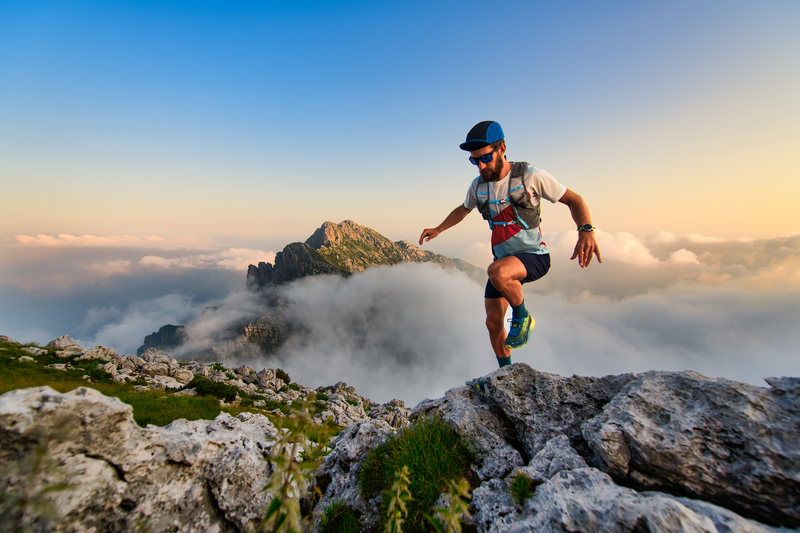
94% of researchers rate our articles as excellent or good
Learn more about the work of our research integrity team to safeguard the quality of each article we publish.
Find out more
ORIGINAL RESEARCH article
Front. Pharmacol. , 17 March 2021
Sec. Obstetric and Pediatric Pharmacology
Volume 12 - 2021 | https://doi.org/10.3389/fphar.2021.636097
This article is part of the Research Topic Model-Based Evaluation of Antimicrobial Agents in Children View all 16 articles
Background: Voriconazole is a potent antifungal drug with complex pharmacokinetics caused by time-dependent inhibition and polymorphisms of metabolizing enzymes. It also exhibits different pharmacokinetic characteristics between adults and children. An understanding of these alterations in pharmacokinetics is essential for pediatric dose optimization.
Objective: To determine voriconazole plasma exposure in the pediatric population and further investigate optimal dosage regimens.
Methods: An adult and pediatric physiologically based pharmacokinetic (PBPK) model of voriconazole, integrating auto-inhibition of cytochrome P450 3A4 (CYP3A4) and CYP2C19 gene polymorphisms, was developed. The model was evaluated with visual predictive checks and quantitative measures of the predicted/observed ratio of the area under the plasma concentration-time curve (AUC) and maximum concentration (Cmax). The validated pediatric PBPK model was used in simulations to optimize pediatric dosage regimens. The probability of reaching a ratio of free drug (unbound drug concentration) AUC during a 24-h period to minimum inhibitory concentration greater than or equal to 25 (fAUC24h/MIC ≥ 25) was assessed as the pharmacokinetic/pharmacodynamic index.
Results: The developed PBPK model well represented voriconazole's pharmacokinetic characteristics in adults; 78% of predicted/observed AUC ratios and 85% of Cmax ratios were within the 1.25-fold range. The model maintained satisfactory prediction performance for intravenous administration in pediatric populations after incorporating developmental changes in anatomy/physiology and metabolic enzymes, with all predicted AUC values within 2-fold and 73% of the predicted Cmax within 1.25-fold of the observed values. The simulation results of the PBPK model suggested that different dosage regimens should be administered to children according to their age, CYP2C19 genotype, and infectious fungal genera.
Conclusion: The PBPK model integrating CYP3A4 auto-inhibition and CYP2C19 gene polymorphisms successfully predicted voriconazole pharmacokinetics during intravenous administration in children and could further be used to optimize dose strategies. The infectious fungal genera should be considered in clinical settings, and further research with large sample sizes is required to confirm the current findings.
Voriconazole is an essential triazole antifungal agent with in vivo activity against a broad spectrum of yeasts and filamentous fungi, commonly used for the prophylaxis and treatment of various invasive fungal infections (IFI) (Clancy and Nguyen, 1998; Saravolatz et al., 2013; Perfect et al., 2003). However, a high interindividual plasma variability has been observed partially due to its nonlinear and time-dependent pharmacokinetics (Purkins et al., 2003; Pfizer, 2010). It also exhibits differences in clearance and bioavailability between adults and children (Schulz et al., 2019). All these factors complicate the successful therapeutic use of voriconazole in pediatric populations.
Voriconazole is a substrate for cytochrome P450 (CYP) enzymes. CYP2C19 is the primary enzyme that contributes to the main circulating metabolite of voriconazole, voriconazole N-oxide, while CYP3A4 and CYP2C9 are also responsible for its metabolism (Hyland et al., 2003). Only 2% of voriconazole is excreted unmetabolized in the urine (Levêque et al., 2006). The time-dependent inhibition (TDI) of CYP3A4 observed in in vitro studies (Li et al., 2020) may play a role in the elevated exposure to voriconazole at multiple doses, which could not be predicted from single-dose data (Purkins et al., 2003). Genetic polymorphisms of CYP2C19 are also a major determinant of the wide pharmacokinetic (PK) variability in voriconazole. Drug exposures from multiple doses of poor metabolizers (PMs) are almost 3 times higher than those from normal metabolizers (NMs) (Lee et al., 2012). Regarding age-related changes, the total body clearance in children is approximately 2–3-fold higher than that in adults (Levêque et al., 2006). In addition, oral bioavailability in children (45–66%) is only half of that in adults (96%) (Schulz et al., 2019), and this may suggest that primarily gut wall metabolism is also increased in children (Zane and Thakker, 2014). These PK discrepancies may be explained by developmental differences in organs, tissues, and enzymes (Kearns et al., 2003), resulting in an increased ratio of hepatic mass to total body mass and a higher clearance of CYP enzymes in children (Walsh et al., 2010).
