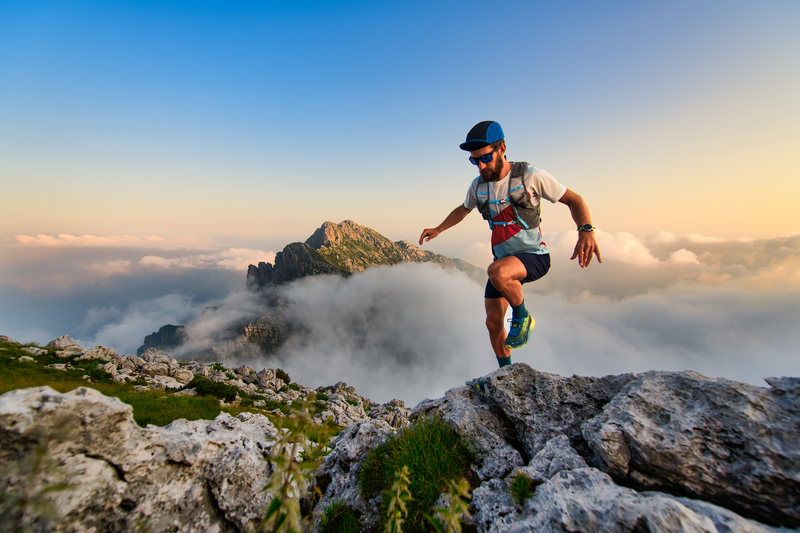
95% of researchers rate our articles as excellent or good
Learn more about the work of our research integrity team to safeguard the quality of each article we publish.
Find out more
ORIGINAL RESEARCH article
Front. Pharmacol. , 06 October 2021
Sec. Inflammation Pharmacology
Volume 12 - 2021 | https://doi.org/10.3389/fphar.2021.700896
This article is part of the Research Topic Medicinal Plants as a Source of Novel Autoimmune-Modulating and Anti-Inflammatory Drug Products View all 14 articles
Purpose: Prunella vulgaris (PV), a traditional Chinese medicine, has been used to treat patients with thyroid disease for centuries in China. The purpose of the present study was to investigate its bioactive ingredients and mechanisms against Hashimoto’s thyroiditis (HT) using network pharmacology and molecular docking technology to provide some basis for experimental research.
Methods: Ingredients of the PV formula were retrieved from the Traditional Chinese Medicine Systems Pharmacology (TCMSP) database. Additionally, HT-related genes were retrieved from the UniProt and GeneCards databases. Cytoscape constructed networks for visualization. A protein–protein interaction (PPI) network analysis was constructed, and a PPI network was built using the Search Tool for the Retrieval of Interacting Genes (STRING) database. These key targets of PV were enriched and analyzed by molecular docking verification, Gene Ontology (GO), and Kyoto Encyclopedia of Genes and Genomes (KEGG) enrichment.
Results: The compound–target network included 11 compounds and 66 target genes. Key targets contained Jun proto-oncogene (JUN), hsp90aa1.1 (AKI), mitogen-activated protein kinase 1 (MAPK1), and tumor protein p53 (TP53). The main pathways included the AGE-RAGE signaling pathway, the TNF signaling pathway, the PI3K–Akt signaling pathway, and the mitogen-activated protein kinase signaling pathway. The molecular docking results revealed that the main compound identified in the Prunella vulgaris was luteolin, followed by kaempferol, which had a strong affinity for HT.
Conclusion: Molecular docking studies indicated that luteolin and kaempferol were bioactive compounds of PV and might play an essential role in treating HT by regulating multiple signaling pathways.
Prunella vulgaris (PV) is a perennial herbaceous plant in the genus Prunella1. It is a Chinese medicine widely used to treat inflammation, eye pain, and headaches (Wang et al., 2019; Chen et al., 2020). The anti-inflammatory effects of PV have been recognized during the long-term practice of traditional Chinese medicine (TCM) (hui ZRGwsbydwy, 2000). Currently, PV is combined with Western medicines, such as levothyroxine, indomethacin, or prednisone, in liquid or capsules and has been used to treat Hashimoto’s thyroiditis patients. It has been shown that PV significantly reduces the antibody titers of thyroid peroxidase antibody (TPO-Ab) and thyroglobulin antibody (TG-Ab) (Yang et al., 2007; Zhang, 2014). However, the potential underlying mechanisms by which PV might exert its anti-inflammatory effects are poorly understood.
