- 1Carbon Logic Biotech (HK) Limited, Hongkong, China
- 2StateKey Laboratory of Oncology in South China, Department of Colorectal Surgery, Sun Yat-sen University Cancer Center, Collaborative Innovation Center for Cancer Medicine, Guangzhou, China
Background: The MSI/MSS status does not fully explain cancer immunotherapy response in colorectal cancer. Thus, we developed a colorectal cancer-specific method that predicts cancer immunotherapy response.
Methods: We used gene expression data of 454 samples (MSI = 131, MSI-L = 23, MSS = 284, and Unknown = 16) and developed a TMEPRE method that models signatures of CD8+ T-cell infiltration and CD8+ T-cell exhaustion states in the tumor microenvironment of colorectal cancer. TMEPRE model was validated on three RNAseq datasets of melanoma patients who received pembrolizumab or nivolumab and one RNAseq dataset of purified CD8+ T cells in different exhaustion states.
Results: TMEPRE showed predictive power in three datasets of anti-PD1-treated patients (p = 0.056, 0.115, 0.003). CD8+ T-cell exhaustion component of TMEPRE model correlates with anti-PD1 responding progenitor exhausted CD8+ T cells in both tumor and viral infection (p = 0.048, 0.001). The global pattern of TMEPRE on 454 colorectal cancer samples indicated that 10.6% of MSS patients and 67.2% of MSI patients show biological characteristics that can potentially benefit from anti-PD1 treatment. Within MSI nonresponders, approximately 50% showed insufficient tumor-infiltrating CD8+ T cells and 50% showed terminal exhaustion of CD8+ T cells. These terminally exhausted CD8+ T cells coexisted with signatures of myeloid-derived suppressor cells in colorectal cancer.
Conclusion: TMEPRE is a colorectal cancer-specific method. It captures characteristics of CD8+ T-cell infiltration and CD8+ T-cell exhaustion state and predicts cancer immunotherapy response. A subset of MSS patients could potentially benefit from anti-PD1 treatment. Anti-PD1 resistance MSI patients with insufficient infiltration of CD8+ T cells or terminal exhaustion of CD8+ T cells need different treatment strategies.
Introduction
Immune checkpoint inhibitors produce durable responses in some microsatellite instable (MSI) colorectal cancer patients. However, still, approximately 60% of MSI colorectal cancer patients do not respond to single immune checkpoint inhibitor treatment such as anti-PD1, and approximately 40% of MSI colorectal patients do not respond to combinations of immune checkpoint inhibitor treatment (Oliveira et al., 2019). The mechanism of resistance is unclear. In colorectal cancer, MSI/MSS status is widely used as an indication of whether a patient should receive immunotherapy. Therefore, most studies on colorectal cancer were focused on the comparison between MSI tumors and MSS tumors. Although these studies provide insights into the difference between these two colorectal cancer subtypes, they do not explain why resistance to immune checkpoint inhibitor treatment occurs within MSI colorectal tumors. In addition to MSI/MSS status, other biomarkers such as TMB, PDL1, POLE/POLD1 mutation, or MSI-like gene signature are also used in colorectal cancer (Tian et al., 2012; Havel et al., 2019). Essentially, PDL1 provides a direct indication of whether a tumor sample of a colorectal cancer patient has high CD8+ T-cell infiltration, while MSI/MSS status, TMB, POLE/POLD1 mutation, and MSI-like gene signature characterize the likelihood of a tumor sample generating high neoantigen level, thus indirectly indicating whether a tumor sample of a colorectal cancer patient could potentially have high CD8+ T-cell infiltration. However, it is already evident from the studies of anti-PD1 response in lung cancer and melanoma that the number of tumor-infiltrating CD8+ T cells is not the only requirement of response to anti-PD1 treatment; the characteristics of exhaustion state of tumor-infiltrating CD8+ T cells is also required (Thommen et al., 2015; Li et al., 2019; Sade-Feldman et al., 2019). Therefore, regardless of how technically robust biomarkers such as MSI/MSS status, TMB, PDL1, POLE/POLD1 mutation, and MSI-like gene signature, these biomarkers will only characterize the quantity of tumor-infiltrating CD8+ T cells. However, the quantity of tumor-infiltrating CD8+ T cells alone will not fully explain anti-PD1 resistance in colorectal cancer. There is a lack of prediction method of anti-PD1 response in colorectal cancer.