The physiologically based pharmacokinetic (PBPK) model combines the knowledge of system-specific factors and drug-specific factors with mathematical modeling methods to quantitatively predict the PK characteristics of drug absorption, distribution, metabolism, and excretion (Maharaj and Edginton, 2014). Previously, an adult and pediatric PBPK model was established with hepatic in vitro data (Zane and Thakker, 2014). However, this model could not predict the nonlinear PKs of voriconazole and alterations in its metabolism over time. In another study, a whole-body PBPK model of voriconazole integrating the TDI of CYP3A4 and genetic polymorphisms of CYP2C19 was constructed (Li et al., 2020). It successfully captured the main PK characteristics of the drug in adults but overpredicted exposure to PMs after multiple doses.
Therefore, the objectives of the present study were to 1) establish an adult PBPK model of voriconazole, focusing on improving predictions of multiple-dose administration, especially in PMs; 2) extrapolate this model to children using age-related scaling methods; and 3) conduct simulations to facilitate the dose-optimization process. Due to the lack of data and high interpatient variability in the PK parameters observed following oral (p.o.) administration of voriconazole in children, the adult model was only extrapolated to intravenous (i.v.) administration in the pediatric model.
In this study, a PBPK model of voriconazole was developed and evaluated in the adult population, subsequently extrapolated to the pediatric population, and verified by comparing the simulated plasma exposure with the observed data. The final PBPK model was then used to simulate PK studies for pediatric dose optimization. The workflow of the model development is presented in Figure 1.
FIGURE 1. Adult and pediatric modeling workflow. PBPK model, physiologically based pharmacokinetic model; TDI, time-dependent inhibition; i.v., intravenously; p.o., orally; RM, rapid metabolizer; NM, normal metabolizer; IM, intermediate metabolizer; PM, poor metabolizer; Vmax, maximum velocity; kinact, maximum inactivation rate constant.
The modeling work was conducted in PK-Sim® (part of Open Systems Pharmacology (OSP) Suite version 8.0, www.open-systems-pharmacology.org). The published data were digitized by GetData Graph Digitizer version 2.26 (getdata-graph-digitizer.com).
PK profiles following i.v. and p.o. administration were searched in PubMed, Embase and the Cochrane Library using the terms “voriconazole” and “pharmacokinetic”. The clinical trials with dosage, concentration, and CYP2C19 genotype information in healthy adults were included.
A generic whole-body standard model for small molecules was selected in PK-Sim, and default system-dependent physiological parameters were implemented. With the assumption that CYP2C19, CYP3A4, and CYP2C9 are responsible for the metabolism of voriconazole, reverse transcription-polymerase chain reaction (RT-PCR) profiles were used to determine tissue expression distributions of these three enzymes, with hepatic reference concentrations of 0.76, 4.32, and 3.84 μmol/L, respectively, for CYP2C19 NMs (*1/*1). The abundance of ultrarapid metabolizers (UMs: *17/*17), rapid metabolizers (RMs: *1/*17), intermediate metabolizers (IMs: 1/*2, *1/*3, *2/*17), and PMs (2/*2, *2/*3, *3/*3) of CYP2C19 (Moriyama et al., 2018) was calculated using the scale factor estimated by Steere et al. (2015), and the values were 1.41, 1.36, 0.29(IMs with *1)/0.63(IMs with *17), and 0 μmol/L, respectively. If the genotype was not explicitly distinguished and mentioned, 0.46 was used for IMs. Due to the limited available data for UMs, the adult PBPK model was only built in CYP2C19 RMs, NMs, IMs and PMs.
Drug-specific parameters such as physicochemical properties and PK characteristics describing absorption, distribution, metabolism, and excretion were obtained from the literature, as shown in Table 1. Tissue-to-plasma partition coefficients were calculated using the Poulin and Theil method. Cellular permeabilities were predicted using the PK-Sim standard method. The enzymatic clearance process was quantified using Michaelis-Menten kinetics (Michaelis et al., 2011). The initial value of the Michaelis-Menten constant (Km) for CYP2C19, CYP3A4, and CYP2C9 was 3.5, 11, and 20 μmol/L, and the maximum velocity (Vmax) was set to 1.19, 2.3, and 0.0556 pmol/min−1/pmol, respectively (Hyland et al., 2003; Damle et al., 2011). As the ratio of AUC within the dosing interval after multiple doses to AUC from zero to infinity after a single dose (AUCτ(multipole dose)/AUCinf(single dose)) is larger than 2 (Purkins et al., 2003), the time-dependent inhibition of CYP3A4 was integrated into the model using Eq. 1.
dEcat/dt describes the turnover of the enzyme, where kdeg is turnover rate constant, E0 is the initial enzyme concentration, I is the inhibitor concentration, kinact is the maximum inactivation rate constant, and KI is the inhibition concentration when reaching 50% of kinact. The initial values of kinact and KI were set to 0.04 min−1 and 9.33 μmol/L, respectively, according to the results of an in vitro inactivity assay (Li et al., 2020). Other parameters related to TDI were set as software default values. The glomerular filtration rate (GFR) fraction was fixed to 1, as there was no evidence for reabsorption and tubular secretion. Initial formulation-related parameters were also obtained from the literature, with the dissolution time when 50% of the substance dissolved (DT,50) set to 30 min and dissolution shape parameter for Weibull function (shape factor for tablet) set to 1.29 (Li et al., 2020).
