal structures of the filtered targets were downloaded from the Protein Data Bank (PDB) database (https://www.rcsb.org/) (Messenguy, 1987). The filtered molecule SMILES were converted into “. pdb” format by Discovery Studio version 2016. Proteins and compounds in “. pdb” format were converted to “. pdbqt” format for molecular docking using OpenBabel Toolkit. The center position (x, y, z coordinates) and radius of potential binding sites of target proteins were calculated using the From Receptor Cavities tool in Discovery Studio. Molecular docking analysis of the filtered compounds and proteins was performed using AutoDock Vina 1.1.2 (Trott and Olson, 2010) with its default parameters, and the highest affinity (the lowest docking energy) of each docking pair was chosen as the docking result. The compound-target pairs with the lowest docking energy ≤ −8 kcal/mol were screened for further analysis.
The filtered compound-target pairs, prescriptions, botanical drugs, and pathways were used to construct the complex network. We calculated the molecular fingerprint similarity matrix for the compounds in the network. For compound pairs with similarity greater than 0.5, the compound with the largest number of targets was retained. Other compounds with similarity of less than 0.5 were retained. The following formula was used to calculate the synergistic score:
In the formula,
The HT22 cell line (murine hippocampal cells) was obtained from CHI SCIENTIFIC (Shanghai, China). The cells were maintained in a humidified atmosphere at 37°C, with 5% CO2 and a complete culture medium (DMEM supplemented with 10% FBS). Oxygen and glucose deprivation/reoxygenation (OGD/R) is a well-established model to mimic ischemic/reperfusion conditions of cells in vitro (Moran et al., 2017). Specifically, the cells were washed three times with PBS, digested by trypsin, and plated in 20,000/200 µL medium per well of a 96-well plate. After 24 h, the cells were changed to low glucose DMEM (1 g/L glucose) and incubated in a hypoxic environment at 1% O2, 5% CO2, and 37°C for 18 h. After the OGD period, the cells were incubated with a complete culture medium under normoxic conditions for 24 h. In addition, different concentrations of compounds were dissolved in a complete culture medium during the period of reoxygenation. 1/10,000 DMSO in culture medium was used as vehicle control.
Cell viability assays were carried out with Cell Counting Kit-8 (RiboBio, China) according to the manufacturer’s instructions. In brief, 10 μL CCK8 solution in 100 μL complete culture medium was added to each well of the 96-well plate and further incubated for 3 h. Then, the absorbance was measured at 450 nm optical density in a microplate reader (KC junior, BioTek, United States). The cell viability was calculated by the mean of the optical density values in 6 repeat wells.
Fetal bovine serum (FBS) and Dulbecco’s Modified Eagle’s Medium (DMEM) were purchased from Thermo Fisher Biochemical Products (Beijing) Co., Ltd. Hypoxic bags were purchased from Mitsubishi Gas Chemical Company, Inc. (Japanese). Cell Counting Kit-8 (CCK-8) was purchased from Dojindo Laboratories (Japanese). Quercetin (>98% purity by HPLC), Baicalin (>93.1% purity by HPLC), Ginsenoside Rg1 (>98.6% purity by HPLC), and Estrone (>98% purity by HPLC) were purchased from Jiangsu Yongjian Pharmaceutical Technology Co., Ltd. (Jiangsu, China).
R statistical software version 4.0.3 was used for statistical analysis of experimental data. Outliers in each repeat that were higher than twofold of the standard deviation were eliminated. Independent sample t-tests were used to compare the individual effects of different concentrations of drugs on cell viability. One-way ANOVA and LSD posthoc tests were used to test the synergistic effects of the active drugs on cell viability. A p value less than 0.05 was considered statistically significant.
We identified 981, 1,688, and 2016 compounds in the DTD, XFZYD, and XXMD, respectively, from the TCMSP database (Supplementary Table S1). According to the rigorous screening criteria, there were 54, 49, and 120 active compounds in DTD, XFZYD, and XXMD, respectively (Supplementary Tables S2–S4). Because there were such few records of the compounds of some herbs were in the TCMSP database, no compounds were found in the Dihuang [Rehmannia glutinosa (Gaertn.) DC. (Plantaginaceae; rehmannia root)], Fuling [Poria cocos (Schw.) Wolf (Polyporaceae; Wolfiporia)], Jiegeng [Platycodon grandiflorus (Jacq.) A. DC. (Campanulaceae; balloonflower root)], Taoren [Prunus persica (L.) Batsch (Rosaceae; Peach kernel)]), or Zhuru [Bambusa tuldoides Munro (Poaceae; Bamboo shavings)] after ADMET screening. Furthermore, we found 33, 45, and 35 herbal compounds in DTD, XFZYD, and XXMD, respectively, through literature search (Supplementary Table S5). The unique compounds screened by ADMET and obtained from the literature search were combined for subsequent analysis.
The numbers of filtered compounds in DTD, XFZYD, and XXMD were 77, 79, and 109, respectively. After combining these compounds, a total of 171 unique compounds were used for target prediction. Three web servers, SEA, HItPickV2, and TargetNet, were used for compound-target prediction, and the union of prediction results was used for subsequent analysis. The number of predicted targets of these compounds ranged from 23 to 189 (Supplementary Table S6). The above compound-target prediction results were used to construct the C-T network for each prescription.
The original C-T network of DTD contains 7,120 compound-target pairs with 77 compounds and 733 targets, the original C-T network of XFZYD contains 7,122 compound-target pairs with 79 compounds and 768 targets, and the original C-T network of XXMD contains 10,372 compound-target pairs with 109 compounds and 755 targets (Figure 2A). All three C-T networks were filtered using the collaborative filtering algorithm. The filtered DTD network contained 3,183 compound-target pairs with 39 compounds and 534 targets, the filtered XFZYD network contained 2,925 compound-target pairs with 40 compounds and 508 targets, and the filtered XXMD network contained 4,837 compound-target pairs with 55 compounds and 599 targets (Figure 2B). There were significant differences in the average shortest path length, closeness centrality, neighborhood connectivity, and radiality between the original and filtered C-T networks of DTD and XFZYD. There was no difference in topological properties between the original and filtered XXMD networks (Figure 2C).
FIGURE 2. Characteristics and topological properties of the original and filtered compound-target (C-T) networks: The original C-T network of DTD, XFZYD, and XXMD (A). The filtered C-T network of DTD, XFZYD, and XXMD (B). Topological properties of the original and filtered C-T network of DTD, XFZYD, and XXMD (C). Significance: *p < 0.05, **p < 0.01, ***p < 0.001, ****p < 0.0001.
Although there were only half of the compounds in the filtered network compared to the original network, most of the herbs (8 out of 9 herbs in DTD, 10 out of 11 herbs in XFZYD, and all herbs in XXMD) and targets (72.9% targets in DTD, 66.1% targets in XFZYD, and 79.3% targets in XXMD) were still retained (Figures 3A,C). Furthermore, the ratio of included pathological genes to the original retained was 79.5, 76.9, and 83.2% (Figures 3B,C). In addition, the proportion of compounds shared by the three prescriptions in the union of all compounds only decreased from 14.6 to 11.5%, and the proportion of targets shared by the three prescriptions in the union of all targets only decreased from 53.7 to 43.9% after filtering (Figure 3A). A total of 1,745 stroke pathological genes were collected in this study (Supplementary Table S7). The intersection of the union targets in three prescriptions and the pathological genes after filtering retained 85.8% (Figure 3B). Finally, comparing the results of GO enrichment, the results indicated that most of the biological functions were maintained in the filtered C-T networks, and that the ratio of overlapped GO biological process to the original were 82.9, 79.6, and 84.1% in DTD, XFZYD, and XXMD, respectively. Additionally, the ratio of overlapped GO cellular components to the original were 80.2, 77.2, and 79.2% in DTD, XFZYD, and XXMD, and the ratio of overlapped GO molecular function to the original were 79.1, 69.9, and 82.6% in DTD, XFZYD, and XXMD, respectively (Figure 3D).
FIGURE 3. The components and biological functions of the original and filtered compound-target (C-T) networks: Venn diagram of herbs, compounds and predicted targets in the original and filtered C-T networks of DTD, XFZYD, and XXMD (A). Venn diagram of predicted targets and pathological genes in the original and filtered C-T network (B). Number of compounds, predicted target genes, and covered pathological genes in the original and filtered C-T networks of DTD, XFZYD, and XXMD (C). Number of enriched GO biological process (GOBP), GO cellular component (GOCC), and GO molecular function (GOMF) terms in the original and filtered target genes of DTD, XFZYD, and XXMD (D).
