- 1Department of Biosciences and Bioengineering, Indian Institute of Technology Bombay, Mumbai, India
- 2Buck Institute for Research on Aging, Novato, CA, United States
- 3Department of Hematopathology, The University of Texas MD Anderson Cancer Center, Houston, TX, United States
- 4Department of Entomology & Plant Pathology, North Carolina State University, Raleigh, NC, United States
The multitude of multi-omics data generated cost-effectively using advanced high-throughput technologies has imposed challenging domain for research in Artificial Intelligence (AI). Data curation poses a significant challenge as different parameters, instruments, and sample preparations approaches are employed for generating these big data sets. AI could reduce the fuzziness and randomness in data handling and build a platform for the data ecosystem, and thus serve as the primary choice for data mining and big data analysis to make informed decisions. However, AI implication remains intricate for researchers/clinicians lacking specific training in computational tools and informatics. Cancer is a major cause of death worldwide, accounting for an estimated 9.6 million deaths in 2018. Certain cancers, such as pancreatic and gastric cancers, are detected only after they have reached their advanced stages with frequent relapses. Cancer is one of the most complex diseases affecting a range of organs with diverse disease progression mechanisms and the effectors ranging from gene-epigenetics to a wide array of metabolites. Hence a comprehensive study, including genomics, epi-genomics, transcriptomics, proteomics, and metabolomics, along with the medical/mass-spectrometry imaging, patient clinical history, treatments provided, genetics, and disease endemicity, is essential. Cancer Moonshot℠ Research Initiatives by NIH National Cancer Institute aims to collect as much information as possible from different regions of the world and make a cancer data repository. AI could play an immense role in (a) analysis of complex and heterogeneous data sets (multi-omics and/or inter-omics), (b) data integration to provide a holistic disease molecular mechanism, (c) identification of diagnostic and prognostic markers, and (d) monitor patient’s response to drugs/treatments and recovery. AI enables precision disease management well beyond the prevalent disease stratification patterns, such as differential expression and supervised classification. This review highlights critical advances and challenges in omics data analysis, dealing with data variability from lab-to-lab, and data integration. We also describe methods used in data mining and AI methods to obtain robust results for precision medicine from “big” data. In the future, AI could be expanded to achieve ground-breaking progress in disease management.
Introduction
Artificial intelligence (AI) is a branch of computer science with enhanced analytical or predictive capabilities to perform interdisciplinary tasks that otherwise require human intellect. AI has intensive problem-solving capabilities including prediction, data scalability, dimensionality, and integration, reasoning about their underlying phenomena and/or big data transformation into clinically actionable knowledge, based on the learning from model data sets. The learning capacity is maximized by improving the prediction task based on problem-specific measurements of performance. Particularly, machine learning (ML) and deep learning (DL)-based approaches were gaining recognition and emerged as key components in biomedical data analysis, driven by health care data availability and rapid progress of analytics techniques (Jiang et al., 2017; Saltz et al., 2018; Huang et al., 2020; Ibrahim et al., 2020). AI is currently used to automate the information extraction, summarize the electronic medical records or hand-written doctor notes, integrate health records, and store information in cloud scaling (big data storage) (Bedi et al., 2015; Chang et al., 2016; Miotto et al., 2016; Osborne et al., 2016; Garvin et al., 2018; Syrjala, 2018) AI has immense potentials to contribute significantly at every stage of cancer management ranging from reliable early detection, stratification, determination of infiltrative tumor margins during surgical treatment, response to drugs/therapy, tracking tumor evolution and potential acquired resistance to treatments over time, prediction of tumor aggressiveness, metastasis pattern, and recurrence (Bi et al., 2019).
Cancer is a major cause of death worldwide, accounting for an estimated 9.6 million deaths in 2018. Cancers can originate from various organs viz. lung, breast, kidney, represent phenotypic diversity like cell surface markers, molecular mutations (p53, PTEN, ER), demonstrate varied growth rate and apoptosis based on the cancer microenvironment and status of blood supply, and its aggressive nature. Also, cancer has a diverse disease progression mechanism and the effectors ranging from gene-epigenetics to a wide array of metabolites. Cancer/tumor being highly heterogeneous in terms of inter-tumor heterogeneity (cancers from different patients) and intra-tumor heterogeneity (within a single tumor) impose challenges for both detection, treatments, and recurrence. Medical decisions for cancer treatment should consider not only its variegated forms with the evolution of disease but also the individual patient’s condition and their ability to receive and respond to treatment. Certain cancers, such as pancreatic and gastric cancers, are detected only after they have reached their advanced stages with frequent relapses. Integration of “multi-omics” (genomics, epi-genomics, transcriptomics, proteomics, and metabolomics), and “non-omics” (medical/mass-spectrometry imaging, patient clinical history, treatments, and disease endemicity) data could help overcome the challenges in the accurate detection, characterization, and monitoring of cancers. AI could play an immense role in the analysis of complex and heterogeneous data sets, particularly from multi-omics and inter-omics approaches and data integration to provide a holistic disease molecular mechanism, identification of novel dynamic diagnostic and prognostic markers and enable precision cancer management, well beyond the prevalent disease stratification patterns such as differential expression, and supervised classification (Figure 1). Advanced computational analyses could also augment a global interpretation and automation of the cancer patient radiographs that most commonly relies upon visual evaluations and hence differ in disease assessments. Cancer Moonsho℠ Research Initiatives by NIH National Cancer Institute aims to collect as many omics and non-omics information as possible from different regions of the world to create a national ecosystem for sharing and analyzing cancer data (Cancer Moonshot - National Cancer Institute, 2016). The project will help develop human tumor atlas, predict response to standard treatments, optimize guidelines for systematic cancer prediction and treatments, and identify ways to overcome drug resistance to improve (i) current understanding of cancer, (ii) enable new strategies/technologies for cancer characterization, (iii) early detection of tumors/cancer, and (iv) extend therapies to more patients in a personalized manner (Cancer Moonshot - National Cancer Institute, 2016). The large multidimensional biological data sets (including individual variability in genes, function, and environment) generated, and/or compiled for the fulfillment of this cross-border project require advanced computational analysis, and AI certainly could be one of the key plays.
Recently AI is successfully applied to tumor image segmentation, identify, and quantify the rate and amount of mitosis (Romo-Bucheli et al., 2017), screening mutations (Coudray et al., 2018), auto-detect and classify benign nuclei from cancer cells (Sirinukunwattana et al., 2016; Xu et al., 2016), protein alignments and spatial localization (Saltz et al., 2018), predicting unknown metabolites, precision medicine matching trials (Korbar et al., 2017; Coudray et al., 2018), drug repurposing (Aliper et al., 2016), liquid biopsies and pharmacogenomics based cancer screening/monitoring and predicting the patient outcomes (Cohen et al., 2018; Low et al., 2018), drug discovery (Abadi et al., 2017; Yu P. et al., 2017) and so on. AI has outperformed pathologists and dermatologists in diagnosing metastatic breast cancer (Low et al., 2018) and melanoma (Bejnordi et al., 2017). Conversely, multi-omics data has immense potentials to identify the caveat in the current AI-based cancer diagnostics, stratification, mutant identification, treatment, and drug repurposing approaches, which could advance precision oncology research (Li et al., 2018). However, we have limited knowledge in the multi-omics and inter-omics data analysis and availability of algorithms (Buchhalter et al., 2014). This review highlights the current AI application in data integration, advancement, scope, and challenges in oncology research and clinical use. The reports mostly cover the articles published in the last two decades (2000–2020).
Implications of Artificial Intelligence in Cancer Multi-Omics
Advancements in multidimensional “omics” technologies ranging from next-generation sequencing to the mass spectrometry have led to a plethora of information. AI mediated data integration obtained from different “-omics” platforms such as genomics, epigenomics, transcriptomics, proteomics, and metabolomics enables the understanding of complex biological systems by describing nearly all biomolecules ranging from DNA to metabolites. Multi-omics researches have diverse applications in veterinary medicine (Li Q. et al., 2015), microbiology (Zhang et al., 2010), agriculture science (Van Emon, 2016), biofuel (Rai et al., 2016), and biomedical sciences (More et al., 2015; Hasin et al., 2017; Awasthi et al., 2018; Patel et al., 2019) including oncology (see Table 1).
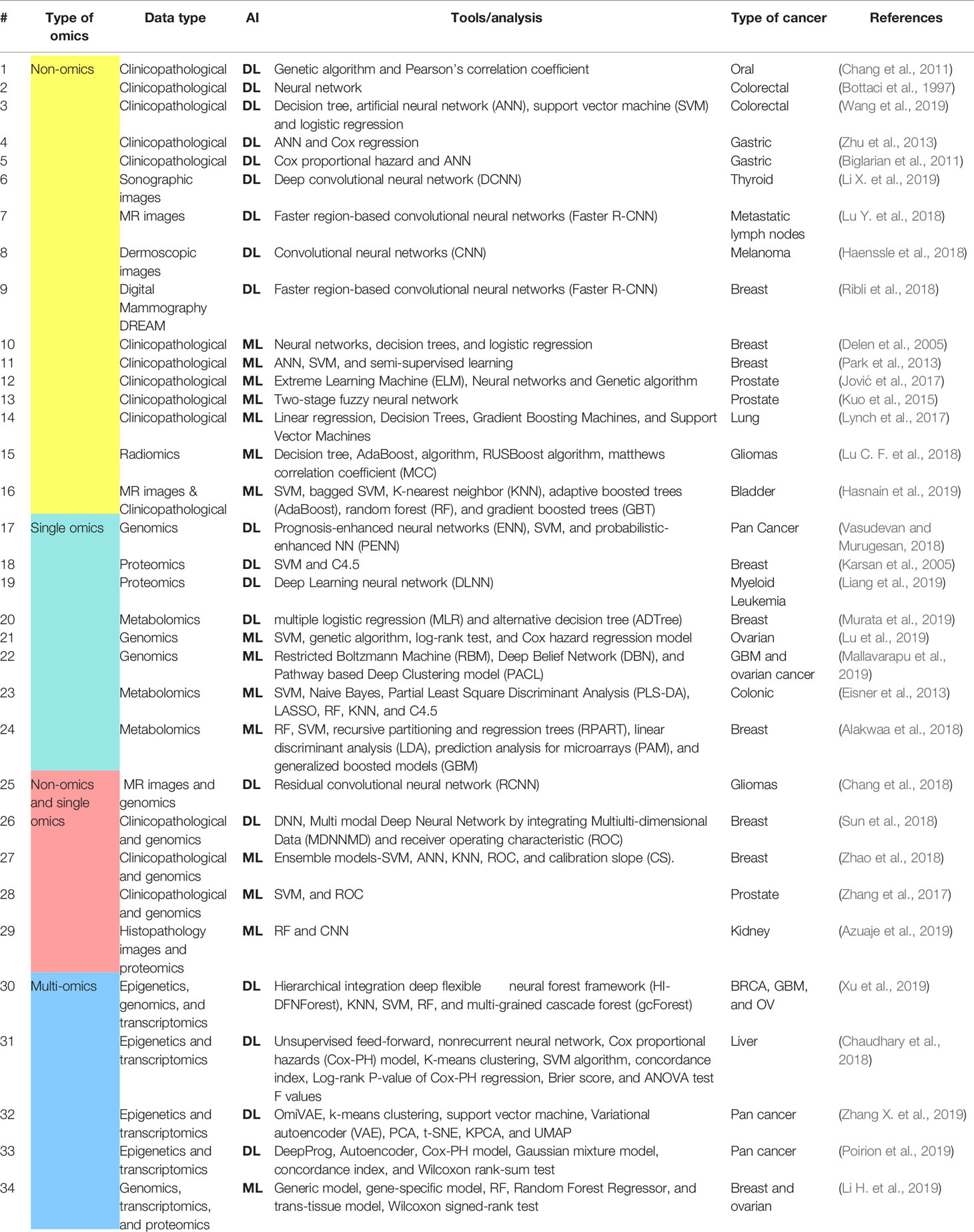
Table 1 Comprehensive list of Artificial Intelligence-based omics and non-omics investigations in oncology.
