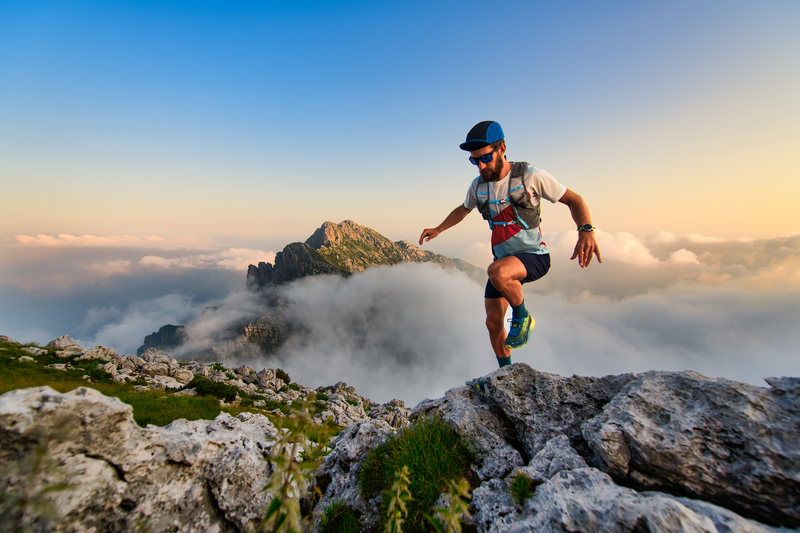
94% of researchers rate our articles as excellent or good
Learn more about the work of our research integrity team to safeguard the quality of each article we publish.
Find out more
ORIGINAL RESEARCH article
Front. Pharmacol. , 11 June 2019
Sec. Pharmacogenetics and Pharmacogenomics
Volume 10 - 2019 | https://doi.org/10.3389/fphar.2019.00654
This article is part of the Research Topic Next-Generation Sequencing in Pharmacogenetics/genomics View all 12 articles
Nudix Hydrolase 15 (NUDT15) and Thiopurine S-Methyltransferase (TPMT) are strong genetic determinants of thiopurine toxicity in pediatric acute lymphoblastic leukemia (ALL) patients. Since patients with NUDT15 or TPMT deficiency suffer severe adverse drug reactions, star (*) allele-based haplotypes have been used to predict an optimal 6-mercaptopurine (6-MP) dosing. However, star allele haplotyping suffers from insufficient, inconsistent, and even conflicting designations with uncertain and/or unknown functional alleles. Gene-wise variant burden (GVB) scoring enables us to utilize next-generation sequencing (NGS) data to predict 6-MP intolerance in children with ALL. Whole exome sequencing was performed for 244 pediatric ALL patients under 6-MP treatments. We assigned star alleles with PharmGKB haplotype set translational table. GVB for NUDT15 and TPMT was computed by aggregating in silico deleteriousness scores of multiple coding variants for each gene. Poor last-cycle dose intensity percent (DIP < 25%) was considered as 6-MP intolerance, resulting therapeutic failure of ALL. DIPs showed significant differences ( p < 0.05) among NUDT15 poor (PM, n = 1), intermediate (IM, n = 48), and normal (NM, n = 195) metabolizers. TPMT exhibited no PM and only seven IMs. GVB showed significant differences among the different haplotype groups of both NUDT15 and TPMT ( p < 0.05). Kruskal–Wallis test for DIP values showed statistical significances for the seven different GVB score bins of NUDT15. GVBNUDT15 outperformed the star allele-based haplotypes in predicting patients with reduced last-cycle DIPs at all DIP threshold levels (i.e., 5%, 10%, 15%, and 25%). In NUDT15-and-TPMT combined interaction analyses, GVBNUDT15,TPMT outperformed star alleles [area under the receiver operating curve (AUROC) = 0.677 vs. 0.645] in specificity (0.813 vs. 0.796), sensitivity (0.526 vs. 0.474), and positive (0.192 vs. 0.164) and negative (0.953 vs. 0.947) predictive values. Overall, GVB correctly classified five more patients (i.e., one into below and four into above 25% DIP groups) than did star allele haplotypes. GVB analysis demonstrated that 6-MP intolerance in pediatric ALL can be reliably predicted by aggregating NGS-based common, rare, and novel variants together without hampering the predictive power of the conventional haplotype analysis.
6-Mercaptopurine (6-MP) is a commonly used drug in the maintenance therapy of pediatric acute lymphoblastic leukemia (ALL). Since patients have a potential to experience medication-induced life-threatening side effects including bone marrow suppression and hepatotoxicity, providing a tailored drug dosing regimen is essential in clinical practice (Vogenberg et al., 2010).