KEGG pathway enrichment results showed that the genes related to stroke pathology and the original and filtered target genes were all enriched in most of the same pathways of cellular processes, environmental information processing, and organismal systems. However, target genes were enriched in multiple metabolic pathways, whereas genes related to stroke pathology had little effect on metabolic pathways (Figure 4). 61 enriched pathways were shared by all groups. Then, we searched the correlation of the above pathways and stroke in the literature database. The top 6 pathways, including the apoptosis pathway, TNF signaling pathway, VEGF signaling pathway, NF−kappa B signaling pathway, PI3K−Akt signaling pathway, and MAPK signaling pathway, were defined with more than 400 studies. The enriched filtered target genes in these pathways in DTD, XFZYD, and XXMD are also listed (Supplementary Table S8). Integrating these pathways and targets showed that most targets in these pathways were shared by two or three prescriptions, especially in the MAPK signaling pathway and the PI3K/AKT signaling pathway (Figure 5).
FIGURE 4. The enriched KEGG pathways of pathological genes and the original and filtered target genes of DTD, XFZYD, and XXMD: The figure shows the union set of the top 100 enriched KEGG pathways (sorted by FDR corrected p value) in each group.
FIGURE 5. Curated KEGG pathways related to the pathogenesis of stroke: Curated pathways are shown in the green boxes. Genes are shown in colored, rounded rectangles.
There were 63 unique filtered targets in the above 6 pathways, and all of their structures were collected from the PDB database. We downloaded these proteins and predicted their potential binding sites (Supplementary Table S9). Molecular docking of the filtered compounds and these target proteins was performed, and the compound-target pairs with high affinity (less than -8 kcal/mol) were screened. Based on these compound-target pairs and information on prescriptions, botanical drugs, and pathways, we constructed a prescription-herb-compound-target-pathway network (Figure 6A). The complex network revealed that the screened targets were involved in multiple pathways, and more than half of the identified compounds targeted NOS3, PTGS2, and MAPK3. After removing the molecular fingerprint similarities higher than 0.5 of these compounds (Figure 6B), the remaining 18 compounds, as key synergistic compounds, were calculated for synergistic scores. Next, the top 5 synergistic scoring compounds were defined as candidate compounds (Figure 6C). These compounds were MOL62 (quercetin), MOL007021 (trans-beta.-terpinyl benzoate), MOL14 (baicalin), MOL42 (ginsenoside Rg1), and MOL010921 (estrone) (Figure 6D).
FIGURE 6. Screening of key compounds by calculating the synergistic score: Network relationship of prescription, herb, compound, target, and pathway (A). Molecular fingerprint similarity of the screened compounds. Compound pairs with similarity >0.5 are marked with an asterisk. Gray color indicates the removed compound (B). Synergistic score of the filtered compounds. The top 5 compounds are shown in red (C). Chemical structure of the top 5 compounds (D).
The OGD/R model was used to verify the reliability of our model, and the independent and synergistic effects of these four compounds on cell viability were deeper explored and analyzed (we did not find a supplier of MOL007021). The results indicated that quercetin significantly improved cell viability from low to high concentrations, compared with the vehicle control group. In addition, baicalin significantly improved cell viability at 12.5 and 25 μM. However, too high of a concentration of baicalin above 50 µM could promote cell death, indicating potential cell cytotoxicity. This was not because the concentration of DMSO as a solvent was too high, even in a 100 uM concentration of Baicalin, DMSO was not more than 1/10,000. Moreover, ginsenoside Rg1 showed activity at only 6.25 μM, and there was no evident cell cytotoxicity in higher concentrations. However, estrone showed no effect on cell viability (Figure 7A). Under the optimal concentrations of these compounds (quercetin: 3.12 μM, baicalin: 12.5 μM, and ginsenoside Rg1: 6.25 μM), the synergetic effect of these three compounds showed better cytoprotective effects in combination, compared with individual drug application. Moreover, the combination of baicalin and ginsenoside Rg1 showed the best protective effect, the combination of quercetin and ginsenoside Rg1 showed the second good effect, whereas the combination of quercetin and baicalin was not so significant (Figure 7B).
FIGURE 7. Experimental verification of the compound synergistic effect: The effect of different concentrations of single drugs on cell viability (A). The synergistic effect of three drugs on cell activity (B). Significance: *p < 0.05, **p < 0.01, ***p < 0.001, and ****p < 0.0001.
The docking modes of the three verified compounds and their targets are shown in Figure 8. Quercetin binds to 6 proteins (CSNK2A1, MAPK3, MAPK10, MCL1, PKN1, and PTGS2). Baicalin binds to 2 proteins (PTGS2 and RPS6KA3); the relevant amino acid residues in PTGS2 are Gly354, Ala516, Phe580, and Ser581, and the relevant amino acid residues in RPS6KA3 are Leu74, Val82, Leu200, Thr210, and Asp211. Ginsenoside Rg1 binds to NOS3 at 11 amino acid residues. Furthermore, the 2D binding pattern diagram shows that quercetin strongly binds to the above proteins, which was consistent with the cell experimental results that demonstrated that quercetin most stably improved cell viability at all concentrations. Furthermore, the targets of ginsenoside Rg1 were complementary with quercetin and baicalin. However, the targets of quercetin and Baicalin overlap, which was also consistent with the synergic effect of the above combinations.
FIGURE 8. Potential binding mode of the compound and the docking target: The structure of the protein and the docking site of the compound. The bonds between the compounds and the amino acid residues are indicated by colored dashed lines.
Numerous clinical practices have revealed the significant effect of TCM prescriptions in the treatment of stroke. Combined with modern medical methods, some extracts of several herbs recorded in TCM prescriptions, such as Calendula officinalis, Asarum sieboldii, Ginkgo biloba, and Salvia miltiorrhiza, have been widely used in clinical treatment and have shown remarkable effects (Wu et al., 2007; Zhu et al., 2021). Although the use of TCM prescriptions for the treatment of stroke can be traced back to the TCM literature more than 2,000 years ago, and many prescriptions and related herbs are still widely used in the treatment of stroke in China and around the world, there have been insufficient RCTs of TCM prescriptions. Among the TCM prescriptions for the treatment of stroke, only a small number of compound prescriptions provide high-quality evidence through RCTs, such as Neuroaid (Chen et al., 2009; Venketasubramanian et al., 2009; Chen et al., 2013) and Danhong injection (Li B. et al., 2015). There are still many challenges in conducting high-quality RCT research on DTD, XFZYD, and XXMD, such as inconsistent terms of diagnosis and treatment in Chinese medicine, unique theoretical basis, difficulty in designing placebos with the same smell, appearance, and taste, etc. To summarize, although the previous RCTs provided a weak therapeutic recommendation of DTD, XFZYD, and XXMD use on stroke, they still give recommendations for efficacy and safety.
There is still a lack of effective methods to reveal the synergistic effects of multiple TCM prescriptions on complex diseases. To solve this problem, we designed a new model to elucidate the synergistic effect of DTD, XFZYD, and XXMD in stroke treatment by combining pharmacological and chemoinformatic approaches. Then, through molecular docking and network analysis, the key active components in the prescription were determined. Finally, the experimental data was used to verify the reliability of our model. The results showed that quercetin, baicalin, and ginsenoside Rg1 independently and synergistically save damaged neuron cells in vitro.
In this study, 28 botanical drugs were checked without HILI report in CNKI and Pubmed databases, and then we found the related hepatotoxicity records in CNKI and TCM-ID databases. Two herbs, including Banxia [Pinellia ternata (Thunb.) Makino (Araceae; Pinellia ternata)] and Nanxing Arisaema heterophyllum Blume (Araceae; Reddish jackinthepulpit), were recorded with potential hepatotoxicity. However, their toxicity were almost eliminated after industry-standard processing with Alunite and Shengjiang [Zingiber officinale Roscoe (Zingiberaceae; fresh ginger)] (Su et al., 2016; Sun et al., 2019; Yu et al., 2019). Although many Chinese medicines are considered to have potential hepatotoxicity, only a small number of herbs have been confirmed to have serious hepatotoxicity. Among the 8,980 traditional Chinese herbs recorded in Chinese pharmacopeia (2015 and 2020) (CommissionNP, 2015; CommissionNP, 2020) and Chinese Materia Medica Dictionary (2006), only 107 herbs have been confirmed to have hepatotoxicity, accounting for 1.2%, such as Tripterygium wilfordii (Tian et al., 2019) and Polygonum multiflorum (Liu Y. et al., 2019), but in Asia with preference to herbal TCM in China, 11,160 published HILI cases had been identified, all with verified causality by using RUCAM (Roussel Uclaf Causality Assessment Method) (Teschke et al., 2020). Although the liver and kidney toxicity of these herbs has been widely discussed and has caused growing concern, most herbs only have slight, reversible, or dose-related hepatotoxicity. We believe that TCM will be better used in the future with more professional toxic-related studies and clinical evidence accumulation.
