- 1Shenzhen Innovation Center for Small Molecule Drug Discovery Co., Ltd., Shenzhen, China
- 2Shenzhen NewDEL Biotech Co., Ltd., Shenzhen, China
Molecular generation (MG) via machine learning (ML) has speeded drug structural optimization, especially for targets with a large amount of reported bioactivity data. However, molecular generation for structural optimization is often powerless for new targets. DNA-encoded library (DEL) can generate systematic, target-specific activity data, including novel targets with few or unknown activity data. Therefore, this study aims to overcome the limitation of molecular generation in the structural optimization for the new target. Firstly, we generated molecules using the structure-affinity data (2.96 million samples) for 3C-like protease (3CLpro) from our own-built DEL platform to get rid of using public databases (e.g., CHEMBL and ZINC). Subsequently, to analyze the effect of transfer learning on the positive rate of the molecule generation model, molecular docking and affinity model based on DEL data were applied to explore the enhanced impact of transfer learning on molecule generation. In addition, the generated molecules are subjected to multiple filtering, including physicochemical properties, drug-like properties, and pharmacophore evaluation, molecular docking to determine the molecules for further study and verified by molecular dynamics simulation.
1 Introduction
Drug structural optimization (Stokes et al., 2020; Wang Z.-Y. et al., 2022) is to design new molecules with better specific properties, either to increase desired bioactivities or decrease side effects. In the early stages, the conception and evaluation of new proposed molecules rely on medicinal chemists’ experience and knowledge of basic chemistry and biology. Later, with the improved computer-aided drug programming level, the ligand-based quantitative structure-activity relationship (QSAR) model (Cherkasov et al., 2014) combined with molecular docking and molecular dynamics simulation were used for large numbers of molecule screening to obtain molecules efficiently. With the substantial increase in data and the continuous improvement of computing resources, deep learning (DL) has developed rapidly. This new tool facilitated drug development, especially structural optimization. Zhavoronkov et al. (Zhavoronkov et al., 2019) discovered a kinase inhibitor of DDR1 in 41 days by building a deep-learning molecular generation architecture GENTRL. Then, applying deep learning in drug design became one of the top 10 breakthrough technologies in MIT Technology Review 2020 (MIT Technology Review, 2020). Full use of this tool can explore more expansive chemical space and generate molecules of desired physicochemical and pharmacological properties, accelerating drug development (Xu et al., 2019; Arús-Pous et al., 2020; Kotsias et al., 2020).
The strategy for molecular generative models through deep learning can be divided into ligand-based and structure-based (also called receptor-based). Ligand-based molecule generation requires a set of experimentally validated active compounds. ML generates molecules by learning the common features of the active compounds (Liu et al., 2021; Wang M.-Y. et al., 2022). Structure-based molecular generation considers ligand and receptor interactions. Traditional structure-based molecular generation is a fragment-based approach that adds, deletes, or replaces chemical fragments of ligands in pockets (Batool et al., 2019; Krishnan et al., 2022). The Algorithm using the protein’s structural information to design new molecules has not been widely validated due to the limitation of high computational resource consumption (Skalic et al., 2019; Born et al., 2021; Grechishnikova, 2021). A structure-based molecular generation often requires three-dimensional information on the binding pockets within ligands (Wang M.-Y. et al., 2022; Long et al., 2022). Facing new targets, often there are neither revealed binding pockets nor experimentally validated ligands. The datasets used for ligand-based molecule generation usually come from public databases (such as CHEMBLE, ZINC, etc.), and the specific targets sub-datasets are generally needed to guide the structural optimization. Such a procedure has an unavoidable limitation because of its heavily dependent on public experimental data. For new targets, such dataset is severely lacking. Machine learning cannot be developed without an available dataset. This is the main reason for molecular generation, and even AIDD is still challenging to apply to the structure optimization of hit compounds for new targets. DNA-encoded library (DEL) (Dickson et al., 2019; Li et al., 2022; Nie et al., 2022; Song et al., 2020; Yang et al., 2022; Zhao et al., 2019; Zhao et al., 2022) is a powerful tool from combinatorial screening and DNA-encoded technology. Compared with traditional high-throughput screening (HTS), DEL technology can efficiently and economically generate a large amount of affinity data for specific targets, including new target data (hundreds of billions scale) (Buller et al., 2010; Kalliokoski, 2015). Therefore, using the DEL dataset, mainly the structure-affinity relationship, for molecular generation could be a reasonable solution to the problem of efficient structural optimization for new target drug development.
Deep learning generative algorithms have been explored for aided drug design. Generally, standard inputs in generative models are linear input symbols like Simplified molecular input line entry specification (SMILES) and molecular graphs. Common generative model architectures include recurrent neural networks (RNNs) (Bjerrum and Threlfall, 2017; Segler et al., 2018; Kotsias et al., 2020), autoencoders [AE, VAE (variational AE), AAE (adversarial AE)] (Kingma and Welling, 2013; Rezende et al., 2014; Makhzani et al., 2015), generative adversarial networks (Goodfellow et al., 2020). Optimization strategies for generative models include transfer learning (Segler et al., 2018), Bayesian optimization (Gómez-Bombarelli et al., 2018), reinforcement learning (Wang et al., 2021), and conditional generation (Li et al., 2018). Transfer learning is a strategy for transferring knowledge from pre-learned tasks to improve learning performance. Public datasets are usually needed for pre-training till obtaining a greater probability of generating valid molecules. Subsequently, the pre-trained model is retrained using known active molecules. Generally, the overall distribution of the pre-trained CHEMBL or ZINC large dataset is quite different from that of specific target active molecules, negatively affecting transfer learning (Zhao et al., 2014). Transfer learning using the DEL dataset is expected to address this obstacle effectively. The DEL dataset herein is composed of 3 groups of building blocks. We used the high-affinity molecules from DEL (which appeared as compounds with high count values in DEL) to reduce the distribution inconsistency between the pre-trained model and transfer learning. The beneficial effect of transfer learning herein is confirmed and consistent with the DEL dataset. In this experiment, we used the molecular dataset with higher counts in DEL, molecules with more potent binding force to the target 3CLpro, for transfer learning, thereby increasing the probability of generating active molecules.
