- Department of Pharmacology, School of Basic Medical Sciences, Health Science Center, Peking University and Beijing Key Laboratory of Tumor Systems Biology, Peking University, Beijing, China
Ganoderma lucidum (Lingzhi) polysaccharide peptide (GL-pp) is a component of the globally acknowledged traditional Chinese medicine Ganoderma lucidum; Ganoderma lucidum is known for its sedative, hypnotic, immune regulatory, antitumor, and other pharmacological effects. In recent years, sleep disorders have been linked to many diseases and human body disorders, including cancer. Some experimental studies in mice found that sleep fragmentation could promote tumor development and progression. However, effects on GL-pp on tumor metastasis under circumstances of sleep disorders have rarely been studied. Thus, in this study, we used mice with sleep fragmentation (SF) bearing B16-F10-luc-G5 melanoma tumors to investigate the effect of SF on melanoma metastasis. Furthermore, we investigated the antitumor and antimetastatic effects of GL-pp (80 mg/kg) in mice suffering from SF and bearing B16-F10-luc-G5. Then, whole proteomics was used to analyze the differences in protein expression in the lung tissue between SF mice bearing B16-F10-luc-G5 with and without GL-pp administration. High-throughput pyrosequencing of 16S rRNA was also used to analyze the impact of GL-pp on the gut microbiota composition in SF mice bearing B16-F10-luc-G5. Last, the effects of GL-pp on macrophage polarization and TNF-α serum levels were detected. Collectively, we found that SF significantly facilitated the B16-F10-luc-G5 melanoma tumor metastasis in mice, while GL-pp significantly reduced B16-F10-luc-G5 melanoma tumor metastasis under the condition of SF, in which proteomics and gut microbiota had been changed greatly.
Introduction
Modern people face great pressure and suffer from anxiety, depression, and other adverse psychological conditions, which lead to sleep disorder of varying degrees. An analysis in China claimed a 15.0% proportion of the pooled insomnia prevalence in 2017 (Cao et al., 2017). Sleep disorders can have extensive adverse effects both physically and mentally; for example, they can promote obesity and impair cognition (Espie, 2002; Wang et al., 2014; André et al., 2019). They are also listed as IARC Group 2A carcinogens, and a number of epidemiological and clinical studies have found that multiple sleep disorders significantly increase the risk of cancer (Davis et al., 2001; Schernhammer et al., 2003; Blask, 2009). Sleep fragmentation (SF) is a common type of sleep disorder generally caused by obstructive sleep apnea (Young et al., 2002); to some extent, it significantly increases the cancer incidence and mortality in younger patients (Campos-Rodriguez et al., 2013; Martínez-García et al., 2014), and this effect has been confirmed in animal experiments (Cao et al., 2015). Moreover, recent clinical studies have indicated that sleep fragmentation strongly correlates with tumor metastasis; for example, a case–control study of 11,412 women indicated that sleep disorders were associated with a significant increase in the presence of breast cancer metastases (Jacob et al., 2018). Sleep disorder is also a prominent problem of cancer patients (Lee et al., 2004), and cancer might cause or aggravate sleep disorder. An epidemiological survey showed that 41% of cancer patients suffered from sleep disorders, with 76% accounting for sleep fragmentation (Davidson et al., 2002). Sleep disorders in cancer patients are mostly caused by worries and cancer pain, and also by medications (Borniger et al., 2015). A previous study indicated that during the chemical therapy, the proportion of sleep disorder reached 43%, which is three times higher than that in the general population (Palesh et al., 2010). The correlation of sleep fragmentation and cancer further implied the importance of considering sleep fragmentation in tumor-related research.
Ganoderma lucidum (Lingzhi) is a multifunctional Chinese herbal medicine and has been extensively studied for the last 20 years. It has a wide range of pharmacological activities, including immunomodulatory effect (Xu et al., 2011), antitumor effect (Zhao et al., 2010), neuropharmacological effect (Cui et al., 2019), and protective effect on the cardiovascular system (Xie et al., 2016). Several studies have shown the inhibitory effects of Ganoderma lucidum on tumor growth and metastasis in vivo (Loganathan et al., 2014; Lin and Hsu, 2016) and its inhibitory effects on cell migration in vitro (Liang et al., 2015; Zhao et al., 2018a). Ganoderma lucidum polysaccharide peptide (GL-pp) is one of the principal active components of Ganoderma lucidum and has demonstrated its antitumor function in several kinds of tumors (Cao and Lin, 2004). However, its role in tumor metastasis under SF has not yet been studied. Ganoderma lucidum has sedation and hypnosis efficacy, and some modern studies have confirmed that the Ganoderma lucidum extract has hypnotic effects in freely moving rats and ICR male mice (Chu et al., 2007; Cui et al., 2012; Feng and Wang, 2019). Hence, in the present study, we used the lung metastasis model of melanoma carcinoma and studied the effect and potential mechanisms of GL-pp on the metastasis of tumors under the SF conditions.
Materials and Methods
GL-pp Extracted From Ganoderma lucidum
GL-pp was isolated by extracting the fruit body of Ganoderma lucidum with boiling water. The content of GL-pp in Ganoderma lucidum is 2.33%. It is a polysaccharide peptide with a molecular weight of 512,500, and the ratio of polysaccharides to peptide is 94.84%: 5.16%. The polysaccharides consist of d-rhamnose, d-xylose, d-fructose, d-galactose, and d-glucose linked together by β-glycosidic bonds with molar ratios of 0.549: 3.614: 3.167: 0.556: 6.89. GL-pp is a hazel-colored water-soluble powder, provided by Prof. Shuqian Lin, Fuzhou Institute of Green Valley Bio-Pharm Technology, China.
Cell Culture
B16-F10-luc-G5 cells were engineered to stably express firefly luciferase (Xenogen Corporation, Alameda, CA). The cells were cultured in DMEM containing 10% heat-inactivated fetal blood serum (FBS), 100 μg/ml streptomycin, and 100 U/mL penicillin in a humidified incubator at 37°C with 5% CO2 in air.
Animal Model and Drug Administration
Six-week-old female BALB/c nude mice, weighing 18–20 g, purchased from the Experimental Animal Center of Peking University (Grade II, Certificate No. 11–00–0,004) were housed under the same conditions (a 12-h light/dark cycle and constant temperature of 24 ± 1°C, free access to food and water) for 7 days of adaptation. The mice were randomly divided into four groups: control group under general conditions with no further treatment (CON group), tumor group with the burden of B16-F10-luc-G5 cells (Tumor group), T + SF group with SF and the burden of B16-F10-luc-G5 cells (T + SF group), and GL-pp group with SF, tumor cells burden, and the administration of 80 mg/kg GL-pp (GL-pp group).
B16-F10-luc-G5 cells (5 × 106 cells/mL per mouse) were injected into the mice through the tail vein. SF was induced by a sweeping pole with an interval of 2 min in the cages during the mouse sleep period (12:00 pm to 16:00 pm) every day. GL-pp (80 mg/kg) was administered orally via a gastric probe for 15 days after the inoculation of B16-F10-luc-G5 cells in the GL-pp group.
On day 15, after anesthesia and euthanasia, the lungs, blood serum, and intestines of all mice were harvested for further processing, and the flowchart is shown in Figure 1.
Animal handling and related procedures were approved by the Institutional Animal Care and Use Committee of Peking University Health Science Center.
In Vivo Bioluminescence Imaging
The mice were anesthetized with 1–3% isoflurane (Abbott Laboratories, Chicago, IL), and they received D-luciferin dissolved in phosphate-buffered saline (PBS) at a dosage of 150 mg/kg by intraperitoneal injection on day 8, day 11, and day 15 for bioluminescence imaging. Ten minutes after the injection, the bioluminescence images were collected and measured with the Xenogen IVIS imaging system (Xenogen Corp, Alameda, CA, United States). A region of interest was drawn on each mouse, and the luminescent images were acquired. The resulting gray scale photographic and pseudocolor luminescent images were automatically superimposed by software so that identification of any optical signal with location on the mouse was facilitated. Optical images were displayed and analyzed with the Igor (WaveMetrics, Lake Oswego, OR) and IVIS Living Image (Xenogen) software packages. Regions were manually drawn around the bodies of the mice to assess signal intensity emitted. Optical signal was expressed as photon flux, in units of photons/s/cm2/steradian.
Lung Histological Observation
The lung tissues were fixed with 4% paraformaldehyde and embedded using paraffin. Paraffin-embedded sections (4 µm) were deparaffinized and stained with hematoxylin and eosin (H&E) using a standard protocol. Five random fields from each sample were taken on each hematoxylin and eosin–stained lung tissue section. The alveolar integrity was evaluated by the area percentage of alveoli in the lung observed by light microscopy with ImageJ software. The mean value was accepted as representative of the sample.
Sample Preparation for Shotgun Analysis
Lung tissues were flash-frozen in liquid nitrogen and stored at −80°C. Proteins were extracted using RIPA lysis buffer, and each group was analyzed in triplicate.
Protein samples (200 μg) from each group were processed in line with the manufacturer’s protocol (Wiśniewski et al., 2009). Proteins were concentrated using Vivacon 500 filtration tubes (Cat No. VNO1HO2, Sartorius Stedim Biotech, United Kingdom), mixed with 100 μL of 8 M urea in 0.1 M Tris/HCL (pH 8.5), and centrifuged at 14,000 g, 20°C, for 15 min. This step was performed twice, after which 10 μL of 0.05 M tris-(2-carboxyethyl) phosphine (TCEP) in water was added, and the samples were incubated at 37°C for 1 h; then, 10 μL of 0.1 M iodoacetamide (IAA) was added, and the samples were incubated in darkness for 30 min. Finally, 4 μg of trypsin (Promega, Madison, WI) in 100 μL of 50 mM NH4HCO3 was added to each filter. The protein to enzyme ratio was 50:1. The samples were incubated overnight at 37°C, and the released peptides were collected by centrifugation.
High-pH reverse-phase chromatography was performed using a Dionex Ultimate 3,000 Micro Binary HPLC Pump system (Yang et al., 2012). The digested peptide mixture was reconstituted with 600 μL buffer A (20 mM ammonium formate in water, pH 10) and loaded onto a 2.1-mm × 150-mm Waters BEH130 C-18 column containing 3.5 μm particles. Peptides were eluted at a flow rate of 230 μL/min with a gradient of buffer B (20 mM ammonium formate in 80% acetonitrile, pH 10): 5% for 5 min, 5%–15% for 15 min, 15%–25% for 10 min, 25%–55% for 10 min, and 55–95% for 5 min. The system was then maintained in 95% buffer B for 5 min before equilibrating with 5% buffer B for 10 min prior to the next injection. Elution was monitored by measuring the absorbance at 214 nm, and fractions were collected every 2 min. The fractions containing eluted peptides were pooled into 15 fractions based on peptide density (Yang et al., 2012) and vacuum-dried for nano-ESI-LC-MS/MS analysis. The related flowchart is shown in Figure 2.