Hashimoto’s thyroiditis (HT) is a genetic autoimmune disorder characterized by the destruction of thyroid cells by cell- and antibody-mediated immune responses (Hu et al., 2019). In developed countries, HT is the most common cause of hypothyroidism. The estimated incidence of HT is 3.5 per 1,000 per year in women and 0.8 per 1,000 per year in men (Ala et al., 2015). Effective treatment options for HT are limited. The main method and purpose of HT treatment is the control of hypothyroidism and consists of oral administration of a synthetic hormone, levothyroxine 4 (L-T4) (Wiersinga and Wilmar, 2001).
Additionally, the association between vitamin D deficiency, HT pathogenesis, and thyroid hypofunction has been demonstrated in several studies (Liontiris and Mazokopakis, 2017; Roehlen et al., 2018; Chao et al., 2020). Therefore, due to the low cost and minimal side effects of vitamin D, monitoring and supplementation in patients with HT may be recommended (Liontiris and Mazokopakis, 2017). Surgical therapy can be recommended for patients with HT concurrent with nodules or malignancies (Caturegli et al., 2013). However, HT patients commonly have a higher prevalence rate of postoperative complications than thyroid disorders (Gan et al., 2021).
Network pharmacology, combined with pharmacology and pharmacodynamics, is a novel research field that clarifies numerous compounds’ synergistic effects and underlying mechanisms by analyzing various networks of complex and multilevel interactions (Cao et al., 2018). The study explored the potential pharmacodynamic material basis and molecular mechanism of PV against HT using network pharmacology and molecular docking technology and predicted their potential targets and signaling pathways.
The active constituents of PV were obtained from the Traditional Chinese Medicine Systems Pharmacology Database, the Analysis Platform (TCMSP, http://tcmspw.com/) database, and subsequent network pharmacology (Tan et al., 2017). The TCMIP database of Chinese herbal medicines is based on the Chinese Pharmacopoeia (2015 edition), which contains 500 kinds of Chinese herbal medicines and 30,069 ingredients (Ru et al., 2014). The names and aliases of herbs and ingredients were used as keywords. Then, two parameters of ADME (the absorption, distribution, metabolism, and excretion screening method) drug-likeness (DL) and oral bioavailability (OB) were used to predict the bioactive compounds. In drug discovery and development processes, absorption, distribution, metabolism, and excretion (ADME) evaluations are necessary to predict biologically active compounds (Su et al., 2007). In this study, OB > = 30% and DL quality > = 0.18 were selected as criteria for screening the active compounds identified within the TCMSP database (Ning et al., 2017).
The potential targets for the components of PV were retrieved from both TCMSP databases (http://lsp.nwsuaf.edu.cn/tcmsp.php), including 6,511 drug molecules and nearly 4,000 targets as well as the interaction between them (Ru et al., 2014). The UniProt Knowledgebase (UniProtKB) is a protein database partially curated by experts and contains 54, 247, 468 sequence entries (Zhang et al., 2020). Gene information, including the gene name and the gene ID, was confirmed by the UniProt database (https://www.uniprot.org).
The GeneCards database (https://www.genecards.org/) and selection according to the criterion of RiskScore >1, the Therapeutic Target Database (TTD, https://db.idrblab.org/ttd/), OMIM (https://omim.org/), PharmGkb (https://www.pharmgkb.org/), and DrugBank (https://www.drugbank.ca/) were used to collect information on HT related to target genes. The association of PV with HT was then gathered as the core targets of PV for HT.
In this research, the network of component–target interactions was established, and the interaction between active compounds and their core target proteins was ascertained by Cytoscape 3.7.2 (http://www.cytoscape.org/) (Wang et al., 2011). Moreover, it was visualized by Cytoscape software, an open-source platform for visualizing complex networks (Shannonand et al., 2003). In the network, nodes represent the herbal medicines, active phytochemical compounds, targets, or signaling pathways, while edges represent the interactions between the nodes (Barabási and Oltvai, 2004). The top two compounds were chosen as the ligand for molecular docking. The degree of a node represents the number of connections (edges) that this node has with the rest of the network (Tang et al., 2020). The larger the quantitative value, the more important the node in the network, and the more likely the component is the PV of the key ingredient.
The candidate targets of PV for HT treatment were imported into the STRING database (https://string-db.org/) (Consortium UP, 2010) to construct a PPI network. The network analysis plug-in in Cytoscape software was used to analyze network topological features to screen the hub nodes in the PPI network (Saito et al., 2012). Degree centrality denotes several direct connections of a node to all other nodes in the network. The value of degree was used as a reference for the importance of the core target by the CytoNCA, a plug-in of Cytoscape for network centrality analysis.
Gene Ontology (GO) functional annotation and Kyoto Encyclopedia of Genes and Genomes (KEGG) pathway enrichment were performed in R using the ClusterProfiler package, and p. adjust (FDR) < 0.05 was considered statistically significant (Yu et al., 2012).