Firstly, in the constructed C-T networks of the three prescriptions, the shared compounds accounted for 14.6% of all compounds. Encouragingly, the shared targets accounted for up to 53.7% of all predicted targets. Combined with previous studies, these results suggested that DTD, XFZYD, and XXMD have therapeutic effects with important multitarget drugs and potential synergistic effects (Lapchak, 2011; Kibble et al., 2015). Because of the complex signal network of stroke, intervention and regulation of a single target often cannot change the overall state, and often fail to achieve the expected effect in the treatment of stroke (Casas et al., 2019). The synergy of multitarget drugs in the prescription can improve efficacy and reduce adverse reactions, which has good application prospects (Chou, 2006).
How to eliminate miscellaneous information to identify the synergistic components of multiple prescriptions is a substantial challenge. Our strategy is based on network pharmacology and a conventional collaborative filtering model to recommend synergistic ingredients, thus preliminarily narrowing the scope of the experiment (Ozsoy et al., 2018; Yang M. et al., 2021). In our modified model, the important drugs identified by molecular structure and C-T connectivity tend to have convergent indications. Consequently, the top 50% of the compounds and their targets based on the synergy score were selected for further analysis. The reliability and accuracy of this new method were verified from three aspects: network topology, pathological gene coverage, and functional enrichment coverage. There was a similar network topology, no less than 75% overlap rate of pathological gene intersection, and no less than 79% overlap rate of GO function enrichment. These results showed that the key components filtered out by our model can retain the functionality of the original formula.
Through KEGG enrichment analysis and a literature search, 6 pathways and 63 targets were filtered according to the pathophysiological process of stroke. TNF-α, as an important inflammatory mediator that increases the degree of pathology, causes inflammatory hyperplasia and neuronal apoptosis (Lambertsen et al., 2012; Chen AQ. et al., 2019). In addition, the activation of the VEGF signaling pathway, which produces excessive NO levels, is a key link in brain edema caused by BBB injury (Guix et al., 2005; Casas et al., 2019). Moreover, the NF−κB signaling pathway, the PI3K−Akt signaling pathway, and the MAPK signaling pathway are reportedly involved in the signal transmission of various inflammatory mediators, including the above (Lai et al., 2014). These key genes involved in multiple pathways provide potential therapeutic targets for neuroprotective therapeutic strategies.
After the next strict docking, these potential therapeutic targets and matched compounds were shown in the multilevel network, including 13 remaining targets and 26 compounds. Among these 13 remaining targets, NOS3 and PTGS2 are the targets of most binding compounds. The overactivation of NOS3 after stroke can produce nitric oxide (NO), which enhances the synthesis of proinflammatory mediators, participates in the formation of oxygen free radical damage, and causes brain edema with vascular smooth muscle relaxation (Guix et al., 2005; Casas et al., 2019). PTGS2 metabolizes arachidonic acid released by damaged cells into inflammatory prostaglandins, which recruit inflammatory cells to cause excessive nerve damage (Dore and Shafique Ahmad, 2015). In addition, among these 26 compounds, after removing the molecular fingerprint similarity, 18 compounds were defined as key synergistic compounds. To evaluate the hepatotoxicity, we used three methods, including searching in Pubchem, predicting in ADMT webserver, and Discovery Studio (Supplementary Table S10). The results showed that Baicalin may have slight hepatotoxicity, and isoimperatorin may have serious hepatotoxicity
To verify the reliability of the model, we intend to select the top five compounds in the collaborative ranking for experimental verification. Because trans-beta-terpinyl benzoate was not available for purchase, we verified the independent and synergistic effects of the other four compounds on nerve cell survival with the OGD/R model. Quercetin, baicalin, and ginsenoside Rg1 significantly improved cell viability. Furthermore, the results showed that the synergistic effects of the two compounds were significant, especially the combination of quercetin and ginsenoside Rg1, as well as the combination of baicalin and ginsenoside Rg1, because these two combinations of binding targets were complementary. The OGD/R model simulated neuroinflammation and nerve damage caused by Is/Rep after stroke. Neuroinflammation caused by long-term hypoxia and hemorrhage was more intense, compared with Is/Rep. This was because the contents of the dead cells from long-term hypoxia and the hemoglobin spines after hemorrhagic stroke included more intense inflammatory factors, recruiting a large number of inflammatory cells in a short time and causing more serious neuroinflammation (Rayasam et al., 2018; Hanafy et al., 2019; Tschoe et al., 2020). However, different types of neuroin had similar flammation pathological processes with the same release of inflammatory mediators to aggregate inflammatory cells. For example, overexpressed inflammatory mediators, including matrix metalloproteinase (MMP), high mobility group frame 1 (HMGB1), arachidonic acid metabolites, mitogen-activated protein kinase (MAPK) activation, interleukin-1 (IL-1), and TNF, attracted inflammatory cells, leading to further damage (Jayaraj et al., 2019; Levard et al., 2021). In addition to inflammatory mediators, other common processes, such as the release of toxic excitatory neurotransmitters and damage of the BBB exacerbated more cell death and inflammation (Yang et al., 2019). Thus, our drugs’ effect may be just as effective in other types of neuroinflammation.
Molecular docking showed that Quercetin binds to 6 proteins: CSNK2A1, MAPK3, MAPK10, MCL1, PKN1, and PTGS2; Baicalin binds to 2 proteins: PTGS2 and RPS6KA; Ginsenoside Rg1 binds to NOS3. According to previous studies, Interactions between compounds and targets have been partially confirmed as follows, Quercetin binding resulted in decreased CSNK2A1 protein activity (Wang et al., 2011; Aichinger et al., 2020), influencing phosphorylation of MAPK3 and MAPK10 proteins (Fridrich et al., 2008; Maso et al., 2014; Li Y. et al., 2015; Gupta et al., 2017), promoting MCL1 degradation (Spagnuolo et al., 2011; Russo et al., 2013) and decreasing PTGS2 protein activity (O'Leary et al., 2004; Al-Fayez et al., 2006). Baicalin not only bound to PTGS2 suppressing prostaglandin E2 production but also indirectly decreased PTGS2 expression (Kim et al., 2007; Singh and Chopra, 2014; Park et al., 2017). Ginsenoside Rg1 reportedly inhibited NOS activity (Shen et al., 2007; Chen et al., 2010).
All compounds reportedly relieve neuronal damage through complex and multifaceted mechanisms, including anti-oxidant, anti-inflammatory, anti-excitatory toxicity, and neuronal regeneration-promoting mechanisms in vitro and in vivo. Previous studies showed that quercetin could decrease the release of TNF-α, IL-1β, and IL-6 by microglia (Zhang et al., 2016), reduce NO and ROS levels (Yang R. et al., 2021), inhibit the destruction of the BBB by MMPs (Lee JK. et al., 2011), and reduce the pro-apoptotic proteins, Bax and caspase-3 (Zhang Y. et al., 2015). Baicalin reportedly decreased protein expression of NF-κB P65, iNOS, COX-2, and MMP9 (Xu et al., 2013; Liang et al., 2017; Chen et al., 2018), protected glutamine synthetase from ROS (Song et al., 2020), and inhibited ferroptosis (Duan et al., 2021). Ginsenosides are the main active components of ginseng, and more than 40 different monomer structures have been identified. Among them, Rg1 has little toxicity, and its neuroprotective effect has been widely studied (Xie et al., 2015). In addition to the similar mechanism of quercetin and baicalin (Zheng et al., 2019), ginsenoside Rg1 reportedly promotes cerebral angiogenesis through the PI3K/Akt/mTOR signaling pathway (Chen J. et al., 2019). Although there have been no clinical trials on stroke treatment, they all have been reported to be safe and have therapeutic effects in the treatment of cardiovascular and skeletal muscle injury repair (Dower et al., 2015; Hang et al., 2018; Bazzucchi et al., 2020; Lee et al., 2021). Based on our criteria, 5 active compounds were finally screened. Preliminary research and our experimental results have shown that quercetin, baicalin, and ginsenoside Rg1 are effective (one compound is unavailable). Due to our strict scoring system, the number of final screened compounds is relatively small. Therefore, more compounds with novel structures may be missed.