LC-MS/MS Analysis
LC-MS experiments were performed on a nano-flow HPLC system (Easy-nLC II, Thermo Fisher Scientific, Waltham, MA, United States) connected to an LTQ-Orbitrap Velos Pro (Linear quadrupole ion trap-Orbitrap mass analyzer) mass spectrometer (Thermo Fisher Scientific) equipped with Nanospray Flex Ion Source (Thermo Fisher Scientific). The peptide mixtures (5 μL) were injected onto a pre-column (Easy-column C18-A1, 100 μm I.D. × 20 mm, 5 μm, Thermo Fisher Scientific) at a flow rate of 5 μL/min. Chromatographic separation was performed on a reverse-phase C18 column (Easy-column C18-A2, 75 μm I.D. × 100 mm, 3 μm, Thermo Fisher Scientific) with a 60-min gradient of 2–40% acetonitrile in 0.1% formic acid at a flow rate of 300 nL/min. The electrospray voltage was maintained at 2.2 kV with the capillary temperature at 250°C. The LTQ-Orbitrap was operated in a data-dependent mode to simultaneously measure full-scan MS spectra (m/z 350–2000) in the Orbitrap with a mass resolution of 60,000 at m/z 400. After the full-scan survey, 15 most abundant ions detected in the full-MS scan were measured in the LTQ-Orbitrap using collision-induced dissociation (CID).
Protein Identification and Quantitation
Data analysis was performed with MaxQuant software (v1.4.1.2). For protein identification, MS/MS data were submitted to the UniProt human protein database (release 3.43, 72,340 sequences) using the Andromeda search engine (Cox et al., 2011) with the following settings: trypsin cleavage, fixed modification of carbamidomethylation of cysteine, variable modifications of methionine oxidation, a maximum of two missed cleavages, and a false discovery rate calculated by searching the decoy database. Other parameters were set as default. The results were imported into Microsoft Excel for further analysis.
Label-free quantitation (LFQ) was also performed in MaxQuant. The minimum ratio count for LFQ was set to 2, with the match-between-runs option enabled. Other parameters were set as default. Upregulated and downregulated proteins were defined based on a significantly altered protein ratio (p < 0.05).
Global Signal Transduction Network
The global signal transduction networks that had been experimentally validated were used for the observation of protein–protein and protein–DNA interactions. From the KEGG database, we were able to illustrate the interaction between differentially expressed genes (Jia et al., 2011). The nodes in the network were connected when their corresponding encoded gene products were connected directly or indirectly by a linker gene in the interaction network. The importance of each gene was measured by counting the number of upstream, downstream, or binding genes, which were shown as in-degree, out-degree, or degree, respectively. The degree was defined as the link number of one node with others, and genes with higher degrees occupied more important positions in the signaling network. p < 0.05 was considered statistically significant.
Functional Enrichment Analysis
Gene Ontology (GO) Biological Process and Kyoto Encyclopedia of Genes and Genomes (KEGG) pathway enrichment analysis were performed on Metascape (https://metascape.org/) (Zhou et al., 2019). All genes in the genome were used as the enrichment background. Terms with a p-value less than 0.01, an enrichment factor over 1.5, and a minimum count of three were grouped into clusters based on the membership similarities. Kappa scores were used as the similarity metric, and the similarity threshold of sub-trees was 0.3. The most statistically significant term within a cluster was chosen to represent the cluster. p-values were calculated based on the accumulative hypergeometric distribution, and the Benjamini–Hochberg procedure was used for multiple test correction.
A subset of enriched terms was selected and rendered as a network plot, and the terms with a similarity over 0.3 were connected by edges. The terms were selected with the best p-values from each of the 20 clusters and with the constraint of no more than 15 terms per cluster and no more than 250 terms in total. The network was visualized using Cytoscape (Shannon et al., 2003), where each node represented an enriched term and was colored by its cluster ID.
Microbial Sequencing
The DNA from the intestinal content was isolated with a QIAamp DNA Stool Mini Kit (Qiagen) following the manufacturer’s instructions. The diluted samples were then PCR-amplified using bar-coded primers, including 16S V4 (515F and 806R), V3–V4, V4–V5, and V5–V7 regions. The Ion Plus Fragment Library Kit (48 rxns, Thermo Fisher Scientific) was used to build the library, and after Qubit quantification and library test, the Ion S5 XL System (Thermo Fisher Scientific) was used for sequencing. The low-quality parts of reads were cut off using Cutadapt (v1.9.1) (Martin, 2011), and then the sample data were split from the reads according to the barcode to get raw reads. The chimeric sequence was identified compared with the species annotation database (https://github.com/torognes/vsearch/) and removed to obtain the final effective data (clean reads) (Haas et al., 2011). The clean reads were clustered to operational taxonomic units (OTUs) at a 97% identity using UPARSE software (v7.0.1001) (Edgar, 2013). The taxonomic information was obtained with the Mothur method and the SSUrRNA database of SILVA132 (Quast et al., 2012) for species annotation analysis, and all data were homogenized based on the sample with the least data. Alpha and beta diversity indexes were calculated using QIIME software (v1.9.1), and the LDA threshold was set at four to perform LDA effect size (LEfSe) analysis. The taxa with significant differences were identified with the T test and the Wilcoxon test.
Flow Cytometry
The tissue (1 mm3 blocks) was added to a tissue grinder with 1 ml saline and homogenate. Then, 10 ml saline was added into the lung homogenate, and cell suspension was collected and filtered with a 300-mesh nylon filter. The fluorescence-labeled PE anti-CD86 (BioLegend, San Diego, CA) or PE anti-CD206 (BioLegend) antibodies and FITC anti-F4/80 antibody (Abcam) were added and incubated at 37°C for 1 h. Samples were then washed twice using PBS and analyzed on an FACS Calibur (BD Biosciences).
Elisa Experiment
Blood samples were obtained from each mouse and allowed to clot at room temperature for 30 min and centrifuged for 10 min at 3,000 rpm to get blood serum. The serum TNF-α level was tested using a tumor necrosis factor-alpha assay kit (DE5101-96T, BioDee Biotechnology, Beijing, China) in accordance with the manufacturer’s instructions. Optical density (OD) of each well was detected at the wavelength of 450 nm within 15 min, and three replicates were repeated.
Statistical Analysis
Data were expressed as mean ± standard deviation (SD). Differences between groups were analyzed with multi-factor analysis of variance (multi-way ANOVA, MANOVA), followed by the Tukey comparison test with GraphPad Prism software (San Diego, CA), unless otherwise stated. Statistical significance was defined as p < 0.05.
Results
GL-pp Abated the Acceleration Effect of SF on Tumor Metastasis in Mice Bearing B16-F10-luc-G5.
The tumor metastasis was reflected by in vivo imaging as the lung luminescent intensity and the number of lung metastatic foci. The survival rate and body weight were used to reflect the overall survival status (Figure 3). The survival rate was equal in all groups (100%), while the body weight in the T + SF group was lighter than that in the Tumor group. In vivo imaging showed significantly stronger lung luminescence intensity in the T + SF group than in the Tumor group (p < 0.05) on day 15. Moreover, the number of lung metastatic foci in the T + SF group was greater than that in the Tumor group. As for the GL-pp group, body weight was similar to that in the Tumor group and higher than that in the T + SF group. The in vivo lung luminescence intensity decreased significantly compared with the T + SF group (p < 0.05), while the number of lung metastatic foci decreased compared with that in the T + SF and Tumor groups.
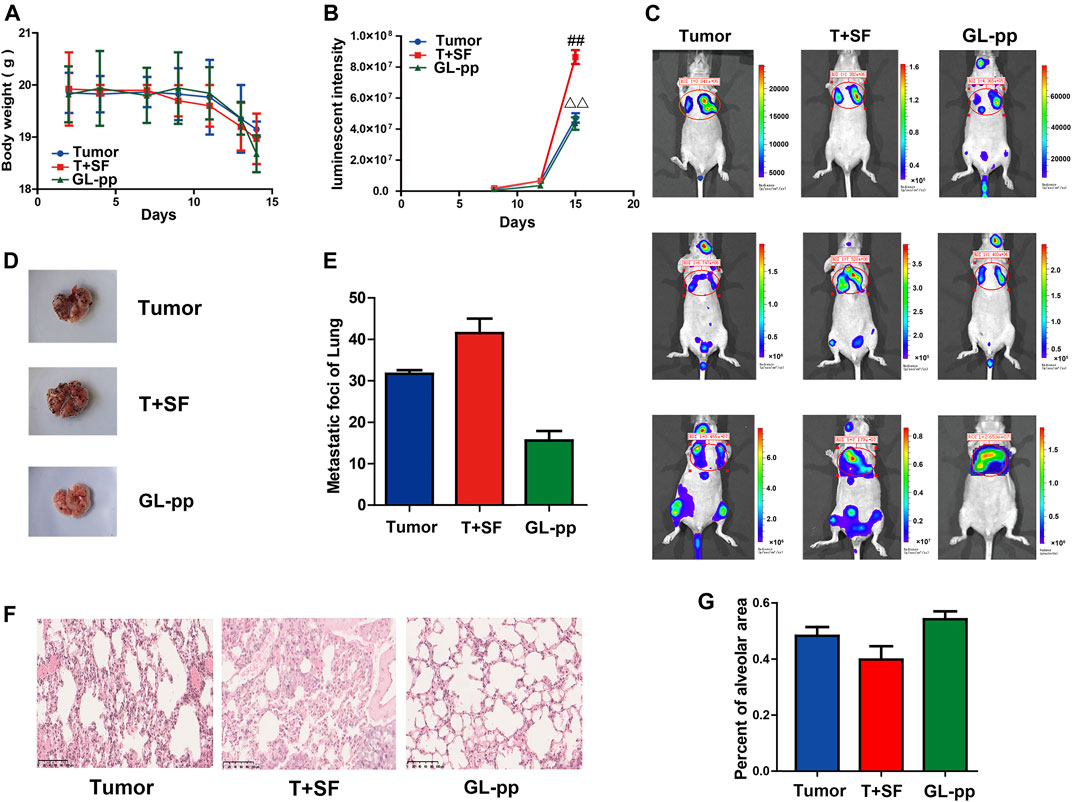
FIGURE 3. Inhibitory effects of GL-pp on tumor metastasis in mice with B16-F10-luc-G5 and SF. To show relative changes of tumor lung metastasis over time, luminescent intensity images were processed using living image in vivo. There were three groups, including tumor group with the burden of B16-F10-luc-G5 cells (Tumor group), T + SF group with SF and the burden of B16-F10-luc-G5 cells (T + SF group), and GL-pp group with SF, tumor cells burden, and the administration of 80 mg/kg GL-pp (GL-pp group). (A) The body weight of mice was observed over time. (B) The quantification of pulmonary luminescent intensity was recorded with living image in vivo. (C) Lung metastasis luminescent intensity images of a representative mouse from each group are shown. (D) Delegated mice pulmonary tissue with B16-F10-luc-G5 tumor foci of each group is shown. (E) The number of lung metastatic foci was counted. (F) H&E staining of pulmonary tissue sections (×100). (G) Percentage of alveolar area was calculated. The results are shown as mean ± SD, n = 5. ##p < 0.01 compared with the Tumor group; △△p < 0.01 compared with the T + SF group.