In this study, we set out to solve the following challenges: using DEL technology to construct two DEL libraries for 3CLpro and performing data analysis combined with chemical synthesis. Active hit compounds H1 and H2 (Scheme 1) were found via bioactivity assay. Subsequently, the own-built DEL dataset was used to establish a molecular generative model to obtain a dataset with broad chemical space distribution. The obtained molecule dataset was directly applied with several subsequent filtering steps. On the other hand, molecules with high count values in the DEL dataset were defined as positive samples for transfer learning to obtain another dataset. The above two datasets were filtered by the druggability and pharmacophore model. Finally, the obtained molecules were verified by molecular docking and dynamics simulation, which confirmed the potential bioactivity of the newly designed molecule (Figure 1).
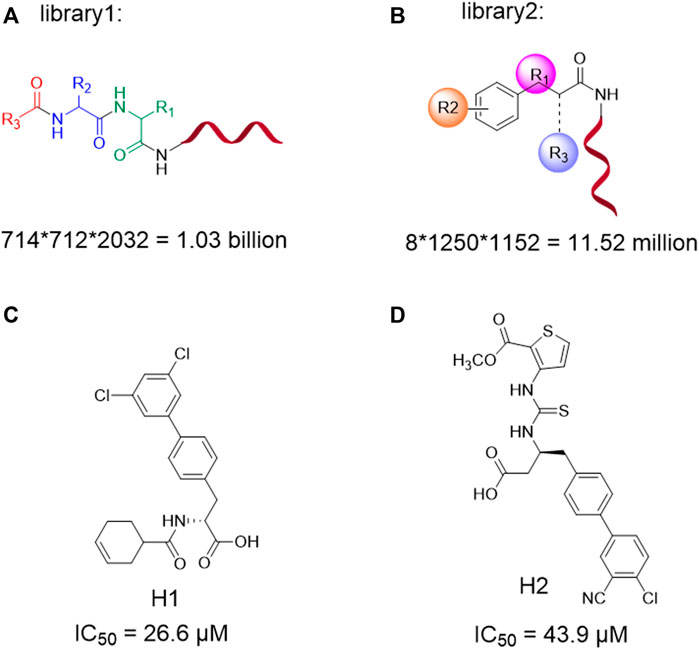
SCHEME 1. DNA-encoded library1 and library2 designed information (A,B); Structures and corresponding inhibition activity for 3CLpro (IC50/µM) of H1 and H2 (C,D).
2 Materials and experiments
2.1 DNA-encoded libraries screening, chemical synthesis, and bio experiments
Supporting information describes DEL screening, chemical synthesis, bio-activity experiments, and compound characterization.
2.2 Machine learning modeling
2.2.1 Data preparation
The two DEL libraries are combined as a dataset containing 1.04 billion molecules with corresponding count and enrichment fold (EF) values. We provide the information of DEL in the supplement material. We cut out the data with very low count, the remaining data is 3,702,672. Then it was divided into a training set and a test set (0.8/0.2), of which the training set and test set have 2,962,138 and 740,534 molecules, respectively. First, the training set is used to train the molecule generation model. Then, molecules 18,129) with higher count values in DEL were selected as the positive samples of transfer learning to fine-tune the pre-trained model.
2.2.2 Molecular generation
SMILES or molecular graphs are commonly used for molecular representation. SMILES is a text of molecular structures (Weininger, 1988). Molecular graphs represent the structure of molecules as graphs, where the edges of the graph represent bonds and the dots represent atomic structures (Sun et al., 2020). Molecules were represented as SMILES in three models, RNN, VAE, and AAE, to perform molecule generation. We used pytorch, sklearn, numpy, rdkit,umap-learn, and MOSES benchmark platform (Polykovskiy et al., 2020) to complete the experiments.
Recurrent neural network (RNN) (Segler et al., 2018) are designed to learn sequential data such as text or speech. The SMILES in DEL are made into a corpus. RNN can learn the grammatical information about the SMILES to know which parts of molecules tend to be connected. RNN can generate sequences through forward propagation (Bjerrum and Threlfall, 2017). By treating the molecule generation process as a series of steps and sampling the network at each step, generating effective molecules is highly probable and structurally similar to the training molecules. The architecture used in RNN consists of an embedding layer, three LSTM layers, and a linear layer.
Variational autoencoder (VAE) (Bowman et al., 2015) consists of an encoder and a decoder. The former encodes the input data into a latent vector, which obeys the Gaussian Distribution. The decoder restores the latent vector result to the target sample. SMILES are used as the model’s input and output to establish a VAE model. The VAE architecture consists of an embedding layer, an encoder layer, and a decoder layer. The encoder and decoder layers consist of a GRU layer and two linear layers.
Adversarial Autoencoder (AAE) (Makhzani et al., 2015) is similar to VAE principally. The difference is that based on the encoder and the decoder, a discriminator is introduced, which is responsible for distinguishing the calculated latent vector in the encoder from the real sample. The encoder and decoder are still accountable for encoding and reconstructing the data. AAE also uses SMILES as input and output. The AAE’s encoder part includes an embedding layer, an LSTM layer, and a linear layer. The decoder consists of two linear layers, one embedding layer, and one LSTM layer. The discriminator consists of two linear layers where the activation function is ELU (Clevert et al., 2015).