One of the strongest ways to determine initial 6-MP dose is an experimental assessment of potential for drug adverse reactions, such as severe neutropenia by monitoring 6-MP metabolite concentration or using in vitro activity profiles (Dubinsky et al., 2000; Ansari et al., 2002;; Cuffari, 2005; Bradford, 2011; Supandi et al., 2018). However, applying such methods into routine clinical practice for predicting drug-induced toxicity is still challenging because it is extremely time-consuming, expensive, and inefficient (González-Lama and Gisbert, 2016).
As recent studies have demonstrated the strong association between genetic polymorphisms and inter-individual variability in 6-MP dose intensity, approaches to predict drug tolerance on the basis of individual genomic profiles have arisen. The primary genetic determinant of thiopurine toxicity is TPMT, which plays a crucial role in identifying patients in need of treatment modification with reduced enzyme activity (Lennard, 2014). However, this has not been applicable to East Asian populations since the frequency of TPMT polymorphisms varies by ethnicity (Relling et al., 2013). Recently, a novel pharmacogenetic marker, NUDT15, has clarified its role in predicting thiopurine toxicity in Asian populations (Yang et al., 2014; Yang et al., 2015; Zgheib et al., 2016; Kakuta et al., 2017). Clinical Pharmacogenetics Implementation Consortium (CPIC) published an updated guideline for thiopurine dosing based on both TPMT and NUDT15 genotypes using the star allele-based dose prediction method (Relling and Klein, 2009; Relling et al., 2018). This prevailing method provides therapeutic recommendations for dosing based on star allele genotypes. However, the utilization of star alleles in clinical practice has many obstacles that occur mainly due to 1) the extremely complex nomenclature system, 2) the limited resolution of phenotype prediction due to many unknown and uncertain function alleles, 3) ignorance of functional impacts of rare and/or novel variants, and 4) limited use in previously studied populations only (Robarge et al., 2007). Next-generation sequencing (NGS) challenges the conventional star alleles on the basis of genotyping technologies and clinical studies in case–control settings.
In the era of NGS, the comprehensive genotyping capabilities of NGS platform have enabled us to capture the true diversity of gene variation, and researchers propose alternative ways to predict individual intolerance towards a drug. One promising method is a gene-wise variant burden (GVB) scoring approach that can calculate gene-wise cumulative variant deleteriousness scores including common, rare, and even novel genetic variants for each gene (Lee et al., 2016). Here, we assessed the utility of GVB scoring method in quantifying the potential contributing effect of variants on enzymatic activity. By combining the clinically proven and well-established associations between the two genes, i.e., NUDT15 and TPMT, and 6-MP dose intensity percent (DIP, actual/planned dose) as a clinical endpoint, we performed a comparison study of the conventional star allele-based haplotyping and GVB scoring methods for predicting the last-cycle 6-MP DIP as an indicator for 6-MP intolerance of ALL patients with NUDT15 and/or TPMT deficiency. Overall, both star alleles and GVB showed significant correlations with 6-MP DIP values. Star allele-based haplotype groups showed significant correlation with GVB score groups. For predicting reduced last-cycle DIP values, GVB analysis outperformed the conventional star allele method for NUDT15 and showed comparable result for TPMT. In NUDT15-and-TPMT combined interaction analyses, GVBNUDT15,TPMT outperformed star allele-based predictions [area under the receiver operating curve (AUROC) = 0.677 vs. 0.645] in specificity (0.813 vs. 0.796), sensitivity (0.526 vs. 0.474), and positive (PPV; 0.192 vs. 0.164) and negative (NPV; 0.953 vs. 0.947) predictive values. It is demonstrated that gene-wise evaluation of in silico deleterious variant score burden can be a useful method for predicting 6-MP intolerance in pediatric ALL patients, considering NGS-based common, rare, and novel variants concurrently while not hampering the predictive power of the conventional haplotype analysis.