Consistent with the differences in lung luminescence intensity and metastatic foci, the pulmonary alveolar integrity in the T + SF group was a little worse than that in the Tumor group. Compared with the T + SF group and the Tumor group, the pulmonary alveolar integrity in the GL-pp group was better, indicating a protective effect of GL-pp on lung alveolar integrity in mice burdened with B16-F10-luc-G5 cells suffering from SF (Figures 3F, G).
GL-pp Altered the Protein Expression of Lung Metastatic Tissue in Tumor-Bearing Mice Subjected to SF and the Related GO and KEGG Pathway Analyses.
To identify the influence of GL-pp on lung tissue metastasis protein expression profiling, we detected the differentially expressed proteins between the GL-pp group and the T + SF group by a label-free quantitative whole proteomics approach. The datasets presented in this study can be found in online repositories (ProteomeXchange Consortium via the PRIDE partner repository with the dataset identifier PXD025171).
In total, 227 significantly differentially expressed (upregulated and downregulated) genes were identified at the p value of 0.05 (Supplementary Table S1). The heat map of the 227 genes is shown in Figure 4A, including 137 upregulated genes and 90 downregulated genes.
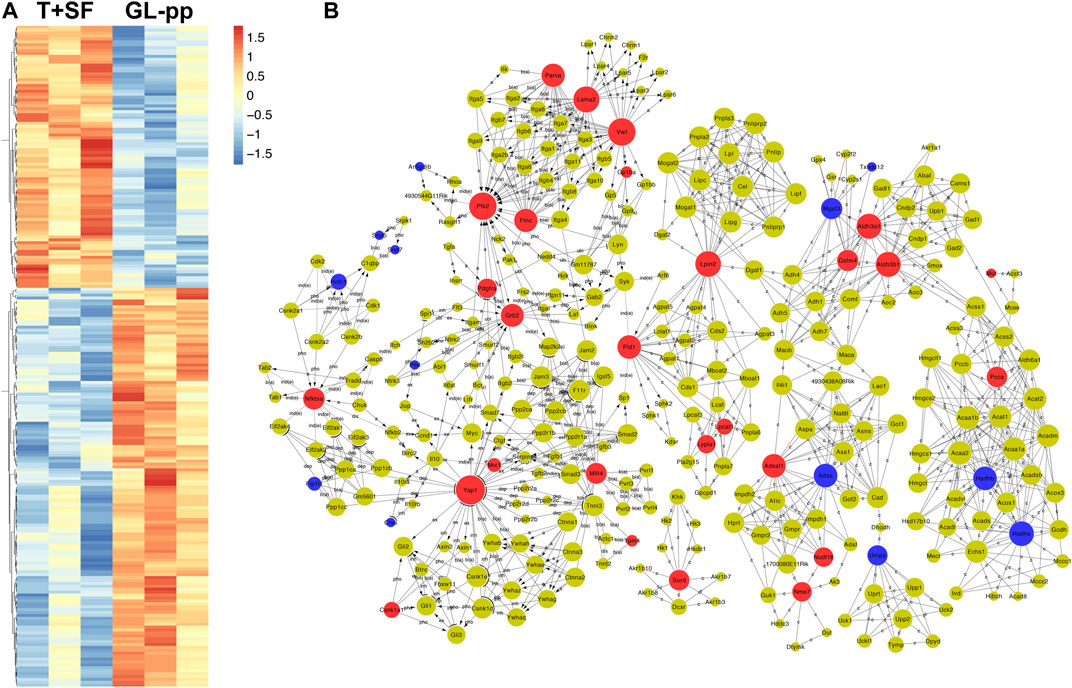
FIGURE 4. Quantitative protein expression profiling and the global signal transduction network of the significantly altered genes between the T + SF group and the GL-pp group. (A) Heat map of 227 significantly differentially expressed proteins between the T + SF group and the GL-pp group with the gene names listed on the right (orange indicates a higher expression level, and blue indicates a lower expression level, p < 0.05). (B) The global signal transduction network, the nodes in which were connected when their corresponding encoded gene products were connected directly or indirectly by a linker gene in the interaction network, and the size of each node indicates the degree of the gene. Red indicates upregulated and blue means downregulated key regulatory genes.
The global signal transduction networks that had been experimentally validated were used for the observation of protein–protein and protein–DNA interactions. We performed gene network analysis based on the KEGG signaling pathway influenced by the 227 differentially expressed genes (Figure 4B). The importance of each gene was measured by counting the number of upstream, downstream, or binding genes, which were shown as in-degree, out-degree, or degree, respectively. From the network, 43 key regulatory genes were identified (Table 1), including 30 upregulated genes (Lama2, Vwf, Ptk2, Yap1, Aldh3a1, Aldh3b1, Lpin2, Grb2, Pld1, Adssl1, Flnc, Parva, Nfkbia, Sord, Gstm4, Mllt4, Pcca, Mtm1, Nme7, Nudt16, Pdgfra, Lypla1, Tpm4, Csnk1a1, Lpcat1, Gp1ba, Mrc1, Naga, Mut, and Pi4ka) and 13 downregulated genes (Hadha, Hadhb, Adss, Mgst3, Umps, Hcfc1, Fip1l1, Pml, Arhgdib, Ctss, Srsf5, Srsf7, and Txndc12). The heat map of the 43 key regulatory genes is shown in Figure 5A. Genes with higher degrees occupied more important positions in the signaling network.
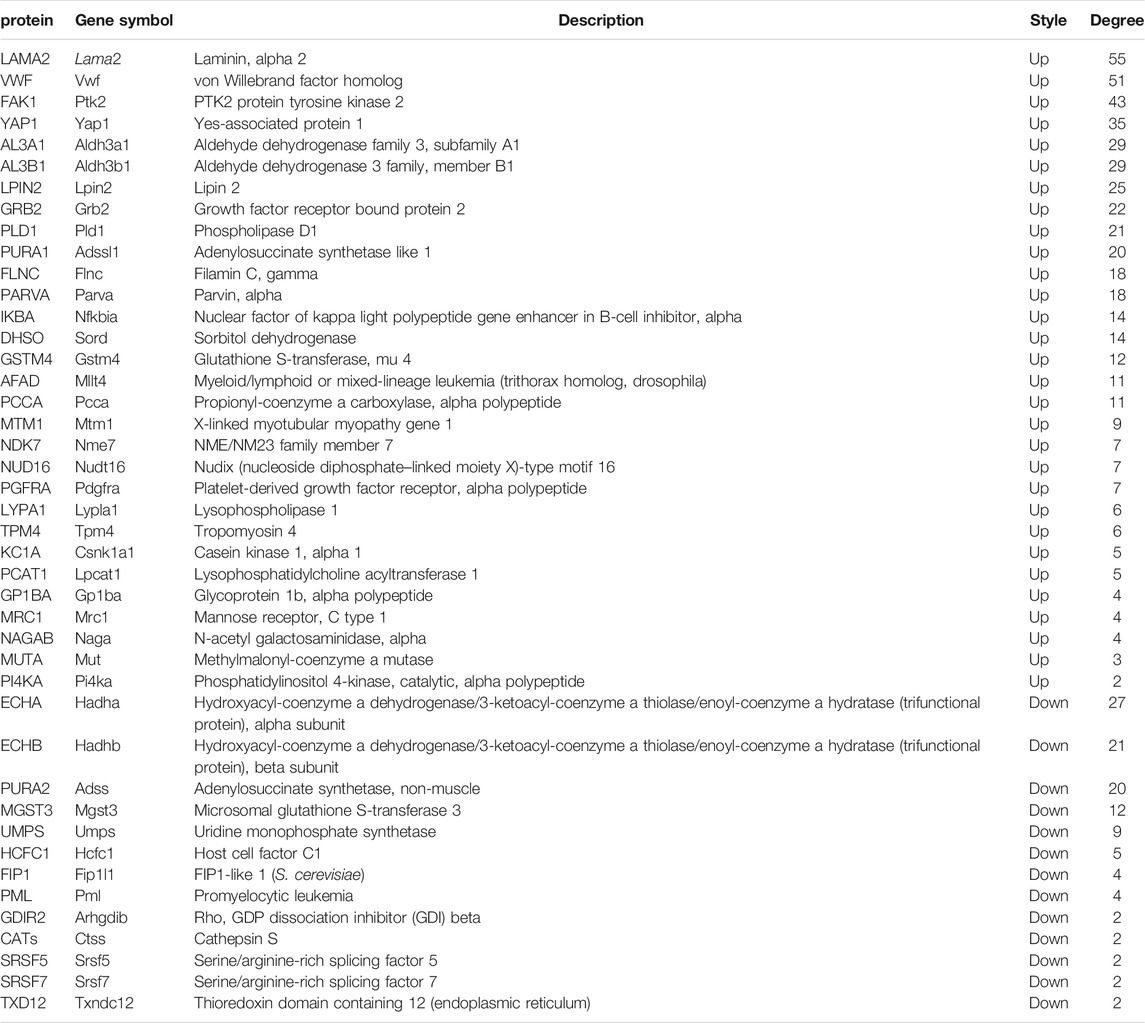
TABLE 1. Forty-three key regulatory genes identified from the global signal transduction network between the T + SF group and the GL-pp group.