The three-dimensional (3D) molecular structures of hsp90aa1.1 (AKI) (PDB ID: P31749) (Wu et al., 2010) and mitogen-activated protein kinase 1 (MAPK1) (PDB ID: P28482) (Ward et al., 2017) were downloaded from the RCSB Protein Data Bank (http://www.rcsb.org/pdb). The protein structure was processed by AutoDock Tools (Morris et al., 2009) to remove water molecules and connect hydrogen atoms, and then Gasteiger charges were added to the ligands and the protein templates and saved as a PDBQT file. Two-dimensional (2D) structures of luteolin and kaempferol were downloaded from PubChem (available online: https://pubchem.ncbi.nlm.nih.gov/) as. sdf. Luteolin and kaempferol were vital active compounds, and AKI and MAPK1 were considered the major targets. Molecular docking of the ligands luteolin and kaempferol to the AKI and MAPK1 protein active sites was carried out using AutoDock Vina 1.1.2. Each docking calculation generated 20 structures, and the molecular docking output was prioritized based on the frequency of the possible ligand-binding site and a free energy score. Finally, the best possible conformations and visualized using Discovery Studio Visualizer 2.5 and PyMOL software (PyMol Molecular Graphics System, Version 1).
Of these, 923 were targets of the herbs comprising PV, and the Venn diagram of PV targets is shown in Figure 1. A Venn plot showing the intersections of herbs and HT-related genes is shown in Figure 2. The main active components of PV containing 11 compounds are shown in Table 1, and the structures are shown in Table 2. Key targets containing Jun proto-oncogene (JUN), AKI, MAPK1, and tumor protein p53 (TP53) are shown in Table 3 and Figure 3C. Moreover, the scores of four centralities were calculated by CytoNCA, and details are provided in Table 4.
The herb–compound–target network contained 77 nodes (11 compounds and 66 genes), as shown in Figure 3. The node size represents the importance of a node, and the bigger size indicates more importance. According to the degree of the compound, we finally chose the eleven more important compounds, including MOL000006 (luteolin), MOL000098 (quercetin), MOL000358 (beta-sitosterol), MOL000422 (kaempferol), MOL000449 (stigmasterol), MOL000737 (morin), MOL004355 (spinasterol), MOL004798 (delphinidin), MOL006767 (vulgaxanthin-I), MOL006772 (poriferasterol monoglucoside_qt), and MOL006774 (stigmast-7-enol), and the details are shown in Table 1 and Table 2.
A total of 66 disease-related targets, 11 PV-related targets, and 17 intersection targets were identified (Figures 3A,4). Our study showed that targets had strong relationships in the PPI network. There were 17 nodes in the PeLBD protein interaction network, which were the core targets of PV in the treatment of HT (Table 4). JUN, AKI, MAPK1, and TP53 (Aziz et al., 2018) were considered hub genes (Figure 3B).
To better understand the function of intersecting genes, we conducted an enrichment analysis of Gene Ontology (GO). The top 10 biological processes mainly an inflammatory response, a cell-to-cell reaction, and a metabolic process are ranked in Figures 5A, C. In the enrichment analysis of GO pathways, cellular components mainly contained membrane rafts, membrane microdomains, and membrane regions. Meanwhile, the GO terms enriched for a molecular function were mainly cytokine receptor binding, cytokine activity, and receptor-ligand activity. Furthermore, the KEGG pathway enrichment analysis was also conducted. A total of 30 top-ranking pathways (Figures 5B, D) were identified (p < 0.05). The relative enrichment analysis showed the following pathways: the AGE-RAGE signaling pathway in diabetic complications, the TNF signaling pathway, the PI3K–Akt signaling pathway, pathways in cancer, the MAPK signaling pathway, and the cancer-associated pathways, as shown in Figures 5B and Figure 6.
FIGURE 5. GO and KEGG functional annotation pathway enrichments. The barplot (A, B) and the bubble (C, D). The KEGG signaling pathways.
The molecular docking assay showed that the AKI and MAPK1 proteins have a stronger affinity for HT disease molecules. Luteolin (MOL000006) was considered as the uppermost active ingredient of PV against HT. Meanwhile, there was also a strong association between kaempferol (MOL000422) and AKI with MAPK1. The AKI and MAPK1 protein active pockets showed that the small molecules luteolin and kaempferol have a compact binding pattern (Figure 7).