The PBPK model was first established based on the initial values indicated above, and the Vmax of CYP3A4 was optimized based on a single i.v. dose in CYP2C19 PMs, assuming that CYP3A4 contributes to almost 100% of the metabolism in this population. The propriety of CYP2C19-related parameters was then evaluated based on the observed PK parameters and plasma concentration profiles from a single i.v. dose in CYP2C19 RMs/NMs/IMs. In the next step, the specific intestinal permeability and formulation-related parameters, including DT,50 and shape factor for tablet, were inspected using data from studies following a single p.o. administration in RMs/NMs/IMs/PMs. If the fits were deemed inadequate, these three parameters were optimized based on p.o. data. Data from Scholz et al. (2009) was used for the above parameter optimization. The TDI-related parameter was optimized in the final step due to the lack of multiple i.v. clinical studies with genotype information. If the predicted PK parameters from multiple p.o. studies in PMs were within 2-fold of the observed values, the modeling process was complete. Otherwise, kinact for CYP3A4 was optimized and the iterations of the above optimization steps were repeated.
The PK simulation of 100 virtual people for each clinical study was carried out, corresponding to the subject demographics (age range, proportion of male/female, and dosing regimens). The predictive performance was evaluated by visually comparing predicted concentration-time data with the observed data from the literature for initial verification. Ninety percent population prediction intervals covering the observed plasma concentration-time datasets were considered as a visual criterion for good performance. Next, the quantitative assessment was conducted by calculating the mean fold error (MFE) of PK parameters such as the area under the plasma concentration-time curve (AUC) and maximum concentrations (Cmax), expressed as the ratio of predicted to observed mean values. The model was acceptable if it met the 0.5- to 2.0-fold limit, and a more stringent criterion was the 0.8- to 1.25-fold range.
PK profiles following i.v. administration were searched in PubMed, Embase and the Cochrane Library using the terms “voriconazole”, “pharmacokinetic”, and children-related items “infant”, “child”, “children”, “pediatric”, and “adolescent”. Studies with sufficient information for dosage, concentration, and CYP2C19 genotype were included.
Drug-specific parameters defined in the adult PK data were kept constant for the pediatric PBPK model.
Developmental changes in anatomical and physiological parameters such as weight, height, organ volumes, blood flows, organ composition, and plasma protein concentration in PK-Sim® are based on the population data from previous studies (NHANES III, 1997; ICRP, 2002). These algorithms were used to generate virtual pediatric populations. For the age-dependent hepatic clearance, default CYP2C19, CYP3A4 and CYP2C9 ontogeny information is described in the online PK-Sim Ontogeny Database Open Systems Pharmacology (2018). In summary, the activity of CYP2C19, CYP3A4 and CYP2C9 increases after birth and reaches the adult level over approximately 1, 4 and 1 year, respectively. The model using these default ontogeny profiles overpredicted the exposure in children; therefore, the ontogeny factors of CYP enzymes were estimated based on a recent meta-analysis using in vivo data (Upreti and Wahlstrom., 2016; Supplementary Figure S1). These data suggested that in childhood, CYP2C19, CYP3A4, and CYP2C9 exhibit maximal activities beyond the levels in adults.
The PBPK model performance in children was evaluated using the quantitative verification described in Adult Model Verification. As some pediatric clinical trials (Walsh et al., 2010) did not clearly show results according to the CYP2C19 genotype as in adults, these data were verified by adding up different genotype results in the model based on genotype ratios from the literature. The visual check was not conducted due to the lack of available plasma concentration-time curves with gene information.
A 100-patients simulation was generated for each subpopulation classified by age (2-6 and 6-12 years) and CYP2C19 genotype (NMs, IMs and PMs). The individual AUC24h (AUC during a 24-h period) was estimated.
The probability of reaching a ratio of free drug (unbound drug concentration) AUC24h to minimum inhibitory concentration greater than or equal to 25 (fAUC24h/MIC ≥ 25) was considered to be the PK/pharmacodynamic (PD) index (Wang et al., 2015). The fraction of unbound drug was set to 42% (US FDA, 2005), with the assumption that this value is similar between children and adults (Yanni et al., 2008). Voriconazole MIC distributions for Aspergillus (4 species) and Candida (14 species) infections were obtained from the European Committee on Antimicrobial Susceptibility Testing database (2020); (Supplementary Figure S2). The probability of target attainment (PTA) at each MIC and the cumulative fraction of response (CFR) for the overall MIC distribution for each species were calculated. CFR values of all species in one genus larger than or equal to 80% was considered an appropriate dosage regimen (Mangal et al., 2018).