Genomics
Genomics data analysis relies on the nucleotide sequences, including expressed sequence tags (ESTs), cDNAs, and gene arrangements on the respective chromosomes. Rapid advances in the next‐generation sequencer (NGS) (Paolillo et al., 2016) and in silico computational algorithms have led to high-throughput data generation for whole genomes sequencing (WGS) and epigenomes. WGS comprehensively explores all types of genomic alterations in cancer and provides information on the repertoire of driver mutations and mutational signatures (including non‐coding regions) in cancer genomes, which remain widely unexplored. Ley et al. reported the first-ever WGS analysis of cancer (cytogenetically normal acute myeloid leukemia, AML) (Ley et al., 2008), merely 6 months post-publication of the first human whole-genome sequence (Wheeler et al., 2008). Since then several cancer genomics databases and projects including The Cancer Genome Atlas (TCGA) (Wang Z. et al., 2016), the International Cancer Genome Consortium (ICGC) (Zhang J. et al., 2019), Catalog of Somatic Mutations in Cancer (COSMIC) (Forbes et al., 2015), Cancer Genomic Hub (CGHub) (Wilks et al., 2014), Therapeutically Applicable Research to Generate Effective Treatments (TARGET) (Therapeutically Applicable Research to Generate Effective Treatments (TARGET)), cBioPortal (Gao et al., 2013), MethyCancer (He et al., 2008), UCSC Cancer Genomics Browser (Goldman et al., 2013) and moonshot project (Cancer Moonshot - National Cancer Institute, 2016) have surfaced (also see Table 2). Data accessibility has further led to the development of tools and resources to facilitate the rapid detection and analysis of biologically relevant genomic outcomes (Cerami et al., 2012; Gao et al., 2013; Gonzalez-Perez et al., 2013; Rubio-Perez et al., 2015; Chakraborty et al., 2018). WGS is thus a powerful tool to understand cancer genomics that typically contains unpredictable numbers of point mutations, fusions, and other aberrations. In contrast, targeted approaches like whole-exome sequencing (WES) are easier to analyze but miss out information of untranslated, intronic, and intergenic regions, which might have an impact on the molecular pathogenesis of cancer (Nik-Zainal et al., 2016). However, there are several associated limitations: (i) a vast majority of cancer genomics efforts remain focused around targeted approaches viz. WES (Morris et al., 2017) (ii) many of the genomics data reported lacks a comprehensive clinical annotation required for linking genomic events to specific cancer types, prognoses, and treatment responses (Robinson et al., 2017) (iii) most of the preliminary studies are performed on untreated cancers, and thus do not provide insight into the response to treatment regimens (Robinson et al., 2017). Integrating the cancer genomics data with clinical physiology data could, therefore, be expected to better define cancer biology and responses to treatments. Several studies have integrated genomics and non-omics cancer data (see Table 1). Histopathological images integration with genomics helps retrieves better information on cancer tissue architecture, which is generally compromised in molecular assays, rendering this rich information underused (López de Maturana et al., 2019). AI algorithms classify breast cancers using prognostic factors to quantitative image (Yuan et al., 2012) and the public data set (TCGA) (Yuan et al., 2012). AI algorithms to integrate (multi-) omics data with the pathology images has been successfully extended to develop predictive models for prostate cancer (Robinson et al., 2015), renal cell carcinoma (Schoof et al., 2019), low-grade glioma (Brat et al., 2015), and non-small cell lung cancer (Yu et al., 2016). Alongside integrating the multi-omics data from different platforms, transcriptomics, proteomics, and metabolomics with genomics could consolidate molecular information. Wu P. et al., 2019 integrated the Clinical Proteomic Tumor Analysis Consortium (CPTAC) mass spectrometry-based proteomics data for selected breast, colon, and ovarian tumors with TCGA into the cBioPortal (cBioPortal for Cancer Genomics) to support easy exploration and integrative analysis of the proteomic data sets in the context of the clinical and genomics data from the same tumors (Wu P. et al., 2019). Considering the diversity of cancer genomes and phenotypes, cataloging and interpretation of the abundant mutation, particularly non‐coding and structure variants, could be performed with confidence via integrating clinicopathological information along with transcriptomics, and epigenomics to decide the precise treatments that will produce the best results for the cancer patients.
Transcriptomics
Transcriptome denotes the active genes as well as long-noncoding RNA, short RNAs such as microRNAs, small nuclear RNAs in a defined physiological condition. The system-wide transcriptomic analysis evaluates overall transcripts in a metabolic process, while the targeted approach provides information regarding known genes. Differential expression of protein-coding RNA could provide insight into the disease mechanism, as well as integrated with genomics and proteomics to discover novel genes and their functional relevance. While non-coding RNAs have regulatory functions in several metabolic diseases, neurological disorders, and cancer. Transcriptome is directly co-related to any epigenomic change that manifests cancer, hence the integration of epigenomics and transcriptomics data could extend our understanding of cancer biology such studies are reported in breast (Robinson et al., 2015), prostate cancer (Varambally et al., 2002; Bhasin et al., 2015), head and neck squamous cell carcinoma (HNSCC) (Kelley et al., 2017). Also, the transcriptomics and epigenomics data integration approach opens-up avenues to know more about the promoter crosstalk through a shared enhancer (Eun et al., 2013) and dynamic switching of promoter and enhancer domains (Sohni et al., 2015). Moarii et al. used a large data set of 672 cancerous and healthy methylomes gene expression and copy number profiles from TCGA and performed a meta-analysis to clarify the interplay between promoter methylation and gene expression in normal and cancer samples (Moarii et al., 2015). Vantaku et al. demonstrated a novel approach for the unbiased integration of transcriptomics, metabolomics, lipidomics, and data to robustly predict high-grade patient survival and discovery of novel therapeutic targets in bladder cancer (Vantaku et al., 2019).
Proteomics
Proteomic profiles reveal cellular/molecular responses to (epi-) genomics, and environmental alterations, and their feedback responses. Post-translation modifications, including phosphorylation, glycosylation, ubiquitination, nitrosylation, enrich the protein repertoire (protein isoforms), and impacts protein functions like transport, enzymatic activity, and intracellular signaling pathways in cancer. Classifying specific protein isoforms provide unmatched clinical sensitivity and specificity. Various tissue and plasma proteomics studies are performed (Peng et al., 2018) to screen and diagnose cancers including colorectal (Tsai et al., 2012; Fayazfar et al., 2019; Thorsen et al., 2019), breast(Mishra et al., 2015), liver (Yang et al., 2013), oral (Lai et al., 2010) and so on. MS has applications beyond disease diagnostics and could be extended to monitor the feedback responses towards therapy, identify drug toxicity, and discovering new biomarkers. High-quality data sets are obligatory for clinical MS. Hence improvements in MS-instrument quality and robustness, automated sample processing, robust data analysis pipelines, and online automation (cloud computing) to synchronize results, data sets, and data portability have contributed to expanding the use and impact of MS in cancer research. Also, to deal with the variations in the proteomics data sets across the globe, Proteomics Standards Initiative (PSI) from the Human Proteome Organization (HUPO) has setup guidelines for sample collection viz. selecting appropriate disease controls, categorizing disease and sub-disease status (Maes et al., 2015), storage to rule-out pre-analytical variables (including patient and instrumental factors) that contribute to a large extent of variation, calibrating MS instrument for data-quality assurance, data reporting for untargeted (Martínez-Bartolomé et al., 2014) and targeted (Abbatiello et al., 2017) analysis. An amalgamation of proteomics data with (epi-)genomics, transcriptomics, metabolomics, and cancer histopathological images using AI gives confidence in the data or metabolic pathways identification. Proteomics investigation of breast cancer contoured more than 12,000 proteins and 33,000 phospho-sites. Proteogenomic analysis associated DNA mutations (data obtained from TCGA) to protein signaling to pinpoint the genetic drives of cancer, and revealed new signaling pathways for the breast cancer subtypes with specific mutations (PIK3CA and TP53) and identified two candidate markers (SKP1 and CETN3) in basal-like breast cancer (Mertins et al., 2016). Liu et al., integrated transcriptome (RNA-seq) and proteome (data-independent acquisition, DIA) data to co-relating RNA splicing links isoform expression with proteome diversity that may help for studying the perturbations associated with cancer (Liu et al., 2017). MS imaging (MSI) is yet another advancement in MS that enables visualization of tumor microenvironmental biochemistry and empowers tumor biology investigation to an entirely novel biochemical perspective, thereby potentially leading to the identification of a new pool of cancer biomarkers (Bi et al., 2019). High-throughput MSI analysis is a powerful tool for biomarker identification in a spatial manner, tracking drugs and its metabolites, imaging drug-response at cellular-level. MSI tool was used to identify unique region-of-interest–specific biomarkers (lipid signature) and therapeutic targets to classify colorectal cancer and subtyping in non-small cell lung cancer (Kriegsmann et al., 2016). MSI also finds application in the identification of prognostic signatures beyond classical histology. Proteins and protein isoforms associated with patient survival in four different high-grade sarcoma subtypes (Lou et al., 2017) and colorectal adenocarcinoma (Hinsch et al., 2017) were identified. In gastric adenocarcinoma, native glycan fragments detected by MALDI-FT-ICR mass spectrometry imaging were linked to patient prognosis (Kunzke et al., 2017). Combining MSI with histology enables the extraction of molecular profiles from specific regions of tissue or histopathological entities, implying MSI can facilitate intelligent knife (iKnife) in sorting tumors during surgery with high sensitivity and specificity (Balog et al., 2013). Certainly, MS-based analysis, along with histopathological diagnosis, can show a stronger association with the clinical outcome (Huber et al., 2014). Recently MSI data is combined with other imaging data like fluorescence in situ hybridization, tissue microarrays, confocal Raman spectroscopy, and MRI, for example, MRI and MSI imaging data were collated to analyze brain pathophysiology (Porta Siegel et al., 2018). Combing vasculature staining (using an anti-CD31 antibody) and MSI could help attain a better picture of vascularization as well as vessel characteristics. However, with emerging MS technologies, there are still challenges in its clinical application including nonoptimized raw data preprocessing, imprecise image co-registration, and limited pattern recognition capabilities due to lack of reference spectra database (Addie et al., 2015). Nevertheless, efforts/measures are taken towards the successful implementation of MS technology for diagnosis of cancer biomarkers translatable to clinical setting. Additionally, the imaging data could be integrated with LC/GC-MS, the workhorse technique of proteomics workflow that includes the extraction of total proteins/peptides, fractionation, and deep proteomic analysis. Delcourt et al., combined MSI and top-down microproteomics to detect potential protein markers in serous ovarian cancer (Delcourt et al., 2017). Using LC-MS and peptide fractionation Kulak et al. achieved deep coverage of cellular proteomes with sub-microgram sample input (Liebl, 1967). Further the cancer signature biomarkers could be used to stratify patients according to subtype, metastatic risk, progression, recurrence, and treatment response. Lately single-cell proteomics is gaining importance to bring comprehensive insights into the cancer heterogeneity, clonality to metastasis or to capture information from rare/mutated cells (Doerr, 2019). Using a quantitative single-cell proteomics approach Schoof et al., characterized an acute myeloid leukemia hierarchy (Schoof et al., 2019).
Metabolomics
Metabolomics is a systematic analysis of small molecules (<1kD) within cells, biofluids, tissues, or organisms involved in primary or secondary metabolic processes. Metabolites (small molecules) are highly diverse classified into multiple categories: amino acids, lipids, nucleotides, carbohydrates, and organic acids. Metabolite repertoire changes significantly during the process of normal growth and development and/or exposure to stress, allergens, and disease conditions (Bertini et al., 2009; Lin et al., 2011; Veselkov et al., 2011), which relates strongly to the final clinical phenotype. Metabolomics thus enhances our molecular understanding of disease mechanisms, progression, response to drugs/treatments, and recurrence probability. Typical metabolomics analysis workflow comprises of metabolite extractions, separation by liquid/gas chromatography, capillary electrophoresis and ion mobility, detection by mass spectrometry (MS), or nuclear magnetic resonance (NMR) spectroscopy and data analysis. MS applications in metabolomics have increased exponentially since the discovery and development of soft ionization tools like electrospray ionization (ESI) and matrix-assisted laser desorption ionization (MALDI). Several separation-free MS techniques including direct infusion-MS, MALDI-MS, mass spectrometry imaging (MSI), and direct analysis in real-time mass spectrometry are gaining popularity. The advantages of separation-free mass spectrometry are reduced sample volume requirements and minimization of the analytical variation. Untargeted metabolomics approaches are ideally used for hypothesis development, as it simultaneously identifies several unknown/known metabolites and quantifies. However, diverse physical and chemical properties and wide concentration ranges of the metabolites, biological variations(Heinemann et al., 2014), and identification of the unknown compounds based on the MS/MS fragmentation patterns impose challenges for untargeted metabolomics. For a long time, researchers have identified the unknows in the biological samples by complementing the MS/MS fragmentation with public repository or standards, which leads to the identification of a very limited number of metabolites, while a majority of the potentially useful information in MS/MS data sets remains uncurated. Molecular networking like GNPS has proved to be very useful in cataloging the uncurated MS/MS data sets via a spectral correlation and visualization approach that can detect sets of spectra from related molecules even when the spectra themselves are not matched to any known compounds (Wang M. et al., 2016). ML in combination with data mining algorithms (supervised and unsupervised) like principal component analysis or hierarchical clustering has transformed metabolomics studies like analyzing several variables/treatments simultaneously (Duan et al., 2005; Bertini et al., 2009; Guan et al., 2009). Particularly unsupervised data mining allows extracting meaningful relationships between samples with less risk of human bias. Metabolomics is applied in biomarker identification for diagnosis, monitoring, and prognosis of several diseases(Alvarez et al., 2017; Chorell et al., 2017; Perng et al., 2017; Patel et al., 2019), particularly those impacting metabolic functions, such as cancer. Metabolomic biomarkers for several cancers including colorectal (Ma et al., 2012; Nishiumi et al., 2012; Manna et al., 2014; Yamazaki, 2015) pancreatic (Zhang et al., 2012), lungs (Koutros et al., 2013; Li et al., 2014; Zhuang et al., 2016), breast (Cui et al., 2016; Li et al., 2020), gastric (Ikeda et al., 2012), ovarian (Zhang et al., 2013), and prostate (Koutros et al., 2013; Mondul et al., 2014; Kelly et al., 2016) have been reported. Despite numerous ongoing studies, limited metabolomics biomarkers reach clinical trials, implying improvements in experimental designs, data analysis with reduced false discovery rates, pinpointing molecules accountable for metabolic aberrations, and data interpretation is needed. Besides, we also must overcome interlaboratory variability by generalizing the protocols that are robust and adaptable to enhance reproducibility. Indeed, MS-based metabolomics biomarker discoveries have entered the new realms of MSI that present intuitive metabolites distribution in tissues or cells. MSI is performed in two modes, namely, imaging(Stoeckli et al., 2001) to correlate with histology and profiling, to know the overall metabolites (Cornett et al., 2006). MSI alone or in conjunction with (immuno-)histochemistry (IHC) enhances our understanding of complex heterogeneous cancer metabolic reprogramming with spatial information and facilitate the discovery of potential metabolic vulnerabilities that might be targeted for tumor therapy. MSI suffers some technical limitations like area of detection limits, instrument sensitivity at the high spatial resolution, ion suppression, matrix effects, and data analysis, particularly normalization and background correction, but has tremendous potential to improve cancer diagnostics. Huang et al. developed a graphical data processing pipeline for MSI based spatially resolved metabolomics (Huang et al., 2019), which could achieve multivariate statistical results in an intuitive and simple way as well as discovery low-abundant but reliable biomarkers in heterogeneous tumors. MSI has been employed to different cancers including brain (Jarmusch et al., 2016; Clark et al., 2018), breast (Guenther et al., 2015; Abdelmoula et al., 2016; Angerer et al., 2016; Wang S. et al., 2016; Torata et al., 2018; Vidavsky et al., 2019), lung (Calligaris et al., 2015; Li T. et al., 2015; Carter et al., 2017; Holzlechner et al., 2018), ovarian (Dória et al., 2016; Briggs et al., 2019), prostrate (Wang et al., 2017), esophageal (Guo et al., 2014; Abbassi-Ghadi et al., 2016; Sun et al., 2019a), colon (Hiraide et al., 2016; Inglese et al., 2017), oral (Uchiyama et al., 2014; Bednarczyk et al., 2019), skin (Xu et al., 2017; Margulis et al., 2018), adrenal gland (Sun et al., 2019b) and gastrointestinal stromal tumors (Abu Sammour et al., 2019) for spatial metabolomics analysis. MSI is also used to determine the metabolite changes in the 3D osteosarcoma cell culture model upon drug treatments (Palubeckaitė et al., 2020). MSI has been used to investigate tumor biopsy tissues for hypoxia (Chughtai et al., 2013; Jiang et al., 2015), driver of tumor resistance to radiotherapy or chemotherapy, and lipid distributions (Inglese et al., 2017; Paine et al., 2019). Esteva et al., employed deep convolutional neural networks (CNNs)-representing a diverse class of multi-layer artificial neural networks, pre-trained on millions of images representing more than 1000 generic image classes to automate the classification of skin cancers (Esteva et al., 2017). The same approach could be extrapolated for analyzing the images captured by MSI for better cancer management. Inglese et al. recently developed a new computational multimodal pipeline Spatial Correlation Image Analysis (SPACiAL) to integrate MSI molecular imaging data with multiplex IHC. The pipeline allows comprehensive analyses of metabolic heterogeneity, thereby increasing the efficiency and precision for spatially resolved analyses of specific cell types (Inglese et al., 2017).