According to previous literature, Quercetin (Yang et al., 2015; Zhang Y. et al., 2015; Yang R. et al., 2021), Baicalin (Xu et al., 2013; Lin et al., 2014; Liang et al., 2017; Chen et al., 2018), and Ginsenoside Rg1 (Chen J. et al., 2019) can exert excellent anti-inflammatory and antioxidant effects in the form of monomers. If the monomer compounds taken orally or intragastrically, they will experience similar metabolic pathways in the intestines, which mainly include metabolic processes such as binding to glucose, or binding to glucuronic acid, methylation, and hydrolysis. The main metabolites of Quercetin, Baicalin, and Ginsenoside Rg1 are Quercetin 3-O-β-D-glucuronide (Morand et al., 2000a; b), Baicalein 7-O-glucuronide (Xing et al., 2005; Zhang J. et al., 2015), and M1 (20- O -β- D -glupyranosyl- 20 (S)- protopanaxadiol (Wang et al., 2014; Wang et al., 2016), which are also major conjugates in human plasma, and are distributed throughout the body to exert beneficial functions in target tissues.
In this study, the synergistic effect of TCM compounds was preliminarily explored by combining network pharmacology and chemoinformatic methods. Cellular experiments show that the compounds we screened have a certain synergistic therapeutic effect. This work provides a methodological reference for the optimization and secondary development of TCM prescriptions. Admittedly, there are some limitations to this study. Firstly, the compound and herbal information we collected may not be sufficient. Secondly, the effects of compounds on the human metabolic network are not considered. Thirdly, more filtered key synergistic compounds should be selected for validating the reliability of our model. Finally, the active effect of the synergistic compounds was validated only with in vitro assay, without animal assay, and experimental work assessing docking affinity. In future work, we will design a more refined mathematical model to calculate the synergistic effect of the compounds and verify it in animal experiments.
The original contributions presented in the study are included in the article/Supplementary Material, further inquiries can be directed to the corresponding authors.
Research design: D-GG, W-XL, and AX. Data collection: D-GG, AX, W-XL, S-XS, Y-PC, and Z-HW. Data analysis: W-XL, AX, S-XS, and D-GG. Experiments: AX, Y-PC, K-XW, and Z-HW. Manuscript writing: W-XL, AX, W-CL, S-QG, and C-ZD. Manuscript revision: C-ZD, W-XL, AX, D-GG, S-XS, X-FL, XZ, N-BX, and RL. The authors declare that there are no conflicts of interest.
This work was supported by the National Natural Science Foundation of China (Nos 81974178 for C-ZD, 82001300 for W-CL, 81974177 for XZ, 32070676 for D-GG). Natural Science Foundation of Guangdong Province (No. 2021A1515010737).
The authors declare that the research was conducted in the absence of any commercial or financial relationships that could be construed as a potential conflict of interest.
All claims expressed in this article are solely those of the authors and do not necessarily represent those of their affiliated organizations, or those of the publisher, the editors and the reviewers. Any product that may be evaluated in this article, or claim that may be made by its manufacturer, is not guaranteed or endorsed by the publisher.
We would like to thank the support of the National Natural Science Foundation.
The Supplementary Material for this article can be found online at: https://www.frontiersin.org/articles/10.3389/fphar.2022.784242/full#supplementary-material
BBB, blood–brain barrier; C-T, compound-target; DTD, Di-Tan Decoction; NO, nitric oxide; OGD/R, oxygen–glucose deprivation and reoxygenation; TC, Tanimoto coefficient; TCM, Traditional Chinese medicine; TCMSP, Traditional Chinese Medicine Systems Pharmacology; TTD, therapeutic target database; XFZYD, Xue-Fu Zhu-Yu Decoction; XXMD, Xiao-Xu-Ming Decoction.
Aichinger, G., Dellafiora, L., Pantazi, F., Del Favero, G., Galaverna, G., Dall'Asta, C., et al. (2020). Alternaria Toxins as Casein Kinase 2 Inhibitors and Possible Consequences for Estrogenicity: a Hybrid In Silico/In Vitro Study. Arch. Toxicol. 94 (6), 2225–2237. doi:10.1007/s00204-020-02746-x
Al-Fayez, M., Cai, H., Tunstall, R., Steward, W. P., and Gescher, A. J. (2006). Differential Modulation of Cyclooxygenase-Mediated Prostaglandin Production by the Putative Cancer Chemopreventive Flavonoids Tricin, Apigenin and Quercetin. Cancer Chemother. Pharmacol. 58 (6), 816–825. doi:10.1007/s00280-006-0228-3
Bazzucchi, I., Patrizio, F., Ceci, R., Duranti, G., Sabatini, S., Sgro, P., et al. (2020). Quercetin Supplementation Improves Neuromuscular Function Recovery from Muscle Damage. Nutrients 12. doi:10.3390/nu12092850
Binns, D., Dimmer, E., Huntley, R., Barrell, D., O'Donovan, C., and Apweiler, R. (2009). QuickGO: a Web-Based Tool for Gene Ontology Searching. Bioinformatics 25 (22), 3045–3046. doi:10.1093/bioinformatics/btp536
Carlson, A. P., Hänggi, D., Macdonald, R. L., and Shuttleworth, C. W. (2020). Nimodipine Reappraised: An Old Drug with a Future. Curr. Neuropharmacol 18 (1), 65–82. doi:10.2174/1570159X17666190927113021
Casals, J. B., Pieri, N. C., Feitosa, M. L., Ercolin, A. C., Roballo, K. C., Barreto, R. S., et al. (2011). The Use of Animal Models for Stroke Research: a Review. Comp. Med. 61 (4), 305–313.
Casas, A. I., Hassan, A. A., Larsen, S. J., Gomez-Rangel, V., Elbatreek, M., Kleikers, P. W. M., et al. (2019). From Single Drug Targets to Synergistic Network Pharmacology in Ischemic Stroke. Proc. Natl. Acad. Sci. U S A. 116 (14), 7129–7136. doi:10.1073/pnas.1820799116
Chen, A. Q., Fang, Z., Chen, X. L., Yang, S., Zhou, Y. F., Mao, L., et al. (2019a). Microglia-derived TNF-α Mediates Endothelial Necroptosis Aggravating Blood Brain-Barrier Disruption after Ischemic Stroke. Cell Death Dis 10 (7), 487. doi:10.1038/s41419-019-1716-9
Chen, C., Venketasubramanian, N., Gan, R. N., Lambert, C., Picard, D., Chan, B. P., et al. (2009). Danqi Piantang Jiaonang (DJ), a Traditional Chinese Medicine, in Poststroke Recovery. Stroke 40 (3), 859–863. doi:10.1161/STROKEAHA.108.531616
Chen, C. L., Young, S. H., Gan, H. H., Singh, R., Lao, A. Y., Baroque, A. C., et al. (2013). Chinese Medicine Neuroaid Efficacy on Stroke Recovery: a Double-Blind, Placebo-Controlled, Randomized Study. Stroke 44 (8), 2093–2100. doi:10.1161/STROKEAHA.113.002055
Chen, H., Guan, B., Chen, X., Chen, X., Li, C., Qiu, J., et al. (2018). Baicalin Attenuates Blood-Brain Barrier Disruption and Hemorrhagic Transformation and Improves Neurological Outcome in Ischemic Stroke Rats with Delayed T-PA Treatment: Involvement of ONOO--MMP-9 Pathway. Transl Stroke Res. 9 (5), 515–529. doi:10.1007/s12975-017-0598-3
Chen, J., Zhang, X., Liu, X., Zhang, C., Shang, W., Xue, J., et al. (2019b). Ginsenoside Rg1 Promotes Cerebral Angiogenesis via the PI3K/Akt/mTOR Signaling Pathway in Ischemic Mice. Eur. J. Pharmacol. 856, 172418. doi:10.1016/j.ejphar.2019.172418
Chen, Y., Sun, A. M., Chen, Z. X., Liu, Y., Chen, L. H., and Yuan, Y. W. (2010). Ginsenoside Rg1 Protects Rat Hippocampal Neurons from Radiation Injury by Regulating NOS Activity. Nan Fang Yi Ke Da Xue Xue Bao 30 (7), 1522–1525.
Chou, T. C. (2006). Theoretical Basis, Experimental Design, and Computerized Simulation of Synergism and Antagonism in Drug Combination Studies. Pharmacol. Rev. 58 (3), 621–681. doi:10.1124/pr.58.3.10
Chu, F. Y., Wang, J., Yao, K. W., and Li, Z. Z. (2010). Effect of Xuefu Zhuyu Capsule (血府逐瘀胶囊) on the Symptoms and Signs and Health-Related Quality of Life in the Unstable Angina Patients with Blood-Stasis Syndrome after Percutaneous Coronary Intervention: A Randomized Controlled Trial. Chin. J. Integr. Med. 16 (5), 399–405. doi:10.1007/s11655-010-9999-9
Chua, K. K., Wong, A., Kwan, P. W., Song, J. X., Chen, L. L., Chan, A. L., et al. (2015). The Efficacy and Safety of the Chinese Herbal Medicine Di-tan Decoction for Treating Alzheimer's Disease: Protocol for a Randomized Controlled Trial. Trials 16, 199. doi:10.1186/s13063-015-0716-z
Collaborators, G. B. D. S. (2019). Global, Regional, and National burden of Stroke, 1990-2016: a Systematic Analysis for the Global Burden of Disease Study 2016. Lancet Neurol. 18 (5), 439–458. doi:10.1016/S1474-4422(19)30034-1
CommissionN.P (2015). Pharmacopoeia of the People's Republic of China 2015 edition. Beijing. China Medical Science and Technology Press.