Input parameters of voriconazole PBPK model were summarized in Table 1. A proper fit was achieved in the adult model, as shown in Figure 2, and most of the observed concentrations fell within the 5% and 95% concentration-time prediction intervals. All PK parameters were predicted to be within the 2-fold difference, with 78% of predicted/observed AUC ratios and 85% of Cmax ratios within the 1.25-fold difference (Table 2; Figure 3). The prediction of PMs following multiple doses was satisfactory.
FIGURE 2. Comparison of adult PBPK model predicted plasma concentration-time profiles (lines) vs. clinical observed data (symbols) in (A) RM, (B) NM, (C) IM and (D) PM. PBPK predictions are presented as mean simulated concentrations (black line) with their 5th to 95th percentiles (grey area). i.v., intravenously; p.o., orally; RM, rapid metabolizer; NM, normal metabolizer; IM, intermediate metabolizer; PM, poor metabolizer.
TABLE 2. Summary of voriconazole pharmacokinetic parameters in clinical studies of healthy adults and comparison with model predicted values.
FIGURE 3. The goodness of fit plot for the PBPK model. Predicted vs. observed AUC for adults (A) and children (C), Cmax for adults (B) and children (D). AUC, area under the concentration–time curve; Cmax, maximum concentration.
The PBPK model well captured the pharmacokinetic features in children after integrating in vivo ontogeny profiles. The PBPK simulation results were consistent with the observed plasma concentration-time profiles of different dosages after i.v. administration. All corresponding model-predicted concentrations fell within the 2-fold prediction error, with 73% of Cmax values falling within the 1.25-fold prediction error (Table 3; Figure 3).
TABLE 3. Summary of voriconazole pharmacokinetic parameters in pediatric clinical studies and comparison with model predicted values.
Twelve pediatric groups were constructed for dose design, and the results are shown in Table 4. IMs with *17 have a similar CYP2C19 abundance to NMs and the recommended dosage for NMs can be a reference for this subpopulation. Therefore, the following IM dosage recommendation is for IMs with *1. Figure 4 shows the contrast of the minimum PTA among different species in two fungal genera at each specific MIC between the following scenarios: one is administered the recommended maintenance dose from the current medication label (8 mg/kg, twice daily (BID)), and the other one is administered the recommended dose determined from the PBPK model. The results suggested that, for the BID dosing regimens, intravenous doses should be adjusted to 12 mg/kg for NMs, 8 mg/kg for IMs, and 5 mg/kg for PMs for 2–6-year-old children infected with Aspergillus spp. As children grow older, the recommended dose decreases, specifically 9 mg/kg for NMs, 6 mg/kg for IMs, and 4 mg/kg for PMs infected with Aspergillus spp. When the infectious fungal genus is Candida spp., approximately half of the above dosages are recommended to attain the appropriate drug exposure using fAUC24h/MIC as the indicator, due to notable differences in these two fungi in terms of susceptibility to voriconazole.
FIGURE 4. The minimum probability that targets pharmacokinetic/pharmacodynamic index of fAUC24h/MIC ≥ 25 among all species achieved at a specific MIC for each CYP2C19 phenotype at the recommended dosage from the medication label (8 mg/kg) and recommended dose from the PBPK model for (A) children aged 2–6 years, infected with Aspergillus spp.; (B) children aged 2–6 years, infected with Candida spp.; (C) children aged 6–12 years, infected with Aspergillus spp.; (D) children aged 6–12 years, infected with Candida spp. PTA, probability of target attainment; MIC, minimum inhibitory concentration; NM, normal metabolizer; IM, intermediate metabolizer; PM, poor metabolizer; all the dosages are for the BID dosing regimens.
The adult and pediatric PBPK model of voriconazole, which incorporated the TDI of CYP3A4, gene polymorphisms of CYP2C19, and developmental changes in physiology and metabolic enzymes, were able to describe the PKs in both populations. Subsequent simulations revealed that age, CYP2C19 genotype, and infectious fungal genera influence target PK/PD index attainment and should be considered in dose optimization.
Voriconazole is often for long-term use, from weeks to months, in the prophylaxis and treatment of IFI (Benitez and Carver, 2019). Therefore, it is essential to elucidate the time-dependent PK characteristics of this medication following multiple doses. To accomplish this goal, the strategy used for model building in this study is slightly different from that used in other studies. TDI-related parameters have always been optimized in the final step in multiple studies. However, the resulting change in these values can affect the previous predictions of single-dose administration. Generally, retrograde clearance can be implemented in the model first, and then sensitivity analysis is conducted to determine the optimal value of uncertain parameters (Wu et al., 2014). However, due to the possible inaccuracies of data digitized from the literature, which may influence the sensitivity analysis, this method was not used. Instead, a “bottom-up” and “top-down” combination strategy incorporating manual iterations was used to facilitate the model building process. The adult PBPK model results showed satisfactory predictive performance for multiple-dose administration in all CYP2C19 genotype populations.
There was an overprediction of exposure in children when default enzyme ontogeny profiles from in vitro experiments were utilized to extrapolate the adult model. Therefore, ontogeny factors based on a meta-analysis of in vivo CYP activity with age-related changes (Upreti and Wahlstrom, 2016) were implemented. In this meta-analysis, the maximal activity of CYP2C19, CYP3A4, and CYP2C9 in children exceeded the corresponding adult values. The higher capacity or expression of CYP enzymes in children was also corroborated from a previous in vitro experiment using human liver microsomes (Yanni et al., 2010). That experiment showed that the apparent Vmax for voriconazole conversion to the N-oxide metabolite was approximately 3-fold higher in children than in adults. After incorporating the new ontogeny profiles, the predictive performance of the PBPK model was improved. Moreover, the clearance was approximately 2–3-fold higher in children than in adults in the simulation, which is consistent with a previous study (Walsh et al., 2004).