It is known that a tumor at least has two well documented immune escape mechanisms to become resistant to anti-PD1 treatment: lack of CD8+ T-cell infiltration and CD8+ T-cell dysfunction (Sharma et al., 2017; Sade-Feldman et al., 2019; Yost et al., 2019; Wu et al., 2020). Therefore, a colorectal tumor should meet at least two characteristics to become a responder to anti-PD1 treatment an anti-PD1 treatment responding tumors should have CD8+ T-cell infiltration and at least a subset of tumor-infiltrating CD8+ T cells display properties that can respond to anti-PD1. In this report, we develop a TMEPRE method that dissects the gene expression patterns of these two characteristics from the tumor microenvironment in colorectal cancer and predict anti-PD1 response.
Materials and Methods
Data Used for the Development of the TMEPRE Model
Publicly available gene expression data with known MSI/MSS status of four colorectal cancer datasets [GSE13294 (Jorissen et al., 2008), GSE26682 (Vilar et al., 2011), GSE18088 (Gröne et al., 2011), and GSE39084 (Kirzin et al., 2014)] were downloaded from GEO database. All four datasets are from the same Affymetrix Human Genome U133 Plus 2.0 Array platform, and normalization was performed using the frozen RMA (fRMA) method in the frma package (McCall et al., 2010). The batch effects of samples in four datasets were removed using ComBat (Johnson et al., 2007). In total, gene expression data of 454 samples were collected (MSI = 131, MSI-L = 23, MSS = 284, Unknown = 16). The public or the patients were not involved in the design, conduct, reporting, or dissemination plans of our research.
Design of the TMEPRE Model
The score function of the TMEPRE model is comprised of two components:
1)
The relative range coverage
2)
Data Used for Testing Predictive Values of the Anti-PD1 Response of the TMEPRE Model
To test the predictive value of the anti-PD1 response, three RNAseq datasets were used. The first dataset includes normalized RNAseq data and clinical data of pretreatment samples of melanoma patients who received pembrolizumab or nivolumab. Patients in this cohort who received MAPK inhibitor were removed (n = 16, GSE78220) (Hugo et al., 2016). The second dataset includes normalized RNAseq data and clinical data of samples of melanoma patients who received nivolumab. Samples at the early treatment time point before cycle 1 day 29 and samples at the pretreatment time point before cycle 1 day 0 were analyzed separately. Patients who received a priori ipilimumab treatment or with incomplete overall survival data were removed (n = 21, GSE91061) (Riaz et al., 2017). The platform of these three anti-PD1-treated patients’ datasets is different from the platform of the datasets used in the development of TMEPRE and the cutoff values can not be directly used. In three datasets of anti-PD1-treated patients, the median was therefore used as the default cutoff. TMEPRE comprises two components; the median was equally split as the cutoffs for each component. A colorectal tumor with either a low
To read the
Results
TMEPRE Model Predicts the Anti-PD1 Treatment Response
The TMEPRE model was developed using gene expression data of colorectal cancer patients and has two components:
To date, melanoma is widely used as the main prototypic cancer type of immune hot tumor to study cancer immunotherapy response. Thus, most of the available gene expression datasets of anti-PD1 response were performed using melanoma as the model system. Because TMEPRE is a method that mainly measures characteristics of the tumor microenvironment and different cancer types have shared tumor microenvironment characteristics, in our study, we also used anti-PD1-treated melanoma datasets as a model system to test predictive values of TMEPRE. TMEPRE was validated on three datasets of melanoma patients who received anti-PD1 treatment. In the first dataset, the survival analysis of the TMEPRE prediction model resulted in a significant hazard ratio (n = 16, pretreatment samples, GSE78220, HR = 4.59, p-value = 0.056, Figure 1A). In the second dataset, although the p-value of the survival analysis of the TMEPRE prediction model is large (n = 21, sampling before cycle 1 day 0, GSE91061, HR = 2.12, p-value = 0.115, Figure 1B), the separation of survival between the TMEPRE-predicted responder group and the TMEPRE-predicted nonresponder group is still clearly observed. In the third dataset, the survival analysis of the TMEPRE prediction model resulted in a significant hazard ratio (n = 21, sampling at an early treatment time point before cycle 1 day 29, GSE91061, HR = 5.04, p-value = 0.003, Figure 1C). The sample sizes of anti-PD1-treated samples in the current publicly available databases are small, and this small sample size artificially increased the p-values of each individual log-rank test (Ioannidis, 2019; Thiese et al., 2016). Still, the trend of separation of the responder group and the nonresponder group is clear in all three validation sets (Figures 1A–C). When three validation sets are combined together, the Z-transform combined probability test showed a significant p-value (p-value = 0.0007) (Whitlock, 2005). Taken together, the survival analysis indicated clinical significance, and the TMEPRE model showed predictive values for anti-PD1 treatment response.