2.2.3 Transfer learning
Transfer learning (Amabilino et al., 2020) is a fine-tuning model technique that fixes the original model’s specific parameters while others are still training and updating. This technique aims to streamline expansive chemical space in the generative model, searching for target molecules in the relatively small chemical space. The model is retrained by inputting molecules with high-count values to generate more distribution-similar molecules than those with high-count values. To AAE, we fine-tune the decoder’s last linear layer and the discriminator’s last linear layer. To VAE, the last two linear layers of the decoder’s model are fine-tuned. To RNN, we also fine-tune the last linear layer.
2.2.4 Evaluation metrics
Each model generated 10,000 molecules, which were evaluated using the evaluation metrics provided in Moses (Polykovskiy et al., 2020), including valid, unique, novelty, internal diversity (IntDiv), and scaffold similarity (Scaff).
Validity is the proportion of valid molecules in the generated molecules.
Uniqueness is the proportion of molecules not duplicated in the generated molecules.
Novelty is the proportion of molecules that do not exist in the training set.
Internal diversity (IntDiv) (Benhenda, 2018) is a metric to assess the chemical variety of generated molecules. The value range is [0,1]. The higher value means higher diversity of the generated molecules.
Scaffold similarity (Scaff) represents the similarity between scaffolds in the generated set and reference dataset. The value range is [0,1]. The higher the value of Scaff, the more similar the two are.
2.2.5 Filtering
The number of molecules generated by each model is 1,000,000 molecular datasets. First, the dataset was filtered for validity and reproducibility, followed by drug-likeness: 250 ≤ MW ≤ 750, logP ≤ 5, HBD ≤ 5, HBA ≤ 10, RB < 10, and 0.5 < QED. The next step was improving drugability by applying Medicinal Chemistry filters (MCFs) (Kalgutkar et al., 2005) and Pan Assay Interference Compounds (PAINS) filtering (Baell and Holloway, 2010). Without transfer learning, RNN, AAE, and VAE have no significant performance differences and predicted affinity distribution. Therefore, these datasets are merged and divided into groups depending on whether transfer learning is applied or not. 5000 molecules were obtained after pharmacophore filtration. Finally, molecular docking was employed, and molecule with higher docking score was selected for molecular dynamics simulation.
2.2.6 Chemical space visualization
The remained SMILES after validity and repeatability filtration and the original 3,702,672 SMILES from the DEL dataset were transformed into Morgan fingerprints with 1024 dimensions and 2 radius (Rogers and Hahn, 2010). These fingerprints were then used to build a UMAP(Uniform manifold approximation and projection) (metric = “jaccard,” n_components = 2) model for dimensionality reduction visualization (McInnes et al., 2018).
2.2.7 Affinity modeling
The model for affinity prediction was established according to our previous study (Xiong et al., 2022). First, we sorted the molecules in the DEL dataset by the count value, then oversampled the top 10,000 ranked molecules by ten times. The step was set as 0, and every other step of the remaining molecules was sampled to form a training set.
2.2.8 3D conformation and pharmacophore-based screening
The 3D molecular similarity was calculated through the shape and color similarity score (SC score), which represents the pharmacophoric feature similarity (Landrum et al., 2006) and the shape similarity (Putta et al., 2005). This score was used for the previously generated dataset. The 3D similarity score is a floating point value in the range of [0, 1], with a higher value indicating higher similarity between candidate and reference molecules. The native ligand in PDB:7L13 from the RSC-PDB database was used as a reference structure (Zhang et al., 2021). 100 conformations were generated for each molecule from the dataset using the RDKit UFF (Universal Force Field) force field. The lowest energy conformation was applied for the next step.
2.2.9 Molecular docking
The A-chain of the complex PDB:7L13 (resolution 2.17 Å) of 3CLpro protein was split as a docking template to obtain accurate docking results. Subsequently, the complex was preprocessed using the Protein Preparation Wizard module of the Maestro suite (version: 13.1.141, Schrödinger Inc.) with the default setting, including the addition of hydrogen and side chains, removal of water molecules, and calculation of partial charges and protonation states using the OPLS4 force field (Poltev et al., 1996). Followed by a grid generation module, a similar-sized grid box centered on the native ligand was made to determine the binding pocket. All molecules were preprocessed by the LigPrep module. The ionization states were calculated using Epik (Shelley et al., 2007) at pH = 7.0 ± 2.0. Finally, all molecules were docked into the binding pocket within the grid and evaluated using the standard precision (SP) of Glide-v9.4. The scale factor and partial charge intercept are set to 0.8 and 0.15, respectively. 1000 poses per ligand were generated for docking evaluation. Post-docking binding site analysis and generation of interaction graphs were finished using Maestro.