Consideration and Challenges For Ai-Mediated Multi-Omics Data Integration
AI-mediated clinical cancer research has attained new heights for its unpreceded learning capabilities to process complex data. ML and deep learning (DL) are the subset of artificial intelligence that enables computers to learn with data without being explicitly programmed. AI analytical skills are primarily due to image recognition, computer vision, data integration, decision making, and natural language processing. AI could thus self-adapt, synchronize qualitative and quantitative information, and validate clinical results obtained from multiple platforms. However, AI applications in oncology research still is infancy and must overcome several challenges (Figure 2).
Data Integration: A Major Challenge in Precision Oncology
A major challenge in precision oncology is to integrate data generated from multiple types of omics to predict biomarkers or phenotypic outcomes (tumor/normal, early/late stage, survival, etc.). Machine learning tasks consist of three key steps in order to develop a computational model for biological data integration and analysis: (i) selection and pre-processing of data set, (ii) selection of algorithm and identify the ways to train it for development of a prediction model, and (iii) validation of the model in another data set (Figure 3).
Input Data Selection and Pre-Processing
Input data for most of the models consists of gene expression data, copy number alteration, epigenomics, proteomics, and single nucleotide mutations data sets. However, an integrated data analysis strategy combines various omics modalities, and this amalgamation of different types of data could help to develop promising prognostic models. Multi-platform data integration relies on (a) advances in sample extraction and processing technologies; (b) the availability of sufficiently large, matched, and carefully annotated data sets for multi-omics data; (c) molecular and physiologically characterized and graded tumor/cancer data set; (d) data sets with more informative images compared to present databases, e.g., the TCGA image collection (Yu K.-H. et al., 2017) for better 3D-fitting of in vivo imaging and ex vivo data. The first step in ML analysis is pre-processing of a defined data set(s). It requires normalization, noise filtration, and feature selection when more than one data sets are combined. Normalization becomes an essential step to eliminate biases during the analysis of different data sets that are merged. Selection of defined features is a critical phase in the success of an algorithm in classification, regression, and pattern recognition(Vougas et al., 2019).
Selection of Algorithm/Prediction Model and Data Integration
Algorithms are trained through optimizing the parameters to reach an ideal model. k-fold cross-validation (KF-CV) is widely used for optimizing without capturing the noise of data so that the results of statistical analysis can be generalized to an independent data set (Gao et al., 2019). Several studies on statistical methods and algorithms for data integration are reported (Huang et al., 2017; Perakakis et al., 2018; Zeng and Lumley, 2018; Wu C. et al., 2019). Standard machine learning techniques are supervised and unsupervised learning. Supervised learning requires algorithms to be provided with labeled inputs (e.g., omics data) and the desired output (e.g., the presence of a disease or not). In unsupervised learning, data are not labeled, and the algorithm is trained to look for naturally occurring pattern to correspond with the output. Another category that is more common in multi-omics studies is semi-supervised learning, where unlabelled data is used in conjunction with small labeled input. Briefly, multi-omics data integration consists of (a) dimension reduction: to reduce complexity, a number of factors are condensed to fewer variables (called components). (b) Clustering: Grouping input variables with common characteristics in same clusters, (c) density estimation to assess the distribution of input variables in specific space, and (d) regression to estimate the relationships among variables and for developing predictive models.
Testing the Prediction Models
Building a model that fits data beyond the current predictive model is the ultimate goal of training a candidate computational model. This can be tested by implementing a candidate predictive model to blind data sets. If the model is for developing tools to identify precision and personalized therapies for individual cancer patients, panels from clinical data sets should be preferentially used. A trained model that fails to generalize might be because of overfitting or underfitting (Dietterich and Bakiri, 1995). In the case of overfitting, noise, or random fluctuations are picked up in the training data, which negatively impact the model’s ability to generalize. Overfitting of a trained model is a major issue in machine learning. In underfitting, the underlying structure of a particular data set is not captured in a set of in silico pipeline. The predictive model’s capacity to make predictions understandable or interpretable to humans is another key requirement, i.e., the higher the complexity of the model, the more challenging interpretability becomes (black box models). This could be achieved at different levels of data processing and abstraction, however the development of methods for interpreting ML models is at a relatively early stage, particularly for precision oncology (Castelvecchi, 2016). Enhancing the interpretability will allow users to peer into the hidden layers of the model and determine how exactly the predictions are made on a case-to-case basis.
Deep Neural Networks: For Multi-Omics Data Integration
Deep neural networks (DNNs) are a subset of machine learning, which is gaining popularity in precision medicine. Today’s complex multi-omics data might be challenging to analyze with traditional machine learning algorithms. DNNs algorithms can integrate multi-omics data with better sensitivity, specificity, and efficiency. Moreover, DNNs have the advantage of integrating other sources of information such as medical images or clinical health records, which is a pre-requisite for personalized medicine. Sakellaropoulos et al. designed the DNNs model, which could capture pathways that linked gene expression with drug response and showed that DNNs are better than other traditional machine learning algorithms. Also, DNNs predicted drug response and survival in a large clinical cohort (Sakellaropoulos et al., 2019). Deep learning is still an emerging area in biomedical field, their effectiveness is not always guaranteed. Cancer multi-omics data integration is done using various approaches: unsupervised cancer subtyping to show patient survival (Ramazzotti et al., 2018), graph-based integration to integrate copy number aberration, epigenome, and transcriptome data sets for ovarian cancer clinical outcome prediction (Kim et al., 2015) and integration DNA methylation and matched imaging data to predict glioblastoma disease progression (Klughammer et al., 2018). However, rigorous mathematical foundations for emerging DNNs architectures are still lacking (Martorell-Marugán et al., 2019). One of the most challenging and futurist modules of the data-integration is combining multi-omics and non-omics data (imaging, biochemical/molecular profile data, clinical symptoms). Yu et al., associated omics data of lung cancer patients with the histopathology data to determine the patient survival rate (Yu K.-H. et al., 2017).
Machine Learning for Drug Response Prediction in Precision Oncology Applications
Identification of a panel of biomarkers that are associated with treatment responses is imperative for the precision oncology approach. Machine learning algorithms are being developed for prediction to drug response using response-predictive biomarkers through integrative analysis of multi-omics data (Ali and Aittokallio, 2019). Drug sensitivity prediction models, which are entirely based on gene expression profile, are less trustworthy compared to those which are based on integrated multi-omics profiling. Input data type, noise ratio, dimensionality, data complexity, and heterogeneity, are the crucial factors for drug response prediction model development. Sometimes, it is difficult to understand prediction models due to the dominance of gene expression profile data sets, which can be decreased by a two-stage method, called TANDEM (Aben et al., 2016). Bayesian efficient multiple kernel learning (BEMKL) is another drug response prediction model based on multi-omics data. It was the top-performing model in the National Cancer Institute - Dialogue for Reverse Engineering Assessment and Methods (NCI-DREAM7) Drug Sensitivity Prediction Challenge (Costello et al., 2014). Currently, the majority of data in repositories that are publicly available represent a significant set of data that are derived using cell lines treated with different doses of drugs and a large number of compounds. Some of these widely used data sets are: (i) The genomics of Drug Sensitivity in Cancer (GDSC), (ii) Cancer Cell Line Encyclopedia (CCLE), and (iii) National Cancer Institute drug screening panel (NCI-60). It is essential to understand that data extracted from clinical samples are ideal for the development of favorable drug prediction models. Heterogeneous properties of cancers make in silico analysis for molecular matching using cancer cell lines challenging in clinical settings (Hanahan and Weinberg, 2011; Turajlic et al., 2019). Importantly, the interplay of tumor-microenvironment that determines cancer development and response to drug treatment cannot be recapitulated using cancer cell lines model, and therefore, molecular changes associated with clinical cancers are diverse than in cancer cell lines (Wu and Dai, 2017). Lack of reliable resources for input data set stalled the success of creating drug prediction models. There is an urgent need to evaluate in silico technologies like transfer learning (TrLe) methods employing different ML algorithms and applications that utilize predictive feature (very complex non-linear relationships between features) learned in cell line trained model to build a new model or leverage information from auxiliary data not directly belonging to the problem being handled, that can be used in real clinical settings. Several studies have executed TrLe approach and tested and trained machine learning model for data obtained from clinical samples (Daemen et al., 2013; Turki et al., 2018). Turki et al. used TrLe-based approach to transfer patterns learned in breast and lung cancer patient data sets to predict drug sensitivity of multiple myeloma patients (Turki et al., 2018). Daemen et al. used breast cancer cell lines data for training model and tested on clinical data sets derived from TCGA (Daemen et al., 2013). Similarly, the Geeleher group built a training model on gene expression data sets extracted from Cancer Genomics Project and tested them on TCGA data sets from non-small-cell-lung cancers (NSCLC) (Geeleher et al., 2014). Using an elastic net model on to B-cell lymphoma cell lines, Falgreen et al. identified gene signatures that are associated with the development of resistance to drug (cyclophosphamide, doxorubicin, and vincristine) in diffuse large B-cell lymphoma (Falgreen et al., 2015). Sevakula et al. transfer learning for molecular cancer classification using DNN (Sevakula et al., 2019).
Machine Learning in Biomarker Discovery and Patient Classification
The identification of the disease biomarkers from -omics data does not only facilitate the stratification of patient cohorts but also provides early diagnostic information to improve patient management and prevent adverse outcomes. Coudray et al. applied CNN on whole-slide images obtained from The Cancer Genome Atlas to accurately and automatically classify subtypes of lung cancer, namely adenocarcinoma (LUAD) and squamous cell carcinoma (LUSC) and normal lung tissue (Coudray et al., 2018). Likewise, Huttunen et al., automated classification of multiphoton microscopy images of ovarian tissue (Huttunen et al., 2018). Further, they reported a prediction performance comparable to that obtained by pathologists. Brinker et al., automated dermoscopic melanoma image classification using CNN and showed its superiority over both junior and board-certified dermatologists (Brinker et al., 2019). Molecular profiling of carcinoma using circulating cell-free DNA is another approach for sub-dividing patients in risk factors (Kaseb et al., 2019). It has the advantage of being a noninvasive panel of biomarkers based on the multi-omics approach to increase the accuracy compared to biomarker-based on single omics data. For instance, protein biomarkers found in small sample sizes in the discovery cohort may be prone to achieve over-fitting and overinterpretation of proteomic data. Combined analysis of genomics with proteomics data sets led to the identification of novel therapeutic targets such as altered PI3K pathway in hormone receptor-positive breast cancer (Stemke-Hale et al., 2008). Transcriptomics with proteomics data sets analysis leads to the identification of gonadotropin-releasing hormone (GnRH) signaling pathway in glioblastoma that was not interpreted with single omics data set (Jayaram et al., 2016). Similarly, integrated analysis of DNA copy number alteration, with gene expression data in breast cancer patients led to understand the biology of cancer type and promoted to identify novel therapeutic interventions (Curtis et al., 2012). Four unique urinary biomarkers were identified in an integrated transcriptomic and metabolomics data analysis that was more reliable than single omics data analysis (Nam et al., 2009). Integrated proteogenomic characterization of paired tumor and adjacent liver samples identified alterations of the liver-specific proteome and metabolism. Biomarkers and patients’ subgroups with distinct features in metabolic reprogramming, microenvironment dysregulation, cell proliferation, and potential therapeutics were identified (Gao et al., 2019).