FIGURE 1. TMEPRE was validated on three datasets of melanoma patients who received anti-PD1 treatment. (1A) n = 16, pretreatment samples, patients were treated with pembrolizumab or nivolumab, HR = 4.59, p-value = 0.056; (1B) n = 21, sampling before cycle 1 day 0, patients were treated with nivolumab, HR = 2.12, p-value = 0.115; (1C) n = 21, sampling at an early treatment time point before cycle 1 day 29, patients were treated with nivolumab, HR = 5.04, p-value = 0.003. Separation of overall survival between TMEPRE-predicted responder group and TMEPRE-predicted nonresponder group is clear in all three validations.
The Underlying Biology of the TMEPRE Model Measures Amounts of Tumor-Infiltrating CD8+ T Cells and Characteristics of Tumor-Infiltrating Terminally Exhausted CD8+ T Cells
In the dataset of all 454 samples, the counts of tumor-infiltrating cytotoxic lymphocytes were read out using MCP-counter and TIDE cytotoxic T lymphocytes count (Becht et al., 2016; Jiang et al., 2018). The first component of the TMEPRE model,
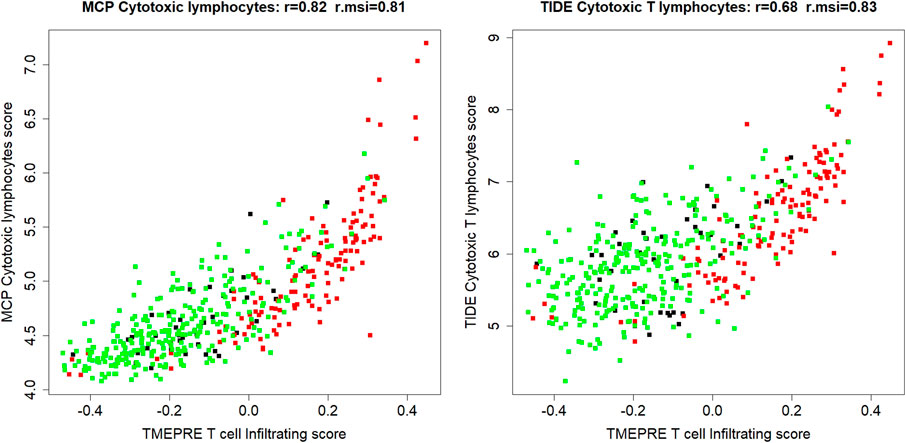
FIGURE 2. In 454 colorectal cancer samples (MSI = 131, MSI-L = 23, MSS = 284, Unknown = 16), the first component of the TMEPRE model,
The second component of the TMEPRE model,
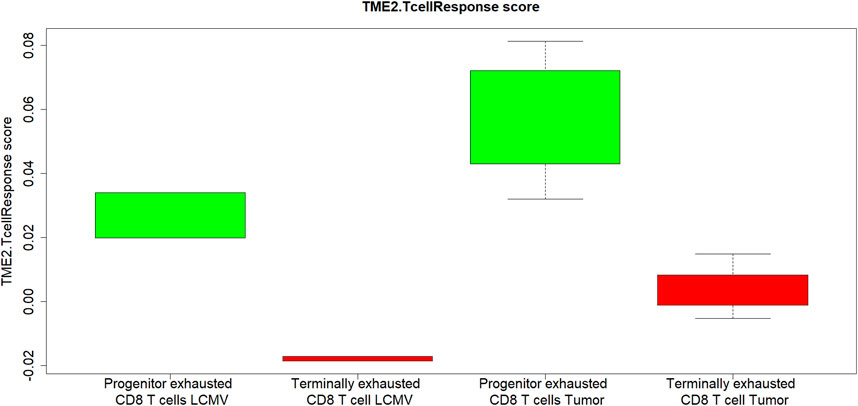
FIGURE 3. In both tumor and chronic viral infection, CD8+ T cells components of
Global Pattern of the TMEPRE Model in MSI and MSS Colorectal Tumors
The TMEPRE model was read out in 454 colorectal samples (MSI = 131, MSI-L = 23, MSS = 284, Unknown = 16). A splitted heatmap was plotted (Gu et al., 2016). Tumors displaying a pattern of sufficient CD8+ T-cell infiltration but no pattern of CD8+ T-cell terminal exhaustion are considered as potential responders to anti-PD1 therapy (Figure 4).