2.2.10 Molecular dynamics simulations
Amolecular dynamics simulation was carried out to analyze further the dynamic interaction process between protein and ligand and the stability of binding status. Molecular dynamics simulation is a popular technique to study protein motion by tracking its conformational changes over time (Collier et al., 2020). Molecular interaction and visualization analysis based on SP docking results, the top-ranked molecules were used for the molecular dynamics simulation (MD-simulation) study. MD-simulation was performed using the GROMACS software package (version 2021.5) (Rakhshani et al., 2019). The AMBER14SB force field parameter was used for the protein. The ligand atomic charge was calculated using the B3LYP/6-31G* basis set. The ligand topology was computed using the GAFF2 force field parameter. The TIP3P water model was used to add Na+ and Cl− ions to neutralize the charge. Electrostatic interactions are handled separately using the Particle Mesh Ewald (PME) and Verlet algorithms. The heavy atoms of the protein are constrained, and the energy minimization is carried out through 50,000 steps using the steepest descent method. The simulated system was equilibrated for 100 ps using a canonical ensemble (NVT) and an isothermal-isobaric ensemble (NPT). Both van der Waals and Coulomb interactions were calculated using a cutoff of 1.4 nm. Afterward, the system was run at constant temperature (300 K) and constant pressure (1 bar) for 100 ns molecular dynamics simulations with a time step of 2 fs and trajectory data saved every 5 ps. Finally, the ligand and protein complex’s root mean square deviation (RMSD, Å) at 100 ns was measured. By examining the interaction of the ligand with active site residues and the structural changes of the complex, the complexes were considered stable.
3 Results and discussion
3.1 Machine learning modeling
3.1.1 Evaluation of molecular generative models
We evaluate each model’s validity, uniqueness, novelty, intDiv, and Scaff metrics (Tables 1, 2). The validity and uniqueness indicators of all models perform satisfactorily, indicating that the models can learn the grammatical information of the SMILES structure. The performance of novelty and IntDiv indicators is relatively poor, meaning that the model’s generalization ability may not be strong enough. VAE and RNN have higher Scaff values, meaning that the model can generate the same skeleton as the training set, but the ability of generating new skeleton is weak, while AAE is the opposite. In other words, the generated dataset and the training dataset had an apparent overlap. Especially after using transfer learning, Novelty’s metrics dropped further. A more complex model may be beneficial to address such a problem, so the MCMG (Multi-constraint molecular generation) model was also established (Wang et al., 2021). Unfortunately, MCMG performed relatively poorly in affinity prediction, so we decided not to analyze it further (Supplementary Figure S13).
3.1.2 Chemical space visualization
The results visualization using UMAP dimensionality reduction are shown in Figure 2. The molecules generated by each model closely resemble the chemical space distribution of DEL’s. This indicates that the model could learn molecular distribution sufficiently from the source dataset.
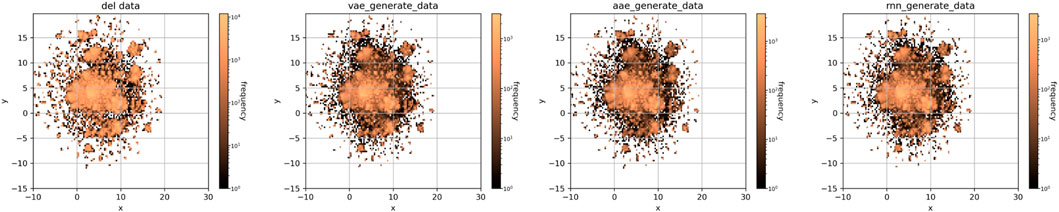
FIGURE 2. Dimensional reduction visualization of the training and generated molecular datasets (from left to right: DEL, VAE, AAE, and RNN).
3.1.3 Affinity model performance
Figure 3 shows the distribution of the molecules from each model’s affinity predictions. The molecules distribution without transfer learning is mainly located in the area of 0.1–0.2, while the corresponding values after using transfer learning are mostly above 0.2. Such improved affinity indicates the beneficial effect of transfer learning, which expectedly to improve the success rate and efficiency for further structural optimization. Scheme 2 shows the representative molecules with high affinity scores, which were expected to be potentially bio-active.
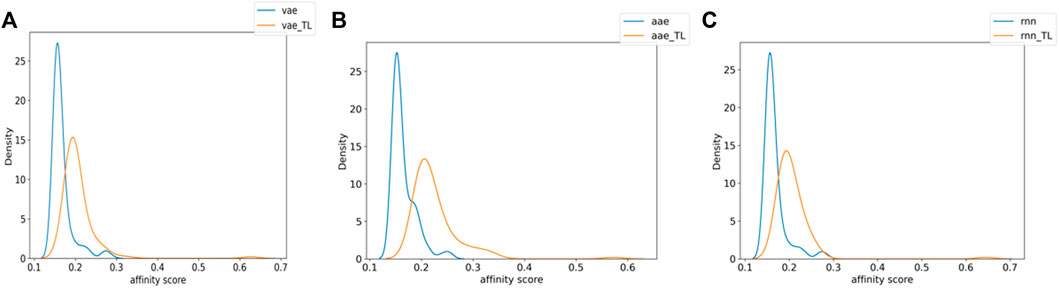
FIGURE 3. The numerical affinity distribution of molecules generated by each model with or without transfer learning [(A) VAE, (B) AAE, (C) RNN].
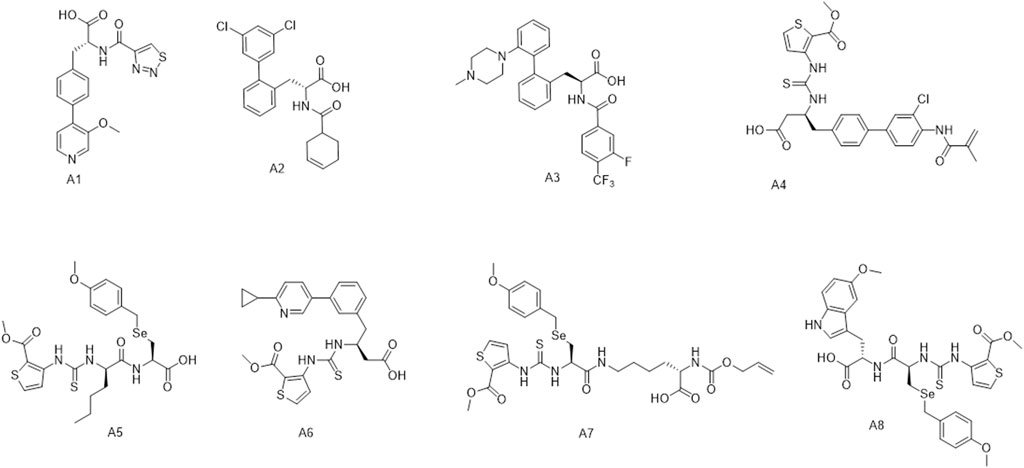
SCHEME 2. The representative molecules (A1–A8) with high affinity scores according to affinity model.