The precise pathogenesis of HT remains unclear. HT is the most prevalent autoimmune thyroid disorder; currently, there is no effective means of preventing and treating HT. Hashimoto’s thyroiditis (HT) is usually manageable by levothyroxine (L-T4) administration, reducing the thyroid volume and supplementing the lack of hormones. Despite thyroid hormone replacement, some euthyroid patients with HT will continue to experience persistent symptoms that reduce their quality of life. PV has been empirically used to treat thyroid disorders, including HT in TCMSP, which has been applied for thousands of years. Some of its basic mechanism remains unknown. Recent studies have shown that PV plays an essential role in reducing the titers of TPO-Ab, TG-Ab, and Th17 cells in autoimmune and inflammatory disorders, including HT (Wang et al., 2019; hui ZRGwsbydwy, 2000; Yang et al., 2007; Zhang, 2014; Hu et al., 2019; Ala et al., 2015; Wiersinga and Wilmar, 2001). In addition, several recent studies have demonstrated that the anti-inflammatory effect of PV is related to NF-κB in stimulated macrophages (Wu et al., 2010; Yu et al., 2012). This study demonstrates that PV has a potential therapeutic effect on HT and could explore novel anti-inflammatory therapies for its treatment.
Studies have suggested that the innate immune response in thyrocytes facilitates autosensitization, which may eventually lead to thyroid autoimmunity (Akira et al., 2011; Kawashima et al., 2013). In the study, eleven main active components were screened of PV: luteolin, quercetin, beta-sitosterol, kaempferol, stigmasterol, morin, spinasterol, delphinidin, vulgaxanthin-I, poriferasterol monoglucoside_qt, and stigmast-7-enol. Molecular docking showed that the active ingredients, including luteolin and kaempferol, had a good affinity for the hub disease proteins in clinical therapeutics (Xia et al., 2016; Habza-Kowalska et al., 2019). The results demonstrated that luteolin has a strong affinity for disease proteins of HT, and quercetin had a strong affinity for serum thyroid peroxidase (TPO). These results indicated that luteolin and kaempferol might play some important roles in the treatment of HT. Flavonoids are a large group of plant‐derived compounds, and it is well established that certain flavonoids exhibit anti‐inflammatory properties (Lee et al., 2017). Luteolin is one of the most common flavones with antioxidant, anticancer, anti-inflammatory, and antiapoptotic properties in TCMSP (De Stefano et al., 2021). A previous study also suggests that kaempferol is a potential bioaccessible TPO activator based on effective LOX inhibitors, further indicating potentially health-promoting effects for HT (Habza-Kowalska et al., 2019). Some studies have also shown that luteolin’s mechanisms showed potent anti-inflammatory activity, including the activation of NF-κB, which leads to the expression of IL-6 and COX-2 (Xagorari et al., 2001; Ching-Chow et al., 2004). Additionally, kaempferol, a polyphenol, is a bioactive substance with antioxidative, antimutagenic, antibacterial, and antiviral activities.
The KEGG enrichment analysis revealed that the chief pathways were concentrated in the AGE-RAGE signaling pathway in the pathogenesis of diabetes and its complications, the TNF signaling pathway, the PI3K–Akt signaling pathway, pathways in cancer, the mitogen-activated protein kinase (MAPK) signaling pathway, and some related to thyroid cancer. The present study identified JUN, AKI, MAPK1, and TP53 hub genes using the PPI network analysis. These results indicated that PV affected HT through the following pathways. Furthermore, a GO functional analysis also demonstrated many biological processes, including the inflammatory response, the cell-to-cell reaction, and the metabolic process in the present study. The TNF signaling pathway plays an essential functional role in regulating the inflammatory response (Noack and Miossec, 2017). Luteolin acts as an anti-inflammatory agent by regulating the TNF signaling pathway (Zhang et al., 2018; Zhang et al., 2019). A previous study demonstrated that the MAPK signaling pathway was revealed to be correlated with the progression of HT (Luo et al., 2018). Several studies have exhibited significant anti-inflammatory activity effects by inhibiting the MAPK signaling pathways (Chen et al., 2016; Wei et al., 2016). Luteolin is a common flavonoid that exhibits intense anti-inflammatory activity through the MAPK signaling pathway (Aziz et al., 2018). The key target gene of MAPK1 was involved in the MAPK signaling pathway.
Meanwhile, the MAPK signaling pathway plays an important role in developing thyroid carcinoma, including cell proliferation and cell survival. The PI3K/AKT signaling pathway plays a key role in regulating the activation of inflammatory response cells and releasing inflammatory transmitters to the chronic inflammatory response in HT (Li et al., 2018). By regulating the PI3K–AKT signaling pathway, luteolin might be the critical pathway against inflammation, thus realizing the treatment of HT (Huang et al., 2020). Kaempferol-activated PI3K/AKT signaling exerts anti-inflammatory effects (Imran et al., 2019; Harikrishnan et al., 2020; Jantan et al., 2021).