The final extrapolated model presented the PK features of voriconazole in the pediatric population following i.v. administration, except that the exposure of several 6–12-year-old children in the study by Walsh et al. was slightly overpredicted. There may be several reasons. First, the predicted PK parameters were calculated from a cohort of children with a certain percentage of different CYP2C19 metabolic types described in the baseline demographic data. However, a few children discontinued treatment due to adverse events. The metabolic types of these children were not disclosed, which may result in differences between the predicted and observed values. Furthermore, although children who were receiving drugs known to interact strongly with voriconazole, such as terfenadine and pimozide, were excluded, concomitant medications that could potentially interact with voriconazole were permitted, which might have resulted in altered PKs that were not considered in the PBPK model.
The results of dose optimization seem to be reasonable. Most of the recommended dosages for NM and IM children aged 2–12 years infected with Aspergillus spp. are similar to the dosages adopted by the European Medicines Agency (EMA) for children aged 2–11 years, specifically a loading i.v. dose of 9 mg/kg BID on day 1, followed by a maintenance dose of 8 mg/kg BID (European Medicines Association, 2012). They are also close to the loading dose of 7 mg/kg BID recommended by the Infectious Disease Society of America (IDSA) (Pappas et al., 2009), except for 12 mg/kg, which is the recommended dosage for 2–6-year-old NMs. Although the tolerance of higher dosage up to 10 mg/kg in children has been confirmed (Sano et al., 2016), further validation of this regimen's exposure and safety is needed before implementing it in clinical practice. In other subpopulations, dose adjustment is proposed based on several factors. First, younger children may have a relatively higher enzyme expression or activity (Upreti and Wahlstrom., 2016), contributing to higher metabolism and lower concentration. Thus, higher recommended doses in younger children, especially <5 years old, seem warranted (Soler-Palacín et al., 2011). Second, as CYP2C19 is the major enzyme in the metabolism of voriconazole (Barbarino et al., 2018), lower doses should be administered to people with alleles associated with enzymatic loss-of-function (Moriyama et al., 2017). Third, invasive fungal infection classification should be considered a key factor for antifungal therapy (Wang et al., 2016). The susceptibility of Candida spp. to voriconazole is higher than that of Aspergillus spp.; therefore, a reduced dose of voriconazole should be sufficient for infections involving the former (Perez-Pitarch et al., 2019).
The recommended dosage in this study should be deemed a reference for the initial dosing regimen because the target CFR value represented the overall distribution of MIC in the population and the clinical data utilized for model validation were mean drug exposure. It cannot completely solve the problem of high PK variability and replace the importance of therapeutic drug monitoring (Gastine et al., 2017). Subsequent dose adjustments should be conducted based on the individual MIC, drug concentration and clinical response (Muto et al., 2015).
One limitation of this study is that the model was established based on some fundamental assumptions. For example, CYP2C19, CYP3A4, and CYP2C9 account for the entire metabolism of voriconazole, and the metabolic pathway is the same in both adults and children. A study of human liver microsomes provided evidence that flavin-containing monooxygenase 3 (FMO3) contributes to higher clearance in children than in adults (Yanni et al., 2010). However, this was not integrated into our model due to the small effect on the main metabolite of voriconazole observed in previous recombinant FMO3 enzyme experiments (Yanni et al., 2008). Moreover, the limited available data on RMs made it impossible to recommend dosage for this subpopulation in children. The established model and the recommended dosage for other subpopulations may also require further verification in carefully designed clinical trials.
In conclusion, the developed PBPK model of voriconazole provides a more accurate description of PK characteristics in adults following single and multiple i.v. and p.o. administrations, especially in PMs. It also predicts exposure in children following i.v. administration with good accuracy. Age, CYP2C19 genotype, and infectious fungal genera were found to significantly influence the attainment of the PK/PD target in the simulation and thus should be considered for clinical dose selection.
The original contributions presented in the study are included in the article/Supplementary Material, further inquiries can be directed to the corresponding author.
YZ and SZ contributed to the study conception and design. YZ, SZ, and CW contributed to PBPK model development and simulations. YZ and PZ drafted the manuscript.
The authors declare that the research was conducted in the absence of any commercial or financial relationships that could be construed as a potential conflict of interest.
The Supplementary Material for this article can be found online at: https://www.frontiersin.org/articles/10.3389/fphar.2021.636097/full#supplementary-material.