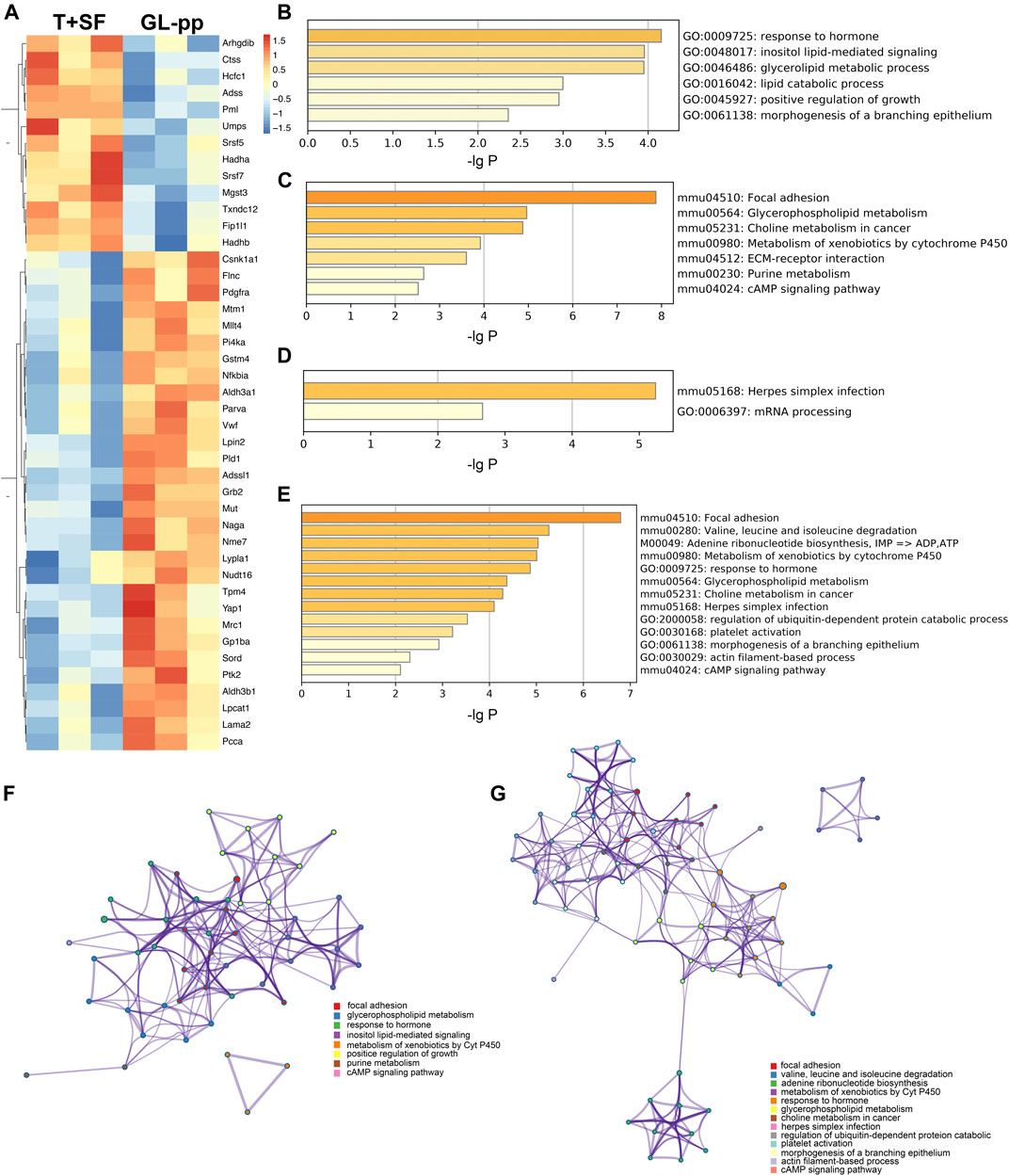
FIGURE 5. The key regulatory genes between the T + SF group and the GL-pp group and the function enrichment analysis. (A) Heat map of 43 key regulatory genes between the T + SF group and the GL-pp group with the gene names listed on the right (orange indicates a higher expression level, and blue indicates a lower expression level). (B) The significantly upregulated GO biological process clusters with upregulated different genes. (C) The significantly upregulated KEGG pathway clusters with upregulated different genes. (D) Significantly downregulated GO biological process and KEGG pathway clusters with downregulated difference genes. (E) Significantly altered GO biological process and KEGG pathway clusters among all 43 key regulatory genes. (F) The network plot of significantly upregulated pathways was visualized with Cytoscape software. (G) The combination of significantly altered pathways was visualized with Cytoscape software. Each node represented an enriched term, and nodes were colored by clusters, that is, the same color in the network means the same; for example, red represents focal adhesion.
Then, the GO biological process and KEGG signaling pathway enrichment analysis were performed separately according to the 30 upregulated key regulatory genes and 13 downregulated key regulatory genes. GL-pp significantly upregulated six main GO biological process clusters, including response to hormone, inositol lipid-mediated signaling, glycerolipid metabolic process, lipid catabolic process, positive regulation of growth, and morphogenesis of a branching epithelium (Figure 5B), and seven KEGG pathways, including focal adhesion, glycerophospholipid metabolism, choline metabolism in cancer, metabolism of xenobiotics by Cyt P450, purine metabolism, extracellular matrix (ECM)–receptor interaction, and cAMP signaling pathway (Figure 5C); in contrast, it significantly downregulated herpes simplex infection and mRNA processing pathways (Figure 5D).
We also performed the same analysis on all the 43 key regulatory genes. While some items were the same as above, some new terms were identified, including valine, leucine, and isoleucine degradation; adenine ribonucleotide biosynthesis; herpes simplex infection; platelet activation; and actin filament–based process (Figure 5E).
The upregulated GO and KEGG terms (Figure 5F) as well as combined terms (Figure 5G) were rendered as network plots to probe the connection between different terms. Visualized by Cytoscape, each node represented an enriched term, and the nodes were colored by clusters. The “focal adhesion” and “response to hormone” clusters had more and tighter correlations to other terms and were situated in the center of the plots. The “focal adhesion” cluster included choline metabolism in cancer (mmu05231), PI3K–Akt signaling pathway (mmu04151), and MAPK signaling pathway (mmu04010), whereas the “response to hormone” cluster included pathways in cancer (mmu05200), small-cell lung cancer (mmu05222), and chemokine signaling pathway (mmu04062). The detailed terms in the two clusters are shown in Table 2. Ptk2, Grb2, and Lama2 were included in most terms of “focal adhesion” and “response to hormone” clusters, and the degrees of the three genes were quite high. Especially, the degree of Lama2 was the highest among the 43 genes.
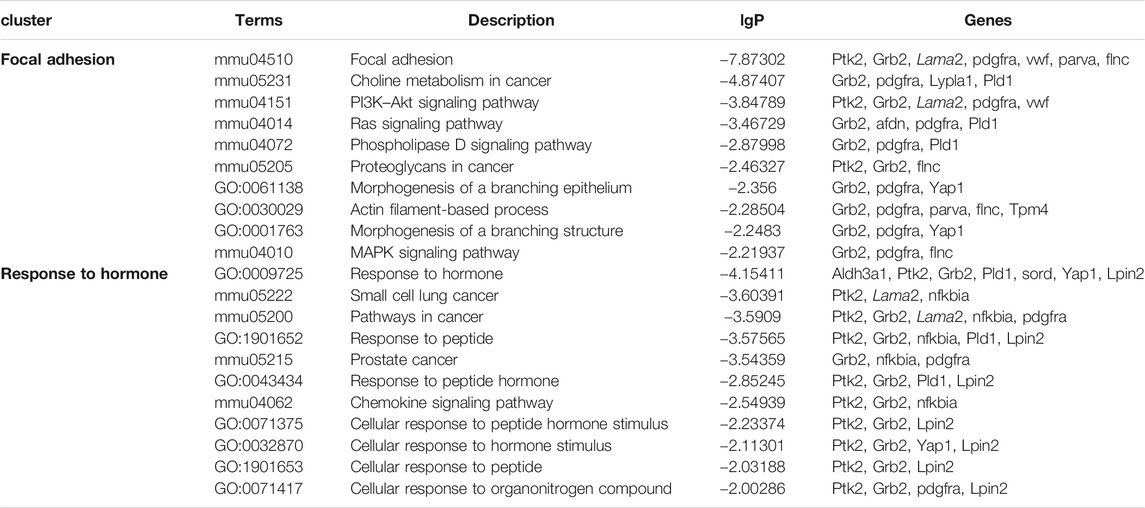
TABLE 2. Terms of the KEGG signal pathway and GO biology function in “focal adhesion” and “response to hormone” clusters based on the analysis of the global signal transduction network.
GL-pp Changed Gut Microbiota Composition in Mice Bearing B16-F10-luc-G5 With SF.
GL-pp may enhance antitumor immune function, and the intestinal tract is one of the tissues in close contact with the external environment and is an important organ system to defend the body against pathogenic microorganisms. In the present research, the gut microbiota composition was evaluated using the 16S rRNA high-throughput pyrosequencing. Data have been uploaded to the NCBI SRA database (Accession no.: PRJNA680896); 930,027 clean reads were clustered into OTUs under a similarity level of 97%, and the gut microbiota composition was elucidated (Figure 6A). The original sequencing data were uploaded to the NCBI SRA database (Accession no.: PRJNA680896). Most species (343) were the same in the four groups. Especially, the T + SF group and the GL-pp group had 396 taxa in common; the GL-pp group had 38 taxa that were not identified in the T + SF group, including 15 unique taxa of the GL-pp group. The rarefaction curve (Figure 6C) and rank abundance curve (Figure 6D) indicated that the observed species of gut microbiota in the T + SF group increased significantly compared with that in the Tumor group. Consistently, the cluster heat map of species abundance (Figure 6E) and the significant changes of observed species (Figure 7A) indicated that SF altered the composition of the gut microbiota intensively, while the GL-pp administration improved the situation.
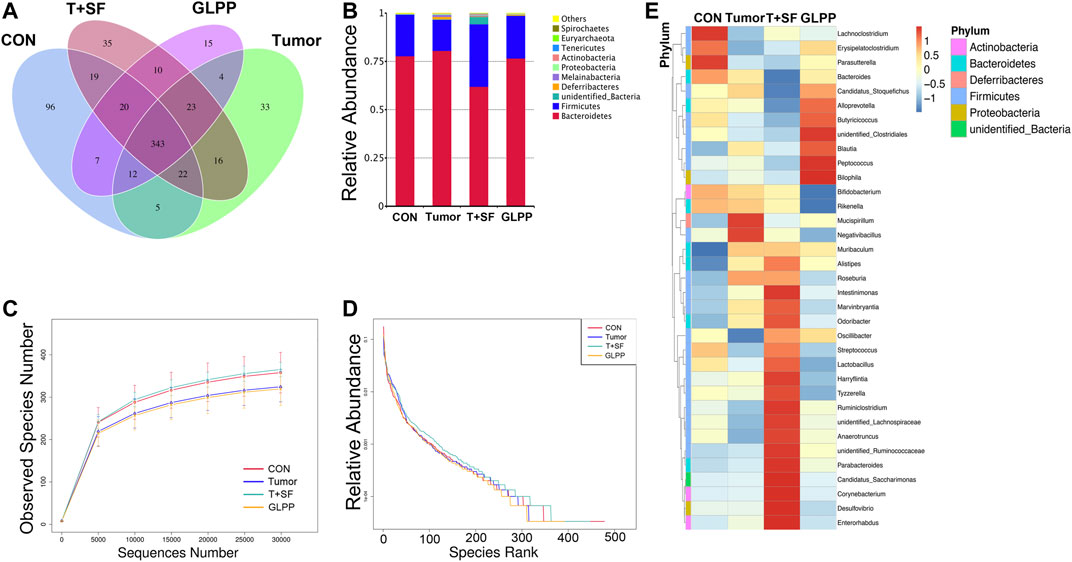
FIGURE 6. Effect of GL-pp on species number and relative abundance of the gut microbiota in mice with B16-F10-luc-G5 and SF. (A) The Venn diagram of OTUs in CON, Tumor, T + SF, and GL-pp groups. (B) Relative abundance of the gut microbiota in the four groups at the phylum level. (C) The rarefaction curves. (D) The relative abundance curves. (E) The heat map of top 35 genera of gut microbiota in the four groups with the phylum on the left side (different phyla are shown in different colors).