3.1.4 Molecular docking
According to the calculated SC scores of all molecules in the dataset and native ligand, 5,000 ligands with the highest SC score were selected for the follow-up study.
Molecular docking was utilized to analyze the 3D conformational and pharmacophore-based screens and to study the structural basis of the interaction between 3CLPro and ligands. First, the reliability of the glide docking algorithm (standard precision mode, enhanced conformational sampling by four times) was confirmed by re-docking the native ligand to the receptor.
The re-docked conformation was presented in supporting information. Subsequently, the selected 5000 molecules were preliminarily docked to the revealed binding site using standard precision mode. According to the docking evaluation score and molecular conformation, 500 different conformations were selected, and four times enhanced conformational sampling was used to generate the ligand-binding pose more accurately.
In addition, the docking scores with and without transfer learning for pharmacophoric models were analyzed (Table 3). Applying transfer learning, 4.9% of ligands possess a score greater than 8, while 0.3% have a score higher than 9. In contrast, the corresponding values without transfer learning are 3.3% and 0.1%. This result is consistent with the affinity model, indicating that transfer learning can effectively increase the percentage of positive samples.
Moreover, the molecules filtered by the affinity model also performed molecular docking as a pharmacophoric model. This aims to explore the possibility of replacing an external pharmacophore model with an own-built affinity model. The docking scores of the filtered molecules were combined in Table 3 for comparison. We found an exciting revelation that the proportion of molecules filtered by the affinity model with docking scores of 9–11 was twice as high as that from the pharmacophore model. Therefore, replacing pharmacophore filtering with affinity models alone may be a promising option to utilize DEL’s datasets more fully.
3.1.5 Binding free energy calculation
The binding free energy can be used as a reference standard for assessing the activity of molecules. Generally, the lower the binding value means, the more stable the complex formed is. The ligands’ binding free energies were calculated by psp-v6.7 MMGBSA. The self-established ADMET prediction model and Maestro’s QikProp module evaluated the corresponding properties of molecules with better conformation. In fact, our ADMET prediction model is similar to ADMETlab (Dong et al., 2018). Molecules N1-N8 were finally selected for follow-up research considering the above ADMET properties with results of binding energy calculation (Scheme 3).
From Table 4, the binding free energies (dG_Bind) of N1 to N8 indicate their potential biological activities. Van der Waals energy (dG_Bind_vdW) shows that hydrophobic interaction is the main contributor to the ligand binding process. According to the molecular docking conformation, we selected N1 and N2 for subsequent research, in which the position and interaction of N1 and N2 on receptors are consistent with the previous report (Figure 4) (Zhang et al., 2021; Stille et al., 2022).
3.1.6 Molecular dynamics simulation
To further analyze the interaction-related atomic details between molecules and 3CLPro, GROMACS was applied for molecular dynamics simulations using the above docking results. 100 ns run time for MD simulation is considered sufficient for side chain rearrangement. The result will confirm whether or not the complex remains in the most stable association. Since N2 is believed to be more compatible with the receptor pocket (ligand conformation), and the calculation of MMGBSA binding free energy indicates that N2 has a stronger interaction with the receptor, molecular dynamics simulations of the complex formed by N2 were conducted.
RMSD values plotted over the simulation time revealed a stable kinetic equilibrium of the complex. In detail, the 3CLPro protein with N2 and the ligand N2 showed steady kinetics after 30 and 50 ns, respectively (Figure 5). By monitoring the fluctuation of RMSD, each system is in the range of 2Å after 50 ns. This suggests that the complex undergoes a conformational change during the simulation that promotes tight binding between the N2 and receptor, and finally, the system reaches a steady state. The RMSF value showed minimal fluctuation, and it remained in the range of 0.05–0.2 nm throughout the simulation period for most residues, except that a peak in RMSF value was observed only at residue 1. The less fluctuating performance confirms the strong attachment of the ligand to the protein (Figure 6).
4 Conclusion
As far as we know, this is the first study that DEL’s dataset has been used for the molecular generation, which will promote the development of the application field of DEL combined with AI. This study preliminarily found that it may be feasible to use DEL data instead of public databases for molecular generation. In particular, for the new target pipeline, molecular generation and affinity model establishment based on DEL data are expected to become a tool with dual functions of drug discovery and further structural optimization. This advantage would be difficult to achieve with public databases due to the scarcity of datasets.
Data availability statement
The original contributions presented in the study are included in the article/Supplementary Material, further inquiries can be directed to the corresponding authors.
Author contributions
All authors listed have made a substantial, direct, and intellectual contribution to the work and approved it for publication.
Funding
This work was supported by the Industry and Information Technology Bureau of Shenzhen Municipality (No. 20200627103531228, for Shenzhen Innovation Center for Small Molecule Drug Discovery Co., Ltd.).
Conflict of interest
All authors were employed by either Shenzhen Innovation Center for Small Molecule Drug Discovery Co., Ltd. or Shenzhen NewDEL Biotech Co., Ltd.