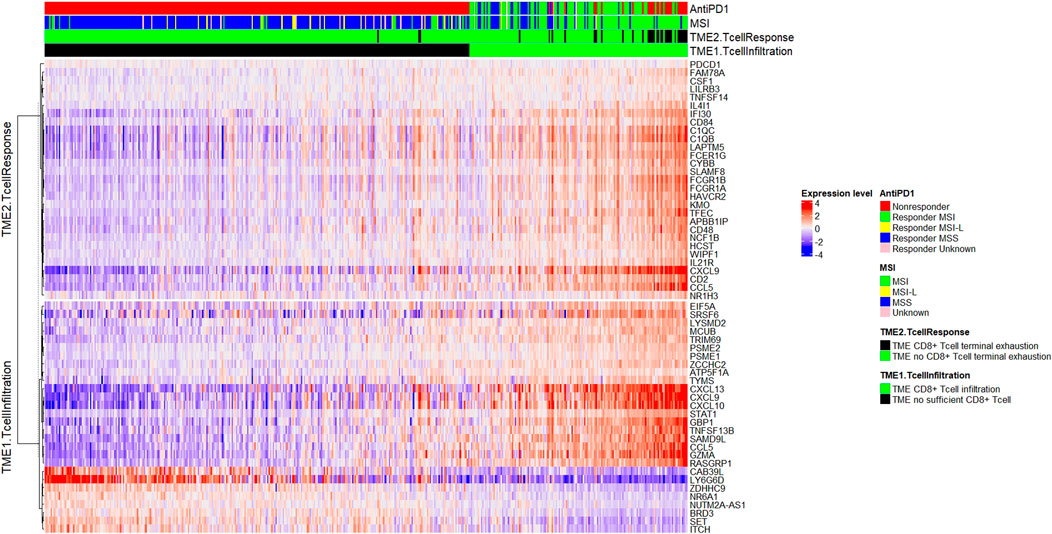
FIGURE 4. Plot of TMEPRE over 454 colorectal cancer samples (MSI = 131, MSI-L = 23, MSS = 284, Unknown = 16). Samples were ranked according to scores of the TMEPRE model. Genes in
Within 284 MSS tumor samples, 10.6% (n = 30) are classified as responders and 89.4% (n = 254) as nonresponders. This predicted percentage of responders, 10.6% by TMEPRE, is consistent with the reported disease control rate, 11%, in pembrolizumab-treated metastatic MSS colorectal cancers (Le et al., 2015). Among MSS nonresponders, 86.6% (n = 246) showed insufficient tumor-infiltrating CD8+ T cells and 2.8% (n = 8) showed sufficient tumor-infiltrating CD8+ T cells; however, those CD8+ T cells display patterns of terminally exhausted CD8+ T cells. As expected, the anti-PD1 resistance mechanism of the majority of MSS tumors is an insufficient amount of tumor-infiltrating CD8+ T cells.
Within 131 MSI tumor samples, 67.2% (n = 88) are classified as responders and 32.8% (n = 43) as nonresponders. This predicted percentage of responders, 67.2% by TMEPRE, is between the range of the reported immune-related objective response rate, 40%, and the reported disease control rate, 78%, in pembrolizumab-treated metastatic MSI colorectal cancers (Le et al., 2015). Among the MSI nonresponders, 16.0% (n = 21) showed insufficient tumor-infiltrating CD8+ T cells and 16.8% (n = 22) showed sufficient tumor-infiltrating CD8+ T cells; however, those CD8+ T cells display patterns of terminally exhausted CD8+ T cells. Therefore, approximately 50% of MSI nonresponders are caused by terminal exhaustion of CD8+ T cells in the tumor microenvironment, and the rest 50% of MSI nonresponders are caused by an insufficient amount of tumor-infiltrating CD8+ T cells. Treatments of MSI nonresponders with insufficient infiltration of CD8+ T cells and MSI nonresponders with terminal exhaustion of CD8+ T cells need to be designed separately.