CommissionN.P (2020). Pharmacopoeia of the People's Republic of China 2020 edition. Beijing. China Medical Science and Technology Press.
Dore, S., and Shafique Ahmad, A. (2015). “Cytoprotective Role of Prostaglandin D2 DP1 Receptor against Neuronal Injury Following Acute Excitotoxicity and Cerebral Ischemia,",” in In Brain Neurotrauma: Molecular, Neuropsychological, and Rehabilitation Aspects. Editor F. H. Kobeissy (Boca Raton (FL).
Dower, J. I., Geleijnse, J. M., Gijsbers, L., Zock, P. L., Kromhout, D., and Hollman, P. C. (2015). Effects of the Pure Flavonoids Epicatechin and Quercetin on Vascular Function and Cardiometabolic Health: a Randomized, Double-Blind, Placebo-Controlled, Crossover Trial. Am. J. Clin. Nutr. 101 (5), 914–921. doi:10.3945/ajcn.114.098590
Duan, L., Zhang, Y., Yang, Y., Su, S., Zhou, L., Lo, P. C., et al. (2021). Baicalin Inhibits Ferroptosis in Intracerebral Hemorrhage. Front. Pharmacol. 12, 629379. doi:10.3389/fphar.2021.629379
Feng, S., Yang, Q., Liu, M., Li, W., Yuan, W., Zhang, S., et al. (2011). Edaravone for Acute Ischaemic Stroke. Cochrane Database Syst. Rev.12, CD007230. doi:10.1002/14651858.CD007230.pub2
Fridrich, D., Teller, N., Esselen, M., Pahlke, G., and Marko, D. (2008). Comparison of Delphinidin, Quercetin and (-)-Epigallocatechin-3-Gallate as Inhibitors of the EGFR and the ErbB2 Receptor Phosphorylation. Mol. Nutr. Food Res. 52 (7), 815–822. doi:10.1002/mnfr.200800026
Fu, D. L., Lu, L., Zhu, W., Li, J. H., Li, H. Q., Liu, A. J., et al. (2013). Xiaoxuming Decoction for Acute Ischemic Stroke: a Systematic Review and Meta-Analysis. J. Ethnopharmacol 148 (1), 1–13. doi:10.1016/j.jep.2013.04.002
Gao, Y., Wang, K. X., Wang, P., Li, X., Chen, J. J., Zhou, B. Y., et al. (2020). A Novel Network Pharmacology Strategy to Decode Mechanism of Lang Chuang Wan in Treating Systemic Lupus Erythematosus. Front. Pharmacol. 11, 512877. doi:10.3389/fphar.2020.512877
Gong, X., and Sucher, N. J. (1999). Stroke Therapy in Traditional Chinese Medicine (TCM): Prospects for Drug Discovery and Development. Trends Pharmacol. Sci. 20 (5), 191–196. doi:10.1016/s0165-6147(98)01276-0
Guix, F. X., Uribesalgo, I., Coma, M., and Muñoz, F. J. (2005). The Physiology and Pathophysiology of Nitric Oxide in the Brain. Prog. Neurobiol. 76 (2), 126–152. doi:10.1016/j.pneurobio.2005.06.001
Gupta, R., Shukla, R. K., Chandravanshi, L. P., Srivastava, P., Dhuriya, Y. K., Shanker, J., et al. (2017). Protective Role of Quercetin in Cadmium-Induced Cholinergic Dysfunctions in Rat Brain by Modulating Mitochondrial Integrity and MAP Kinase Signaling. Mol. Neurobiol. 54 (6), 4560–4583. doi:10.1007/s12035-016-9950-y
Hamad, S., Adornetto, G., Naveja, J. J., Chavan Ravindranath, A., Raffler, J., and Campillos, M. (2019). HitPickV2: a Web Server to Predict Targets of Chemical Compounds. Bioinformatics 35 (7), 1239–1240. doi:10.1093/bioinformatics/bty759
Hanafy, K. A., Gomes, J. A., and Selim, M. (2019). Rationale and Current Evidence for Testing Iron Chelators for Treating Stroke. Curr. Cardiol. Rep. 21 (4), 20. doi:10.1007/s11886-019-1106-z
Hang, Y., Qin, X., Ren, T., and Cao, J. (2018). Baicalin Reduces Blood Lipids and Inflammation in Patients with Coronary Artery Disease and Rheumatoid Arthritis: a Randomized, Double-Blind, Placebo-Controlled Trial. Lipids Health Dis. 17 (1), 146. doi:10.1186/s12944-018-0797-2
Hermann, D. M., Popa-Wagner, A., Kleinschnitz, C., and Doeppner, T. R. (2019). Animal Models of Ischemic Stroke and Their Impact on Drug Discovery. Expert Opin. Drug Discov. 14 (3), 315–326. doi:10.1080/17460441.2019.1573984
Hongqin, S., Jianfei, Z., Qingfang, C., Zhiqiang, P., Dongping, W., Hui, L., et al. (2019). Clinical Observation of Xuefu Zhuyu Decoction in Treating Convalescent Period of Ischemic Stroke. Bright Chin. Med. 34 (22), 3388–3390.
Hopkins, A. L. (2008). Network Pharmacology: the Next Paradigm in Drug Discovery. Nat. Chem. Biol. 4 (11), 682–690. doi:10.1038/nchembio.118
Jayaraj, R. L., Azimullah, S., Beiram, R., Jalal, F. Y., and Rosenberg, G. A. (2019). Neuroinflammation: Friend and Foe for Ischemic Stroke. J. Neuroinflammation 16 (1), 142. doi:10.1186/s12974-019-1516-2
Jiang, W. Y. (2005). Therapeutic Wisdom in Traditional Chinese Medicine: a Perspective from Modern Science. Discov. Med. 5 (11), 455–461. doi:10.1016/j.tips.2005.09.006
Johnston, S. C., Easton, J. D., Farrant, M., Barsan, W., Conwit, R. A., Elm, J. J., et al. (2018). Clopidogrel and Aspirin in Acute Ischemic Stroke and High-Risk TIA. N. Engl. J. Med. 379 (3), 215–225. doi:10.1056/NEJMoa1800410
Juan, L., Yisong, L., and Yaning, W. (2021). Clinical Observation on Treatment of Acute Ischemic Apoplexy with Wind-Phlegm and Blood Stasis by Xiao Xuming Decoction. Bright Chin. Med. 36 (10), 1625–1627.