Barbarino, J. M., Owusu Obeng, A., Klein, T. E., and Altman, R. B. (2018). PharmGKB summary: voriconazole pathway, pharmacokinetics. Pharmacogenet Genomics 27 (5), 201–209. doi:10.1097/FPC.0000000000000276
Benitez, L. L., and Carver, P. L. (2019). Adverse effects associated with long-term administration of azole antifungal agents. Drugs 79 (8), 833–853. doi:10.1007/s40265-019-01127-8 |
Clancy, C. J., and Nguyen, M. H. (1998). In vitro efficacy and fungicidal activity of voriconazole against Aspergillus and Fusarium species. Eur. J. Clin. Microbiol. Infect. Dis. 17 (8), 573–575. doi:10.1007/BF01708622 |
Damle, B., Varma, M. V., and Wood, N. (2011). Pharmacokinetics of voriconazole administered concomitantly with fluconazole and population-based simulation for sequential use. Antimicrob. Agents Chemother. 55 (11), 5172–5177. doi:10.1128/AAC.00423-11 |
European Committee on Antimicrobial Susceptibility Testing. Available from: https://mic.eucast.org/Eucast2/SearchController/search.jsp?action=performSearch&BeginIndex=0&Micdif=mic&NumberIndex=50&Antib=152&Specium=-1. (Accessed October 27, 2020).
European Medicines Association (2012). Annex I: summary of product characteristics. Available from: http://www.ema.europa.eu/docs/en_GB/document_library/EPAR_-_Product_Information/human/000387/WC500049756.pdf (Accessed October 31, 2019).
Gastine, S., Lehrnbecher, T., Müller, C., Farowski, F., Bader, P., Ullmann-Moskovits, J., et al. (2017). Pharmacokinetic modelling of voriconazole to develop an alternative dosing regimen in children. Antimicrob. Agents Chemother. 62 (1), e01194–1217. doi:10.1128/AAC.01194-17 |
Hohmann, N., Kocheise, F., Carls, A., Burhenne, J., Weiss, J., Haefeli, W. E., et al. (2016). Dose-dependent bioavailability and CYP3A inhibition contribute to non-linear pharmacokinetics of voriconazole. Clin. Pharmacokinet. 55 (12), 1535–1545. doi:10.1007/s40262-016-0416-1 |
Hyland, R., Jones, B. C., and Smith, D. A. (2003). Identification of the cytochrome P450 enzymes involved in the N-oxidation of voriconazole. Drug Metab. Dispos 31 (5), 540–547. doi:10.1124/dmd.31.5.540 |
ICRP (2002). Basic anatomical and physiological data for use in radiological protection: reference values. A report of age- and gender-related differences in the anatomical and physiological characteristics of reference individuals. Ann. ICRP 32 (3–4), 5–265.
Kearns, G. L., Abdel-Rahman, S. M., Alander, S. W., Blowey, D. L., Leeder, J. S., and Kauffman, R. E. (2003). Developmental pharmacology--drug disposition, action, and therapy in infants and children. N. Engl. J. Med. 349 (12), 1157–1167. doi:10.1056/NEJMra035092.PMID:13679 |
Lee, S., Kim, B. H., Nam, W. S., Yoon, S. H., Cho, J. Y., Shin, S. G., et al. (2012). Effect of CYP2C19 polymorphism on the pharmacokinetics of voriconazole after single and multiple doses in healthy volunteers. J. Clin. Pharmacol. 52 (2), 195–203. doi:10.1177/0091270010395510 |
Lei, H. P., Wang, G., Wang, L. S., Ou-yang, D. S., Chen, H., Li, Q., et al. (2009). Lack of effect of Ginkgo biloba on voriconazole pharmacokinetics in Chinese volunteers identified as CYP2C19 poor and extensive metabolizers. Ann. Pharmacother. 43 (4), 726–731. doi:10.1345/aph.1L537 |
Levêque, D., Nivoix, Y., Jehl, F., and Herbrecht, R. (2006). Clinical pharmacokinetics of voriconazole. Int. J. Antimicrob. Agents 27 (4), 274–284. doi:10.1016/j.ijantimicag.2006.01.003 |
Li, X., Frechen, S., Moj, D., Lehr, T., Taubert, M., Hsin, C. H., et al. (2020). A physiologically based pharmacokinetic model of voriconazole integrating time-dependent inhibition of CYP3A4, genetic polymorphisms of CYP2C19 and predictions of drug-drug interactions. Clin. Pharmacokinet. 59 (6), 781–808. doi:10.1007/s40262-019-00856-z |
Maharaj, A. R., and Edginton, A. N. (2014). Physiologically based pharmacokinetic modeling and simulation in pediatric drug development. CPT Pharmacometrics Syst. Pharmacol. 3 (11), e150. doi:10.1038/psp.2014.45
Mangal, N., Hamadeh, I. S., Arwood, M. J., Cavallari, L. H., Samant, T. S., Klinker, K. P., et al. (2018). Optimization of voriconazole therapy for the treatment of invasive fungal infections in adults. Clin. Pharmacol. Ther. 104 (5), 957–965. doi:10.1002/cpt.1012 |
Michaelis, L., Menten, M. L., Johnson, K. A., and Goody, R. S. (2011). The original Michaelis constant: translation of the 1913 Michaelis-Menten paper. Biochemistry 50 (39), 8264–8269. doi:10.1021/bi201284u |
Mikus, G., Schöwel, V., Drzewinska, M., Rengelshausen, J., Ding, R., Riedel, K. D., et al. (2006). Potent cytochrome P450 2C19 genotype-related interaction between voriconazole and the cytochrome P450 3A4 inhibitor ritonavir. Clin. Pharmacol. Ther. 80 (2), 126–135. doi:10.1016/j.clpt.2006.04.004 |
Mori, M., Kobayashi, R., Kato, K., Maeda, N., Fukushima, K., Goto, H., et al. (2015). Pharmacokinetics and safety of voriconazole intravenous-to-oral switch regimens in immunocompromised Japanese pediatric patients. Antimicrob. Agents Chemother. 59 (2), 1004–1013. doi:10.1128/AAC.04093-14 |
Moriyama, B., Obeng, A. O., Barbarino, J., Penzak, S. R., Henning, S. A., Scott, S. A., et al. (2017). Clinical pharmacogenetics implementation consortium (CPIC) guidelines for CYP2C19 and voriconazole therapy. Clin. Pharmacol. Ther. 102 (1), 45–51. doi:10.1002/cpt.583 |
Murayama, N., Imai, N., Nakane, T., Shimizu, M., and Yamazaki, H. (2007). Roles of CYP3A4 and CYP2C19 in methyl hydroxylated and N-oxidized metabolite formation from voriconazole, a new anti-fungal agent, in human liver microsomes. Biochemical pharmacology. 73 (12), 2020–2026. doi:10.1016/j.bcp.2007.03.012
Muto, C., Shoji, S., Tomono, Y., and Liu, P. (2015). Population pharmacokinetic analysis of voriconazole from a pharmacokinetic study with immunocompromised Japanese pediatric subjects. Antimicrob. Agents Chemother. 59 (6), 3216–3223. doi:10.1128/AAC.04993-14 |
NHANES III (1997). Third national health and nutrition examination survey. Hyattsville, MD: National center for health statistics hyattsville.