The TMEPRE model identified 10.6% of MSS and 67.2% of MSI colorectal cancer patients whose tumors show biological characteristics that can potentially benefit from anti-PD1 treatment. These predicted percentages of responders in MSS tumors and MSI tumors are consistent with the reported benefits of immune-related disease control rate at 20 weeks of a cohort of colorectal cancer patients treated with pembrolizumab (Le et al., 2015).
Discussion
Drug resistance tumors in colorectal cancer often consist of heterogeneous subgroups of populations. A drug response prediction method needs first to identify different drug resistance patterns of subgroups and then reinforce the patterns later (Tian et al., 2013; Tian et al., 2020). Conceptually, a drug response prediction method must never consider the drug resistance tumors as a homogenous group. Current biomarkers for anti-PD1 in colorectal cancer, MSI/MSS, TMB, PDL1, and POLE/POLD1 mutation, share the same notion that anti-PD1 resistance is dominantly caused by one homogenous factor of an insufficient amount of CD8+ T-cell infiltration. The quantity of tumor-infiltrating CD8+ T cells needs to be supplemented by characteristics of tumor-infiltrating CD8+ T cells to predict anti-PD1 response. In this report, we developed the computational method TMEPRE for colorectal cancer patients, which measures two factors of the tumor microenvironment that contribute to anti-PD1 resistance: CD8+ T-cell infiltration (
Another example of a prediction method of anti-PD1 response using tumor microenvironment of CD8+ T-cell exclusion and CD8+ T-cell exhaustion is TIDE (Jiang et al., 2018). The TIDE method also has good validation performance in anti-PD1-treated melanoma data. It is difficult to directly compare TIDE and TMEPRE as these two methods are optimized for different tumor types. TIDE method was trained using survival data of melanoma and was specifically designed for five cancer types: melanoma, neuroblastoma, triple-negative breast cancer, endometrial cancer, and acute myeloid leukemia. On the contrary, the TMEPRE method is designed for colorectal cancer. Partially because the methods are optimized for different cancer types, the overlap between genes used in the TMEPRE model and genes used in the TIDE model is small (IL21R, GZMA). We expect that TMEPRE will be a more specific reflection of the tumor microenvironment of colorectal cancer.
The first component of TMEPRE,
By only assessing the MSI/MSS status, it is not recommended that MSS colorectal cancer patients be treated with anti-PD1. However, data from clinical trials showed that the disease control rate in pembrolizumab-treated metastatic MSS colorectal cancers was 11%, and further, in a more recent clinical trial of neoadjuvant setting, the pathological response rate of ipilimumab + nivolumab–treated early-stage MSS colorectal cancers is 27% (Le et al., 2015; Chalabi et al., 2020). These results indicated that responders to anti-PD1 treatment exist within the MSS colorectal cancer population. In our analysis, approximately 10.6% of MSS tumor samples showed both high
To conclude, we develop a colorectal cancer-specific method, TMEPRE, that predicts cancer immunotherapy response. The global patterns of TMEPRE in colorectal cancer patients explained the mechanism underlying the response of anti-PD1 in MSS patients and the resistance of anti-PD1 in MSI patients. A subset of MSS patients could potentially benefit from anti-PD1 treatment. Anti-PD1-resistant MSI patients could result from tumor microenvironment of insufficient infiltration of CD8+ T-cell or tumor microenvironment of terminal exhaustion of CD8+ T cells, and treatment strategies need to be different. TMEPRE will aid personalized medicine options of cancer immunotherapy for colorectal cancer patients.
Data Availability Statement
The datasets presented in this study can be found in online repositories. The names of the repository/repositories and accession number(s) can be found in the article/Supplementary Material.
Ethics Statement
The study uses publicly available dataset. Ethical review and approval were not required for the study on human participants in accordance with the local legislation and institutional requirements. Written informed consent for participation was not required for this study in accordance with the national legislation and the institutional requirements.
Author Contributions
ST was responsible for the conceptualization, methodology, and formal analysis and wrote the original draft; ST and GC investigated and interpreted the results; FW, RZ, and GC acquired the resources; ST, FW, RZ, and GC reviewed and edited the manuscript; ST and GC were responsible for project administration.
Conflict of Interest
ST has stocks and/or stock options in Carbon Logic Biotech. ST is a named inventor of a patent application.
The remaining authors declare that the research was conducted in the absence of any commercial or financial relationships that could be construed as a potential conflict of interest.
Publisher’s Note
All claims expressed in this article are solely those of the authors and do not necessarily represent those of their affiliated organizations, or those of the publisher, the editors, and the reviewers. Any product that may be evaluated in this article, or claim that may be made by its manufacturer, is not guaranteed or endorsed by the publisher.