The present study included some limitations. First, some further verified experiments need to be conducted. Second, their specific molecular docking methods and locations need to be verified by further experiments. A comprehensive understanding of the key gene target of PV and HT is key towards therapeutic discovery and development.
In summary, the network pharmacology and molecular docking show that luteolin and kaempferol were the main active components of PV, which indicated that they might play an essential role in treating HT. PV may act against HT mainly through the TNF signaling pathway, the MAPK signaling pathway, and the PI3K–Akt signaling pathway.
The raw data supporting the conclusions of this article will be made available by the authors, without undue reservation.
Conception: X-xG and BX. Design and revision of the manuscript: X-xG, L-kZ, and FS. Analysis and interpretation of data: X-xG, L-kZ, FS, J-h, W-sC, Y-yL, and S-jL.
This research was supported by Guangzhou Medicine and Health Care Technology Projects (20211A011010).
The authors declare that the research was conducted in the absence of any commercial or financial relationships that could be construed as a potential conflict of interest.
All claims expressed in this article are solely those of the authors and do not necessarily represent those of their affiliated organizations, or those of the publisher, the editors, and the reviewers. Any product that may be evaluated in this article, or claim that may be made by its manufacturer, is not guaranteed or endorsed by the publisher.
Special thanks are due to Keqin Liu for support and encouragement.
Akira, K., Kazunari, T., Takeshi, A., Wu, H., Mariko, S., Aya, Y., et al. (2011). Fragments of Genomic DNA Released by Injured Cells Activate Innate Immunity and Suppress Endocrine Function in the Thyroid. Endocrinology 152 (4), 1702–1712. doi:10.1210/en.2010-1132
Ala, S., Akha, O., Kashi, Z., Asgari, H., Bahar, A., and Sasanpour, N. (2015). Dose Administration Time from before Breakfast to before Dinner Affect Thyroid Hormone Levels? Caspian J. Intern. Med. 6, 134–140.
Aziz, N., Kim, M. Y., and Cho, J. Y. (2018). Anti-inflammatory Effects of Luteolin: A Review of In Vitro, In Vivo, and In Silico Studies. J. Ethnopharmacol 225, 342–358. doi:10.1016/j.jep.2018.05.019
Barabási, A., and Oltvai, Z. N. (2004). Network Biology: Understanding the Cell's Functional Organization. Nat. Rev. Genet. 5 (2), 101–113. doi:10.1038/nrg1272
Cao, H., Li, S., Xie, R., Xu, N., Qian, Y., Chen, H., et al. (2018). Exploring the Mechanism of Dangguiliuhuang Decoction against Hepatic Fibrosis by Network Pharmacology and Experimental Validation. Front. Pharmacol. 9, 187. doi:10.3389/fphar.2018.00187
Caturegli, P., De Remigis, A., Chuang, K., Dembele, M., Iwama, A., Iwama, S., and Hashimoto's, H. (2013). Hashimoto's Thyroiditis: Celebrating the Centennial through the Lens of the Johns Hopkins Hospital Surgical Pathology Records. Thyroid 23, 142–150. doi:10.1089/thy.2012.0554
Chao, G., Zhu, Y., and Fang, L. (2020). Correlation between Hashimoto's Thyroiditis–Related Thyroid Hormone Levels and 25-Hydroxyvitamin D. Front. Endocrinol. 11, 4. doi:10.3389/fendo.2020.00004
Chen, C. C., Lin, M. W., Liang, C. J., and Wang, S. H. (2016). The Anti-inflammatory Effects and Mechanisms of Eupafolin in Lipopolysaccharide-Induced Inflammatory Responses in RAW264.7 Macrophages. Plos One 11, e0158662. doi:10.1371/journal.pone.0158662
Chen, F., Kawashima, A., Luo, Y., Kiriya, M., and Suzuki, K. (2020). Innate Immune-Modulatory Activity of Prunella Vulgaris in Thyrocytes Functions as a Potential Mechanism for Treating Hashimoto's Thyroiditis. Front. Endocrinol. 11, 579648. doi:10.3389/fendo.2020.579648