Keiser, M. J., Roth, B. L., Armbruster, B. N., Ernsberger, P., Irwin, J. J., and Shoichet, B. K. (2007). Relating Protein Pharmacology by Ligand Chemistry. Nat. Biotechnol. 25 (2), 197–206. doi:10.1038/nbt1284
Kibble, M., Saarinen, N., Tang, J., Wennerberg, K., Mäkelä, S., and Aittokallio, T. (2015). Network Pharmacology Applications to Map the Unexplored Target Space and Therapeutic Potential of Natural Products. Nat. Prod. Rep. 32 (8), 1249–1266. doi:10.1039/c5np00005j
Kim, K. W., Ha, K. T., Park, C. S., Jin, U. H., Chang, H. W., Lee, I. S., et al. (2007). Polygonum Cuspidatum, Compared with Baicalin and Berberine, Inhibits Inducible Nitric Oxide Synthase and Cyclooxygenase-2 Gene Expressions in RAW 264.7 Macrophages. Vascul Pharmacol. 47 (2-3), 99–107. doi:10.1016/j.vph.2007.04.007
Kohl, M., Wiese, S., and Warscheid, B. (2011). Cytoscape: Software for Visualization and Analysis of Biological Networks. Methods Mol. Biol. 696, 291–303. doi:10.1007/978-1-60761-987-1_18
Krafft, P. R., Bailey, E. L., Lekic, T., Rolland, W. B., Altay, O., Tang, J., et al. (2012). Etiology of Stroke and Choice of Models. Int. J. Stroke 7 (5), 398–406. doi:10.1111/j.1747-4949.2012.00838.x
Kwon, H.-K., Zhang, T., Wu, X. G., Qiu, J. Y., and Park, S. (2021). Efficacy and Safety of Di-tan Decoction for Treating post-stroke Neurological Disorders: a Systematic Review and Meta-Analysis of Randomized Clinical Trials. Chin. J. Nat. Medicines 19 (5), 339–350. doi:10.1016/S1875-5364(21)60035-3
Lai, T. W., Zhang, S., and Wang, Y. T. (2014). Excitotoxicity and Stroke: Identifying Novel Targets for Neuroprotection. Prog. Neurobiol. 115, 157–188. doi:10.1016/j.pneurobio.2013.11.006
Lambertsen, K. L., Biber, K., and Finsen, B. (2012). Inflammatory Cytokines in Experimental and Human Stroke. J. Cereb. Blood Flow Metab. 32 (9), 1677–1698. doi:10.1038/jcbfm.2012.88
Lan, R., Zhang, Y., Wu, T., Ma, Y. Z., Wang, B. Q., Zheng, H. Z., et al. (2018). Xiao-Xu-Ming Decoction Reduced Mitophagy Activation and Improved Mitochondrial Function in Cerebral Ischemia and Reperfusion Injury. Behav. Neurol. 2018, 4147502. doi:10.1155/2018/4147502
Lapchak, P. A. (2011). Emerging Therapies: Pleiotropic Multi-Target Drugs to Treat Stroke Victims. Transl Stroke Res. 2 (2), 129–135. doi:10.1007/s12975-011-0074-4
Lee, J. J., Hsu, W. H., Yen, T. L., Chang, N. C., Luo, Y. J., Hsiao, G., et al. (2011a). Traditional Chinese Medicine, Xue-Fu-Zhu-Yu Decoction, Potentiates Tissue Plasminogen Activator against Thromboembolic Stroke in Rats. J. Ethnopharmacol 134 (3), 824–830. doi:10.1016/j.jep.2011.01.033
Lee, J. K., Kwak, H. J., Piao, M. S., Jang, J. W., Kim, S. H., and Kim, H. S. (2011b). Quercetin Reduces the Elevated Matrix Metalloproteinases-9 Level and Improves Functional Outcome after Cerebral Focal Ischemia in Rats. Acta Neurochir (Wien) 153 (6), 1321–1329. doi:10.1007/s00701-010-0889-x
Lee, T. X. Y., Wu, J., Jean, W. H., Condello, G., Alkhatib, A., Hsieh, C. C., et al. (2021). Reduced Stem Cell Aging in Exercised Human Skeletal Muscle Is Enhanced by Ginsenoside Rg1. Aging (Albany NY) 13 (12), 16567–16576. doi:10.18632/aging.203176
Lee, W. Y., Lee, C. Y., Kim, Y. S., Kim, C. E., Lei, K., Renyi, Y., et al. (20192020). The Methodological Trends of Traditional Herbal Medicine Employing Network PharmacologyAnalysis on the Academic Thought of Treating Apoplexy in Qixiaoliangfang. Biomolecules中国中医急症 929 (06), 1095–1097. doi:10.3390/biom9080362
Levard, D., Buendia, I., Lanquetin, A., Glavan, M., Vivien, D., and Rubio, M. (2021). Filling the Gaps on Stroke Research: Focus on Inflammation and Immunity. Brain Behav. Immun. 91, 649–667. doi:10.1016/j.bbi.2020.09.025
Li, B., Wang, Y., Lu, J., Liu, J., Yuan, Y., Yu, Y., et al. (2015a). Evaluating the Effects of Danhong Injection in Treatment of Acute Ischemic Stroke: Study Protocol for a Multicenter Randomized Controlled Trial. Trials 16, 561. doi:10.1186/s13063-015-1076-4
Li, Y., Wang, J., Chen, G., Feng, S., Wang, P., Zhu, X., et al. (2015b). Quercetin Promotes the Osteogenic Differentiation of Rat Mesenchymal Stem Cells via Mitogen-Activated Protein Kinase Signaling. Exp. Ther. Med. 9 (6), 2072–2080. doi:10.3892/etm.2015.2388
Li, Y., Wang, Z., and Weng, J. (1999). Effect of Xuefu Zhuyu Decoction on Function of Platelet and Endothelial Cell. Zhongguo Zhong Xi Yi Jie He Za Zhi 19 (5), 289–291.
Liang, W., Huang, X., and Chen, W. (2017). The Effects of Baicalin and Baicalein on Cerebral Ischemia: A Review. Aging Dis. 8 (6), 850–867. doi:10.14336/AD.2017.0829
Lin, M., Li, L., Li, L., Pokhrel, G., Qi, G., Rong, R., et al. (2014). The Protective Effect of Baicalin against Renal Ischemia-Reperfusion Injury through Inhibition of Inflammation and Apoptosis. BMC Complement. Altern. Med. 14, 19. doi:10.1186/1472-6882-14-19
Liu, H. Y., Zhao, H., and Li, W. X. (2019a). Integrated Analysis of Transcriptome and Prognosis Data Identifies FGF22 as a Prognostic Marker of Lung Adenocarcinoma. Technol. Cancer Res. Treat. 18, 1533033819827317. doi:10.1177/1533033819827317
Liu, Y., Wang, W., Sun, M., Ma, B., Pang, L., Du, Y., et al. (2019b). Polygonum Multiflorum-Induced Liver Injury: Clinical Characteristics, Risk Factors, Material Basis, Action Mechanism and Current Challenges. Front. Pharmacol. 10, 1467. doi:10.3389/fphar.2019.01467
Marti-Carvajal, A. J., Valli, C., Marti-Amarista, C. E., Sola, I., Marti-Fabregas, J., and Bonfill Cosp, X. (2020). Citicoline for Treating People with Acute Ischemic Stroke. Cochrane Database Syst. Rev. 8, CD013066. doi:10.1002/14651858.CD013066.pub2
Maso, V., Calgarotto, A. K., Franchi, G. C., Nowill, A. E., Filho, P. L., Vassallo, J., et al. (2014). Multitarget Effects of Quercetin in Leukemia. Cancer Prev. Res. (Phila) 7 (12), 1240–1250. doi:10.1158/1940-6207.CAPR-13-0383
Messenguy, F. (1987). Multiplicity of Regulatory Mechanisms Controlling Amino Acid Biosynthesis in Saccharomyces cerevisiae. Microbiol. Sci. 4 (5), 150–153.