Publisher’s note
All claims expressed in this article are solely those of the authors and do not necessarily represent those of their affiliated organizations, or those of the publisher, the editors and the reviewers. Any product that may be evaluated in this article, or claim that may be made by its manufacturer, is not guaranteed or endorsed by the publisher.
Supplementary material
The Supplementary Material for this article can be found online at: https://www.frontiersin.org/articles/10.3389/fphar.2022.1085665/full#supplementary-material
References
Amabilino, Silvia, Pogany, P., Pickett, S. D., and Green, D. V. S. (2020). Guidelines for recurrent neural network transfer learning-based molecular generation of focused libraries. J. Chem. Inf. Model. 6012, 5699–5713. doi:10.1021/acs.jcim.0c00343
Arús-Pous, J., Patronov, A., Bjerrum, E. J., Tyrchan, C., Reymond, J.-L., Chen, H., et al. (2020). SMILES-based deep generative scaffold decorator for de -novo drug design. J. Cheminform. 12 (1), 38–18. doi:10.1186/s13321-020-00441-8
Baell, J. B., and Holloway, G. A. (2010). New substructure filters for removal of pan assay interference compounds (PAINS) from screening libraries and for their exclusion in bioassays. J. Med. Chem. 53 (7), 2719–2740. doi:10.1021/jm901137j
Batool, M., Ahmad, B., and Choi, S. (2019). A structure-based drug discovery paradigm. Int. J. Mol. Sci. 20 (11), 2783. doi:10.3390/ijms20112783
Benhenda, M. (2018). Can AI reproduce observed chemical diversity? bioRxiv [Preprint] (Accessed October 10, 2022). doi:10.1101/292177
Bjerrum, E. J., and Threlfall, R. (2017). Molecular generation with Recurrent Neural Networks (RNNs) arXiv [preprint] (Accessed October 10, 2022). doi:10.48550/arXiv.1705.04612
Born, J., Manica, M., Cadow, J., Markert, G., Mill, N. A., Filipavicius, M., et al. (2021). Data-driven molecular design for discovery and synthesis of novel ligands: A case study on SARS-CoV-2. Mach. Learn, Sci. Technol. 2 (2), 025024. doi:10.1088/2632-2153/abe808
Bowman, S. R., Vilnis, L., Vinyals, O., Dai, A. M., Jozefowicz, R., and Bengio, S. (201506349). Generating sentences from a continuous space. arXiv [Preprint] (Accessed October 10, 2022). doi:10.48550/arXiv.1511
Buller, F., Mannocci, L., Scheuermann, Jr, and Neri, D. (2010). Drug discovery with DNA-encoded chemical libraries. Bioconjug. Chem. 21 (9), 1571–1580. doi:10.1021/bc1001483
Cherkasov, A., Muratov, E. N., Fourches, D., Varnek, A., Baskin, II, Cronin, M., et al. (2014). QSAR modeling: Where have you been? Where are you going to? J. Med. Chem. 57 (12), 4977–5010. doi:10.1021/jm4004285
Clevert, D.-A., Unterthiner, T., and Hochreiter, S. (2015). Fast and accurate deep network learning by exponential linear units (elus). arXiv [preprint] Available at:Accessed Oct 10, 2022. doi:10.48550/arXiv.1511.07289
Collier, T. A., Piggot, T. J., and Allison, J. R. (2020). “Molecular dynamics simulation of proteins,” in Protein nanotechnology (Springer), Berlin, Germany, 311–327.
Dickson, P., and Kodadek, T. (2019). Chemical composition of DNA-encoded libraries, past present and future. Org. Biomol. Chem. 17 (19), 4676–4688. doi:10.1039/c9ob00581a
Dong, Jie, Wang, N. N., Yao, Z. J., Zhang, L., Cheng, Y., Ouyang, D., et al. (2018). ADMETlab: A platform for systematic ADMET evaluation based on a comprehensively collected ADMET database. J. Cheminform. 10 (1), 29–11. doi:10.1186/s13321-018-0283-x
Gómez-Bombarelli, R., Wei, J. N., Duvenaud, D., Hernández-Lobato, J. M., Sánchez-Lengeling, B., Sheberla, D., et al. (2018). Automatic chemical design using a data-driven continuous representation of molecules. ACS Cent. Sci. 4 (2), 268–276. doi:10.1021/acscentsci.7b00572
Goodfellow, I., Pouget-Abadie, J., Mirza, M., Xu, B., Warde-Farley, D., Ozair, S., et al. (2020). Generative adversarial networks. Commun. ACM 63 (11), 139–144. doi:10.1145/3422622
Grechishnikova, D. (2021). Transformer neural network for protein-specific de novo drug generation as a machine translation problem. Sci. Rep. 11 (1), 1–13. doi:10.1038/s41598-020-79682-4
Kalgutkar, A. S., Gardner, I., Obach, R. S., Shaffer, C. L., Callegari, E., Henne, K. R., et al. (2005). A comprehensive listing of bioactivation pathways of organic functional groups. Curr. Drug Metab. 6 (3), 161–225. doi:10.2174/1389200054021799
Kalliokoski, T. (2015). Price-focused analysis of commercially available building blocks for combinatorial library synthesis. ACS Comb. Sci. 17 (10), 600–607. doi:10.1021/acscombsci.5b00063
Kingma, D. P., and Welling, M. (2013). Auto-encoding variational bayes. arXiv [Preprint] (Accessed October 10, 2022). doi:10.48550/arXiv.1312.6114
Kotsias, P.-C., Arús-Pous, J., Chen, H., Engkvist, O., Tyrchan, C., and Bjerrum, E. J. (2020). Direct steering of de novo molecular generation with descriptor conditional recurrent neural networks. Nat. Mach. Intell. 2 (5), 254–265. doi:10.1038/s42256-020-0174-5
Krishnan, S. R., Bung, N., Vangala, S. R., Srinivasan, R., Bulusu, G., and Roy, A. (2022). De novo structure-based drug design using deep learning. J. Chem. Inf. Model. 62 (21), 5100–5109. doi:10.1021/acs.jcim.1c01319
Landrum, G. A., Penzotti, J. E., and Putta, S. (2006). Feature-map vectors: A new class of informative descriptors for computational drug discovery. J. Comput. Aided. Mol. Des. 20 (12), 751–762. doi:10.1007/s10822-006-9085-8
Li, X., Zhang, J., Liu, C., Sun, J., Li, Y., Zhang, G., et al. (2022). Aryl diazonium intermediates enable mild DNA-compatible CC bond formation for medicinally relevant combinatorial library synthesis. Chem. Sci. 13, 13100–13109. doi:10.1039/d2sc04482j
Li, Y.-J., Vinyals, O., Dyer, C., Pascanu, R., and Battaglia, P. (2018). Learning deep generative models of graphs. arXiv [Preprint] (Accessed October 10, 2022). doi:10.48550/arXiv.1803
Liu, R.-Z., Song, J.-K., Liu, A.-L., and Du, G.-H. (2021). Progress on the application of artificial intelligence technology in ligand-based and receptor structure-based drug screening. Acta Pharm. Sin. 12, 2136–2145.