Ching-Chow, C., Man-Ping, C., Wei-Chien, H., Yi-Chu, L., and Ya-Jen, C. (2004). Flavonoids Inhibit Tumor Necrosis Factor-α-Induced Up-Regulation of Intercellular Adhesion Molecule-1 (ICAM-1) in Respiratory Epithelial Cells through Activator Protein-1 and Nuclear Factor-Κb: Structure-Activity Relationships. Mol. Pharmacol. 66 (3), 683–693. doi:10.1124/mol.66.3
Consortium UP (2010). The Universal Protein Resource (UniProt) in 2010. Nucleic Acids Res. 38, D142–D148. doi:10.1093/nar/gkp846
De Stefano, A., Caporali, S., Di Daniele, N., Rovella, V., Cardillo, C., Schinzari, F., et al. (2021). Anti-Inflammatory and Proliferative Properties of Luteolin-7-O-Glucoside. Int. J. Mol. Sci. 22, 1321. doi:10.3390/ijms22031321
Gan, X., Feng, J., Deng, X., Shen, F., Lu, J., Liu, Q., et al. (2021). The Significance of Hashimoto's Thyroiditis for Postoperative Complications of Thyroid Surgery: a Systematic Review and Meta-Analysis. Ann. R. Coll. Surg. Engl. 103, 223–230. doi:10.1308/rcsann.2020.7013
Habza-Kowalska, E., Gawlik-Dziki, U., and Dziki, D. (2019). Mechanism of Action and Interactions between Thyroid Peroxidase and Lipoxygenase Inhibitors Derived from Plant Sources. Biomolecules 9, 663. doi:10.3390/biom9110663
Harikrishnan, H., Jantan, I., Alagan, A., and Haque, M. A. (2020). Modulation of Cell Signaling Pathways by Phyllanthus Amarus and its Major Constituents: Potential Role in the Prevention and Treatment of Inflammation and Cancer. Inflammopharmacology 28, 1–18. doi:10.1007/s10787-019-00671-9
Hu, Y., Zhang, L., Chen, H., Liu, X., Zheng, X., Shi, H., et al. (2019). Analysis of Regulatory T Cell Subsets and Their Expression of Helios and PD-1 in Patients with Hashimoto Thyroiditis. Int. J. Endocrinol. 2019, 5368473. doi:10.1155/2019/5368473
Huang, X. F., Zhang, J. L., Huang, D. P., Huang, A. S., Huang, H. T., Liu, Q., et al. (2020). A Network Pharmacology Strategy to Investigate the Anti-inflammatory Mechanism of Luteolin Combined with In Vitro Transcriptomics and Proteomics. Int. Immunopharmacol 86, 106727. doi:10.1016/j.intimp.2020.106727
hui ZRGwsbydwy (2000). Pharmacopoeia of the People's Republic of China. Beijing, China: Chemical Industry Press.
Imran, M., Rauf, A., Shah, Z. A., Saeed, F., Imran, A., Arshad, M. U., et al. (2019). Chemo-preventive and Therapeutic Effect of the Dietary Flavonoid Kaempferol: A Comprehensive Review. Phytother Res. 33, 263–275. doi:10.1002/ptr.6227
Jantan, I., Haque, M. A., Arshad, L., Harikrishnan, H., Septama, A. W., and Mohamed-Hussein, Z. A. (2021). Dietary Polyphenols Suppress Chronic Inflammation by Modulation of Multiple Inflammation-Associated Cell Signaling Pathways. J. Nutr. Biochem. 93, 108634. doi:10.1016/j.jnutbio.2021.108634
Kawashima, A., Yamazaki, K., Hara, T., Akama, T., Yoshihara, A., Sue, M., et al. (2013). Demonstration of Innate Immune Responses in the Thyroid Gland: Potential to Sense Danger and a Possible Trigger for Autoimmune Reactions. Thyroid 23, 477–487. doi:10.1089/thy.2011.0480
Lee, S. B., Kang, J. W., Kim, S. J., Ahn, J., Kim, J., and Lee, S. M. (2017). Afzelin Ameliorates D-Galactosamine and Lipopolysaccharide-Induced Fulminant Hepatic Failure by Modulating Mitochondrial Quality Control and Dynamics. Br. J. Pharmacol. 174, 195–209. doi:10.1111/bph.13669
Li, H., Min, J., Mao, X., Wang, X., Yang, Y., and Chen, Y. (2018). Edaravone Ameliorates Experimental Autoimmune Thyroiditis in Rats through HO-1-dependent STAT3/PI3K/Akt Pathway. Am. J. Transl Res. 10, 2037–2046.