A total of 298 Korean pediatric ALL patients with 6-MP treatment during maintenance therapy were recruited in the present study from two major teaching hospitals, i.e., Asan Medical Center (AMC) and Seoul National University Hospital (SNUH). Of the 298 subjects, 244 individuals who did not meet the exclusion criteria (i.e., relapse of the disease, stem cell transplantation, Burkitt’s lymphoma, mixed phenotype acute leukemia, infant ALL, or very high risk) were selected. All participants provided written informed consent. The study was approved by the AMC Review Boards and the SNUH Review Boards. The 6-MP dose per meter body surface area over a 12-week cycle was recorded. The maximum tolerated dose of 6-MP was defined as the dose at the last maintenance cycle for each patient. Patients from two hospitals had received treatment under the same treatment protocol and dose adjustment guidelines to maintain the ANC levels within target levels (500–1,500/µL). Genotype-guided dose modification was not conducted. Additional demographic data are shown in Table 1.
Exome sequencing was performed using Ion AmpliSeq™ Exome panel to screen coding sequence region of entire genome. This panel included the exome of 19,072 genes and the size of the total targeted region was 57.7 Mb. The panel contained 293,903 primer pairs that were multiplexed into 12 pools to avoid primer-dimer formation and interference during PCR. The range of amplicons amplified by these oligo primer pairs ranged from 125 to 275 bp, and the rate of “on target” coverage for this panel was 95.69%. PCR assays were performed directly to amplify 100 ng of genomic DNA samples extracted from normal blood cells in bone marrow aspirates or peripheral blood so as to collect the target regions using the oligo primer pairs of the panel. Reaction parameters were as follows: 99°C for 2 min, followed by 10 cycles of 99°C for 15 s, 60°C for 16 min, and 10°C for 1 min. After amplification, library construction was performed by using the Ion AmpliSeq library kit plus as described in the manufacturer’s instructions (Thermo Scientific, Waltham, MA). Libraries were quantified using an Agilent 2100 Bioanalyzer (Agilent, Santa Clara, CA) and then diluted to ∼10 pM. Subsequently, 33.3 μL of the barcoded libraries was combined in sets of three barcodes. The combined libraries were sequenced using the Ion Proton platform with PI chip V3, following the manufacturer’s instructions (Thermo Scientific, Waltham, MA). Reads were mapped to the human reference genome build (hg19) with a mapping alignment program from Thermo Fisher (version 4.4, Torrent Suite Software) on germ-line and low stringency settings (minimum observed allele frequency required for a non-reference variant call is 0.18 for single-nucleotide variant (SNV) and 0.23 for InDel, minimum phred scales call quality is 14 for SNV and 19 for InDel, minimum coverage for called variants is 35 for SNV and 40 for InDel, and maximum strand bias is 0.95 for SNV and 0.75 for InDel). Single-nucleotide variants (SNVs) and short insertions/deletions (InDels) were identified via Genome Analysis Toolkit (GATK) 2.8-1 Unified Genotyper (DePristo et al., 2011). To estimate the pathogenicity of variants, two in silico variant deleteriousness prediction scores were annotated: sorting intolerant from tolerant (SIFT) (Ng, 2003) and combined annotation dependent depletion (CADD) (Kircher et al., 2014). The protein-coding gene region was defined using ANNOVAR (http://annovar.openbioinformatics.org/) (Wang et al., 2010). All the variants identified in 244 ALL samples are described in Supplementary Table S1 and S2.
Gene-wise deleterious variant burden was computed for NUDT15 and TPMT as described by Lee et al. (2016) and Seo et al. (2018). Under the hypothesis that variants that have potential effects to change protein function not necessarily guarantee but have power to cause harmful phenotypes, only variants with SIFT scores less than 0.7 were further considered.
As SIFT does not provide functional scores for InDels, adjv for all InDel variants were assigned as 1e-8 under the hypothesis that InDels are more deleterious than single-nucleotide substitutions. Considering the dosage effects, adjusted SIFT score adjv was calculated for each variant according to their genotype.
For each gene Gi with n deleterious variants, we calculated GVB(Gi), the cumulative genic effect for all coding variants of the gene, by calculating the geometric mean of adjv (Equation). GVBg is considered as 1 if the count n of variant j with scores less than 0.7 in a gene is 0, indicating that the gene is not displaying any deleterious variant.
We obtained GVBg values for each individual ranging from 0 to 1. To predict 6-MP sensitivity, GVBNUDT15,TPMT was generated by calculating the geometric mean of GVBNUDT15 and GVBTPMT.
To classify 244 ALL samples into three metabolizer groups, we inferred haplotypes using the PHASE 2.1.1 software (Stephens et al., 2001; Stephens and Scheet, 2005) (Supplementary Figure S3). On the basis of the inferred haplotype information, we extracted star alleles that matched the haplotype set translational table from PharmGKB (https://www.pharmgkb.org/) (Whirl-Carrillo et al., 2012). Predicted genotypes were translated into molecular phenotypes on the basis of the coded genotype–phenotype translation tables from Moriyama et al. (2016) for NUDT15 and from PharmGKB tables for TPMT.