Long, S.-Y., Zhou, Y., Dai, X.-Y., and Zhou, H. (2022). Zero-shot 3D drug design by sketching and generating. arXiv [Preprint] (Accessed October 10, 2022). doi:10.48550/arXiv.2209.13865
Makhzani, A., Shlens, J., Jaitly, N., Goodfellow, I., and Frey, B. (2015). Adversarial autoencoders. arXiv [Preprint] (Accessed October 10, 2022). doi:10.48550/arXiv.1511
McInnes, L., Healy, J., and Melville, J. (2018). Umap: Uniform manifold approximation and projection for dimension reduction. arXiv [Preprint] (Accessed October 10, 2022). doi:10.48550/arXiv.1802
MIT Technology Review (2020). 10 Breakthrough technologies 2020. Available at: https://www.technologyreview.com/10-breakthrough-technologies/2020/#ai-discovered-molecules (Accessed October 10, 2022).
Nie, Q., Zhong, S., Li, Y., Zhang, G., and Li, Y. (2022). Second-generation DNA-encoded multiple display on a constant macrocyclic scaffold enabled by an orthogonal protecting group strategy. Chin. Chem. Lett. 33 (5), 2559–2563. doi:10.1016/j.cclet.2021.09.041
Poltev, V., Malenkov, G., Gonzalez, E., Teplukhin, A., Rein, R., Shibata, M., et al. (1996). Modeling DNA hydration: Comparison of calculated and experimental hydration properties of nuclic acid bases. J. Biomol. Struct. Dyn. 13 (4), 717–726. doi:10.1080/07391102.1996.10508884
Polykovskiy, D., Zhebrak, A., Sanchez-Lengeling, B., Golovanov, S., Tatanov, O., Belyaev, S., et al. (2020). Molecular sets (MOSES): A benchmarking platform for molecular generation models. Front. Pharmacol. 11, 565644. doi:10.3389/fphar.2020.565644
Putta, S., Landrum, G. A., and Penzotti, J. E. (2005). Conformation mining: An algorithm for finding biologically relevant conformations. J. Med. Chem. 48 (9), 3313–3318. doi:10.1021/jm049066l
Rakhshani, H., Dehghanian, E., and Rahati, A. (2019). Enhanced GROMACS: Toward a better numerical simulation framework. J. Mol. Model. 25 (12), 355–358. doi:10.1007/s00894-019-4232-z
Rezende, D. J., Mohamed, S., and Wierstra, D. (2014). “Stochastic backpropagation and approximate inference in deep generative models,” in Proceedings of the International conference on machine learning: PMLR), Beijing China, June 21-26,2014, 1278–1286.