Liontiris, M. I., and Mazokopakis, E. E. (2017). A Concise Review of Hashimoto Thyroiditis (HT) and the Importance of Iodine, Selenium, Vitamin D and Gluten on the Autoimmunity and Dietary Management of HT patients.Points that Need More Investigation. Hell J. Nucl. Med. 20, 51–56. doi:10.1967/s002449910507
Luo, X., Zheng, T., Mao, C., Dong, X., Mou, X., Xu, C., et al. (2018). Aberrant MRP14 Expression in Thyroid Follicular Cells Mediates Chemokine Secretion through the IL-1β/MAPK Pathway in Hashimoto's Thyroiditis. Endocr. Connect. 7, 850–858. doi:10.1530/EC-18-0019
Morris, G. M., Huey, R., Lindstrom, W., Sanner, M. F., Belew, R. K., Goodsell, D. S., et al. (2009). AutoDock4 and AutoDockTools4: Automated Docking with Selective Receptor Flexibility. J. Comput. Chem. 30 (16), 2785–2791. doi:10.1002/jcc.21256
Ning, K., Zhao, X., Ansgar, P., Chen, W. H., and Yang, J. (2017). Computational Molecular Networks and Network Pharmacology. Biomed. Res. Int. 2017, 7573904. doi:10.1155/2017/7573904
Noack, M., and Miossec, P. (2017). Selected Cytokine Pathways in Rheumatoid Arthritis. Semin. Immunopathol 39, 365–383. doi:10.1007/s00281-017-0619-z
Roehlen, N., Doering, C., Hansmann, M. L., Gruenwald, F., Vorlaender, C., Bechstein, W. O., et al. (2018). Vitamin D, FOXO3a, and Sirtuin1 in Hashimoto's Thyroiditis and Differentiated Thyroid Cancer. Front. Endocrinol. (Lausanne) 9, 527. doi:10.3389/fendo.2018.00527
Ru, J., Li, P., Wang, J., Zhou, W., Li, B., Huang, C., et al. (2014). TCMSP: a Database of Systems Pharmacology for Drug Discovery from Herbal Medicines. J. Cheminform 6, 13. doi:10.1186/1758-2946-6-13
Saito, R., Smoot, M. E., Ono, K., Ruscheinski, J., Wang, P. L., Lotia, S., et al. (2012). A Travel Guide to Cytoscape Plugins. Nat. Methods 9, 1069–1076. doi:10.1038/nmeth.2212
Shannon, P., Markiel, A., Ozier, O., Baliga, N. S., Wang, J. T., Ramage, D., et al. (2003). Cytoscape: A Software Environment for Integrated Models of Biomolecular Interaction Networks. Genome Res. 13: 2498–2504. doi:10.1101/gr.1239303
Su, X., Kong, L., Lei, X., Hu, L., Ye, M., and Zou, H. (2007). Biological Fingerprinting Analysis of Traditional Chinese Medicines with Targeting ADME/Tox Property for Screening of Bioactive Compounds by Chromatographic and MS Methods. Mini Rev. Med. Chem. 7, 87–98. doi:10.2174/138955707779317830
Tan, X., Zhang, X., Pan, L., Tian, X., and Dong, P. (2017). Identification of Key Pathways and Genes in Advanced Coronary Atherosclerosis Using Bioinformatics Analysis. Biomed. Res. Int. 2017, 1–12. doi:10.1155/2017/4323496
Tang, S., Jing, H., Huang, Z., Huang, T., Lin, S., Liao, M., et al. (2020). Identification of Key Candidate Genes in Neuropathic Pain by Integrated Bioinformatic Analysis. J. Cell Biochem. 121 (2), 1635–1648. doi:10.1002/jcb.29398
Wang, C., Jiang, W., Li, W., Lian, B., Chen, X., Hua, L., et al. (2011). Topological Properties of the Drug Targets Regulated by microRNA in Human Protein-Protein Interaction Network. J. Drug Target. 19, 354–364. doi:10.3109/1061186X.2010.504261
Wang, S. J., Wang, X. H., Dai, Y. Y., Ma, M. H., and Zhang, H. (2019). Prunella Vulgaris: A Comprehensive Review of Chemical Constituents, Pharmacological Effects and Clinical Applications. Curr. Pharm. Des. 25 (3), 359–369. doi:10.2174/1381612825666190313121608
Ward, R. A., Bethel, P., Cook, C., Davies, E., Debreczeni, J. E., Fairley, G., et al. (2017). Structure-Guided Discovery of Potent and Selective Inhibitors of ERK1/2 from a Modestly Active and Promiscuous Chemical Start Point. J. Med. Chem. 60, 3438–3450. doi:10.1021/acs.jmedchem.7b00267
Wei, L., Jiang, H. L., Cai, L. L., Min, Y., Dong, S. J., and Bing, M. (2016). Tanreqing Injection Attenuates Lipopolysaccharide-Induced Airway Inflammation through MAPK/NF-κB Signaling Pathways in Rats Model. Evidence-Based Complementray Altern. Med. 2016, 5292346. doi:10.1155/2016/5292346
Wiersinga, W. M., and Wilmar, M. (2001). Thyroid Hormone Replacement Therapy. Horm. Res. 56 Suppl 1, 74–81. doi:10.1159/000048140
Wu, W. I., Voegtli, W. C., Sturgis, H. L., Dizon, F. P., Vigers, G. P., and Brandhuber, B. J. (2010). Crystal Structure of Human AKT1 with an Allosteric Inhibitor Reveals a New Mode of Kinase Inhibition. PloS one 5, e12913. doi:10.1371/journal.pone.0012913
Xagorari, A., Papapetropoulos, A., Mauromatis, A., Economou, M., Fotsis, T., and Roussos, C. (2001). Luteolin Inhibits an Endotoxin-Stimulated Phosphorylation cascade and Proinflammatory Cytokine Production in Macrophages. J. Pharmacol. Exp. Ther. 296, 181–187.