Open Systems Pharmacology (2018). PK-Sim Ontogeny Database Version 7.3.pdf: Github; 2018 [Document describing the ontogeny functions used for different CYP enzymes]. Available from: https://github.com/Open-Systems-Pharmacology/OSPSuite.Documentation/blob/master/PK-Sim%20Ontogeny%20Database%20Version%207.3.pdf (Accessed Oct 31, 2018).
Pappas, P. G., Kauffman, C. A., Andes, D., Benjamin, D. K., Calandra, T. F., Edwards, J. E., et al. (2009). Clinical practice guidelines for the management of candidiasis: 2009 update by the Infectious Diseases Society of America. Clin. Infect. Dis. 48 (5), 503–535. doi:10.1086/596757 |
Perez-Pitarch, A., Guglieri-Lopez, B., Ferriols-Lisart, R., Pérez, A., Ezquer-Garín, C., Hernández-Boluda, J. C., et al. (2019). Pharmacokinetic/pharmacodynamic analysis of voriconazole against Candida spp. and Aspergillus spp. in allogeneic stem cell transplant recipients. Ther. Drug Monit. 41 (6), 740–747. doi:10.1097/FTD.0000000000000657 |
Perfect, J. R., Marr, K. A., Walsh, T. J., Greenberg, R. N., DuPont, B., de la Torre-Cisneros, J., et al. (2003). Voriconazole treatment for less-common, emerging, or refractory fungal infections. Clin. Infect. Dis. 36 (9), 1122–1131. doi:10.1086/374557 |
Purkins, L., Wood, N., Greenhalgh, K., Eve, M. D., Oliver, S. D., and Nichols, D. (2003). The pharmacokinetics and safety of intravenous voriconazole - a novel wide-spectrum antifungal agent. Br. J. Clin. Pharmacol. 56 (Suppl. 1), 2–9. doi:10.1046/j.1365-2125.2003.01992.x |
Sano, H., Kobayashi, R., Hori, D., Kishimoto, K., Suzuki, D., Yasuda, K., et al. (2016). Prophylactic administration of voriconazole with two different doses for invasive fungal infection in children and adolescents with acute myeloid leukemia. J. Microbiol. Immunol. Infect. 51 (2), 260–266. doi:10.1016/j.jmii.2016.05.002 |
Saravolatz, L. D., Johnson, L. B., and Kauffman, C. A. (2003). Voriconazole: a new triazole antifungal agent. Clin. Infect. Dis. 36 (5), 630–637. doi:10.1086/36793
Scholz, I., Oberwittler, H., Riedel, K. D., Burhenne, J., Weiss, J., Haefeli, W. E., et al. (2009). Pharmacokinetics, metabolism and bioavailability of the triazole antifungal agent voriconazole in relation to CYP2C19 genotype. Br. J. Clin. Pharmacol. 68 (6), 906–915. doi:10.1111/j.1365-2125.2009.03534.x |
Schulz, J., Kluwe, F., Mikus, G., Michelet, R., and Kloft, C. (2019). Novel insights into the complex pharmacokinetics of voriconazole: a review of its metabolism. Drug Metab. Rev. 51 (3), 247–265. doi:10.1080/03602532.2019.1632888 |
Soler-Palacín, P., Frick, M. A., Martín-Nalda, A., Lanaspa, M., Pou, L., Roselló, E., et al. (2011). Voriconazole drug monitoring in the management of invasive fungal infection in immunocompromised children: a prospective study. J. Antimicrob. Chemother. 67 (3), 700–706. doi:10.1093/jac/dkr517 |
Steere, B., Baker, J. A., Hall, S. D., and Guo, Y. (2015). Prediction of in vivo clearance and associated variability of CYP2C19 substrates by genotypes in populations utilizing a pharmacogenetics-based mechanistic model. Drug Metab. Dispos 43 (6), 870–883. doi:10.1124/dmd.114.061523 |
Upreti, V. V., and Wahlstrom, J. L. (2016). Meta-analysis of hepatic cytochrome P450 ontogeny to underwrite the prediction of pediatric pharmacokinetics using physiologically based pharmacokinetic modeling. J. Clin. Pharmacol. 56 (3), 266–283. doi:10.1002/jcph.585 |
US FDA (2005). Pfizer Label: voriconazole for injection, tablets, oral suspension: LAB-0271-12. Silver Spring, MD: US FDA.