Morán, J., Perez-Basterrechea, M., Garrido, P., Díaz, E., Alonso, A., Otero, J., et al. (2017). Effects of Estrogen and Phytoestrogen Treatment on an In Vitro Model of Recurrent Stroke on HT22 Neuronal Cell Line. Cell Mol Neurobiol 37 (3), 405–416. doi:10.1007/s10571-016-0372-1
Morand, C., Manach, C., Crespy, V., and Remesy, C. (2000a). Quercetin 3-O-Beta-Glucoside Is Better Absorbed Than Other Quercetin Forms and Is Not Present in Rat Plasma. Free Radic. Res. 33 (5), 667–676. doi:10.1080/10715760000301181
Morand, C., Manach, C., Crespy, V., and Remesy, C. (2000b). Respective Bioavailability of Quercetin Aglycone and its Glycosides in a Rat Model. Biofactors 12 (1-4), 169–174. doi:10.1002/biof.5520120127
O'Boyle, N. M., Morley, C., and Hutchison, G. R. (2008). Pybel: a Python Wrapper for the OpenBabel Cheminformatics Toolkit. Chem. Cent. J. 2, 5. doi:10.1186/1752-153X-2-5
O'Leary, K. A., de Pascual-Teresa, S., de Pascual-Tereasa, S., Needs, P. W., Bao, Y. P., O'Brien, N. M., et al. (2004). Effect of Flavonoids and Vitamin E on Cyclooxygenase-2 (COX-2) Transcription. Mutat. Res. 551 (1-2), 245–254. doi:10.1016/j.mrfmmm.2004.01.015
Ogata, H., Goto, S., Sato, K., Fujibuchi, W., Bono, H., and Kanehisa, M. (1999). KEGG: Kyoto Encyclopedia of Genes and Genomes. Nucleic Acids Res. 27 (1), 29–34. doi:10.1093/nar/27.1.29
Ozsoy, M. G., Özyer, T., Polat, F., and Alhajj, R. (2018). Realizing Drug Repositioning by Adapting a Recommendation System to Handle the Process. BMC Bioinformatics 19 (1), 136. doi:10.1186/s12859-018-2142-1
Park, E., Lee, M. Y., Seo, C. S., Jeon, W. Y., and Shin, H. K. (2017). Yongdamsagan-tang, a Traditional Herbal Formula, Inhibits Cell Growth through the Suppression of Proliferation and Inflammation in Benign Prostatic Hyperplasia Epithelial-1 Cells. J. Ethnopharmacol 209, 230–235. doi:10.1016/j.jep.2017.08.002
Piñero, J., Ramírez-Anguita, J. M., Saüch-Pitarch, J., Ronzano, F., Centeno, E., Sanz, F., et al. (2020). The DisGeNET Knowledge Platform for Disease Genomics: 2019 Update. Nucleic Acids Res. 48 (D1), D845–D855. doi:10.1093/nar/gkz1021
Pollastri, M. P. (2010). Overview on the Rule of Five. Curr. Protoc. Pharmacol. Chapter 9. Unit 9 12. doi:10.1002/0471141755.ph0912s49
Rayasam, A., Hsu, M., Kijak, J. A., Kissel, L., Hernandez, G., Sandor, M., et al. (2018). Immune Responses in Stroke: How the Immune System Contributes to Damage and Healing after Stroke and How This Knowledge Could Be Translated to Better Cures? Immunology 154 (3), 363–376. doi:10.1111/imm.12918
Ru, J., Li, P., Wang, J., Zhou, W., Li, B., Huang, C., et al. (2014). TCMSP: a Database of Systems Pharmacology for Drug Discovery from Herbal Medicines. J. Cheminform 6, 13. doi:10.1186/1758-2946-6-13
Russo, M., Spagnuolo, C., Volpe, S., Tedesco, I., Bilotto, S., and Russo, G. L. (2013). ABT-737 Resistance in B-Cells Isolated from Chronic Lymphocytic Leukemia Patients and Leukemia Cell Lines Is Overcome by the Pleiotropic Kinase Inhibitor Quercetin through Mcl-1 Down-Regulation. Biochem. Pharmacol. 85 (7), 927–936. doi:10.1016/j.bcp.2013.01.011
Safran, M., Dalah, I., Alexander, J., Rosen, N., Iny Stein, T., Shmoish, M., et al. (2010). GeneCards Version 3: the Human Gene Integrator. Database baq020. doi:10.1093/database/baq020
Shao, J., Zhou, L., Shao, T., Ding, M., and Jin, Z. (2020). Effectiveness and Safety of the Xuefu Zhuyu Tang for post-stroke Depression: A Systematic Review and Meta-Analysis. Eur. J. Integr. Med. 37, 101150. doi:10.1016/j.eujim.2020.101150
Shen, L., Han, J. Z., Li, C., Yue, S. J., Liu, Y., Qin, X. Q., et al. (2007). Protective Effect of Ginsenoside Rg1 on Glutamate-Induced Lung Injury. Acta Pharmacol. Sin 28 (3), 392–397. doi:10.1111/j.1745-7254.2007.00511.x
Shen, Y. C., Lu, C. K., Liou, K. T., Hou, Y. C., Lin, Y. L., Wang, Y. H., et al. (2015). Common and Unique Mechanisms of Chinese Herbal Remedies on Ischemic Stroke Mice Revealed by Transcriptome Analyses. J. Ethnopharmacol 173, 370–382. doi:10.1016/j.jep.2015.07.018
Shi, W. L., Zhang, J. S., and Hu, Y. Q. (2013). Prevention and Treatment of Blood Vessels Related Diseases by Xuefu Zhuyu Decoction (See Text): its Clinical Application and Progress in Researches of Mechanisms. Zhongguo Zhong Xi Yi Jie He Za Zhi 33 (5), 712–716.
Singh, D. P., and Chopra, K. (2014). Flavocoxid, Dual Inhibitor of Cyclooxygenase-2 and 5-lipoxygenase, Exhibits Neuroprotection in Rat Model of Ischaemic Stroke. Pharmacol. Biochem. Behav. 120, 33–42. doi:10.1016/j.pbb.2014.02.006
Song, X., Gong, Z., Liu, K., Kou, J., Liu, B., and Liu, K. (2020). Baicalin Combats Glutamate Excitotoxicity via Protecting Glutamine Synthetase from ROS-Induced 20S Proteasomal Degradation. Redox Biol. 34, 101559. doi:10.1016/j.redox.2020.101559
Spagnuolo, C., Cerella, C., Russo, M., Chateauvieux, S., Diederich, M., and Russo, G. L. (2011). Quercetin Downregulates Mcl-1 by Acting on mRNA Stability and Protein Degradation. Br. J. Cancer 105 (2), 221–230. doi:10.1038/bjc.2011.229
Su, T., Zhang, W. W., Zhang, Y. M., Cheng, B. C., Fu, X. Q., Li, T., et al. (2016). Standardization of the manufacturing procedure for Pinelliae Rhizoma Praeparatum cum Zingibere et Alumine. J. Ethnopharmacol 193, 663–669. doi:10.1016/j.jep.2016.09.038
Sun, L. M., Zhang, B., Wang, Y. C., He, H. K., Chen, X. G., and Wang, S. J. (2019). Metabolomic Analysis of Raw Pinelliae Rhizoma and its Alum-Processed Products via UPLC-MS and Their Cytotoxicity. Biomed. Chromatogr. 33 (2), e4411. doi:10.1002/bmc.4411
Teschke, R., Zhu, Y., and Jing, J. (2020). Herb-induced Liver Injury in Asia and Current Role of RUCAM for Causality Assessment in 11,160 Published Cases. J. Clin. Transl Hepatol. 8 (2), 200–214. doi:10.14218/JCTH.2020.00009
Tian, Y. G., Su, X. H., Liu, L. L., Kong, X. Y., and Lin, N. (2019). Overview of Hepatotoxicity Studies on Tripterygium Wilfordii in Recent 20 Years. Zhongguo Zhong Yao Za Zhi 44 (16), 3399–3405. doi:10.19540/j.cnki.cjcmm.20190527.408
Trott, O., and Olson, A. J. (2010). AutoDock Vina: Improving the Speed and Accuracy of Docking with a New Scoring Function, Efficient Optimization, and Multithreading. J. Comput. Chem. 31 (2), 455–461. doi:10.1002/jcc.21334
Tschoe, C., Bushnell, C. D., Duncan, P. W., Alexander-Miller, M. A., and Wolfe, S. Q. (2020). Neuroinflammation after Intracerebral Hemorrhage and Potential Therapeutic Targets. J. Stroke 22 (1), 29–46. doi:10.5853/jos.2019.02236
Venketasubramanian, N., Chen, C. L., Gan, R. N., Chan, B. P., Chang, H. M., Tan, S. B., et al. (2009). A Double-Blind, Placebo-Controlled, Randomized, Multicenter Study to Investigate Chinese Medicine Neuroaid Efficacy on Stroke Recovery (CHIMES Study). Int. J. Stroke 4 (1), 54–60. doi:10.1111/j.1747-4949.2009.00237.x
Voicu, A., Duteanu, N., Voicu, M., Vlad, D., and Dumitrascu, V. (2020). The Rcdk and Cluster R Packages Applied to Drug Candidate Selection. J. Cheminform 12 (1), 3. doi:10.1186/s13321-019-0405-0
Wang, J. R., Tong, T. T., Yau, L. F., Chen, C. Y., Bai, L. P., Ma, J., et al. (2016). Characterization of Oxygenated Metabolites of Ginsenoside Rg1 in Plasma and Urine of Rat. J. Chromatogr. B Analyt Technol. Biomed. Life Sci. 1026, 75–86. doi:10.1016/j.jchromb.2015.12.028
Wang, K. X., Gao, Y., Gong, W. X., Ye, X. F., Fan, L. Y., Wang, C., et al. (2020a). A Novel Strategy for Decoding and Validating the Combination Principles of Huanglian Jiedu Decoction from Multi-Scale Perspective. Front. Pharmacol. 11, 567088. doi:10.3389/fphar.2020.567088
Wang, K. X., Gao, Y., Lu, C., Li, Y., Zhou, B. Y., Qin, X. M., et al. (2020b). Uncovering the Complexity Mechanism of Different Formulas Treatment for Rheumatoid Arthritis Based on a Novel Network Pharmacology Model. Front. Pharmacol. 11, 1035. doi:10.3389/fphar.2020.01035
Wang, R. E., Hunt, C. R., Chen, J., and Taylor, J. S. (2011). Biotinylated Quercetin as an Intrinsic Photoaffinity Proteomics Probe for the Identification of Quercetin Target Proteins. Bioorg. Med. Chem. 19 (16), 4710–4720. doi:10.1016/j.bmc.2011.07.005
Wang, X., Wang, C., Pu, F., Lin, P., and Qian, T. (2014). Metabolite Profiling of Ginsenoside Rg1 after Oral Administration in Rat. Biomed. Chromatogr. 28 (10), 1320–1324. doi:10.1002/bmc.3164
Wang, Y., Zhang, S., Li, F., Zhou, Y., Zhang, Y., Wang, Z., et al. (2020c). Therapeutic Target Database 2020: Enriched Resource for Facilitating Research and Early Development of Targeted Therapeutics. Nucleic Acids Res. 48 (D1), D1031–D1041. doi:10.1093/nar/gkz981
Wu, B., Liu, M., and Zhang, S. (2007). Dan Shen Agents for Acute Ischaemic Stroke. Cochrane Database Syst. Rev. 2, CD004295. doi:10.1002/14651858.CD004295.pub3
Xie, C. L., Wang, W. W., Xue, X. D., Zhang, S. F., Gan, J., and Liu, Z. G. (2015). A Systematic Review and Meta-Analysis of Ginsenoside-Rg1 (G-Rg1) in Experimental Ischemic Stroke. Sci. Rep. 5, 7790. doi:10.1038/srep07790
Xing, J., Chen, X., and Zhong, D. (2005). Absorption and Enterohepatic Circulation of Baicalin in Rats. Life Sci. 78 (2), 140–146. doi:10.1016/j.lfs.2005.04.072
Xu, M., Chen, X., Gu, Y., Peng, T., Yang, D., Chang, R. C., et al. (2013). Baicalin Can Scavenge Peroxynitrite and Ameliorate Endogenous Peroxynitrite-Mediated Neurotoxicity in Cerebral Ischemia-Reperfusion Injury. J. Ethnopharmacol 150 (1), 116–124. doi:10.1016/j.jep.2013.08.020
Xue, A. (2020). Clinical Observation on Ditan Decoction and Qianzheng Powder in the Treatment of Acute CIS in the Recovery Period. Bright Chin. Med. 35 (23), 3740–3742.