To assess prediction accuracies, we calculated DIP, the percentage of the actual administered dose to the planned dose, as an index for 6-MP drug toxicity. Dose in the last maintenance cycle was used, since the doses of 6-MP in the final maintenance cycle were supposed to be the maximum tolerated doses for patients (Kim et al., 2012). DIP prediction accuracies of GVB (GVBNUDT15, GVBTPMT, and GVBNUDT15,TPMT) and star allele-based predictions were compared using AUROC analysis with the R language pROC package (Robin et al., 2011). We computed specificity, sensitivity, PPV, and NPV under the binary classification model with nine different cutoff levels (i.e., 5%, 10%, 15%, 25%, 35%, 45%, 60%, 80%, and 100%) for defining high-risk DIP groups. All statistical analyses were performed using R version 3.5.1.
NUDT15 and TPMT haplotypes of each subject were first inferred from whole exome sequencing (WXS) data by using the PHASE tool, and matched star allele genotypes were assigned for each subject. The star allele genotypes were then translated into three molecular phenotype groups according to their allele combinations; poor (PM, No function|No function), intermediate (IM, Normal|No function or Normal|Decreased), and normal (NM, Normal|Normal) metabolizers. Six and four star alleles were identified for NUDT15 and TPMT genes, respectively, from the 244 ALL patients with their frequencies (Table 2). Table 3 shows the distribution of subsequently predicted enzymatic metabolizer phenotypes for NUDT15 and TPMT among the 244 ALL patients.
While 49 (20.1%) of 244 ALL patients were classified into non-NM (one PM and 48 IMs) phenotype for NUDT15, only seven (2.9%) IMs were identified for TPMT, reflecting ethnic variation of NUDT15 and TPMT variants, in a consistent manner (Table 3). Since individuals with TPMT homozygous mutant alleles are rarely observed in East Asian population, none of the patients were classified into the poor metabolizer group. IMs were stratified into two groups: 1) individuals carrying one copy of a normal function allele and one copy of a decreased function allele and 2) individuals carrying one copy of a normal function allele and one copy of no function allele. Carriers of non-functional allele, compared with carriers of decreased function allele, are considered to be at an increased risk for functional decline.
Patients with NUDT15 normal metabolizing alleles (DIP = 67.608 ± 28.2, n = 195) tolerated significantly higher DIPs of 6-MP than did slow metabolizers [5.712 (PM, n = 1), 56.452 ± 28.2 (IM, n = 48)] (Figure 1A). Clinical usefulness of the conventional star allele-based classification was successfully demonstrated for NUDT15 variants in the present study. Due to the small number of non-NM subjects for TPMT in Korean ALL patients, the difference of DIPs between NM (65.702 ± 28.4, n = 237) and IM (46.805 ± 35.7, n = 7) did not reach statistical significance (p = 0.10, Figure 1B).
Figure 1 Distribution of last-cycle dose intensity percent of 6-mercaptopurine according to star allele-based molecular phenotype groups in ALL. Dose intensity percent distribution across (A)Nudix Hydrolase 15 (NUDT15) and (B)Thiopurine S-Methyltransferase (TPMT) molecular phenotype groups. Normal metabolizers of NUDT15 showed significantly higher dose intensity percent than did intermediate ( p = 0.006) and poor ( p = 0.090) metabolizers. *p < 0.1, **p < 0.05, and ***p < 0.01 by Mann–Whitney U test.
GVB scores among different molecular phenotype groups for NUDT15 (PM = 0.09, IM = 0.248 ± 0.1, and NM = 0.995 ± 0.1, Figure 2A) and for TPMT (IM = 0.229 ± 0.3, NM = 1 ± 0.0, Figure 2B) showed statistically significant differences. The observed positive correlation between our GVB score and the conventional enzymatic metabolizer phenotypes for both NUDT15 and TPMT variants strongly supported our further analysis. Note that those pharmacogenetic star alleles have long been empirically developed by clinical case–control studies and/or animal and molecular studies. In contrast, the GVB analysis is based on purely theoretical ab initio and in silico methods without requiring empirical studies that are prohibitively costly considering the numerous drugs and genetic variants discovered by NGS technologies and the interactions. In the following sections, we explore the potential of the GVB scoring method for predicting DIPs as an indicator of 6-MP intolerance in pediatric ALL patients.