Acknowledgments
This manuscript has been released as a pre-print at: https://www.researchsquare.com/article/rs-89397/v1.
Supplementary Material
The Supplementary Material for this article can be found online at: https://www.frontiersin.org/articles/10.3389/fphar.2021.715721/full#supplementary-material
References
Bagger, F. O., Kinalis, S., and Rapin, N. (2019). BloodSpot: a Database of Healthy and Malignant Haematopoiesis Updated with Purified and Single Cell mRNA Sequencing Profiles. Nucleic Acids Res. 47, D881–D885. doi:10.1093/nar/gky1076
Becht, E., Giraldo, N. A., Lacroix, L., Buttard, B., Elarouci, N., Petitprez, F., et al. (2016). Estimating the Population Abundance of Tissue-Infiltrating Immune and Stromal Cell Populations Using Gene Expression. Genome Biol. 17, 218. doi:10.1186/s13059-016-1070-5
Bournazos, S., Wang, T. T., and Ravetch, J. V. (2016). The Role and Function of Fcγ Receptors on Myeloid Cells. Microbiol. Spectr. 4, 4. doi:10.1128/microbiolspec.MCHD-0045-2016
Busselaar, J., Tian, S., van Eenennaam, H., and Borst, J. (2020). Helpless Priming Sends CD8+ T Cells on the Road to Exhaustion. Front. Immunol. 11, 11. doi:10.3389/fimmu.2020.592569
Chalabi, M., Fanchi, L. F., Dijkstra, K. K., Van den Berg, J. G., Aalbers, A. G., Sikorska, K., et al. (2020). Neoadjuvant Immunotherapy Leads to Pathological Responses in MMR-Proficient and MMR-Deficient Early-Stage colon Cancers. Nat. Med. 26, 566–576. doi:10.1038/s41591-020-0805-8
Duan, Q., Zhang, H., Zheng, J., and Zhang, L. (2020). Turning Cold into Hot: Firing up the Tumor Microenvironment. Trends Cancer. 6, 605–618. doi:10.1016/j.trecan.2020.02.022
Giorgini, F., Huang, S.-Y., Sathyasaikumar, K. V., Notarangelo, F. M., Thomas, M. A. R., Tararina, M., et al. (2013). Targeted Deletion of Kynurenine 3-Monooxygenase in Mice. J. Biol. Chem. 288, 36554–36566. doi:10.1074/jbc.M113.503813
Gröne, J., Lenze, D., Jurinovic, V., Hummel, M., Seidel, H., Leder, G., et al. (2011). Molecular Profiles and Clinical Outcome of Stage UICC II Colon Cancer Patients. Int. J. Colorectal Dis. 26, 847–858. doi:10.1007/s00384-011-1176-x
Gu, Z., Eils, R., and Schlesner, M. (2016). Complex Heatmaps Reveal Patterns and Correlations in Multidimensional Genomic Data. Bioinformatics. 32, 2847–2849. doi:10.1093/bioinformatics/btw313
Haibe-Kains, B., Desmedt, C., Sotiriou, C., and Bontempi, G. (2008). A Comparative Study of Survival Models for Breast Cancer Prognostication Based on Microarray Data: Does a Single Gene Beat Them All? Bioinformatics. 24, 2200–2208. doi:10.1093/bioinformatics/btn374
Havel, J. J., Chowell, D., and Chan, T. A. (2019). The Evolving Landscape of Biomarkers for Checkpoint Inhibitor Immunotherapy. Nat. Rev. Cancer. 19, 133–150. doi:10.1038/s41568-019-0116-x
Hugo, W., Zaretsky, J. M., Sun, L., Song, C., Moreno, B. H., Hu-Lieskovan, S., et al. (2016). Genomic and Transcriptomic Features of Response to Anti-PD-1 Therapy in Metastatic Melanoma. Cell. 165, 35–44. doi:10.1016/j.cell.2016.02.065
Ioannidis, J. P. A. (2019). The Importance of Predefined Rules and Prespecified Statistical Analyses. JAMA. 321, 2067–2068. doi:10.1001/jama.2019.4582
Jiang, P., Gu, S., Pan, D., Fu, J., Sahu, A., Hu, X., et al. (2018). Signatures of T Cell Dysfunction and Exclusion Predict Cancer Immunotherapy Response. Nat. Med. 24, 1550–1558. doi:10.1038/s41591-018-0136-1