Concluding Remarks and Outlook
Cancer refers to a compendium of related diseases with uncontrolled dividing and spreading cells. More than 100s of different types of cancers are known. Cancer will be the leading cause of mortality in developed countries by 2030 (Centre for Disease Control). Cancer treatments are challenging due to its heterogenicity (temporal and spatial), high recurrence, and low median survival rate causing millions of deaths every. The molecular understanding of tumor biology has notably changed cancer treatment paradigms during the past 15 years. Still, the success of cancer therapeutics in clinical trials is the lowest of all major diseases. Future cancer treatments thus vouch for tailoring personalized therapies and targeting components of the tumor microenvironment. Accurate early diagnosis and prognosis of cancer greatly increases the chances for successful treatment and patient’s survival rate. Present cancer diagnosis relies on the clinician’s judgment based on their knowledge and clinical experience, which certainly cannot be guaranteed accurate diagnosis. This aspect points to the variability of the human brain to integrate large amounts of sample data. AI (ML and deep learning) is extremely proficient at handling vast amounts of complex nonlinear data (multi-omics and non-omics) generated during cancer treatments and researches, fault tolerance, parallel distributed processing, learning, and decision-making capabilities to improve oncologic care. AI could thus not only integrate various aspects of the clinical diversity but also helps to address the current lack of objectivity and universality in expert systems. Various researches showed impressive diagnostic and prognosis performance of AI using ML (Esteva et al., 2017; Ferroni et al., 2019; Jiang and Xu, 2019). Yoon et al. showed the potential of AI models for personalized oncology treatments that can estimate individualized treatment effects based on the analysis of counterfactual clinical outcomes (Yoon et al., 2018). ML algorithms (supervised or unsupervised learning) guided by clinicians could unravel the hidden molecular patterns within the data sets (multi-omics and non-omics) to support discovery of biomarkers (diagnostic, prognostic, recovery, and recurrence), candidate therapeutic targets associated with a specific patient group, and clinically relevant subtypes without explicit programming in clinical setups. Clinicians’ roles are inevitable in selecting the training data sets and multiple combinations of parameters necessary for building a classification ML model to address specific research questions. In turn, AI can help train junior physicians in clinical diagnosis and decision making. Expanding AI applications from pattern recognition capacity to dealing with multiple data modalities, insufficient data, evaluation of selective and predictive performance, guiding the learning process, and fine-tune models via feedback could revolutionize the cancer managements. Another step forward towards AI mediated clinical application is the development of ML pipelines that not only automate the design and evaluation of algorithms but also delineate the clinician the reasoning underlying the model predictions. This is a crucial step considering the fact although AI has learning potential but is in its infancy and cannot be left unattended. Yet another aspect is the extrapolation of the models generated using the cell line data to the patients, as the majority of the previous studies are performed on cell lines or limited small patient sample size, and the portability of the models generated in one cancer to another. AI has come long way but still it must achieve several landmarks: (a) non-reproducible results, (b) population heterogeneity, (c) instrument-variation, (d) lab-to-lab variation, (e) data normalization, (f) cross-compare results by different studies, (g) simulate results in vitro to clinics, (h) personalize, and (i) cost-effectiveness. Taken together, advancements in AI-based clinical cancer research will remarkably improve cancer prognosis and diagnosis with precision, resulting in enhanced prediction rates and patient survival.
Author Contributions
VR and SKP conceived the idea, wrote the manuscript, made table, and figures. BG contributed to writing.
Conflict of Interest
The authors declare that the research was conducted in the absence of any commercial or financial relationships that could be construed as a potential conflict of interest.
References
Abadi, S., Yan, W. X., Amar, D., Mayrose, I. (2017). A machine learning approach for predicting CRISPR-Cas9 cleavage efficiencies and patterns underlying its mechanism of action. PLoS Comput. Biol. 13, e1005807. doi: 10.1371/journal.pcbi.1005807
Abbassi-Ghadi, N., Golf, O., Kumar, S., Antonowicz, S., McKenzie, J. S., Huang, J., et al. (2016). Imaging of Esophageal Lymph Node Metastases by Desorption Electrospray Ionization Mass Spectrometry. Cancer Res. 76, 5647–5656. doi: 10.1158/0008-5472.CAN-16-0699
Abbatiello, S., Ackermann, B. L., Borchers, C., Bradshaw, R. A., Carr, S. A., Chalkley, R., et al. (2017). New Guidelines for Publication of Manuscripts Describing Development and Application of Targeted Mass Spectrometry Measurements of Peptides and Proteins. Mol. Cell. Proteomics 16, 327–328. doi: 10.1074/mcp.E117.067801
Abdelmoula, W. M., Balluff, B., Englert, S., Dijkstra, J., Reinders, M. J. T., Walch, A., et al. (2016). Data-driven identification of prognostic tumor subpopulations using spatially mapped t-SNE of Mass spectrometry imaging data. Proc. Natl. Acad. Sci. U. S. A. 113, 12244–12249. doi: 10.1073/pnas.1510227113
Aben, N., Vis, D. J., Michaut, M., Wessels, L. F. A. (2016). TANDEM: a two-stage approach to maximize interpretability of drug response models based on multiple molecular data types. Bioinformatics 32, i413–i420. doi: 10.1093/bioinformatics/btw449
Abu Sammour, D., Marsching, C., Geisel, A., Erich, K., Schulz, S., Ramallo Guevara, C., et al. (2019). Quantitative Mass Spectrometry Imaging Reveals Mutation Status-independent Lack of Imatinib in Liver Metastases of Gastrointestinal Stromal Tumors. Sci. Rep. 9, 10698. doi: 10.1038/s41598-019-47089-5
Addie, R. D., Balluff, B., Bovée, J. V. M. G., Morreau, H., McDonnell, L. A. (2015). Current State and Future Challenges of Mass Spectrometry Imaging for Clinical Research. Anal. Chem. 87, 6426–6433. doi: 10.1021/acs.analchem.5b00416
Alakwaa, F. M., Chaudhary, K., Garmire, L. X. (2018). Deep Learning Accurately Predicts Estrogen Receptor Status in Breast Cancer Metabolomics Data. J. Proteome Res. 17, 337–347. doi: 10.1021/acs.jproteome.7b00595
Ali, M., Aittokallio, T. (2019). Machine learning and feature selection for drug response prediction in precision oncology applications. Biophys. Rev. 11, 31–39. doi: 10.1007/s12551-018-0446-z
Aliper, A., Plis, S., Artemov, A., Ulloa, A., Mamoshina, P., Zhavoronkov, A. (2016). Deep learning applications for predicting pharmacological properties of drugs and drug repurposing using transcriptomic data. Mol. Pharm. 13, 2524–2530. doi: 10.1021/acs.molpharmaceut.6b00248
Alvarez, J. A., Chong, E. Y., Walker, D., II, Chandler, J. D., Michalski, E. S., Grossmann, R. E., et al. (2017). Plasma metabolomics in adults with cystic fibrosis during a pulmonary exacerbation: A pilot randomized study of high-dose vitamin D3 administration. Metabolism 70, 31–41. doi: 10.1016/j.metabol.2017.02.006
Angerer, T. B., Magnusson, Y., Landberg, G., Fletcher, J. S. (2016). Lipid heterogeneity resulting from fatty acid processing in the human breast cancer microenvironment identified by GCIB-ToFSIMS imaging. Anal. Chem. 88, 11946–11954. doi: 10.1021/acs.analchem.6b03884
Awasthi, G., Tyagi, S., Kumar, V., Patel, S. K., Rojh, D., Sakrappanavar, V., et al. (2018). A Proteogenomic Analysis of Haptoglobin in Malaria. Proteomics - Clin. Appl. 12, e1700077. doi: 10.1002/prca.201700077
Azuaje, F., Kim, S.-Y., Perez Hernandez, D., Dittmar, G. (2019). Connecting Histopathology Imaging and Proteomics in Kidney Cancer through Machine Learning. J. Clin. Med. 8:1535. doi: 10.3390/jcm8101535
Balog, J., Sasi-Szabó, L., Kinross, J., Lewis, M. R., Muirhead, L. J., Veselkov, K., et al. (2013). Intraoperative tissue identification using rapid evaporative ionization mass spectrometry. Sci. Transl. Med. 5, 194ra93. doi: 10.1126/scitranslmed.3005623
Bedi, G., Carrillo, F., Cecchi, G. A., Slezak, D. F., Sigman, M., Mota, N. B., et al. (2015). Automated analysis of free speech predicts psychosis onset in high-risk youths. NPJ Schizophr. 1, 1–7. doi: 10.1038/npjschz.2015.30
Bednarczyk, K., Gawin, M., Chekan, M., Kurczyk, A., Mrukwa, G., Pietrowska, M., et al. (2019). Discrimination of normal oral mucosa from oral cancer by mass spectrometry imaging of proteins and lipids. J. Mol. Histol. 50, 1–10. doi: 10.1007/s10735-018-9802-3
Bejnordi, B. E., Veta, M., Van Diest, P. J., Van Ginneken, B., Karssemeijer, N., Litjens, G., et al. (2017). Diagnostic assessment of deep learning algorithms for detection of lymph node metastases in women with breast cancer. JAMA - J. Am. Med. Assoc. 318, 2199–2210. doi: 10.1001/jama.2017.14585
Bertini, I., Calabró, A., De Carli, V., Luchinat, C., Nepi, S., Porfirio, B., et al. (2009). The metabonomic signature of celiac disease. J. Proteome Res. 8, 170–177. doi: 10.1021/pr800548z
Bhasin, J. M., Lee, B. H., Matkin, L., Taylor, M. G., Hu, B., Xu, Y., et al. (2015). Methylome-wide Sequencing Detects DNA Hypermethylation Distinguishing Indolent from Aggressive Prostate Cancer. Cell Rep. 13, 2135–2146. doi: 10.1016/j.celrep.2015.10.078
Bi, W. L., Hosny, A., Schabath, M. B., Giger, M. L., Birkbak, N. J., Mehrtash, A., et al. (2019). Artificial intelligence in cancer imaging: Clinical challenges and applications. CA. Cancer J. Clin 69, 127-157. doi: 10.3322/caac.21552
Biglarian, A., Hajizadeh, E., Kazemnejad, A., Zali, M. R. (2011). Application of artificial neural network in predicting the survival rate of gastric cancer patients. Iran. J. Public Health 40, 80–86.
Bottaci, L., Drew, P. J., Hartley, J. E., Hadfield, M. B., Farouk, R., Lee, P. W., et al. (1997). Artificial neural networks applied to outcome prediction for colorectal cancer patients in separate institutions. Lancet (Lond. Engl.) 350, 469–472. doi: 10.1016/S0140-6736(96)11196-X
Brat, D. J., Verhaak, R. G. W., Aldape, K. D., Yung, W. K. A., Salama, S. R., Cooper, L. A. D., et al. (2015). Comprehensive, integrative genomic analysis of diffuse lower-grade gliomas. N. Engl. J. Med. 372, 2481–2498. doi: 10.1056/NEJMoa1402121
Briggs, M. T., Condina, M. R., Ho, Y. Y., Everest-Dass, A. V., Mittal, P., Kaur, G., et al. (2019). MALDI Mass Spectrometry Imaging of Early- and Late-Stage Serous Ovarian Cancer Tissue Reveals Stage-Specific N-Glycans. Proteomics 19, e1800482. doi: 10.1002/pmic.201800482
Brinker, T. J., Hekler, A., Enk, A. H., Berking, C., Haferkamp, S., Hauschild, A., et al. (2019). Deep neural networks are superior to dermatologists in melanoma image classification. Eur. J. Cancer 119, 11–17. doi: 10.1016/j.ejca.2019.05.023
Buchhalter, I., Hutter, B., Alioto, T. S., Beck, T. A., Boutros, P. C., Brors, B., et al. (2014). A comprehensive multicenter comparison of whole genome sequencing pipelines using a uniform tumor-normal sample pair. Cold Spring Harbor Labs J. 013177. doi: 10.1101/013177
Calligaris, D., Feldman, D. R., Norton, I., Brastianos, P. K., Dunn, I. F., Santagata, S., et al. (2015). Molecular typing of meningiomas by desorption electrospray ionization mass spectrometry imaging for surgical decision-making. Int. J. Mass Spectrom. 377, 690–698. doi: 10.1016/j.ijms.2014.06.024
Cancer Moonshot - National Cancer Institute (2016). Natl. Cancer Inst. Available at: https://www.cancer.gov/research/key-initiatives/moonshot-cancer-initiative (Accessed April 19, 2020).
Carter, C. L., Jones, J. W., Farese, A. M., MacVittie, T. J., Kane, M. A. (2017). Lipidomic dysregulation within the lung parenchyma following whole-thorax lung irradiation: Markers of injury, inflammation and fibrosis detected by MALDI-MSI. Sci. Rep. 7, 10343. doi: 10.1038/s41598-017-10396-w
cBioPortal for Cancer Genomics. Available at: http://www.cbioportal.org/ (Accessed March 24, 2020).
Cerami, E., Gao, J., Dogrusoz, U., Gross, B. E., Sumer, S. O., Aksoy, B. A., et al. (2012). The cBio Cancer Genomics Portal: An open platform for exploring multidimensional cancer genomics data. Cancer Discov. 2, 401–404. doi: 10.1158/2159-8290.CD-12-0095
Chakraborty, S., Hosen, M., II, Ahmed, M., Shekhar, H. U. (2018). Onco-Multi-OMICS Approach: A New Frontier in Cancer Research. BioMed. Res. Int. 2018:9836256. doi: 10.1155/2018/9836256
Chang, S.-W., Kareem, S. A., Kallarakkal, T. G., Merican, A. F. M. A., Abraham, M. T., Zain, R. B. (2011). Feature selection methods for optimizing clinicopathologic input variables in oral cancer prognosis. Asian Pac. J. Cancer Prev. 12, 2659–2664.