Figure 2 Distribution of gene-wise variant burden (GVB) scores according to the star allele-based molecular phenotype groups. Gene-wise variant burden (GVB) scores across (A)NUDT15 and (B)TPMT molecular phenotype groups. Normal metabolizers showed significantly higher dose intensity percent than did intermediate (NUDT15, p = 4.17E−52; TPMTp = 5.84E−47) and poor (NUDT15, p = 1.9E−22) metabolizers. *p < 0.1, **p < 0.05, and ***p < 0.01 by Mann–Whitney U test.
Since both NUDT15 and TPMT genes are not highly variable, only seven and two GVB value groups for NUDT15 and TPMT, respectively, were identified in the 244 ALL patients. GVBNUDT15 demonstrated statistically significant positive correlation with DIP (p = 0.016 by Kruskal–Wallis test, p = 0.001 (p = 0.21) by Spearman’s rank correlation, p = 0.001 (τ = 0.17) by Kendall’s rank correlation) (Figure 3A). Exclusion of the two patients having both NUDT15 and TPMT variants slightly improved statistical significance (Supplementary Figure S4). Due to the low frequency of TPMT alleles in East Asian population, 97.5% (n = 238) of all ALL patients were classified into wild type (GVBTPMT = 1.00 ± 0.00) and only six (2.50%) were classified into variant type (GVBTPMT = 0.10 ± 0.00) groups, resulting in poor statistical significance (p = 0.408 by T-test, p = 0.272 (ρ = 0.07) by Spearman’s rank correlation, p = 0.271 (τ = 0.06) by Kendall’s rank correlation) (Figure 3B).
Figure 3 Distribution of last-cycle dose intensity percent of 6-mercaptopurine according to gene-wise variant burden (GVB) score bins. (A) GVBNUDT15 [Kruskal–Wallis p-value = 0.016, Spearman’s rank correlation p-value = 0.001 ( ρ = 0.21), and Kendall’s rank correlation p-value = 0.001 (τ = 0.17)]. (B) GVBTPMT [Kruskal–Wallis p-value = 0.271, Spearman’s rank correlation p-value = 0.272 ( ρ = 0.07), and Kendall’s rank correlation p-value = 0.271 (τ = 0.06)].
Using ROC analysis, we evaluated the performances of GVB at nine cutoff levels (i.e., DIP < 5%, 10%, 15%, 25%, 35%, 45%, 60%, 80%, and 100%) for defining the 6-MP high-risk groups. Star allele-based classification was also applied for systematic comparison across different DIP threshold levels. DIP below 25% of planned dose of 6-MP is a generally accepted threshold for predicting 6-MP intolerance. Figure 4A demonstrates that GVBNUDT15 showed better AUCs at all threshold DIP levels below 25% (0.998 (DIP < 5%), 0.676 (DIP < 10%), 0.669 (DIP < 15%), and 0.653 (DIP < 25%)) than did the conventional star allele-based molecular phenotypes (AUC = 0.618). Moreover, exclusion of the two confounding patients with both NUDT15 and TPMT variant alleles slightly improved performances than did both before-exclusion GVBNUDT15 at all threshold DIP levels below 25% [AUC = 0.998 (DIP < 5%), 0.676 (DIP < 10%), 0.639 (DIP < 15%), and 0.627 (DIP < 25%)] and the star allele-based (AUC = 0.596) analyses (Figure 4B). Mainly due to the low frequency of TPMT variant alleles in East Asian population, both GVBTPMT and star allele-based predictions using TPMT seem to show poor AUCs for predicting DIP at all threshold levels (Figure 4C and D).
Figure 4 Comparison of diagnostic accuracies between star allele-based molecular phenotyping and GVB scoring for 6-mercaptopurine intolerance in ALL. Diagnostic accuracies are measured by using AUROC analysis for (A) GVBNUDT15 excluding two subjects with TPMT variants (DeLong’s p-value = 0.163), (B) GVBNUDT15 (DeLong’s p-value = 0.163), (C) GVBTPMT excluding seven subjects with NUDT15 variants (DeLong’s p-value = 0.5), and (D) GVBTPMT (DeLong’s p-value = 0.841). Numbers in the last parentheses indicate area under the curve (AUC) with 95% confidence intervals. DIP, dose intensity percent; AUC, area under the curve.