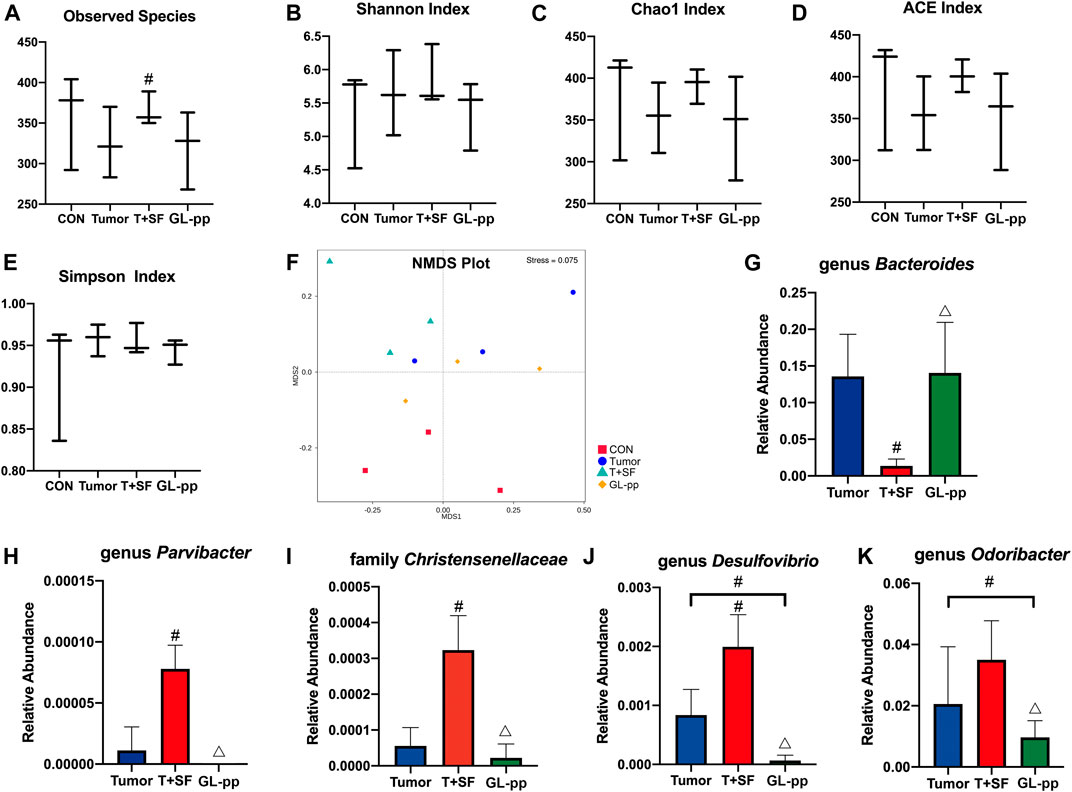
FIGURE 7. Effect of GL-pp on the diversity and the significantly altered taxa of gut microbiota in mice with B16-F10-luc-G5 and SF. The α-diversity analysis including observed species (A), Shannon Index (B), Chao1 Index (C), ACE Index (D), and Simpson Index (E) is shown. The NMDS analysis results are shown in (F). The relative abundance of significantly altered taxa, including genus Bacteroides(G), genus Parvibacter(H), family Christensenellaceae(I), genus Desulfovibrio(J), and genus Odoribacter(K) in the Tumor, T + SF, and GL-pp groups are shown in (G–K). n = 3. Results are shown as mean ± SD. #p < 0.05 compared with the Tumor group; △p < 0.05 compared with the T + SF group.
Compared with the CON and the Tumor groups, the relative abundance of Firmicutes increased significantly in the T + SF group (p < 0.05), while the relative abundance of Bacteroidetes decreased, leading to a rise in the Firmicutes:Bacteroidetes (F:B) ratio (p < 0.05). After the administration of GL-pp, this situation was significantly reversed to the former level as the CON group’s and the Tumor group’s abundance (p < 0.05, Figure 6B). However, the α-diversity analysis, including the Shannon index (Figure 7B), Chao1 index (Figure 7C), ACE index (Figure 7D), and Simpson index (Figure 7E), showed no statistically significant difference in gut microbiota diversity among the four groups, whereas the β-diversity analysis (including PCA, PCoA, and NMDS analysis) indicated a distinct clustering of the samples (Figure 7F).
Furthermore, the significantly altered taxa in the Tumor group, the T + SF group, and the GL-pp group were analyzed using the t test, MetaStat, and LDA effect size (LEfSe) method. Five typical taxa were identified: genus Bacteroides (phylum Bacteroidetes, Figure 7G), genus Parvibacter (phylum Actinobacteria, Figure 7H), family Christensenellaceae (phylum Firmicutes, Figure 7I), genus Desulfovibrio (phylum Proteobacteria, Figure 7J), and genus Odoribacter (phylum Bacteroidetes, Figure 7K). They were decreased (Bacteroides) or increased (Parvibacter, Christensenellaceae, Desulfovibrio, and Odoribacter) significantly in the T + SF group (p < 0.05), while the levels of Bacteroides increased and those of Parvibacter, Christensenellaceae, Desulfovibrio, and Odoribacter decreased in the GL-pp group when compared with the levels of those in the T + SF group (p < 0.05).
GL-pp Influenced the Macrophages Polarization and Blood Serum TNF-α Level in Mice Bearing B16-F10-luc-G5 with SF.
To determine the differentiation direction of macrophages, which existed in tumor environment, we detected the ratio of M1 macrophage to M2 macrophage in the lung tissue of mice bearing B16-F10-luc-G5 with SF. As shown in Figure 8, compared with the CON group, the M1/M2 ratio in both the Tumor group and the T + SF group slightly diminished, indicating a tendency to more polarization to M2 macrophages. The level of serum TNF-α significantly increased in both the Tumor group and the T + SF group, compared with the CON group (p < 0.01). The M1/M2 ratio was significantly elevated in the GL-pp group compared with that in the Tumor group or the T + SF group (p < 0.01). Consistently, the TNF-α serum level decreased significantly in the GL-pp group compared with that in the Tumor group or the T + SF group (p < 0.01).
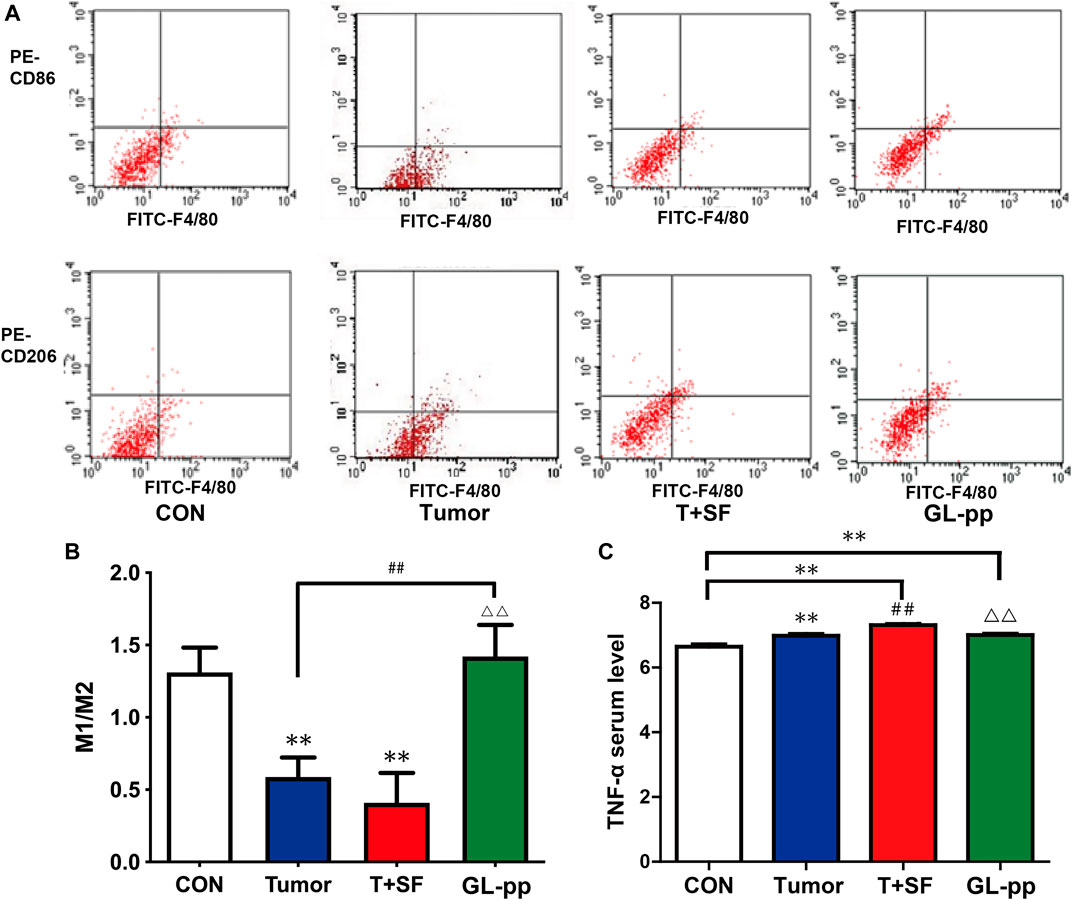
FIGURE 8. Effect of GL-pp on TAM polarization and TNF-α serum level in mice with B16-F10-luc-G5 and SF. (A) The flow cytometry results with CD86 and CD206 label to show TAM polarization. The type of tumor-related macrophages in pulmonary metastasis tumor tissue was detected with CD86 for type M1 macrophage and CD206 for type M2 macrophage. (B) The statistical results of M1/M2 ratio. (C) The TNF-α serum level in mice detected by ELISA. n = 5. The results are shown as mean ± SD. **p <0.01 compared with the CON group; ##p < 0.01 compared with the Tumor group; △△p < 0.01 compared with the T + SF group.
Discussion
This study clarified the inhibitory effect of GL-pp on tumor metastasis in mice bearing B16-F10-luc-G5 subjected to SF. First, we confirmed that SF significantly facilitated the lung metastasis in mice bearing B16-F10-luc-G5, which is consistent with previous studies (Cao et al., 2015; Hakim et al., 2014). Next, GL-pp treatment was able to significantly inhibit lung tumor metastasis in mice bearing B16-F10-luc-G5 tumor cells under the SF conditions. GL-pp’s antitumor effects have been studied and shown in several animal tumor models (Ma et al., 2013; Lin and Hsu, 2016). Nevertheless, this is the first time that GL-pp’s antitumor function under the influence of sleep disorder has been clarified.