Rogers, D., and Hahn, M. (2010). Extended-connectivity fingerprints. J. Chem. Inf. Model. 50 (5), 742–754. doi:10.1021/ci100050t
Segler, M. H., Kogej, T., Tyrchan, C., and Waller, M. P. (2018). Generating focused molecule libraries for drug discovery with recurrent neural networks. ACS Cent. Sci. 4 (1), 120–131. doi:10.1021/acscentsci.7b00512
Shelley, J. C., Cholleti, A., Frye, L. L., Greenwood, J. R., Timlin, M. R., and Uchimaya, M. (2007). Epik: A software program for pK a prediction and protonation state generation for drug-like molecules. J. Comput. Aided. Mol. Des. 21 (12), 681–691. doi:10.1007/s10822-007-9133-z
Skalic, M., Sabbadin, D., Sattarov, B., Sciabola, S., and De Fabritiis, G. (2019). From target to drug: Generative modeling for the multimodal structure-based ligand design. Mol. Pharm. 16 (10), 4282–4291. doi:10.1021/acs.molpharmaceut.9b00634
Song, M., and Hwang, G. T. (2020). DNA-encoded library screening as core platform technology in drug discovery: Its synthetic method development and applications in DEL synthesis. J. Med. Chem. 63 (13), 6578–6599. doi:10.1021/acs.jmedchem.9b01782
Stille, J. K., Tjutrins, J., Wang, G., Venegas, F. A., Hennecker, C., Rueda, A. M., et al. (2022). Design, synthesis and in vitro evaluation of novel SARS-CoV-2 3CLpro covalent inhibitors. Eur. J. Med. Chem. 229, 114046. doi:10.1016/j.ejmech.2021.114046
Stokes, J. M., Yang, K., Swanson, K., Jin, W., Cubillos-Ruiz, A., Donghia, N. M., et al. (2020). A deep learning approach to antibiotic discovery. Cell 180 (4), 688–702. doi:10.1016/j.cell.2020.01.021
Sun, M., Zhao, S., Gilvary, C., Elemento, O., Zhou, J., and Wang, F. (2020). Graph convolutional networks for computational drug development and discovery. Brief. Bioinform. 21 (3), 919–935. doi:10.1093/bib/bbz042
Wang, J.-K., Hsieh, C.-Y., Wang, M.-Y., Wang, X.-R., Wu, Z.-X., Jiang, D.-J., et al. (2021). Multi-constraint molecular generation based on conditional transformer, knowledge distillation and reinforcement learning. Nat. Mach. Intell. 3 (10), 914–922. doi:10.1038/s42256-021-00403-1
Wang, M.-Y., Hsieh, C.-Y., Wang, J.-K., Wang, D., Weng, G.-Q., Shen, C., et al. (2022a). Relation : A deep generative model for structure-based de novo drug design. J. Med. Chem. 65 (13), 9478–9492. doi:10.1021/acs.jmedchem.2c00732
Wang, Z.-Y., Liu, M., Luo, Y.-Z., Xu, Z., Xie, Y.-C., Wang, L.-M., et al. (2022b). Advanced graph and sequence neural networks for molecular property prediction and drug discovery. Bioinformatics 38 (9), 2579–2586. doi:10.1093/bioinformatics/btac112
Weininger, D. (1988). SMILES, a chemical language and information system. 1. Introduction to methodology and encoding rules. J. Chem. Inf. Model. 28 (1), 31–36. doi:10.1021/ci00057a005
Xiong, F., Yu, M.-G., Xu, H.-G., Zhong, Z.-M., Li, Z.-W., Guo, Y.-H., et al. (2022). Discovery of TIGIT inhibitors based on DEL and machine learning. Front. Chem. 10, 982539. doi:10.3389/fchem.2022.982539
Xu, Y.-J., Lin, K.-J., Wang, S.-W., Wang, L., Cai, C.-J., Song, C., et al. (2019). Deep learning for molecular generation. Future Med. Chem. 11 (6), 567–597. doi:10.4155/fmc-2018-0358
Yang, S., Zhao, G., Gao, Y., Sun, Y., Zhang, G., Fan, X., et al. (2022). In-solution direct oxidative coupling for the integration of sulfur/selenium into DNA-encoded chemical libraries. Chem. Sci. 13 (9), 2604–2613. doi:10.1039/d1sc06268a
Zhang, C.-H., Stone, E. A., Deshmukh, M., Ippolito, J. A., Ghahremanpour, M. M., Tirado-Rives, J., et al. (2021). Potent noncovalent inhibitors of the main protease of SARS-CoV -2 from molecular sculpting of the drug perampanel guided by free energy perturbation calculations. ACS Cent. Sci. 7 (3), 467–475. doi:10.1021/acscentsci.1c00039
Zhao, G., Huang, Y., Zhou, Y., Li, Y., and Li, X. (2019). Future challenges with DNA-encoded chemical libraries in the drug discovery domain. Expert Opin. Drug Discov. 14 (8), 735–753. doi:10.1080/17460441.2019.1614559
Zhao, G., Zhong, S., Zhang, G., Li, Y., and Li, Y. (2022). Reversible covalent headpiece enables interconversion between double-and single-stranded DNA-encoded chemical libraries. Angew. Chem. Int. Ed. Engl. 134 (7), e202115157. doi:10.1002/anie.202115157
Zhao, P.-L., Hoi, S. C. H., Wang, J.-L., and Li, B. (2014). Online transfer learning. Artif. Intell. 216, 76–102. doi:10.1016/j.artint.2014.06.003
Keywords: del, machine learning, molecule generation, 3C-like protease, transfer learning
Citation: Xiong F, Xu H, Yu M, Chen X, Zhong Z, Guo Y, Chen M, Ou H, Wu J, Xie A, Xiong J, Xu L, Zhang L, Zhong Q, Huang L, Li Z, Zhang T, Jin F and He X (2022) 3CLpro inhibitors: DEL-based molecular generation. Front. Pharmacol. 13:1085665. doi: 10.3389/fphar.2022.1085665
Received: 31 October 2022; Accepted: 23 November 2022;
Published: 07 December 2022.
Edited by:
Dan Gao, Tsinghua University, ChinaReviewed by:
Feng Ni, Ningbo University, ChinaYizhou Li, Chongqing University, China
Xiaoni Ai, Peking University, China
Copyright © 2022 Xiong, Xu, Yu, Chen, Zhong, Guo, Chen, Ou, Wu, Xie, Xiong, Xu, Zhang, Zhong, Huang, Li, Zhang, Jin and He. This is an open-access article distributed under the terms of the Creative Commons Attribution License (CC BY). The use, distribution or reproduction in other forums is permitted, provided the original author(s) and the copyright owner(s) are credited and that the original publication in this journal is cited, in accordance with accepted academic practice. No use, distribution or reproduction is permitted which does not comply with these terms.
*Correspondence: Feng Xiong, fxiong@innosmd.com; Feng Jin, jinfeng@newdel.com.cn; Xun He, xhe@innosmd.com
†These authors have contributed equally to this work