Chang, E. K., Yu, C. Y., Clarke, R., Hackbarth, A., Sanders, T., Esrailian, E., et al. (2016). Defining a Patient Population With Cirrhosis. J. Clin. Gastroenterol. 50, 889–894. doi: 10.1097/MCG.0000000000000583
Chang, K., Bai, H. X., Zhou, H., Su, C., Bi, W. L., Agbodza, E., et al. (2018). Residual convolutional neural network for the determination of IDH status in low- and high-grade gliomas from mr imaging. Clin. Cancer Res. 24, 1073–1081. doi: 10.1158/1078-0432.CCR-17-2236
Chaudhary, K., Poirion, O. B., Lu, L., Garmire, L. X. (2018). Deep Learning-Based Multi-Omics Integration Robustly Predicts Survival in Liver Cancer. Clin. Cancer Res. 24, 1248–1259. doi: 10.1158/1078-0432.CCR-17-0853
Chorell, E., Hall, U. A., Gustavsson, C., Berntorp, K., Puhkala, J., Luoto, R., et al. (2017). Pregnancy to postpartum transition of serum metabolites in women with gestational diabetes. Metabolism 72, 27–36. doi: 10.1016/j.metabol.2016.12.018
Chughtai, K., Jiang, L., Greenwood, T. R., Glunde, K., Heeren, R. M. A. (2013). Mass spectrometry images acylcarnitines, phosphatidylcholines, and sphingomyelin in MDA-MB-231 breast tumor models. J. Lipid Res. 54, 333–344. doi: 10.1194/jlr.M027961
Clark, A. R., Calligaris, D., Regan, M. S., Pomeranz Krummel, D., Agar, J. N., Kallay, L., et al. (2018). Rapid discrimination of pediatric brain tumors by mass spectrometry imaging. J. Neurooncol. 140, 269–279. doi: 10.1007/s11060-018-2978-2
Cohen, J. D., Li, L., Wang, Y., Thoburn, C., Afsari, B., Danilova, L., et al. (2018). Detection and localization of surgically resectable cancers with a multi-analyte blood test. Sci. (80-. ). 359, 926–930. doi: 10.1126/science.aar3247
Cornett, D. S., Mobley, J. A., Dias, E. C., Andersson, M., Arteaga, C. L., Sanders, M. E., et al. (2006). A novel histology-directed strategy for MALDI-MS tissue profiling that improves throughput and cellular specificity in human breast cancer. Mol. Cell. Proteomics 5, 1975–1983. doi: 10.1074/mcp.M600119-MCP200
Costello, J. C., Heiser, L. M., Georgii, E., Gönen, M., Menden, M. P., Wang, N. J., et al. (2014). A community effort to assess and improve drug sensitivity prediction algorithms. Nat. Biotechnol. 32, 1202–1212. doi: 10.1038/nbt.2877
Coudray, N., Ocampo, P. S., Sakellaropoulos, T., Narula, N., Snuderl, M., Fenyö, D., et al. (2018). Classification and mutation prediction from non-small cell lung cancer histopathology images using deep learning. Nat. Med. 24, 1559–1567. doi: 10.1038/s41591-018-0177-5
Cui, M., Wang, Q., Chen, G. (2016). Serum metabolomics analysis reveals changes in signaling lipids in breast cancer patients. Biomed. Chromatogr. 30, 42–47. doi: 10.1002/bmc.3556
Curtis, C., Shah, S. P., Chin, S. F., Turashvili, G., Rueda, O. M., Dunning, M. J., et al. (2012). The genomic and transcriptomic architecture of 2,000 breast tumours reveals novel subgroups. Nature 486, 346–352. doi: 10.1038/nature10983
Daemen, A., Griffith, O. L., Heiser, L. M., Wang, N. J., Enache, O. M., Sanborn, Z., et al. (2013). Modeling precision treatment of breast cancer. Genome Biol. 14, R110. doi: 10.1186/gb-2013-14-10-r110
Delcourt, V., Franck, J., Leblanc, E., Narducci, F., Robin, Y. M., Gimeno, J. P., et al. (2017). Combined Mass Spectrometry Imaging and Top-down Microproteomics Reveals Evidence of a Hidden Proteome in Ovarian Cancer. EBioMedicine 21, 55–64. doi: 10.1016/j.ebiom.2017.06.001
Delen, D., Walker, G., Kadam, A. (2005). Predicting breast cancer survivability: A comparison of three data mining methods. Artif. Intell. Med. 34, 113–127. doi: 10.1016/j.artmed.2004.07.002
Dietterich, T. G., Bakiri, G. (1995). Solving Multiclass Learning Problems via Error-Correcting Output Codes (AI Access Foundation and Morgan Kaufmann Publishers).
Dória, M. L., McKenzie, J. S., Mroz, A., Phelps, D. L., Speller, A., Rosini, F., et al. (2016). Epithelial ovarian carcinoma diagnosis by desorption electrospray ionization mass spectrometry imaging. Sci. Rep. 6. doi: 10.1038/srep39219
Duan, K. B., Rajapakse, J. C., Wang, H., Azuaje, F. (2005). Multiple SVM-RFE for gene selection in cancer classification with expression data. IEEE Trans. Nanobiosci. 4, 228–233. doi: 10.1109/TNB.2005.853657
Eisner, R., Greiner, R., Tso, V., Wang, H., Fedorak, R. N. (2013). A Machine-Learned Predictor of Colonic Polyps Based on Urinary Metabolomics. BioMed. Res. Int. 2013, 11. doi: 10.1155/2013/303982
Esteva, A., Kuprel, B., Novoa, R. A., Ko, J., Swetter, S. M., Blau, H. M., et al. (2017). Dermatologist-level classification of skin cancer with deep neural networks. Nature 542, 115–118. doi: 10.1038/nature21056
Eun, B., Sampley, M. L., Good, A. L., Gebert, C. M., Pfeifer, K. (2013). Promoter cross-talk via a shared enhancer explains paternally biased expression of Nctc1 at the Igf2/H19/Nctc1 imprinted locus. Nucleic Acids Res. 41, 817–826. doi: 10.1093/nar/gks1182
Falgreen, S., Dybkær, K., Young, K. H., Xu-Monette, Z. Y., El-Galaly, T. C., Laursen, M. B., et al. (2015). Predicting response to multidrug regimens in cancer patients using cell line experiments and regularised regression models. BMC Cancer 15, 235. doi: 10.1186/s12885-015-1237-6
Fayazfar, S., Zali, H., Arefi Oskouie, A., Asadzadeh Aghdaei, H., Rezaei Tavirani, M., Nazemalhosseini Mojarad, E. (2019). Early diagnosis of colorectal cancer via plasma proteomic analysis of CRC and advanced adenomatous polyp. Gastroenterol. Hepatol. Bed Bench 12, 328–339.
Ferroni, P., Zanzotto, F. M., Riondino, S., Scarpato, N., Guadagni, F., Roselli, M. (2019). Breast cancer prognosis using a machine learning approach. Cancers (Basel). 11. doi: 10.3390/cancers11030328
Forbes, S. A., Beare, D., Gunasekaran, P., Leung, K., Bindal, N., Boutselakis, H., et al. (2015). COSMIC: exploring the world’s knowledge of somatic mutations in human cancer. Nucleic Acids Res. 43, D805–D811. doi: 10.1093/nar/gku1075
Gao, J., Aksoy, B. A., Dogrusoz, U., Dresdner, G., Gross, B., Sumer, S. O., et al. (2013). Integrative analysis of complex cancer genomics and clinical profiles using the cBioPortal. Sci. Signal. 6, pl1. doi: 10.1126/scisignal.2004088
Gao, Q., Zhu, H., Dong, L., Shi, W., Chen, R., Song, Z., et al. (2019). Integrated Proteogenomic Characterization of HBV-Related Hepatocellular Carcinoma. Cell 179, 561–577.e22. doi: 10.1016/j.cell.2019.08.052
Garvin, J. H., Kim, Y., Gobbel, G. T., Matheny, M. E., Redd, A., Bray, B. E., et al. (2018). Automating quality measures for heart failure using natural language processing:a descriptive study in the department of veterans affairs. J. Med. Internet Res. 20, e5. doi: 10.2196/medinform.9150
Geeleher, P., Cox, N. J., Huang, R. S. (2014). Clinical drug response can be predicted using baseline gene expression levels and in vitro drug sensitivity in cell lines. Genome Biol. 15, R47. doi: 10.1186/gb-2014-15-3-r47
Goldman, M., Craft, B., Swatloski, T., Ellrott, K., Cline, M., Diekhans, M., et al. (2013). The UCSC Cancer Genomics Browser: update 2013. Nucleic Acids Res. 41, D949–D954. doi: 10.1093/nar/gks1008
Gonzalez-Perez, A., Perez-Llamas, C., Deu-Pons, J., Tamborero, D., Schroeder, M. P., Jene-Sanz, A., et al. (2013). IntOGen-mutations identifies cancer drivers across tumor types. Nat. Methods 10, 1081–1082. doi: 10.1038/nmeth.2642
Guan, W., Zhou, M., Hampton, C. Y., Benigno, B. B., Walker, L. D. E., Gray, A., et al. (2009). Ovarian cancer detection from metabolomic liquid chromatography/mass spectrometry data by support vector machines. BMC Bioinf. 10:259. doi: 10.1186/1471-2105-10-259
Guenther, S., Muirhead, L. J., Speller, A. V. M., Golf, O., Strittmatter, N., Ramakrishnan, R., et al. (2015). Spatially resolved metabolic phenotyping of breast cancer by desorption electrospray ionization mass spectrometry. Cancer Res. 75, 1828–1837. doi: 10.1158/0008-5472.CAN-14-2258
Guo, S., Wang, Y., Zhou, D., Li, Z. (2014). Significantly increased monounsaturated lipids relative to polyunsaturated lipids in six types of cancer microenvironment are observed by mass spectrometry imaging. Sci. Rep. 4, 5959. doi: 10.1038/srep05959
Haenssle, H. A., Fink, C., Schneiderbauer, R., Toberer, F., Buhl, T., Blum, A., et al. (2018). Man against machine: diagnostic performance of a deep learning convolutional neural network for dermoscopic melanoma recognition in comparison to 58 dermatologists. Ann. Oncol. 29, 1836–1842. doi: 10.1093/annonc/mdy166
Hanahan, D., Weinberg, R. A. (2011). Hallmarks of cancer: The next generation. Cell 144, 646–674. doi: 10.1016/j.cell.2011.02.013
Hasin, Y., Seldin, M., Lusis, A. (2017). Multi-omics approaches to disease. Genome Biol. 18, 1–15. doi: 10.1186/s13059-017-1215-1
Hasnain, Z., Mason, J., Gill, K., Miranda, G., Gill, I. S., Kuhn, P., et al. (2019). Machine learning models for predicting post-cystectomy recurrence and survival in bladder cancer patients. PLoS One 14, e0210976. doi: 10.1371/journal.pone.0210976
He, X., Chang, S., Zhang, J., Zhao, Q., Xiang, H., Kusonmano, K., et al. (2008). MethyCancer: The database of human DNA methylation and cancer. Nucleic Acids Res. 36, D836–D841. doi: 10.1093/nar/gkm730
Heinemann, J., Mazurie, A., Tokmina-Lukaszewska, M., Beilman, G. J., Bothner, B. (2014). Application of support vector machines to metabolomics experiments with limited replicates. Metabolomics 10, 1121–1128. doi: 10.1007/s11306-014-0651-0
Hinsch, A., Buchholz, M., Odinga, S., Borkowski, C., Koop, C., Izbicki, J. R., et al. (2017). MALDI imaging mass spectrometry reveals multiple clinically relevant masses in colorectal cancer using large-scale tissue microarrays. J. Mass Spectrom. 52, 165–173. doi: 10.1002/jms.3916
Hiraide, T., Ikegami, K., Sakaguchi, T., Morita, Y., Hayasaka, T., Masaki, N., et al. (2016). Accumulation of arachidonic acid-containing phosphatidylinositol at the outer edge of colorectal cancer. Sci. Rep. 6, 29935. doi: 10.1038/srep29935
Holzlechner, M., Bonta, M., Lohninger, H., Limbeck, A., Marchetti-Deschmann, M. (2018). Multisensor Imaging-From Sample Preparation to Integrated Multimodal Interpretation of LA-ICPMS and MALDI MS Imaging Data. Anal. Chem. 90, 8831–8837. doi: 10.1021/acs.analchem.8b00816
Huang, S., Chaudhary, K., Garmire, L. X. (2017). More is better: Recent progress in multi-omics data integration methods. Front. Genet. 8, 84. doi: 10.3389/fgene.2017.00084
Huang, L., Mao, X., Sun, C., Luo, Z., Song, X., Li, X., et al. (2019). A graphical data processing pipeline for mass spectrometry imaging-based spatially resolved metabolomics on tumor heterogeneity. Anal. Chim. Acta 1077, 183–190. doi: 10.1016/j.aca.2019.05.068
Huang, S., Yang, J., Fong, S., Zhao, Q. (2020). Artificial intelligence in cancer diagnosis and prognosis: Opportunities and challenges. Cancer Lett. 471, 61–71. doi: 10.1016/j.canlet.2019.12.007
Huber, K., Feuchtinger, A., Borgmann, D. M., Li, Z., Aichler, M., Hauck, S. M., et al. (2014). Novel approach of MALDI drug imaging, immunohistochemistry, and digital image analysis for drug distribution studies in tissues. Anal. Chem. 86, 10568–10575. doi: 10.1021/ac502177y
Huttunen, M. J., Hassan, A., McCloskey, C. W., Fasih, S., Upham, J., Vanderhyden, B. C., et al. (2018). Automated classification of multiphoton microscopy images of ovarian tissue using deep learning. J. Biomed. Opt. 23, 1. doi: 10.1117/1.jbo.23.6.066002
Ibrahim, A., Gamble, P., Jaroensri, R., Abdelsamea, M. M., Mermel, C. H., Chen, P.-H. C., et al. (2020). Artificial intelligence in digital breast pathology: Techniques and applications. Breast 49, 267–273. doi: 10.1016/j.breast.2019.12.007