With the whole proteomics analysis, we found 43 key regulatory genes in lung tissue of mice bearing tumor cells with SF after GL-pp administration. Genes with higher degrees occupied more important positions in the signaling network, and the degree of Lama2 was the highest among the 43 genes when analyzed by the global signal transduction network, indicating a strong correlation between Lama2 and other genes in the signal transduction network. Downregulation of Lama2 expression was also found, notably in liver, ovarian, lung, and colorectal cancer (Jhunjhunwala et al., 2014). In laryngeal squamous cell carcinoma (Ni et al., 2012) and breast cancer (Mefford and Mefford, 2012), downregulation of Lama2 expression was linked to tumor progression. Previous studies found that a decrease in Lama2 expression was accompanied by an increase in DNA methylation near the transcription start site; promoter DNA methylation and downregulation across multiple cancer types indicate that Lama2 is a tumor suppressor gene (Wang et al., 2019).
The GO and KEGG analyses revealed that focal adhesion and response to hormone clusters had more and tighter correlations to other terms and were situated in the center of the plots. Focal adhesion, in which focal adhesion kinase (FAK) is overexpressed in cancer cells and tumor microenvironment, has been shown to improve tumor progression and metastasis (Sulzmaier et al., 2014). Lin TY et al. found that one kind of G. lucidum recombinant protein inhibited the epithelial to mesenchymal transition (EMT) process by disturbing the function of cell adhesion and focal adhesion kinase (FAK) in lung cancer cells (A549 and CL1-5 human NSCLC adenocarcinoma cell lines and LLC1 Lewis lung carcinoma cell line) (Lin and Hsu, 2016; Sun and Sun, 2019).
Ptk2 and Grb2 are important genes participating in the pathway of focal adhesion, and we found that the degrees of the two genes were quite high. Overexpression of Ptk2, which encodes FAK, improves cell migration, invasion, adhesion, proliferation, and survival in ovarian and other cancers (Levy et al., 2019). Growth factor receptor–bound protein 2 (Grb2) has been linked to many oncogenic pathways. For example, in breast cancer, Grb2 links with SHP1 to proceed tumor progression. In non–small-cell lung cancer, Grb2 is involved in tumor metastasis by regulating both MAPK and Akt pathways (Ijaz et al., 2018). According to our experiment and previous studies, we speculate that GL-pp might inhibit tumor metastasis by disturbing the function of FAK and the pathway involving Ptk2 and Grb2.
Recently, the correlation among gut microbiota, inflammation, and cancer has been widely considered (Morgillo et al., 2018). Under normal conditions, the gut microbiota and gastrointestinal environment are in a mutually beneficial relationship (Lozupone et al., 2012). However, a lot of evidence has indicated that gut microbiota has a strong influence on oncogenesis, tumor progression, and response to therapy (Zitvogel et al., 2015). For example, gut microbiota can significantly affect tumor growth in mice (Sethi et al., 2018), contribute to antitumor immunity, limit tumor expansion (Li et al., 2019), and significantly influence the efficacy of immunotherapy (Routy et al., 2018). These findings highlighted the importance to investigate the alterations in the gut microbiota.
As expected, the 16S rRNA sequencing indicated that the Firmicutes:Bacteroidetes (F:B) ratio significantly increased in tumor-bearing mice subjected to SF; after the administration of GL-pp, the ratio decreased significantly compared with the T + SF group. Notably, the F:B ratio is considered an indicator of structural modifications (Poroyko et al., 2016), and the increased F:B ratio is associated with inflammation (Ismail et al., 2011) and poor prognosis of many diseases (Li et al., 2017; Spychala et al., 2018). As for the specific taxa, our results showed an antagonizing effect of GL-pp against tumor under SF conditions. The genus Bacteroides, which has an immunosuppressive function (Zhang et al., 2018), was significantly downregulated in the T + SF group and upregulated in the GL-pp group. Desulfovibrio, a kind of opportunistic pathogens, has been related to some inflammatory diseases (Cani et al., 2012) through H2S-related damage of the gut epithelium energy metabolism (Babidge et al., 1998; Cao et al., 2019). Our results also showed a significant decrease of Desulfovibrio in the GL-pp group compared with both the T + SF and the Tumor groups. As for Christensenellaceae and Odoribacter, Mancabelli L et al. showed that Christensenellaceae could be one of the specific disease microbial biomarkers for colorectal cancer when compared with control subjects (Mancabelli et al., 2017). Zackular JP et al. demonstrated that the destructive changes in the gut microbiome were closely related to the development of colorectal cancer, and mice with intestinal colonization of intestinal microflora among tumor-bearing mice had a higher risk of tumorigenesis. Tumor-bearing mice showed enrichment of Odoribacter genera and others, and antibiotic treatment of these bacteria was shown to reduce the number and size of tumors (Zackular et al., 2013). These findings indicate a possible immunoregulative effect of GL-pp, which enhances the intestinal barrier function. Therefore, GL-pp might achieve the antitumor effect partially through altering the gut microbiota.
In our present study, we used immunecompromised Balb/c athymic nude mice to observe the lung metastasis effect of B16F10 under the IVIS luminescent system. We could deduce that GL-pp had inhibitory effects on SF-promoted tumor metastasis even under the circumstances of T-cell deficiency.
Tumor-associated macrophages (TAMs) are a cluster of immune cells observed in the microenvironment of solid tumors; they have two principal phenotypes (M1 and M2) (Komohara et al., 2016). At present, M1 is mostly related to the inflammatory response and antitumor immunity, whereas M2 is associated with immunosuppressive functions and tumor growth promotion (Chanmee et al., 2014). Clinically, cancer patients with a higher M1/M2 ratio displayed a better prognosis and overall survival rate (de la Cruz-Merino et al., 2013; Williams et al., 2016). Hakim et al. (2014) exposed a group of mice to chronic SF, mimicking several sleep disorders; they observed significant increases in TAM counts in tumor tissue compared with the controls. They also found that SF induced TAM polarity shift toward M2 (Hakim et al., 2014). Our results also showed the decrease in the M1/M2 ratio in the Tumor and the T + SF groups. Our findings also showed that GL-pp at the dose of 80 mg/kg was able to significantly increase the M1/M2 ratio in lung metastasis tumor of mice with SF. Clearly, GL-pp increased the tendency of macrophage polarization from M2 to M1 in TAMs in vivo, indicating an antitumor effect of GL-pp.
Meanwhile, we showed that the serum TNF-α level increased in tumor-bearing mice subjected to SF; after the administration of GL-pp, the serum TNF-α level decreased. Some studies found that TNF-α was increased by the Ganoderma lucidum extract complex in the tumor-bearing mice serum (Zhao et al., 2018b); polysaccharides from fresh fruiting bodies of Ganoderma lucidum were also able to greatly increase the TNF-α level in macrophage culture cells (Wang et al., 1997). Interestingly, other studies found that triterpene ganoderic acid C1 isolated from Ganoderma lucidum downregulated TNF-α production by macrophages and peripheral blood mononuclear cells (PBMCs) from Crohn’s disease subjects (Liu et al., 2015). Ganoderma lucidum also reduced the TNF-α level under the inflammatory conditions (Dudhgaonkar et al., 2009); inflammation is frequent in some kinds of cancers and is known to facilitate the growth and metastasis of tumor cells (Ali et al., 2018). Increased TNF-α is related to cancer cachexia, which is manifested by body weight loss, chronic nausea, fatigue, insomnia, and profuse sweating; in contrast, the reduction in TNF-α correlates with improved fatigability in cancer patients (Argilés and López, 1999; Mendes et al., 2015). GL-pp can also be used to treat chemotherapy-related fatigue and improve the quality of life of individuals by lowering the serum levels of TNF-α that were upregulated in patients undergoing chemotherapy (Lin and Sun, 2019). Therefore, the reduction of TNF-α observed in the current study may imply an anti-inflammatory effect of GL-pp.
Conclusion
Our study suggested that GL-pp had an antitumor metastasis effect under the SF conditions. Moreover, GL-pp affected the proteomics profile, gut microbiota composition, macrophage polarization, and TNF-α level in mice bearing B16-F10-luc-G5 with SF. We believe that the present study indicates a prospect for Ganoderma lucidum to be further studied and used widely in the future.
Data Availability Statement
The datasets presented in this study can be found in online repositories. The names of the repository/repositories and accession number(s) for microbiota data can be found below: https://www.ncbi.nlm.nih.gov/sra/PRJNA680896, and the mass spectrometry proteomic data can be found in the ProteomeXchange Consortium via the PRIDE partner repository with the dataset identifier PXD025171.
Ethics Statement
The animal study was reviewed and approved by the Institutional Animal Care and Use Committee of Peking University Health Science Center.
Author Contributions
HX completed the gut microbiota experiment and proteomic analysis and wrote the manuscript. YMZ, YNZ, and JL did the animal experiment. YMZ and JL revised the manuscript. DL, SL, and ZT helped to carry out the mechanism study and proteomic analysis. XL and ZL guided the experiments. YP designed the research and wrote the manuscript.
Funding
The National Natural Science Foundation of China (Nos. 81773765, 81270049, 30901815, 81874318, 81673453, and 81473235) funded this project.
Conflict of Interest
The authors declare that the research was conducted in the absence of any commercial or financial relationships that could be construed as a potential conflict of interest.
Supplementary Material
The Supplementary Material for this article can be found online at: https://www.frontiersin.org/articles/10.3389/fphar.2021.650216/full#supplementary-material
References
Ali, N. A. M., Saeed, H. A., and Othman, R. T. (2018). Immunostimulatory and Anti-inflammatory Effect of Ganoderma Lucidum on Breast Cancer Patients. Asian Pac. J. Cancer Biol. 3, 51–57.
André, C., Tomadesso, C., Flores, R., Branger, P., Rehel, S., Mézenge, F., et al. (2019). Brain and Cognitive Correlates of Sleep Fragmentation in Elderly Subjects with and without Cognitive Deficits. Alzheimer's Demen. Diagn. Assess. Dis. Monit. 11, 142–150. doi:10.1016/j.dadm.2018.12.009
Argilés, J. M., and López, S. F. J. (1999). The Role of Cytokines in Cancer Cachexia. Med. Res. Rev. 19, 223–248.
Babidge, W., Millard, S., and Roediger, W. (1998). Sulfides Impair Short Chain Fatty Acid β-oxidation at Acyl-CoA Dehydrogenase Level in Colonocytes: Implications for Ulcerative Colitis. Mol. Cell. Biochem. 181, 117–124. doi:10.1023/a:1006838231432
Blask, D. E. (2009). Melatonin, Sleep Disturbance and Cancer Risk. Sleep Med. Rev. 13, 257–264. doi:10.1016/j.smrv.2008.07.007
Borniger, J. C., Gaudier-Diaz, M. M., Zhang, N., Nelson, R. J., and DeVries, A. C. (2015). Cytotoxic Chemotherapy Increases Sleep and Sleep Fragmentation in Non-tumor-bearing Mice. Brain Behav. Immun. 47, 218–227. doi:10.1016/j.bbi.2014.11.001
Campos-Rodriguez, F., Martinez-Garcia, M. A., Martinez, M., Duran-Cantolla, J., Peña, M. d. l., Masdeu, M. J., et al. (2013). Association between Obstructive Sleep Apnea and Cancer Incidence in a Large Multicenter Spanish Cohort. Am. J. Respir. Crit. Care Med. 187, 99–105. doi:10.1164/rccm.201209-1671oc
Cani, P. D., Osto, M., Geurts, L., and Everard, A. (2012). Involvement of Gut Microbiota in the Development of Low-Grade Inflammation and Type 2 Diabetes Associated with Obesity. Gut microbes 3, 279–288. doi:10.4161/gmic.19625
Cao, J., Feng, J., Li, L., and Chen, B. (2015). Obstructive Sleep Apnea Promotes Cancer Development and Progression: a Concise Review. Sleep Breath 19, 453–457. doi:10.1007/s11325-015-1126-x
Cao, Q. Z., and Lin, Z. B. (2004). Antitumor and Anti-angiogenic Activity of Ganoderma Lucidum Polysaccharides Peptide. Acta Pharmacol. Sin 25, 833–838.