More importantly, we performed ROC analysis by aggregating the genetic effects of these two genes, NUDT15 for East Asian and TPMT for European heritages. We computed and evaluated GVBNUDT15,TPMT, which outperformed GVBNUDT15 or GVBTPMT alone as well as the combined molecular phenotypes of both NUDT15 and TPMT at all DIP threshold levels (Figure 5). In summary, at the clinically important DIP level of below or above 25%, the best AUC values for GVBNUDT15,TPMT, GVBNUDT15, GVBTPMT, and combined star alleles were 0.677, 0.653, 0.574, and 0.645, respectively. GVBNUDT15,TPMT not only showed the best performance but also successfully included the two confounding patients with both NUDT15 and TPMT variant alleles. While combining GVB scores of multiple genes is simple and straightforward, it is not the case for star alleles, which do not provide a uniform way of combining method for multiple genes.
Figure 5 Comparison of diagnostic accuracies between combined (NUDT15 and TPMT) star allele-based molecular phenotyping and GVB scoring for 6-mercaptopurine intolerance in ALL. Diagnostic accuracies are measured by using AUC analysis for GVBNUDT15,TPMT (DeLong’s p-value = 0.175). Numbers in the last parentheses indicate AUC with 95% confidence intervals. DIP, dose intensity percent; AUC, area under the curve.
To test the clinical utility of GVB method for guiding 6-MP dosing and/or for providing systematic framework for clinical studies of 6-MP intolerance and its genetic determinants of NUDT15 and TPMT for predicting DIP groups, we evaluated the diagnostic characteristics of the conventional star allele-based and GVB scoring methods in a simulated clinical setting. Table 4A and 4B exhibits diagnostic accuracies for star allele-based molecular phenotype groups and gene-wise variant burden score groups, respectively, for 6-MP intolerance among 244 pediatric ALL patients by the last-cycle DIP of 6-MP. Of the 244 ALL patients, 189 (84.4%) exhibited no NUDT15 or TPMT variant and hence was classified into NMs for both genes (Table 4A). Of the rest 55 non-NM patients, nine (16.4%) showed DIP below 25%, while 10 of 189 (5.3%) NM patients showed low DIP values.
Table 4 Comparison of star allele-based haplotyping versus gene-wise variant burden (GVBNUDT15,TPMT) analyses for 6-mercaptopurine intolerance measured by last-cycle dose intensity percent in ALL. Diagnostic accuracy table of (A) star allele-based haplotypes and dose intensity percent groups and (B) gene-wise variant burden score and dose intensity percent groups.
Although one can choose many threshold levels of GVB, because star alleles can just provide a small number of categories, we chose the most reliable binning threshold of GVBNUDT15,TPMT ≤ 0.3, the cut-point that maximizes the Youden index (Supplementary Figure S5), for classifying the patients into the below and above 25% DIP groups as shown in Table 4B. It is a coincidence that Lee et al. (2016) also suggested GVBPharmacogenes ≤ 0.3 as the threshold for predicting pharmaceutical market withdrawals in general. GVBNUDT15,TPMT correctly classified one more high-risk (DIP ≤ 25%) and four more low-risk (DIP > 25%) patients into the correct-risk groups (Table 4B) than did the traditional haplotype-based method (Table 4A), with an improved sensitivity from 47.36% to 52.63% and an improved specificity from 79.56% to 81.33%, though the difference did not reach statistical significance (p-value for sensitivity = 1 and p-value for specificity = 0.134, as determined using a McNemar test). Both PPV and NPV increase from 16.36% to 19.23% and from 94.70% to 95.31%, respectively. Overall, it is suggested that the “computational” GVBNUDT15,TPMT is an improved or at least comparable predictor than the “empirical” star allele-based haplotypes for determining subjects with increased risk of 6-MP intolerance in pediatric ALL patients measured by the last-cycle 6-MP DIP.
An enduring challenge in precision medicine is to predict adequate drug responses for individual patients (Shah and Shah, 2012). Recent discoveries have revealed a few highly functional and clinically relevant novel variants associated with 6-MP intolerance. However, since implicating drug toxicity based on a single variant is notoriously unreliable as shown in Supplementary Figure S1 for SIFT and Supplementary Figure S2 for CADD, developing strategies to aggregate the key effects over a range of genomic region is highly required. In the present study, we evaluated the utility of gene-wise deleterious variant burden scoring method, as a sequencing-based, simple, reliable, quantitative, and easy-to-compare score for predicting 6-MP intolerance of 244 pediatric ALL patients. In addition to DIP, GVB showed a statistically significant negative correlation with the incidence of grade 4 neutropenia (p = 1.43E−04 by Kruskal–Wallis test, p = 3.89E−07 (ρ = −0.32) by Spearman’s rank correlation, and p = 8.06E−07 (τ = −0.27) by Kendall’s rank correlation (Supplementary Figure S6). This implies that GVB is a reliable score that can predict hematological toxicity in pediatric ALL patients. When beginning treatment, NGS-based drug intolerance prediction is useful because it is practical to detect patients at high risk of toxicity. For example, patients with low GVB have a high probability of 6-MP toxicity at the initial recommended dose range; thus, clinicians may attempt to reduce the initial target dose of 6-MP. After an initial target dose is determined, a close therapeutic drug monitoring could help to avoid potential causes for toxicity, such as clinically relevant drug–drug interactions, reduced drug clearance due to liver and/or renal impairment, and altered drug utilization due to physiological conditions, as a complementary type of practice during the treatment (Ju-Seop Kang, 2009).