Ikeda, A., Nishiumi, S., Shinohara, M., Yoshie, T., Hatano, N., Okuno, T., et al. (2012). Serum metabolomics as a novel diagnostic approach for gastrointestinal cancer. Biomed. Chromatogr. 26, 548–558. doi: 10.1002/bmc.1671
Inglese, P., McKenzie, J. S., Mroz, A., Kinross, J., Veselkov, K., Holmes, E., et al. (2017). Deep learning and 3D-DESI imaging reveal the hidden metabolic heterogeneity of cancer††Electronic supplementary information (ESI) available. See DOI: 10.1039/c6sc03738kClick here for additional data file.Click here for additional data file.Click here for. Chem. Sci. 8, 3500–3511. doi: 10.1039/c6sc03738k
Jarmusch, A. K., Alfaro, C. M., Pirro, V., Hattab, E. M., Cohen-Gadol, A. A., Cooks, R. G. (2016). Differential Lipid Profiles of Normal Human Brain Matter and Gliomas by Positive and Negative Mode Desorption Electrospray Ionization - Mass Spectrometry Imaging. PLoS One 11, e0163180. doi: 10.1371/journal.pone.0163180
Jayaram, S., Gupta, M. K., Raju, R., Gautam, P., Sirdeshmukh, R. (2016). Multi-Omics Data Integration and Mapping of Altered Kinases to Pathways Reveal Gonadotropin Hormone Signaling in Glioblastoma. OMICS 20, 736–746. doi: 10.1089/omi.2016.0142
Jiang, N., Xu, X. (2019). Exploring the survival prognosis of lung adenocarcinoma based on the cancer genome atlas database using artificial neural network. Med. (Baltimore). 98, e15642. doi: 10.1097/MD.0000000000015642
Jiang, L., Chughtai, K., Purvine, S. O., Bhujwalla, Z. M., Raman, V., Paša-Tolić, L., et al. (2015). MALDI-Mass Spectrometric Imaging Revealing Hypoxia-Driven Lipids and Proteins in a Breast Tumor Model. Anal. Chem. 87, 5947–5956. doi: 10.1021/ac504503x
Jiang, F., Jiang, Y., Zhi, H., Dong, Y., Li, H., Ma, S., et al. (2017). Artificial intelligence in healthcare: past, present and future. Stroke Vasc. Neurol. 2, 230–243. doi: 10.1136/svn-2017-000101
Jović, S., Miljković, M., Ivanović, M., Šaranović, M., Arsić, M. (2017). Prostate Cancer Probability Prediction By Machine Learning Technique. Cancer Invest. 35, 647–651. doi: 10.1080/07357907.2017.1406496
Karsan, A., Eigl, B. J., Flibotte, S., Gelmon, K., Switzer, P., Hassell, P., et al. (2005). Analytical and preanalytical biases in serum proteomic pattern analysis for breast cancer diagnosis. Clin. Chem. 51, 1525–1528. doi: 10.1373/clinchem.2005.050708
Kaseb, A. O., Sánchez, N. S., Sen, S., Kelley, R. K., Tan, B., Bocobo, A. G., et al. (2019). Molecular Profiling of Hepatocellular Carcinoma Using Circulating Cell-Free DNA. Clin. Cancer Res. 25, 6107–6118. doi: 10.1158/1078-0432.CCR1-18-3341
Kelley, D. Z., Flam, E. L., Izumchenko, E., Danilova, L. V., Wulf, H. A., Guo, T., et al. (2017). Integrated analysis of whole-genome ChIP-Seq and RNA-Seq data of primary head and neck tumor samples associates HPV integration sites with open chromatin marks. Cancer Res. 77, 6538–6550. doi: 10.1158/0008-5472.CAN-17-0833
Kelly, R. S., Heiden, M. G. V., Giovannucci, E., Mucci, L. A. (2016). Metabolomic biomarkers of prostate cancer: Prediction, diagnosis, progression, prognosis, and recurrence. Cancer Epidemiol. Biomarkers Prev. 25, 887–906. doi: 10.1158/1055-9965.EPI-15-1223
Kim, D., Joung, J.-G., Sohn, K.-A., Shin, H., Park, Y. R., Ritchie, M. D., et al. (2015). Knowledge boosting: a graph-based integration approach with multi-omics data and genomic knowledge for cancer clinical outcome prediction. J. Am. Med. Inform. Assoc. 22, 109–120. doi: 10.1136/amiajnl-2013-002481
Klughammer, J., Kiesel, B., Roetzer, T., Fortelny, N., Nemc, A., Nenning, K.-H., et al. (2018). The DNA methylation landscape of glioblastoma disease progression shows extensive heterogeneity in time and space. Nat. Med. 24, 1611–1624. doi: 10.1038/s41591-018-0156-x
Korbar, B., Olofson, A., Miraflor, A., Nicka, C., Suriawinata, M., Torresani, L., et al. (2017). Deep learning for classification of colorectal polyps on whole-slide images. J. Pathol. Inform. 8, 30. doi: 10.4103/jpi.jpi_34_17
Koutros, S., Meyer, T. E., Fox, S. D., Issaq, H. J., Veenstra, T. D., Huang, W.-Y., et al. (2013). Prospective evaluation of serum sarcosine and risk of prostate cancer in the Prostate, Lung, Colorectal and Ovarian Cancer Screening Trial. Carcinogenesis 34, 2281–2285. doi: 10.1093/carcin/bgt176
Kriegsmann, M., Casadonte, R., Kriegsmann, J., Dienemann, H., Schirmacher, P., Hendrik Kobarg, J., et al. (2016). Reliable Entity Subtyping in Non-small Cell Lung Cancer by Matrix-assisted Laser Desorption/Ionization Imaging Mass Spectrometry on Formalin-fixed Paraffin-embedded Tissue Specimens. Mol. Cell. Proteomics 15, 3081–3089. doi: 10.1074/mcp.M115.057513
Kunzke, T., Balluff, B., Feuchtinger, A., Buck, A., Langer, R., Luber, B., et al. (2017). Native glycan fragments detected by MALDI-FT-ICR mass spectrometry imaging impact gastric cancer biology and patient outcome. Oncotarget 8, 68012–68025. doi: 10.18632/oncotarget.19137
Kuo, R.-J., Huang, M.-H., Cheng, W.-C., Lin, C.-C., Wu, Y.-H. (2015). Application of a two-stage fuzzy neural network to a prostate cancer prognosis system. Artif. Intell. Med. 63, 119–133. doi: 10.1016/j.artmed.2014.12.008
Lai, C.-H., Chang, N.-W., Lin, C.-F., Lin, C.-D., Lin, Y.-J., Wan, L., et al. (2010). Proteomics-based identification of haptoglobin as a novel plasma biomarker in oral squamous cell carcinoma. Clin. Chim. Acta 411, 984–991. doi: 10.1016/j.cca.2010.03.028
Ley, T. J., Mardis, E. R., Ding, L., Fulton, B., McLellan, M. D., Chen, K., et al. (2008). DNA sequencing of a cytogenetically normal acute myeloid leukaemia genome. Nature 456, 66–72. doi: 10.1038/nature07485
Li, Y., Song, X., Zhao, X., Zou, L., Xu, G. (2014). Serum metabolic profiling study of lung cancer using ultra high performance liquid chromatography/quadrupole time-of-flight mass spectrometry. J. Chromatogr. B Anal. Technol. Biomed. Life Sci. 966, 147–153. doi: 10.1016/j.jchromb.2014.04.047
Li, Q., Freeman, L. M., Rush, J. E., Huggins, G. S., Kennedy, A. D., Labuda, J. A., et al. (2015). Veterinary Medicine and Multi-Omics Research for Future Nutrition Targets: Metabolomics and Transcriptomics of the Common Degenerative Mitral Valve Disease in Dogs. OMICS 19, 461–470. doi: 10.1089/omi.2015.0057
Li, T., He, J., Mao, X., Bi, Y., Luo, Z., Guo, C., et al. (2015). In situ biomarker discovery and label-free molecular histopathological diagnosis of lung cancer by ambient mass spectrometry imaging. Sci. Rep. 5. doi: 10.1038/srep14089
Li, Y., Wu, F. X., Ngom, A. (2018). A review on machine learning principles for multi-view biological data integration. Brief. Bioinform. 19, 325–340. doi: 10.1093/bib/bbw113
Li, H., Siddiqui, O., Zhang, H., Guan, Y. (2019). Joint learning improves protein abundance prediction in cancers. BMC Biol. 17, 107. doi: 10.1186/s12915-019-0730-9
Li, X., Zhang, S., Zhang, Q., Wei, X., Pan, Y., Zhao, J., et al. (2019). Diagnosis of thyroid cancer using deep convolutional neural network models applied to sonographic images: a retrospective, multicohort, diagnostic study. Lancet Oncol. 20, 193–201. doi: 10.1016/S1470-2045(18)30762-9
Li, L., Zheng, X., Zhou, Q., Villanueva, N., Nian, W., Liu, X., et al. (2020). Metabolomics-Based Discovery of Molecular Signatures for Triple Negative Breast Cancer in Asian Female Population. Sci. Rep. 10, 370. doi: 10.1038/s41598-019-57068-5
Liang, C. A., Chen, L., Wahed, A., Nguyen, A. N. D. (2019). Proteomics analysis of FLT3-ITD mutation in acute myeloid leukemia using deep learning neural network. Ann. Clin. Lab. Sci. 49, 119–126. doi: 10.1093/ajcp/aqx121.148
Liebl, H. (1967). Ion microprobe mass analyzer. J. Appl. Phys. 38, 5277–5283. doi: 10.1063/1.1709314
Lin, X., Wang, Q., Yin, P., Tang, L., Tan, Y., Li, H., et al. (2011). A method for handling metabonomics data from liquid chromatography/mass spectrometry: Combinational use of support vector machine recursive feature elimination, genetic algorithm and random forest for feature selection. Metabolomics 7, 549–558. doi: 10.1007/s11306-011-0274-7
Liu, Y., Gonzàlez-Porta, M., Santos, S., Brazma, A., Marioni, J. C., Aebersold, R., et al. (2017). Impact of Alternative Splicing on the Human Proteome. Cell Rep. 20, 1229–1241. doi: 10.1016/j.celrep.2017.07.025
López de Maturana, E., Alonso, L., Alarcón, P., Martín-Antoniano, I. A., Pineda, S., Piorno, L., et al. (2019). Challenges in the Integration of Omics and Non-Omics Data. Genes (Basel). 10. doi: 10.3390/genes10030238
Lou, S., Balluff, B., Cleven, A. H. G., Bovée, J. V. M. G., McDonnell, L. A. (2017). Prognostic Metabolite Biomarkers for Soft Tissue Sarcomas Discovered by Mass Spectrometry Imaging. J. Am. Soc Mass Spectrom. 28, 376–383. doi: 10.1007/s13361-016-1544-4
Low, S.-K., Zembutsu, H., Nakamura, Y. (2018). Breast cancer: The translation of big genomic data to cancer precision medicine. Cancer Sci. 109, 497–506. doi: 10.1111/cas.13463
Lu, C. F., Hsu, F. T., Hsieh, K. L. C., Kao, Y. C. J., Cheng, S. J., Hsu, J. B. K., et al. (2018). Machine learning–based radiomics for molecular subtyping of gliomas. Clin. Cancer Res. 24, 4429–4436. doi: 10.1158/1078-0432.CCR-17-3445
Lu, Y., Yu, Q., Gao, Y., Zhou, Y., Liu, G., Dong, Q., et al. (2018). Identification of metastatic lymph nodes in MR imaging with faster region-based convolutional neural networks. Cancer Res. 78, 5135–5143. doi: 10.1158/0008-5472.CAN-18-0494
Lu, T.-P., Kuo, K.-T., Chen, C.-H., Chang, M.-C., Lin, H.-P., Hu, Y.-H., et al. (2019). Developing a Prognostic Gene Panel of Epithelial Ovarian Cancer Patients by a Machine Learning Model. Cancers (Basel). 11. doi: 10.3390/cancers11020270
Lynch, C. M., Abdollahi, B., Fuqua, J. D., de Carlo, A. R., Bartholomai, J. A., Balgemann, R. N., et al. (2017). Prediction of lung cancer patient survival via supervised machine learning classification techniques. Int. J. Med. Inform. 108, 1–8. doi: 10.1016/j.ijmedinf.2017.09.013
Ma, Y., Zhang, P., Wang, F., Liu, W., Yang, J., Qin, H. (2012). An integrated proteomics and metabolomics approach for defining oncofetal biomarkers in the colorectal cancer. Ann. Surg. 255, 720–730. doi: 10.1097/SLA.0b013e31824a9a8b
Maes, E., Cho, W. C., Baggerman, G. (2015). Translating clinical proteomics: the importance of study design. Expert Rev. Proteomics 12, 217–219. doi: 10.1586/14789450.2015.1041512
Mallavarapu, T., Hao, J., Kim, Y., Oh, J. H., Kang, M. (2019). Pathway-based deep clustering for molecular subtyping of cancer. Methods 173, 24–31. doi: 10.1016/j.ymeth.2019.06.017
Manna, S. K., Tanaka, N., Krausz, K. W., Haznadar, M., Xue, X., Matsubara, T., et al. (2014). Biomarkers of coordinate metabolic reprogramming in colorectal tumors in mice and humans. Gastroenterology 146, 1313–1324. doi: 10.1053/j.gastro.2014.01.017
Margulis, K., Chiou, A. S., Aasi, S. Z., Tibshirani, R. J., Tang, J. Y., Zare, R. N. (2018). Distinguishing malignant from benign microscopic skin lesions using desorption electrospray ionization mass spectrometry imaging. Proc. Natl. Acad. Sci. U. S. A. 115, 6347–6352. doi: 10.1073/pnas.1803733115
Martínez-Bartolomé, S., Binz, P.-A., Albar, J. P. (2014). The Minimal Information about a Proteomics Experiment (MIAPE) from the Proteomics Standards Initiative. Methods Mol. Biol. 1072, 765–780. doi: 10.1007/978-1-62703-631-3_53
Martorell-Marugán, J., Tabik, S., Benhammou, Y., del Val, C., Zwir, I., Herrera, F., et al. (2019). "Deep Learning in Omics Data Analysis and Precision Medicine," in Computational Biology. Ed. Husi, H. (Brisbane (AU): Codon Publications).