Xia, N., Chen, G., Liu, M., Ye, X., Pan, Y., Ge, J., et al. (2016). Anti-inflammatory Effects of Luteolin on Experimental Autoimmune Thyroiditis in Mice. Exp. Ther. Med. 12 (6), 4049–4054. doi:10.3892/etm.2016.3854
Yang, K., Guo, K. Q., and Wu, H. Y. (2007). Clinical Effect of Prunellae Oral Liquid on Goiter with Different Thyroid Function. Zhongguo Zhong XI Yi Jie He Za Zhi 27, 37–39.
Yu, G., Wang, L. G., Han, Y., and He, Q. Y. (2012). clusterProfiler: an R Package for Comparing Biological Themes Among Gene Clusters. OMICS 16, 284–287. doi:10.1089/omi.2011.0118
Zhang, B. C., Li, Z., Xu, W., Xiang, C. H., and Ma, Y. F. (2018). Luteolin Alleviates NLRP3 Inflammasome Activation and Directs Macrophage Polarization in Lipopolysaccharide-Stimulated RAW264.7 Cells. Am. J. Transl Res. 10, 265–273.
Zhang, L., Wang, X., Zhang, L., Virgous, C., and Si, H. (2019). Combination of Curcumin and Luteolin Synergistically Inhibits TNF-α-Induced Vascular Inflammation in Human Vascular Cells and Mice. J. Nutr. Biochem. 73, 108222. doi:10.1016/j.jnutbio.2019.108222
Zhang, M., Yuan, Y., Zhou, W., Qin, Y., Xu, K., Men, J., et al. (2020). Network Pharmacology Analysis of Chaihu Lizhong Tang Treating Non-alcoholic Fatty Liver Disease. Comput. Biol. Chem. 86, 107248. doi:10.1016/j.compbiolchem.2020.107248
Keywords: Prunella vulgaris, Hashimoto’s thyroiditis, network pharmacology, molecular docking, anti-inflammatory response
Citation: Gan X-x, Zhong L-k, Shen F, Feng J-h, Li Y-y, Li S-j, Cai W-s and Xu B (2021) Network Pharmacology to Explore the Molecular Mechanisms of Prunella vulgaris for Treating Hashimoto’s Thyroiditis. Front. Pharmacol. 12:700896. doi: 10.3389/fphar.2021.700896
Received: 27 April 2021; Accepted: 25 August 2021;
Published: 06 October 2021.
Edited by:
Xiao Bin Zeng, Jinan University, ChinaReviewed by:
Claudio Ferrante, University of Studies G d'Annunzio Chieti and Pescara, ItalyCopyright © 2021 Gan, Zhong, Shen, Feng, Li, Li, Cai and Xu. This is an open-access article distributed under the terms of the Creative Commons Attribution License (CC BY). The use, distribution or reproduction in other forums is permitted, provided the original author(s) and the copyright owner(s) are credited and that the original publication in this journal is cited, in accordance with accepted academic practice. No use, distribution or reproduction is permitted which does not comply with these terms.
*Correspondence: Bo Xu, ZXlib3h1QHNjdXQuZWR1LmNu; Wen-song Cai, Y2Fpd2Vuc29uZ0AxNjMuY29t
†These authors share first authorship
‡ORCID: Bo Xu, https://orcid.org/0000-0001-6384-6685
Disclaimer: All claims expressed in this article are solely those of the authors and do not necessarily represent those of their affiliated organizations, or those of the publisher, the editors and the reviewers. Any product that may be evaluated in this article or claim that may be made by its manufacturer is not guaranteed or endorsed by the publisher.
Research integrity at Frontiers
Learn more about the work of our research integrity team to safeguard the quality of each article we publish.