GVB analysis has several benefits over conventional star allele-based approaches. GVB 1) quantitates gene-wise variant burden with a single score; 2) provides a measure of inter-individual genetic variability for each gene; 3) considers common, rare, and novel genetic variants together; 4) provides an ethnic variability-neutral method for studying pharmacogenomics; 5) provides a systematic and reliable framework for designing further pharmacogenomics studies considering many gene interactions for clinical endpoints; and 6) adopts the contributing effect of novel low-frequency variants with potentially reduced function in predicting individual drug toxicity.
Based upon the very recent CPIC updates on NUDT15, three newly enrolled alleles were characterized (Moriyama et al., 2017). Since new haplotype designation is highly dependent on the characteristics of the study population, there will be restrictions in incorporating new or even as-yet-unidentified evidences in predicting future drug intensity. GVB can be used to develop a model to determine optimal doses without requiring a multi-ethnic population study, especially for under-studied subpopulations.
The following limitations are inherent in the present study. To evaluate the validity of GVB, independent replication studies for an expanded gene–drug set with sufficient sample sizes in diverse ethnic groups are required as no novel variant was identified in the current study. A conventional single variant-based association test of rare variants requires infeasible magnitude of sample sizes (Bansal et al., 2010), but approaches that aggregate common, rare, and novel variants jointly will substantially reduce a required effective sample sizes (Witte, 2012). The robustness of the analysis framework shall further be improved as novel prognostic markers on 6-MP DIP are acquired. The limitations in interpreting the score includes that all InDels are treated as highly damaging as SIFT provides scores for only single-nucleotide variants. As there are many in silico variant deleteriousness scoring method based on different principles, comprehensive evaluation of different method is required (Supplementary Figure S7). We also performed CADD-based computation of GVB values, resulting in similar results (Supplementary Figures S8 and S9). It has been reported that CADD tends to evaluate in-frame InDels as relatively benign (Kircher et al., 2014). However, recent in vitro activity assay of NUDT15 (Moriyama et al., 2017) proved that in-frame InDel carriers are more likely to be in states with severely diminished response to 6-MP. It is strongly recommended that for clinical applications, potential clinical impacts of genetic variants on drug sensitivity should be further examined to improve estimation accuracy, as in silico prediction scores can exhibit incorrect predictions. Producing a custom capture panel for clinically actionable genes could be more cost-effective than an exome-based approach.
One subject who was correctly classified by GVB carried a low-frequency novel deletion and predicted to belong to the high-risk group by GVB, whereas star allele-based prediction classified this patient into the NM group for both NUDT15 and TPMT. The patient required reduced dose than recommended (DIP = 23.7%), supporting that GVB analysis resulted in 6-MP dose-related adverse drug reactions. The patient’s variant was heterozygous p.Gly17_Val18del, which was very recently assigned as NUDT15*9 with uncertain functionality. The other four who were correctly classified by GVB had p.Arg139His on one allele, which has assigned them to the IM (NUDT15 *1/*4) group. GVB classified them as relatively safe for drug toxicity, and none of them required a 25% reduction from the starting dose. Additionally, one patient who was classified as high risk by GVB was assigned to IM for both NUDT15 and TPMT and required a severely reduced dose (14%), suggesting that GVBNUDT15,TPMT exhibits benefits in aggregating effects of many moderate genetic determinants into a single quantitative value.
The study was approved by the Asan Medical Center (AMC) Review Boards and the Institutional Review Board of Seoul National University Hospital (SNUH). Informed written consents for blood sampling and analyses were obtained from all participants.