Mertins, P., Mani, D. R., Ruggles, K. V., Gillette, M. A., Clauser, K. R., Wang, P., et al. (2016). Proteogenomics connects somatic mutations to signalling in breast cancer. Nature 534, 55–62. doi: 10.1038/nature18003
Miotto, R., Li, L., Kidd, B. A., Dudley, J. T. (2016). Deep Patient: An Unsupervised Representation to Predict the Future of Patients from the Electronic Health Records. Sci. Rep. 6, 1–10. doi: 10.1038/srep26094
Mishra, S., Srivastava, A. K., Suman, S., Kumar, V., Shukla, Y. (2015). Circulating miRNAs revealed as surrogate molecular signatures for the early detection of breast cancer. Cancer Lett. 369, 67–75. doi: 10.1016/j.canlet.2015.07.045
Moarii, M., Boeva, V., Vert, J.-P., Reyal, F. (2015). Changes in correlation between promoter methylation and gene expression in cancer. BMC Genomics 16, 873. doi: 10.1186/s12864-015-1994-2
Mondul, A. M., Moore, S. C., Weinstein, S. J., Männistö, S., Sampson, J. N., Albanes, D. (2014). 1-Stearoylglycerol is associated with risk of prostate cancer: Results from a serum metabolomic profiling analysis. Metabolomics 10, 1036–1041. doi: 10.1007/s11306-014-0643-0
More, T., RoyChoudhury, S., Gollapalli, K., Patel, S. K., Gowda, H., Chaudhury, K., et al. (2015). Metabolomics and its integration with systems biology: PSI 2014 conference panel discussion report. J. Proteomics 127, 73–79. doi: 10.1016/j.jprot.2015.04.024
Morris, V., Rao, X., Pickering, C., Foo, W. C., Rashid, A., Eterovic, K., et al. (2017). Comprehensive Genomic Profiling of Metastatic Squamous Cell Carcinoma of the Anal Canal. Mol. Cancer Res. 15, 1542–1550. doi: 10.1158/1541-7786.MCR-17-0060
Murata, T., Yanagisawa, T., Kurihara, T., Kaneko, M., Ota, S., Enomoto, A., et al. (2019). Salivary metabolomics with alternative decision tree-based machine learning methods for breast cancer discrimination. Breast Cancer Res. Treat. 177, 591–601. doi: 10.1007/s10549-019-05330-9
Nam, H., Chung, B. C., Kim, Y., Lee, K., Lee, D. (2009). Combining tissue transcriptomics and urine metabolomics for breast cancer biomarker identification. Bioinformatics 25, 3151–3157. doi: 10.1093/bioinformatics/btp558
Nik-Zainal, S., Davies, H., Staaf, J., Ramakrishna, M., Glodzik, D., Zou, X., et al. (2016). Landscape of somatic mutations in 560 breast cancer whole-genome sequences. Nature 534, 47–54. doi: 10.1038/nature17676
Nishiumi, S., Kobayashi, T., Ikeda, A., Yoshie, T., Kibi, M., Izumi, Y., et al. (2012). A novel serum metabolomics-based diagnostic approach for colorectal cancer. PLoS One 7. doi: 10.1371/journal.pone.0040459
Osborne, J. D., Wyatt, M., Westfall, A. O., Willig, J., Bethard, S., Gordon, G. (2016). Efficient identification of nationally mandated reportable cancer cases using natural language processing and machine learning. J. Am. Med. Inf. Assoc. 23, 1077–1084. doi: 10.1093/jamia/ocw006
Paine, M. R. L., Liu, J., Huang, D., Ellis, S. R., Trede, D., Kobarg, J. H., et al. (2019). Three-Dimensional Mass Spectrometry Imaging Identifies Lipid Markers of Medulloblastoma Metastasis. Sci. Rep. 9, 2205. doi: 10.1038/s41598-018-38257-0
Palubeckaitė, I., Crooks, L., Smith, D. P., Cole, L. M., Bram, H., Le Maitre, C., et al. (2020). Mass spectrometry imaging of endogenous metabolites in response to doxorubicin in a novel 3D osteosarcoma cell culture model. J. Mass Spectrom. 55, e4461. doi: 10.1002/jms.4461
Paolillo, C., Londin, E., Fortina, P. (2016). Next generation sequencing in cancer: opportunities and challenges for precision cancer medicine. Scand. J. Clin. Lab. Invest. Suppl. 245, S84–S91. doi: 10.1080/00365513.2016.1210331
Park, K., Ali, A., Kim, D., An, Y., Kim, M., Shin, H. (2013). Robust predictive model for evaluating breast cancer survivability. Eng. Appl. Artif. Intell. 26, 2194–2205. doi: 10.1016/j.engappai.2013.06.013
Patel, S. K., Rajora, N., Kumar, S., Sahu, A., Kochar, S. K., Krishna, C. M., et al. (2019). Rapid Discrimination of Malaria- and Dengue-Infected Patients Sera Using Raman Spectroscopy. Anal. Chem. 91, 7054–7062. doi: 10.1021/acs.analchem.8b05907
Peng, L., Cantor, D., II, Huang, C., Wang, K., Baker, M. S., Nice, E. C. (2018). Tissue and plasma proteomics for early stage cancer detection. Mol. Omi. 14, 405–423. doi: 10.1039/c8mo00126j
Perakakis, N., Yazdani, A., Karniadakis, G. E., Mantzoros, C. (2018). Omics, big data and machine learning as tools to propel understanding of biological mechanisms and to discover novel diagnostics and therapeutics. Metabolism 87, A1–A9. doi: 10.1016/j.metabol.2018.08.002
Perng, W., Rifas-Shiman, S. L., McCulloch, S., Chatzi, L., Mantzoros, C., Hivert, M. F., et al. (2017). Associations of cord blood metabolites with perinatal characteristics, newborn anthropometry, and cord blood hormones in project viva. Metabolism 76, 11–22. doi: 10.1016/j.metabol.2017.07.001
Poirion, O. B., Chaudhary, K., Huang, S., Garmire, L. X. (2019). Multi-omics-based pan-cancer prognosis prediction using an ensemble of deep-learning and machine-learning models. medRxiv, 19010082. doi: 10.1101/19010082
Porta Siegel, T., Hamm, G., Bunch, J., Cappell, J., Fletcher, J. S., Schwamborn, K. (2018). Mass Spectrometry Imaging and Integration with Other Imaging Modalities for Greater Molecular Understanding of Biological Tissues. Mol. Imaging Biol. 20, 888–901. doi: 10.1007/s11307-018-1267-y
Rai, V., Karthikaichamy, A., Das, D., Noronha, S., Wangikar, P. P., Srivastava, S. (2016). Multi-omics Frontiers in Algal Research: Techniques and Progress to Explore Biofuels in the Postgenomics World. OMICS 20, 387–399. doi: 10.1089/omi.2016.0065
Ramazzotti, D., Lal, A., Wang, B., Batzoglou, S., Sidow, A. (2018). Multi-omic tumor data reveal diversity of molecular mechanisms that correlate with survival. Nat. Commun. 9, 4453. doi: 10.1038/s41467-018-06921-8
Ribli, D., Horváth, A., Unger, Z., Pollner, P., Csabai, I. (2018). Detecting and classifying lesions in mammograms with Deep Learning. Sci. Rep. 8, 4165. doi: 10.1038/s41598-018-22437-z
Robinson, D., Van Allen, E. M., Wu, Y. M., Schultz, N., Lonigro, R. J., Mosquera, J. M., et al. (2015). Integrative clinical genomics of advanced prostate cancer. Cell 161, 1215–1228. doi: 10.1016/j.cell.2015.05.001
Robinson, D. R., Wu, Y.-M., Lonigro, R. J., Vats, P., Cobain, E., Everett, J., et al. (2017). Integrative clinical genomics of metastatic cancer. Nature 548, 297–303. doi: 10.1038/nature23306
Romo-Bucheli, D., Janowczyk, A., Gilmore, H., Romero, E., Madabhushi, A. (2017). A deep learning based strategy for identifying and associating mitotic activity with gene expression derived risk categories in estrogen receptor positive breast cancers. Cytometry A 91, 566–573. doi: 10.1002/cyto.a.23065
Rubio-Perez, C., Tamborero, D., Schroeder, M. P., Antolín, A. A., Deu-Pons, J., Perez-Llamas, C., et al. (2015). In Silico Prescription of Anticancer Drugs to Cohorts of 28 Tumor Types Reveals Targeting Opportunities. Cancer Cell 27, 382–396. doi: 10.1016/j.ccell.2015.02.007
Sakellaropoulos, T., Vougas, K., Narang, S., Koinis, F., Kotsinas, A., Polyzos, A., et al. (2019). A Deep Learning Framework for Predicting Response to Therapy in Cancer. Cell Rep. 29, 3367–3373.e4. doi: 10.1016/j.celrep.2019.11.017
Saltz, J., Gupta, R., Hou, L., Kurc, T., Singh, P., Nguyen, V., et al. (2018). Spatial Organization and Molecular Correlation of Tumor-Infiltrating Lymphocytes Using Deep Learning on Pathology Images. Cell Rep. 23, 181–193.e7. doi: 10.1016/j.celrep.2018.03.086
Schoof, E. M., Rapin, N., Savickas, S., Gentil, C., Lechman, E., Haile, J. S., et al. (2019). A Quantitative Single-Cell Proteomics Approach to Characterize an Acute Myeloid Leukemia Hierarchy. bioRxiv, 745679. doi: 10.1101/745679
Sevakula, R. K., Singh, V., Verma, N. K., Kumar, C., Cui, Y. (2019). Transfer Learning for Molecular Cancer Classification Using Deep Neural Networks. IEEE/ACM Trans. Comput. Biol. Bioinforma. 16, 2089–2100. doi: 10.1109/TCBB.2018.2822803
Sirinukunwattana, K., Ahmed Raza, S. E., Tsang, Y.-W., Snead, D. R. J., Cree, I. A., Rajpoot, N. M. (2016). Locality Sensitive Deep Learning for Detection and Classification of Nuclei in Routine Colon Cancer Histology Images. IEEE Trans. Med. Imaging 35, 1196–1206. doi: 10.1109/TMI.2016.2525803
Sohni, A., Bartoccetti, M., Khoueiry, R., Spans, L., Vande Velde, J., De Troyer, L., et al. (2015). Dynamic Switching of Active Promoter and Enhancer Domains Regulates Tet1 and Tet2 Expression during Cell State Transitions between Pluripotency and Differentiation. Mol. Cell. Biol. 35, 1026–1042. doi: 10.1128/mcb.01172-14
Stemke-Hale, K., Gonzalez-Angulo, A. M., Lluch, A., Neve, R. M., Kuo, W.-L., Davies, M., et al. (2008). An integrative genomic and proteomic analysis of PIK3CA, PTEN, and AKT mutations in breast cancer. Cancer Res. 68, 6084–6091. doi: 10.1158/0008-5472.CAN-07-6854
Stoeckli, M., Chaurand, P., Hallahan, D. E., Caprioli, R. M. (2001). Imaging mass spectrometry: A new technology for the analysis of protein expression in mammalian tissues. Nat. Med. 7, 493–496. doi: 10.1038/86573
Sun, D., Wang, M., Li, A. (2018). A multimodal deep neural network for human breast cancer prognosis prediction by integrating multi-dimensional data. IEEE/ACM Trans. Comput. Biol. Bioinforma. 16, 841–850. doi: 10.1109/TCBB.2018.2806438
Sun, C., Li, T., Song, X., Huang, L., Zang, Q., Xu, J., et al. (2019a). Spatially resolved metabolomics to discover tumor-associated metabolic alterations. Proc. Natl. Acad. Sci. U. S. A. 116, 52–57. doi: 10.1073/pnas.1808950116
Sun, N., Kunzke, T., Sbiera, S., Kircher, S., Feuchtinge, A., Aichler, M., et al. (2019b). Prognostic relevance of steroid sulfation in adrenocortical carcinoma revealed by molecular phenotyping using high-resolution mass spectrometry imaging. Clin. Chem. 65, 1276–1286. doi: 10.1373/clinchem.2019.306043
Syrjala, K. L. (2018). Opportunities for improving oncology care. Lancet Oncol. 19, 449. doi: 10.1016/S1470-2045(18)30208-0
Therapeutically Applicable Research to Generate Effective Treatments (TARGET. Available at: https://ocg.cancer.gov/programs/target (Accessed March 24, 2020).