Walsh, T. J., Driscoll, T., Milligan, P. A., Wood, N. D., Schlamm, H., Groll, A. H., et al. (2010). Pharmacokinetics, safety, and tolerability of voriconazole in immunocompromised children. Antimicrob. Agents Chemother. 54 (10), 4116–4123. doi:10.1128/AAC.00896-10 |
Walsh, T. J., Karlsson, M. O., Driscoll, T., Arguedas, A. G., Adamson, P., Saez-Llorens, X., et al. (2004). Pharmacokinetics and safety of intravenous voriconazole in children after single- or multiple-dose administration. Antimicrob. Agents Chemother. 48 (6), 2166–2172. doi:10.1128/AAC.48.6.2166-2172.2004 |
Wang, G., Lei, H. P., Li, Z., Tan, Z. R., Guo, D., Fan, L., et al. (2009). The CYP2C19 ultra-rapid metabolizer genotype influences the pharmacokinetics of voriconazole in healthy male volunteers. Eur. J. Clin. Pharmacol. 65 (3), 281–285. doi:10.1007/s00228-008-0574-7 |
Wang, T., Xie, J., Wang, Y., Zheng, X., Lei, J., Wang, X., et al. (2015). Pharmacokinetic and pharmacodynamic properties of oral voriconazole in patients with invasive fungal infections. Pharmacotherapy 35 (9), 797–804. doi:10.1002/phar.1631 |
Wang, Y., Wang, T., Xie, J., Yang, Q., Zheng, X., Dong, W., et al. (2016). Risk factors for voriconazole-associated hepatotoxicity in patients in the intensive care unit. Pharmacotherapy 36 (7), 757–765. doi:10.1002/phar.1779.Epub2016Jul5 |
Weiss, J., Ten Hoevel, M. M., Burhenne, J., Walter-Sack, I., Hoffmann, M. M., Rengelshausen, J., et al. (2009). CYP2C19 genotype is a major factor contributing to the highly variable pharmacokinetics of voriconazole. J. Clin. Pharmacol. 49 (2), 196–204. doi:10.1177/0091270008327537 |
Wu, F., Gaohua, L., Zhao, P., Jamei, M., Huang, S. M., Bashaw, E. D., et al. (2014). Predicting nonlinear pharmacokinetics of omeprazole enantiomers and racemic drug using physiologically based pharmacokinetic modeling and simulation: application to predict drug/genetic interactions. Pharm. Res. 31 (8), 1919–1929. doi:10.1007/s11095-013-1293-z |
Yanni, S. B., Annaert, P. P., Augustijns, P., Bridges, A., Gao, Y., Benjamin, D. K., et al. (2008). Role of flavin-containing monooxygenase in oxidative metabolism of voriconazole by human liver microsomes. Drug Metab. Dispos 36 (6), 1119–1125. doi:10.1124/dmd.107.019646 |
Yanni, S. B., Annaert, P. P., Augustijns, P., Ibrahim, J. G., Benjamin, D. K., and Thakker, D. R. (2010). In vitro hepatic metabolism explains higher clearance of voriconazole in children versus adults: role of CYP2C19 and flavin-containing monooxygenase 3. Drug Metab. Dispos 38 (1), 25–31. doi:10.1124/dmd.109.029769 |
Zane, N. R., and Thakker, D. R. (2014). A physiologically based pharmacokinetic model for voriconazole disposition predicts intestinal first-pass metabolism in children. Clin. Pharmacokinet. 53 (12), 1171–1182. doi:10.1007/s40262-014-0181-y |
Keywords: voriconazole, physiologically based pharmacokinetic model, children, gene, dose optimization
Citation: Zhang Y, Zhao S, Wang C, Zhou P and Zhai S (2021) Application of a Physiologically Based Pharmacokinetic Model to Characterize Time-dependent Metabolism of Voriconazole in Children and Support Dose Optimization. Front. Pharmacol. 12:636097. doi: 10.3389/fphar.2021.636097
Received: 30 November 2020; Accepted: 01 February 2021;
Published: 17 March 2021.
Edited by:
Wei Zhao, Shandong University, ChinaCopyright © 2021 Zhang, Zhao, Wang, Zhou and Zhai. This is an open-access article distributed under the terms of the Creative Commons Attribution License (CC BY). The use, distribution or reproduction in other forums is permitted, provided the original author(s) and the copyright owner(s) are credited and that the original publication in this journal is cited, in accordance with accepted academic practice. No use, distribution or reproduction is permitted which does not comply with these terms.
*Correspondence: Suodi Zhai, emhhaXN1b2RpQDE2My5jb20=
Disclaimer: All claims expressed in this article are solely those of the authors and do not necessarily represent those of their affiliated organizations, or those of the publisher, the editors and the reviewers. Any product that may be evaluated in this article or claim that may be made by its manufacturer is not guaranteed or endorsed by the publisher.
Research integrity at Frontiers
Learn more about the work of our research integrity team to safeguard the quality of each article we publish.