Cao, X., Wang, S-B., Zhong., B-L., Zhang, L., Ungvari, G. S., Ng, C. H., et al. (2017). The Prevalence of Insomnia in the General Population in China: A Meta-Analysis. PloS one 12. doi:10.1371/journal.pone.0170772
Cao, Y., Yao, G., Sheng, Y., Yang, L., Wang, Z., Yang, Z., et al. (2019). JinQi Jiangtang Tablet Regulates Gut Microbiota and Improve Insulin Sensitivity in Type 2 Diabetes Mice. J. Diabetes Res., 2019, 1872134. doi:10.1155/2019/1872134
Chanmee, T., Ontong, P., Konno, K., and Itano, N. (2014). Tumor-associated Macrophages as Major Players in the Tumor Microenvironment. Cancers 6, 1670–1690. doi:10.3390/cancers6031670
Chu, Q.-P., Wang, L.-E., Cui, X.-Y., Fu, H.-Z., Lin, Z.-B., Lin, S.-Q., et al. (2007). Extract of Ganoderma Lucidum Potentiates Pentobarbital-Induced Sleep via a GABAergic Mechanism. Pharmacol. Biochem. Behav. 86, 693–698. doi:10.1016/j.pbb.2007.02.015
Cox, J., Neuhauser, N., Michalski, A., Scheltema, R. A., Olsen, J. V., and Mann, M. (2011). Andromeda: a Peptide Search Engine Integrated into the MaxQuant Environment. J. Proteome Res. 10, 1794–1805. doi:10.1021/pr101065j
Cui, X.-Y., Cui, S.-Y., Zhang, J., Wang, Z.-J., Yu, B., Sheng, Z.-F., et al. (2012). Extract of Ganoderma Lucidum Prolongs Sleep Time in Rats. J. ethnopharmacology 139, 796–800. doi:10.1016/j.jep.2011.12.020
Cui, X., and Zhang, Y. (2019). “Neuropharmacological Effect and Clinical Applications of Ganoderma (Lingzhi),” in Ganoderma and Health. Editors Z. B. Lin, and B. X. Yang (Springer), 143–157. doi:10.1007/978-981-32-9421-9_5
Davidson, J. R., MacLean, A. W., Brundage, M. D., and Schulze, K. (2002). Sleep Disturbance in Cancer Patients. Soc. Sci. Med. 54, 1309–1321. doi:10.1016/s0277-9536(01)00043-0
Davis, S., Mirick, D. K., and Stevens, R. G. (2001). Night Shift Work, Light at Night, and Risk of Breast Cancer. JNCI J. Natl. Cancer Inst. 93, 1557–1562. doi:10.1093/jnci/93.20.1557
de la Cruz-Merino, L., Barco-Sánchez, A., Henao Carrasco, F., Nogales Fernández, E., Vallejo Benítez, A., Brugal Molina, J., et al. (2013). New Insights into the Role of the Immune Microenvironment in Breast Carcinoma. Clin. Dev. Immunol. 2013. doi:10.1155/2013/785317
Dudhgaonkar, S., Thyagarajan, A., and Sliva, D. (2009). Suppression of the Inflammatory Response by Triterpenes Isolated from the Mushroom Ganoderma Lucidum. Int. immunopharmacology 9, 1272–1280. doi:10.1016/j.intimp.2009.07.011
Edgar, R. C. (2013). UPARSE: Highly Accurate OTU Sequences from Microbial Amplicon Reads. Nat. Methods 10, 996–998. doi:10.1038/nmeth.2604
Espie, C. A. (2002). Insomnia: Conceptual Issues in the Development, Persistence, and Treatment of Sleep Disorder in Adults. Annu. Rev. Psychol. 53, 215–243. doi:10.1146/annurev.psych.53.100901.135243
Feng, X., and Wang, Y. (2019). Anti-inflammatory, Anti-nociceptive and Sedative-Hypnotic Activities of Lucidone D Extracted from Ganoderma Lucidum. Cel Mol Biol (Noisy-le-grand) 65, 37–42. doi:10.14715/cmb/2019.65.4.6
Haas, B. J., Gevers, D., Earl, A. M., Feldgarden, M., Ward, D. V., Giannoukos, G., et al. (2011). Chimeric 16S rRNA Sequence Formation and Detection in Sanger and 454-pyrosequenced PCR Amplicons. Genome Res. 21, 494–504. doi:10.1101/gr.112730.110
Hakim, F., Wang, Y., Zhang, S. X. L., Zheng, J., Yolcu, E. S., Carreras, A., et al. (2014). Fragmented Sleep Accelerates Tumor Growth and Progression through Recruitment of Tumor-Associated Macrophages and TLR4 Signaling. Cancer Res. 74, 1329–1337. doi:10.1158/0008-5472.can-13-3014
Ijaz, M., Wang, F., Shahbaz, M., Jiang, W., Fathy, A. H., and Nesa, E. U. (2018). The Role of Grb2 in Cancer and Peptides as Grb2 Antagonists. Ppl 24, 1084–1095. doi:10.2174/0929866525666171123213148
Ismail, N. A., Ragab, S. H., ElBaky, A. A., Shoeib, A. R. S., Alhosary, Y., and Fekry, D. (2011). Frequency of Firmicutes and Bacteroidetes in Gut Microbiota in Obese and normal Weight Egyptian Children and Adults. aoms 3, 501–507. doi:10.5114/aoms.2011.23418
Jacob, L., Scholten, P. C., Kostev, K., and Kalder, M. (2018). Association between Sleep Disorders and the Presence of Breast Cancer Metastases in Gynecological Practices in Germany: a Case-Control Study of 11,412 Women. Breast Cancer Res. Treat. 171, 443–448. doi:10.1007/s10549-018-4831-x
Jhunjhunwala, S., Jiang, Z., Stawiski, E. W., Gnad, F., Liu, J., Mayba, O., et al. (2014). Diverse Modes of Genomic Alteration in Hepatocellular Carcinoma. Genome Biol. 15, 436. doi:10.1186/s13059-014-0436-9
Jia, D., Wei, L., Guo, W., Zha, R., Bao, M., Chen, Z., et al. (2011). Genome-wide Copy Number Analyses Identified Novel Cancer Genes in Hepatocellular Carcinoma. Hepatology 54, 1227–1236. doi:10.1002/hep.24495
Komohara, Y., Fujiwara, Y., Ohnishi, K., and Takeya, M. (2016). Tumor-associated Macrophages: Potential Therapeutic Targets for Anti-cancer Therapy. Adv. Drug Deliv. Rev. 99, 180–185. doi:10.1016/j.addr.2015.11.009
Lee, K., Cho, M., Miaskowski, C., and Dodd, M. (2004). Impaired Sleep and Rhythms in Persons with Cancer. Sleep Med. Rev. 8, 199–212. doi:10.1016/j.smrv.2003.10.001
Levy, A., Alhazzani, K., Dondapati, P., Alaseem, A., Cheema, K., Thallapureddy, K., et al. (2019). Focal Adhesion Kinase in Ovarian Cancer: A Potential Therapeutic Target for Platinum and Taxane-Resistant Tumors. Ccdt 19, 179–188. doi:10.2174/1568009618666180706165222
Li, H.-L., Lu, L., Wang, X.-S., Qin, L.-Y., Wang, P., Qiu, S.-P., et al. (2017). Alteration of Gut Microbiota and Inflammatory Cytokine/Chemokine Profiles in 5-Fluorouracil Induced Intestinal Mucositis. Front. Cel. Infect. Microbiol. 7. doi:10.3389/fcimb.2017.00455
Li, Y., Tinoco, R., Elmén, L., Segota, I., Xian, Y., Fujita, Y., et al. (2019). Gut Microbiota Dependent Anti-tumor Immunity Restricts Melanoma Growth in Rnf5−/− Mice. Nat. Commun. 10, 1–16. doi:10.1038/s41467-019-09525-y
Liang, Z.-E., Yi, Y.-J., Guo, Y.-T., Wang, R.-C., Hu, Q.-L., and Xiong, X.-Y. (2015). Inhibition of Migration and Induction of Apoptosis in LoVo Human colon Cancer Cells by Polysaccharides from Ganoderma Lucidum. Mol. Med. Rep. 12, 7629–7636. doi:10.3892/mmr.2015.4345
Lin, T.-Y., and Hsu, H.-Y. (2016). Ling Zhi-8 Reduces Lung Cancer Mobility and Metastasis through Disruption of Focal Adhesion and Induction of MDM2-Mediated Slug Degradation. Cancer Lett. 375, 340–348. doi:10.1016/j.canlet.2016.03.018
Liu, C., Dunkin, D., Lai, J., Song, Y., Ceballos, C., Benkov, K., et al. (2015). Anti-inflammatory Effects of Ganoderma Lucidum Triterpenoid in Human Crohnʼs Disease Associated with Downregulation of NF-Κb Signaling. Inflamm. Bowel Dis. 21, 1918–1925. doi:10.1097/mib.0000000000000439
Loganathan, J., Jiang, J., Smith, A., Jedinak, A., Thyagarajan-sahu, A., Sandusky, G. E., et al. (2014). The Mushroom Ganoderma Lucidum Suppresses Breast-To-Lung Cancer Metastasis through the Inhibition of Pro-invasive Genes. Int. J. Oncol. 44, 2009–2015. doi:10.3892/ijo.2014.2375
Lozupone, C. A., Stombaugh, J. I., Gordon, J. I., Jansson, J. K., and Knight, R. (2012). Diversity, Stability and Resilience of the Human Gut Microbiota. Nature 489, 220–230. doi:10.1038/nature11550
Ma, C.-w., Feng, M., Zhai, X., Hu, M., You, L., Luo, W., et al. (2013). Optimization for the Extraction of Polysaccharides from Ganoderma Lucidum and Their Antioxidant and Antiproliferative Activities. J. Taiwan Inst. Chem. Eng. 44, 886–894. doi:10.1016/j.jtice.2013.01.032
Mancabelli, L., Milani, C., Lugli, G. A., Turroni, F., Cocconi, D., Sinderen, D. V., et al. (2017). Identification of Universal Gut Microbial Biomarkers of Common Human Intestinal Diseases by Meta-Analysis. FEMS Microbiol. Ecol. 93. doi:10.1093/femsec/fix153
Martin, M. (2011). Cutadapt Removes Adapter Sequences from High-Throughput Sequencing Reads. EMBnet j. 17, 10–12. doi:10.14806/ej.17.1.200
Martínez-García, M. A., Campos-Rodriguez, F., Durán-Cantolla, J., de la Peña, M., Masdeu, M. J., González, M., et al. (2014). Obstructive Sleep Apnea Is Associated with Cancer Mortality in Younger Patients. Sleep Med. 15, 742–748. doi:10.1016/j.sleep.2014.01.020