Thorsen, S. F., Gromova, I., Christensen, I. J., Fredriksson, S., Andersen, C. L., Nielsen, H. J., et al. (2019). Gel-Based Proteomics of Clinical Samples Identifies Potential Serological Biomarkers for Early Detection of Colorectal Cancer. Int. J. Mol. Sci. 20. doi: 10.3390/ijms20236082
Torata, N., Kubo, M., Miura, D., Ohuchida, K., Mizuuchi, Y., Fujimura, Y., et al. (2018). Visualizing energy charge in breast carcinoma tissues by MALDI mass-spectrometry imaging profiles of low-molecular-weight metabolites, in Anticancer Research (International Institute of Anticancer Research), 4267–4272. doi: 10.21873/anticanres.12723
Tsai, M.-H., Wu, C.-C., Peng, P.-H., Liang, Y., Hsiao, Y.-C., Chien, K.-Y., et al. (2012). Identification of secretory gelsolin as a plasma biomarker associated with distant organ metastasis of colorectal cancer. J. Mol. Med. (Berl). 90, 187–200. doi: 10.1007/s00109-011-0817-4
Turajlic, S., Sottoriva, A., Graham, T., Swanton, C. (2019). Resolving genetic heterogeneity in cancer. Nat. Rev. Genet. 20, 404–416. doi: 10.1038/s41576-019-0114-6
Turki, T., Wei, Z., Wang, J. T. L. (2018). A transfer learning approach via procrustes analysis and mean shift for cancer drug sensitivity prediction. J. Bioinform. Comput. Biol. 16:1840014. doi: 10.1142/S0219720018400140
Uchiyama, Y., Hayasaka, T., Masaki, N., Watanabe, Y., Masumoto, K., Nagata, T., et al. (2014). Imaging mass spectrometry distinguished the cancer and stromal regions of oral squamous cell carcinoma by visualizing phosphatidylcholine (16:0/16:1) and phosphatidylcholine (18:1/20:4). Anal. Bioanal. Chem. 406 (5), 1307–1316. doi: 10.1007/s00216-013-7062-3
Van Emon, J. M. (2016). The Omics Revolution in Agricultural Research. J. Agric. Food Chem. 64, 36–44. doi: 10.1021/acs.jafc.5b04515
Vantaku, V., Dong, J., Ambati, C. R., Perera, D., Donepudi, S. R., Amara, C. S., et al. (2019). Multi-omics Integration Analysis Robustly Predicts High-Grade Patient Survival and Identifies CPT1B Effect on Fatty Acid Metabolism in Bladder Cancer. Clin. Cancer Res. 25, 3689–3701. doi: 10.1158/1078-0432.CCR-18-1515
Varambally, S., Dhanasekaran, S. M., Zhou, M., Barrette, T. R., Kumar-Sinha, C., Sanda, M. G., et al. (2002). The polycomb group protein EZH2 is involved in progression of prostate cancer. Nature 419, 624–629. doi: 10.1038/nature01075
Vasudevan, P., Murugesan, T. (2018). Cancer Subtype Discovery Using Prognosis-Enhanced Neural Network Classifier in Multigenomic Data. Technol. Cancer Res. Treat. 17:1533033818790509. doi: 10.1177/1533033818790509
Veselkov, K. A., Vingara, L. K., Masson, P., Robinette, S. L., Want, E., Li, J. V., et al. (2011). Optimized preprocessing of ultra-performance liquid chromatography/mass spectrometry urinary metabolic profiles for improved information recovery. Anal. Chem. 83, 5864–5872. doi: 10.1021/ac201065j
Vidavsky, N., Kunitake, J. A. M. R., Diaz-Rubio, M. E., Chiou, A. E., Loh, H. C., Zhang, S., et al. (2019). Mapping and Profiling Lipid Distribution in a 3D Model of Breast Cancer Progression. ACS Cent. Sci. 5, 768–780. doi: 10.1021/acscentsci.8b00932
Vougas, K., Sakellaropoulos, T., Kotsinas, A., Foukas, G. R. P., Ntargaras, A., Koinis, F., et al. (2019). Machine learning and data mining frameworks for predicting drug response in cancer: An overview and a novel in silico screening process based on association rule mining. Pharmacol. Ther. 203, 107395. doi: 10.1016/j.pharmthera.2019.107395
Wang, M., Carver, J. J., Phelan, V. V., Sanchez, L. M., Garg, N., Peng, Y., et al. (2016). Sharing and community curation of mass spectrometry data with Global Natural Products Social Molecular Networking. Nat. Biotechnol. 34, 828–837. doi: 10.1038/nbt.3597
Wang, S., Chen, X., Luan, H., Gao, D., Lin, S., Cai, Z., et al. (2016). Matrix-assisted laser desorption/ionization mass spectrometry imaging of cell cultures for the lipidomic analysis of potential lipid markers in human breast cancer invasion. Rapid Commun. Mass Spectrom. 30, 533–542. doi: 10.1002/rcm.7466
Wang, Z., Jensen, M. A., Zenklusen, J. C. (2016). “A practical guide to The Cancer Genome Atlas (TCGA),” in Methods in Molecular Biology (Humana Press Inc.), 111–141. doi: 10.1007/978-1-4939-3578-9_6
Wang, X., Han, J., Hardie, D. B., Yang, J., Pan, J., Borchers, C. H. (2017). Metabolomic profiling of prostate cancer by matrix assisted laser desorption/ionization-Fourier transform ion cyclotron resonance mass spectrometry imaging using Matrix Coating Assisted by an Electric Field (MCAEF). Biochim. Biophys. Acta - Proteins Proteomics 1865, 755–767. doi: 10.1016/j.bbapap.2016.12.012
Wang, Y., Wang, D., Ye, X., Wang, Y., Yin, Y., Jin, Y. (2019). A tree ensemble-based two-stage model for advanced-stage colorectal cancer survival prediction. Inf. Sci. (NY). 474, 106–124. doi: 10.1016/j.ins.2018.09.046
Wheeler, D. A., Srinivasan, M., Egholm, M., Shen, Y., Chen, L., McGuire, A., et al. (2008). The complete genome of an individual by massively parallel DNA sequencing. Nature 452, 872–876. doi: 10.1038/nature06884
Wilks, C., Cline, M. S., Weiler, E., Diehkans, M., Craft, B., Martin, C., et al. (2014). The Cancer Genomics Hub (CGHub): overcoming cancer through the power of torrential data. Database (Oxford) 2014. doi: 10.1093/database/bau093
Wu, T., Dai, Y. (2017). Tumor microenvironment and therapeutic response. Cancer Lett. 387, 61–68. doi: 10.1016/j.canlet.2016.01.043
Wu, C., Zhou, F., Ren, J., Li, X., Jiang, Y., Ma, S. (2019). A selective review of multi-level omics data integration using variable selection. High-Throughput 8. doi: 10.3390/ht8010004
Wu, P., Heins, Z. J., Muller, J. T., Katsnelson, L., de Bruijn, I., Abeshouse, A. A., et al. (2019). Integration and Analysis of CPTAC Proteomics Data in the Context of Cancer Genomics in the cBioPortal. Mol. Cell. Proteomics 18, 1893–1898. doi: 10.1074/mcp.TIR119.001673
Xu, J., Xiang, L., Liu, Q., Gilmore, H., Wu, J., Tang, J., et al. (2016). Stacked sparse autoencoder (SSAE) for nuclei detection on breast cancer histopathology images. IEEE Trans. Med. Imaging 35, 119–130. doi: 10.1109/TMI.2015.2458702
Xu, C., Zhou, D., Luo, Y., Guo, S., Wang, T., Liu, J., et al. (2017). Tissue and serum lipidome shows altered lipid composition with diagnostic potential in mycosis fungoides. Oncotarget 8, 48041–48050. doi: 10.18632/oncotarget.18228
Xu, J., Wu, P., Chen, Y., Meng, Q., Dawood, H., Dawood, H. (2019). A hierarchical integration deep flexible neural forest framework for cancer subtype classification by integrating multi-omics data. BMC Bioinf. 20, 527. doi: 10.1186/s12859-019-3116-7
Yamazaki, Y. (2015). Metabolome Analysis of Human Serum: Implications for Early Detection of Colorectal Cancer. Rinsho Byori. 63, 328–335.
Yang, L., Rong, W., Xiao, T., Zhang, Y., Xu, B., Liu, Y., et al. (2013). Secretory/releasing proteome-based identification of plasma biomarkers in HBV-associated hepatocellular carcinoma. Sci. China Life Sci. 56, 638–646. doi: 10.1007/s11427-013-4497-x
Yoon, J., Jordon, J., Van Der Schaar, M. (2018). Ganite: Estimation of individualized treatment effects using generative adversarial nets. in 6th International Conference on Learning Representations, ICLR 2018 - Conference Track Proceedings.
Yu, K.-H., Zhang, C., Berry, G. J., Altman, R. B., Ré, C., Rubin, D. L., et al. (2016). Predicting non-small cell lung cancer prognosis by fully automated microscopic pathology image features. Nat. Commun. 7, 12474. doi: 10.1038/ncomms12474
Yu, K.-H., Berry, G. J., Rubin, D. L., Ré, C., Altman, R. B., Snyder, M. (2017). Association of Omics Features with Histopathology Patterns in Lung Adenocarcinoma. Cell Syst. 5, 620–627.e3. doi: 10.1016/j.cels.2017.10.014
Yu, P., Wilhelm, K., Dubrac, A., Tung, J. K., Alves, T. C., Fang, J. S., et al. (2017). FGF-dependent metabolic control of vascular development. Nature 545, 224–241. doi: 10.1038/nature22322
Yuan, Y., Failmezger, H., Rueda, O. M., Raza Ali, H., Gräf, S., Chin, S. F., et al. (2012). Quantitative image analysis of cellular heterogeneity in breast tumors complements genomic profiling. Sci. Transl. Med. 4, 157ra143–157ra143. doi: 10.1126/scitranslmed.3004330
Zeng, I. S. L., Lumley, T. (2018). Review of statistical learning methods in integrated omics studies (An integrated information science). Bioinform. Biol. Insights 12. doi: 10.1177/1177932218759292
Zhang, W., Li, F., Nie, L. (2010). Integrating multiple “omics” analysis for microbial biology: Application and methodologies. Microbiology 156, 287–301. doi: 10.1099/mic.0.034793-0
Zhang, L., Jin, H., Guo, X., Yang, Z., Zhao, L., Tang, S., et al. (2012). Distinguishing pancreatic cancer from chronic pancreatitis and healthy individuals by 1H nuclear magnetic resonance-based metabonomic profiles. Clin. Biochem. 45, 1064–1069. doi: 10.1016/j.clinbiochem.2012.05.012
Zhang, T., Wu, X., Ke, C., Yin, M., Li, Z., Fan, L., et al. (2013). Identification of potential biomarkers for ovarian cancer by urinary metabolomic profiling. J. Proteome Res. 12, 505–512. doi: 10.1021/pr3009572
Zhang, S., Xu, Y., Hui, X., Yang, F., Hu, Y., Shao, J., et al. (2017). Improvement in prediction of prostate cancer prognosis with somatic mutational signatures. J. Cancer 8, 3261–3267. doi: 10.7150/jca.21261
Zhang, J., Bajari, R., Andric, D., Gerthoffert, F., Lepsa, A., Nahal-Bose, H., et al. (2019). The International Cancer Genome Consortium Data Portal. Nat. Biotechnol. 37, 367–369. doi: 10.1038/s41587-019-0055-9
Zhang, X., Zhang, J., Sun, K., Yang, X., Dai, C., Guo, Y. (2019). “Integrated Multi-omics Analysis Using Variational Autoencoders: Application to Pan-cancer Classification,” in Proceedings - 2019 IEEE International Conference on Bioinformatics and Biomedicine, BIBM 2019, 765–769. doi: 10.1109/BIBM47256.2019.8983228
Zhao, M., Tang, Y., Kim, H., Hasegawa, K. (2018). Machine Learning With K-Means Dimensional Reduction for Predicting Survival Outcomes in Patients With Breast Cancer. Cancer Inform. 17:1176935118810215. doi: 10.1177/1176935118810215
Zhu, L., Luo, W., Su, M., Wei, H., Wei, J., Zhang, X., et al. (2013). Comparison between artificial neural network and Cox regression model in predicting the survival rate of gastric cancer patients. Biomed. Rep. 1, 757–760. doi: 10.3892/br.2013.140
Keywords: multi-omics, artificial intelligence (AI), data integration, cancer biomarkers, patient stratification
Citation: Patel SK, George B and Rai V (2020) Artificial Intelligence to Decode Cancer Mechanism: Beyond Patient Stratification for Precision Oncology. Front. Pharmacol. 11:1177. doi: 10.3389/fphar.2020.01177
Received: 25 April 2020; Accepted: 20 July 2020;
Published: 12 August 2020.
Edited by:
Feng Zhu, Zhejiang University, ChinaReviewed by:
Manisha Sawhney, University of Mary, United StatesGui Zhao Liang, Chongqing University, China
Copyright © 2020 Patel, George and Rai. This is an open-access article distributed under the terms of the Creative Commons Attribution License (CC BY). The use, distribution or reproduction in other forums is permitted, provided the original author(s) and the copyright owner(s) are credited and that the original publication in this journal is cited, in accordance with accepted academic practice. No use, distribution or reproduction is permitted which does not comply with these terms.
*Correspondence: Vineeta Rai, dmluZWV0YWlpdGJvbWJheUBnbWFpbC5jb20=; orcid.org/0000-0003-4621-1260