Johnson, W. E., Li, C., and Rabinovic, A. (2007). Adjusting Batch Effects in Microarray Expression Data Using Empirical Bayes Methods. Biostat Oxf Engl. 8, 118–127. doi:10.1093/biostatistics/kxj037
Jorissen, R. N., Lipton, L., Gibbs, P., Chapman, M., Desai, J., Jones, I. T., et al. (2008). DNA Copy-Number Alterations Underlie Gene Expression Differences between Microsatellite Stable and Unstable Colorectal Cancers. Clin. Cancer Res. 14, 8061–8069. doi:10.1158/1078-0432.ccr-08-1431
Kirzin, S., Marisa, L., Guimbaud, R., De Reynies, A., Legrain, M., Laurent-Puig, P., et al. (2014). Sporadic Early-Onset Colorectal Cancer Is a Specific Sub-type of Cancer: a Morphological, Molecular and Genetics Study. PLoS One 9, e103159. doi:10.1371/journal.pone.0103159
Kuang, Z., Li, L., Zhang, P., Chen, B., Wu, M., Ni, H., et al. (2020). A Novel Antibody Targeting TIM-3 Resulting in Receptor Internalization for Cancer Immunotherapy. Antib Ther. 3, 227–236. doi:10.1093/abt/tbaa022
Le, D. T., Uram, J. N., Wang, H., Bartlett, B. R., Kemberling, H., Eyring, A. D., et al. (2015). PD-1 Blockade in Tumors With Mismatch-Repair Deficiency. N. Engl. J. Med. 372, 2509–2520. doi:10.1056/NEJMoa1500596
Li, H., van der Leun, A. M., Yofe, I., Lubling, Y., Gelbard-Solodkin, D., van Akkooi, A. C. J., et al. (2019). Dysfunctional CD8 T Cells Form a Proliferative, Dynamically Regulated Compartment within Human Melanoma. Cell. 176, 775–789. doi:10.1016/j.cell.2018.11.043
Lind, H., Gameiro, S. R., Jochems, C., Donahue, R. N., Strauss, J., Gulley, J. L., et al. (2020). Dual Targeting of TGF-β and PD-L1 via a Bifunctional Anti-PD-l1/tgf-βrii Agent: Status of Preclinical and Clinical Advances. J. Immunother. Cancer. 8, e000433. doi:10.1136/jitc-2019-000433
McCall, M. N., Bolstad, B. M., and Irizarry, R. A. (2010). Frozen Robust Multiarray Analysis (fRMA). Biostatistics. 11, 242–253. doi:10.1093/biostatistics/kxp059
Miller, B. C., Sen, D. R., Al Abosy, R., Bi, K., Virkud, Y. V., LaFleur, M. W., et al. (2019). Subsets of Exhausted CD8+ T Cells Differentially Mediate Tumor Control and Respond to Checkpoint Blockade. Nat. Immunol. 20, 326–336. doi:10.1038/s41590-019-0312-6
Oliveira, A. F., Bretes, L., and Furtado, I. (2019). Review of PD-1/pd-L1 Inhibitors in Metastatic dMMR/MSI-H Colorectal Cancer. Front. Oncol. 9, 396. doi:10.3389/fonc.2019.00396
Riaz, N., Havel, J. J., Makarov, V., Desrichard, A., Urba, W. J., Sims, J. S., et al. (2017). Tumor and Microenvironment Evolution During Immunotherapy With Nivolumab. Cell. 171, 934–949. doi:10.1016/j.cell.2017.09.028
Ryan, A. F., Nasamran, C. A., Pak, K., Draf, C., Fisch, K. M., Webster, N., et al. (2020). Single-Cell Transcriptomes Reveal a Complex Cellular Landscape in the Middle Ear and Differential Capacities for Acute Response to Infection. Front. Genet. 11, 11. doi:10.3389/fgene.2020.00358
Sade-Feldman, M., Yizhak, K., Bjorgaard, S. L., Ray, J. P., de Boer, C. G., Jenkins, R. W., et al. (2019). Defining T Cell States Associated With Response to Checkpoint Immunotherapy in Melanoma. Cell. 176, 404. doi:10.1016/j.cell.2018.12.034
Sharma, P., Hu-Lieskovan, S., Wargo, J. A., and Ribas, A. (2017). Primary, Adaptive, and Acquired Resistance to Cancer Immunotherapy. Cell .168, 707–723. doi:10.1016/j.cell.2017.01.017
Therneau, T. M., and Grambsch, P. M. (2000). Modeling Survival Data: Extending the Cox Model. New York: Springer-Verlag.