Yan, S., Fangbo, M., Lu, L., and Zhiyan, G. (2017). Clinical Observation on Treatment of 32 Cases of Ischemic Apoplexy with Phlegm Stasis and Collateral Obstruction at the Recovery Stage with Huayu Ditan Decoction. J. Hunan Traditional Chin. Med. 33 (11), 44–45.
Yang, C., Hawkins, K. E., Doré, S., and Candelario-Jalil, E. (2019). Neuroinflammatory Mechanisms of Blood-Brain Barrier Damage in Ischemic Stroke. Am. J. Physiol. Cel Physiol 316 (2), C135–C153. doi:10.1152/ajpcell.00136.2018
Yang, F., Song, L., Wang, H., Wang, J., Xu, Z., and Xing, N. (2015). Combination of Quercetin and 2-Methoxyestradiol Enhances Inhibition of Human Prostate Cancer LNCaP and PC-3 Cells Xenograft Tumor Growth. PLoS One 10, e0128277. doi:10.1371/journal.pone.0128277
Yang, J., Tian, S., Zhao, J., and Zhang, W. (2020). Exploring the Mechanism of TCM Formulae in the Treatment of Different Types of Coronary Heart Disease by Network Pharmacology and Machining Learning. Pharmacol. Res. 159, 105034. doi:10.1016/j.phrs.2020.105034
Yang, J., Liu, M., Zhou, J., Zhang, S., Lin, S., and Zhao, H. (2011). Edaravone for Acute Intracerebral Haemorrhage. Cochrane Database Syst. Rev. 2, CD007755. doi:10.1002/14651858.CD007755.pub2
Yang, L., Fan, L., Wang, K., Chen, Y., Liang, L., Qin, X., et al. (2021a). Analysis of Molecular Mechanism of Erxian Decoction in Treating Osteoporosis Based on Formula Optimization Model. Oxid Med. Cel Longev 2021, 6641838. doi:10.1155/2021/6641838
Yang, M., Wu, G., Zhao, Q., Li, Y., and Wang, J. (2021b). Computational Drug Repositioning Based on Multi-Similarities Bilinear Matrix Factorization. Brief Bioinform 22. doi:10.1093/bib/bbaa267
Yang, R., Shen, Y. J., Chen, M., Zhao, J. Y., Chen, S. H., Zhang, W., et al. (2021c). Quercetin Attenuates Ischemia Reperfusion Injury by Protecting the Blood-Brain Barrier through Sirt1 in MCAO Rats. J. Asian Nat. Prod. Res., 1–12. doi:10.1080/10286020.2021.1949302
Yao, Z. J., Dong, J., Che, Y. J., Zhu, M. F., Wen, M., Wang, N. N., et al. (2016). TargetNet: a Web Service for Predicting Potential Drug-Target Interaction Profiling via Multi-Target SAR Models. J. Comput. Aided Mol. Des. 30 (5), 413–424. doi:10.1007/s10822-016-9915-2
Yi, L., and Hongyan, X. (2018). Clinical Research Progress of Xuefu Zhuyu Decoction in Treating Encephalopathy of Traditional Chinese Medicine. yunnan J. traditional Chin. Med. materia Med. 39 (08), 88–91.
Yu, H. L., Wang, W., Wu, H., Shen, M., Zhang, Y. B., and Li, S. H. (2019). Effect of Processing on Toxic Components Lectin from Four Kinds of Araceae Toxic Medicines. Zhongguo Zhong Yao Za Zhi 44 (24), 5398–5404. doi:10.19540/j.cnki.cjcmm.20190916.302
Zhang, J., Cai, W., Zhou, Y., Liu, Y., Wu, X., Li, Y., et al. (2015a). Profiling and Identification of the Metabolites of Baicalin and Study on Their Tissue Distribution in Rats by Ultra-high-performance Liquid Chromatography with Linear Ion Trap-Orbitrap Mass Spectrometer. J. Chromatogr. B Analyt Technol. Biomed. Life Sci. 985, 91–102. doi:10.1016/j.jchromb.2015.01.018
Zhang, L. L., Zhang, H. T., Cai, Y. Q., Han, Y. J., Yao, F., Yuan, Z. H., et al. (2016). Anti-inflammatory Effect of Mesenchymal Stromal Cell Transplantation and Quercetin Treatment in a Rat Model of Experimental Cerebral Ischemia. Cel Mol Neurobiol 36 (7), 1023–1034. doi:10.1007/s10571-015-0291-6
Zhang, Y., Yi, B., Ma, J., Zhang, L., Zhang, H., Yang, Y., et al. (2015b). Quercetin Promotes Neuronal and Behavioral Recovery by Suppressing Inflammatory Response and Apoptosis in a Rat Model of Intracerebral Hemorrhage. Neurochem. Res. 40 (1), 195–203. doi:10.1007/s11064-014-1457-1
Zheng, T., Jiang, H., Jin, R., Zhao, Y., Bai, Y., Xu, H., et al. (2019). Ginsenoside Rg1 Attenuates Protein Aggregation and Inflammatory Response Following Cerebral Ischemia and Reperfusion Injury. Eur. J. Pharmacol. 853, 65–73. doi:10.1016/j.ejphar.2019.02.018
Keywords: stroke, traditional Chinese medicine, compound-target network, synergy, neuroinflammation
Citation: Xu A, Wen Z-H, Su S-X, Chen Y-P, Liu W-C, Guo S-Q, Li X-F, Zhang X, Li R, Xu N-B, Wang K-X, Li W-X, Guan D-G and Duan C-Z (2022) Elucidating the Synergistic Effect of Multiple Chinese Herbal Prescriptions in the Treatment of Post-stroke Neurological Damage. Front. Pharmacol. 13:784242. doi: 10.3389/fphar.2022.784242
Received: 29 September 2021; Accepted: 14 February 2022;
Published: 09 March 2022.
Edited by:
Juei-Tang Cheng, Chang Jung Christian University, TaiwanCopyright © 2022 Xu, Wen, Su, Chen, Liu, Guo, Li, Zhang, Li, Xu, Wang, Li, Guan and Duan. This is an open-access article distributed under the terms of the Creative Commons Attribution License (CC BY). The use, distribution or reproduction in other forums is permitted, provided the original author(s) and the copyright owner(s) are credited and that the original publication in this journal is cited, in accordance with accepted academic practice. No use, distribution or reproduction is permitted which does not comply with these terms.
*Correspondence: Wen-Xing Li, bGl3ZW54aW5nMjAxNkBnbWFpbC5jb20=; Dao-Gang Guan, Z3VhbmRnMDkyOUBob3RtYWlsLmNvbQ==; Chuan-Zhi Duan, ZG9jdG9yX2R1YW56akAxNjMuY29t
†These authors have contributed equally to this work and share the first authorship
Disclaimer: All claims expressed in this article are solely those of the authors and do not necessarily represent those of their affiliated organizations, or those of the publisher, the editors and the reviewers. Any product that may be evaluated in this article or claim that may be made by its manufacturer is not guaranteed or endorsed by the publisher.
Research integrity at Frontiers
Learn more about the work of our research integrity team to safeguard the quality of each article we publish.