Mefford, D., and Mefford, J. (2012). Stromal Genes Add Prognostic Information to Proliferation and Histoclinical Markers: a Basis for the Next Generation of Breast Cancer Gene Signatures. PLoS One 7, e37646. doi:10.1371/journal.pone.0037646
Mendes, M. C. S., Pimentel, G. D., Costa, F. O., and Carvalheira, J. B. C. (2015). Molecular and Neuroendocrine Mechanisms of Cancer Cachexia. J. Endocrinol. 226, R29–R43. doi:10.1530/joe-15-0170
Morgillo, F., Dallio, M., Della Corte, C. M., Gravina, A. G., Viscardi, G., Loguercio, C., et al. (2018). Carcinogenesis as a Result of Multiple Inflammatory and Oxidative Hits: a Comprehensive Review from Tumor Microenvironment to Gut Microbiota. Neoplasia 20, 721–733. doi:10.1016/j.neo.2018.05.002
Ni, R. S., Shen, X., Qian, X., Yu, C., Wu, H., and Gao, X. (2012). Detection of Differentially Expressed Genes and Association with Clinicopathological Features in Laryngeal Squamous Cell Carcinoma. Oncol. Lett. 4, 1354–1360. doi:10.3892/ol.2012.920
Palesh, O. G., Roscoe, J. A., Mustian, K. M., Roth, T., Savard, J., Ancoli-Israel, S., et al. (2010). Prevalence, Demographics, and Psychological Associations of Sleep Disruption in Patients with Cancer: University of Rochester Cancer Center-Community Clinical Oncology Program. Jco 28, 292–298. doi:10.1200/jco.2009.22.5011
Poroyko, V. A., Carreras, A., Khalyfa, A., Khalyfa, A. A., Leone, V., Peris, E., et al. (2016). Chronic Sleep Disruption Alters Gut Microbiota, Induces Systemic and Adipose Tissue Inflammation and Insulin Resistance in Mice. Scientific Rep. 6, 1–11. doi:10.1038/srep35405
Quast, C., Pruesse, E., Yilmaz, P., Gerken, J., Schweer, T., Yarza, P., et al. (2012). The SILVA Ribosomal RNA Gene Database Project: Improved Data Processing and Web-Based Tools. Nucleic Acids Res. 41, D590–D596. doi:10.1093/nar/gks1219
Routy, B., Le Chatelier, E., Derosa, L., Duong, C. P. M., Alou, M. T., Daillère, R., et al. (2018). Gut Microbiome Influences Efficacy of PD-1-Based Immunotherapy against Epithelial Tumors. Science 359, 91–97. doi:10.1126/science.aan3706
Schernhammer, E. S., Laden, F., Speizer, F. E., Willett, W. C., Hunter, D. J., Kawachi, I., et al. (2003). Night-Shift Work and Risk of Colorectal Cancer in the Nurses' Health Study. JNCI J. Natl. Cancer Inst. 95, 825–828. doi:10.1093/jnci/95.11.825
Sethi, V., Kurtom, S., Tarique, M., Lavania, S., Malchiodi, Z., Hellmund, L., et al. (2018). Gut Microbiota Promotes Tumor Growth in Mice by Modulating Immune Response. Gastroenterology 155, 33–37. doi:10.1053/j.gastro.2018.04.001
Shannon, P., Markiel, A., Ozier, O., Baliga, N. S., Wang, J. T., Ramage, D., et al. (2003). Cytoscape: a Software Environment for Integrated Models of Biomolecular Interaction Networks. Genome Res. 13, 2498–2504. doi:10.1101/gr.1239303
Spychala, M. S., Venna, V. R., Jandzinski, M., Doran, S. J., Durgan, D. J., Ganesh, B. P., et al. (2018). Age‐related Changes in the Gut Microbiota Influence Systemic Inflammation and Stroke Outcome. Ann. Neurol. 84, 23–36. doi:10.1002/ana.25250
Sulzmaier, F. J., Jean, C., and Schlaepfer, D. D. (2014). FAK in Cancer: Mechanistic Findings and Clinical Applications. Nat. Rev. Cancer 14, 598–610. doi:10.1038/nrc3792
Sun, Y., and Sun, L. (2019). Cellular and Molecular Mechanism of Ganoderma (Lingzhi) against Tumor. Adv. Exp. Med. Biol. 1182, 79–118. doi:10.1007/978-981-32-9421-9_3
Wang, R.-Q., Lan, Y-L., Lou, J-C., Lyu, Y-Z., Hao, Y-C., Su, Q. F., et al. (2019). Expression and Methylation Status of LAMA2 Are Associated with the Invasiveness of Nonfunctioning PitNET. Ther. Adv. Endocrinol. Metab. 10, 2042018818821296. doi:10.1177/2042018818821296
Wang, S.-Y., Hsu, M.-L., Hsu, H.-C., Lee, S.-S., Shiao, M.-S., and Ho, C.-K. (1997). The Anti-tumor Effect of Ganoderma Lucidum Is Mediated by Cytokines Released from Activated Macrophages and T Lymphocytes. Int. J. Cancer 70, 699–705. doi:10.1002/(sici)1097-0215(19970317)70:6<699::aid-ijc12>3.0.co;2-5
Wang, Y., Carreras, A., Lee, S., Hakim, F., Zhang, S. X., Nair, D., et al. (2014). Chronic Sleep Fragmentation Promotes Obesity in Young Adult Mice. Obesity 22, 758–762. doi:10.1002/oby.20616
Williams, C. B., Yeh, E. S., and Soloff, A. C. (2016). Tumor-associated Macrophages: Unwitting Accomplices in Breast Cancer Malignancy. NPJ breast cancer 2, 1–12. doi:10.1038/npjbcancer.2015.25
Wiśniewski, J. R., Zougman, A., Nagaraj, N., and Mann, M. (2009). Universal Sample Preparation Method for Proteome Analysis. Nat. Methods 6, 359–362. doi:10.1038/nmeth.1322
Xie, Y.-Z., Yang, F., Tan, W., Li, X., Jiao, C., Huang, R., et al. (2016). The Anti-cancer Components of Ganoderma Lucidum Possesses Cardiovascular Protective Effect by Regulating Circular RNA Expression. Oncoscience 3, 203–207. doi:10.18632/oncoscience.316
Xu, Z., Chen, X., Zhong, Z., Chen, L., and Wang, Y. (2011). Ganoderma Lucidum Polysaccharides: Immunomodulation and Potential Anti-tumor Activities. Am. J. Chin. Med. 39, 15–27. doi:10.1142/s0192415x11008610
Yang, F., Shen, Y., Camp, D. G., and Smith, R. D. (2012). High-pH Reversed-phase Chromatography with Fraction Concatenation for 2D Proteomic Analysis. Expert Rev. Proteomics 9, 129–134. doi:10.1586/epr.12.15
Young, T., Peppard, P. E., and Gottlieb, D. J. (2002). Epidemiology of Obstructive Sleep Apnea. Am. J. Respir. Crit. Care Med. 165, 1217–1239. doi:10.1164/rccm.2109080
Zackular, J. P., Baxter, N. T., Iverson, K. D., Sadler, W. D., Petrosino, J. F., Chen, G. Y., et al. (2013). The Gut Microbiome Modulates colon Tumorigenesis. mBio 4, e00692–00613. doi:10.1128/mbio.00692-13
Zhang, Z., Liu, L., Tang, H., Jiao, W., Zeng, S., Xu, Y., et al. (2018). Immunosuppressive Effect of the Gut Microbiome Altered by High-Dose Tacrolimus in Mice. Am. J. Transpl. 18, 1646–1656. doi:10.1111/ajt.14661
Zhao, L., Dong, Y., Chen, G., and Hu, Q. (2010). Extraction, Purification, Characterization and Antitumor Activity of Polysaccharides from Ganoderma Lucidum. Carbohydr. Polym. 80, 783–789. doi:10.1016/j.carbpol.2009.12.029
Zhao, R., Chen, Q., and He, Y.-m. (2018). The Effect of Ganoderma Lucidum Extract on Immunological Function and Identify its Anti-tumor Immunostimulatory Activity Based on the Biological Network. Sci. Rep. 8, 12680. doi:10.1038/s41598-018-30881-0
Zhao, X., Zhou, D., Liu, Y., Li, C., Zhao, X., Li, Y., et al. (2018). Ganoderma Lucidum Polysaccharide Inhibits Prostate Cancer Cell Migration via the Protein Arginine Methyltransferase 6 Signaling Pathway. Mol. Med. Rep. 17, 147–157. doi:10.3892/mmr.2017.7904
Zhou, Y., Zhou, B., Pache, L., Chang, M., Khodabakhshi, A. H., Tanaseichuk, O., et al. (2019). Metascape Provides a Biologist-Oriented Resource for the Analysis of Systems-Level Datasets. Nat. Commun. 10, 1–10. doi:10.1038/s41467-019-09234-6
Keywords: Ganoderma lucidum polysaccharide peptide, sleep fragmentation, tumor metastasis, proteomics, gut microbiota
Citation: Xian H, Li J, Zhang Y, Li D, Zhu Y, Li S, Tan Z, Lin Z, Li X and Pan Y (2021) Antimetastatic Effects of Ganoderma lucidum Polysaccharide Peptide on B16-F10-luc-G5 Melanoma Mice With Sleep Fragmentation. Front. Pharmacol. 12:650216. doi: 10.3389/fphar.2021.650216
Received: 07 January 2021; Accepted: 09 June 2021;
Published: 08 July 2021.
Edited by:
Min Huang, Shanghai Institute of Materia Medica, Chinese Academy of Sciences, ChinaReviewed by:
Qiyang Shou, Zhejiang Chinese Medical University, ChinaJinhua Wang, Peking Union Medical College, Chinese Academy of Sciences, China
Copyright © 2021 Xian, Li, Zhang, Li, Zhu, Li, Tan, Lin, Li and Pan. This is an open-access article distributed under the terms of the Creative Commons Attribution License (CC BY). The use, distribution or reproduction in other forums is permitted, provided the original author(s) and the copyright owner(s) are credited and that the original publication in this journal is cited, in accordance with accepted academic practice. No use, distribution or reproduction is permitted which does not comply with these terms.
*Correspondence: Yan Pan, pannay26@bjmu.edu.cn