Thiese, M. S., Ronna, B., and Ott, U. (2016). P Value Interpretations and Considerations. J. Thorac. Dis. 8, E928–E931. doi:10.21037/jtd.2016.08.16
Thommen, D. S., Schreiner, J., Müller, P., Herzig, P., Roller, A., Belousov, A., et al. (2015). Progression of Lung Cancer Is Associated With Increased Dysfunction of T Cells Defined by Coexpression of Multiple Inhibitory Receptors. Cancer Immunol. Res. 3, 1344–1355. doi:10.1158/2326-6066.CIR-15-0097
Tian, S., Roepman, P., Popovici, V., Michaut, M., Majewski, I., Salazar, R., et al. (2012). A Robust Genomic Signature for the Detection of Colorectal Cancer Patients with Microsatellite Instability Phenotype and High Mutation Frequency. J. Pathol. 4, 586–595. doi:10.1002/path.4092
Tian, S., Simon, I., Moreno, V., Roepman, P., Tabernero, J., Snel, M., et al. (2013). A Combined Oncogenic Pathway Signature ofBRAF,KRASandPI3KCAmutation Improves Colorectal Cancer Classification and Cetuximab Treatment Prediction. Gut. 62, 540–549. doi:10.1136/gutjnl-2012-302423
Tian, S., Wang, F., Lu, S., and Chen, G. (2020). Identification of Two Subgroups of FOLFOX Resistance Patterns and Prediction of FOLFOX Response in Colorectal Cancer Patients. Cancer Invest. 39, 62–72. doi:10.1080/07357907.2020.1843662
Vilar, E., Bartnik, C. M., Stenzel, S. L., Raskin, L., Ahn, J., Moreno, V., et al. (2011). MRE11 Deficiency Increases Sensitivity to Poly(ADP-Ribose) Polymerase Inhibition in Microsatellite Unstable Colorectal Cancers. Cancer Res. 71, 2632–2642. doi:10.1158/0008-5472.CAN-10-1120
Whitlock, M. C. (2005). Combining Probability from Independent Tests: the Weighted Z-Method Is superior to Fisher's Approach. J. Evol. Biol. 18, 1368–1373. doi:10.1111/j.1420-9101.2005.00917.x
Wu, T. D., Madireddi, S., de Almeida, P. E., Banchereau, R., Chen, Y.-J. J., Chitre, A. S., et al. (2020). Peripheral T Cell Expansion Predicts Tumour Infiltration and Clinical Response. Nature. 579, 274–278. doi:10.1038/s41586-020-2056-8
Yost, K. E., Satpathy, A. T., Wells, D. K., Qi, Y., Wang, C., Kageyama, R., et al. (2019). Clonal Replacement of Tumor-specific T Cells Following PD-1 Blockade. Nat. Med. 25, 1251–1259. doi:10.1038/s41591-019-0522-3
Keywords: biomarker, prediction method, colorectal cancer, cancer immunotherapy response, anti-PD1
Citation: Tian S, Wang F, Zhang R and Chen G (2021) Global Pattern of CD8+ T-Cell Infiltration and Exhaustion in Colorectal Cancer Predicts Cancer Immunotherapy Response. Front. Pharmacol. 12:715721. doi: 10.3389/fphar.2021.715721
Received: 27 May 2021; Accepted: 22 July 2021;
Published: 10 September 2021.
Edited by:
Marc Diederich, Seoul National University, South KoreaReviewed by:
Anne Jarry, Institut National de la Santé et de la Recherche Médicale (INSERM), FranceVeronica Aran, Instituto Estadual do Cérebro Paulo Niemeyer (IECPN), Brazil
Copyright © 2021 Tian, Wang, Zhang and Chen. This is an open-access article distributed under the terms of the Creative Commons Attribution License (CC BY). The use, distribution or reproduction in other forums is permitted, provided the original author(s) and the copyright owner(s) are credited and that the original publication in this journal is cited, in accordance with accepted academic practice. No use, distribution or reproduction is permitted which does not comply with these terms.
*Correspondence: Sun Tian, c3VudGlhbi5nd0BnbWFpbC5jb20=; Gong Chen, Y2hlbmdvbmdAc3lzdWNjLm9yZy5jbg==