YP and JK designed the model and the framework. HK, JC, HI, and HK collected samples and clinical data. BM and MS carried out the experiment. YP and SY analyzed the data and carried out the implementation. YP performed the calculations. YP and JK wrote the manuscript. JK conceived the study and was in charge of overall direction and planning. All authors read and approved the final manuscript.
This research was supported by a grant (16183MFDS541) from the Ministry of Food and Drug Safety in 2019.
The authors declare that the research was conducted in the absence of any commercial or financial relationships that could be construed as a potential conflict of interest.
The Supplementary Material for this article can be found online at: https://www.frontiersin.org/articles/10.3389/fphar.2019.00654/full#supplementary-material
Ansari, A., Hassan, C., Duley, J., Marinaki, A., Shobowale-Bakre, E. M., Seed, P., et al. (2002). Thiopurine methyltransferase activity and the use of azathioprine in inflammatory bowel disease. Aliment. Pharmacol. Ther. 16, 1743–1750. doi: 10.1046/j.1365-2036.2002.01353.x
Bansal, V., Libiger, O., Torkamani, A., Schork, N. J. (2010). Statistical analysis strategies for association studies involving rare variants. Nat. Rev. Genet. 11, 773–785. doi: 10.1038/nrg2867
Bradford, K. (2011). Optimizing 6-mercaptopurine and azathioprine therapy in the management of inflammatory bowel disease. WJG. 17, 4166–4168. doi: 10.3748/wjg.v17.i37.4166
Cuffari, C. (2005). A physician’s guide to azathioprine metabolite testing. Gastroenterol. Hepatol. 2, 58–63. Retrieved from http://www.gastroenterologyandhepatology.net/.
DePristo, M. A., Banks, E., Poplin, R., Garimella, K. V., Maguire, J. R., Hartl, C., et al. (2011). A framework for variation discovery and genotyping using next-generation DNA sequencing data. Nat. Genet. 43, 491–498. doi: 10.1038/ng.806
Dubinsky, M. C., Lamothe, S., Yang, H. Y., Targan, S. R., Sinnett, D., Théorêt, Y., et al. (2000). Pharmacogenomics and metabolite measurement for 6-mercaptopurine therapy in inflammatory bowel disease. Gastroenterology 118, 705–713. doi: 10.1016/S0016-5085(00)70140-5
González-Lama, Y., Gisbert, J. P. (2016). Monitoring thiopurine metabolites in inflammatory bowel disease. Frontline Gastroenterol. 7, 301–307. doi: 10.1136/flgastro-2015-100681
Ju-Seop Kang, M.-H. L. (2009). Overview of therapeutic drug monitoring. Korean J. Inter. Med. 24, 1. doi: 10.3904/kjim.2009.24.1.1
Kakuta, Y., Kinouchi, Y., Shimosegawa, T. (2017). Pharmacogenetics of thiopurines for inflammatory bowel disease in East Asia: prospects for clinical application of NUDT15 genotyping. J. Gastroenterol. 53, 172–180. doi: 10.1007/s00535-017-1416-0
Kim, H., Kang, H. J., Kim, H. J., Jang, M. K., Kim, N. H., Oh, Y., et al. (2012). Pharmacogenetic analysis of pediatric patients with acute lymphoblastic leukemia: a possible association between survival rate and ITPA polymorphism. PLoS ONE 7, e45558–45510. doi: 10.1371/journal.pone.0045558
Kircher, M., Witten, D. M., Jain, P., O’Roak, B. J., Cooper, G. M., Shendure, J. (2014). A general framework for estimating the relative pathogenicity of human genetic variants. Nat. Genet. 46, 310–315. doi: 10.1038/ng.2892
Lee, K. H., Baik, S. Y., Lee, S. Y., Park, C. H., Park, P. J., Kim, J. H. (2016). Genome sequence variability predicts drug precautions and withdrawals from the market. PLoS ONE 11, e0162135–0162115. doi: 10.1371/journal.pone.0162135
Lennard, L. (2014). Implementation of TPMT testing. Br. J. Clin. Pharmacol. 77, 704–714. doi: 10.1111/bcp.12226
Moriyama, T., Nishii, R., Perez-Andreu, V., Yang, W., Klussmann, F. A., Zhao, X., et al. (2016). NUDT15 polymorphisms alter thiopurine metabolism and hematopoietic toxicity. Nat. Genet. 48, 367–373. doi: 10.1038/ng.3508
Moriyama, T., Yang, Y.-L., Nishii, R., Ariffin, H., Liu, C., Lin, T.-N., et al. (2017). Novel variants in NUDT15 and thiopurine intolerance in children with acute lymphoblastic leukemia from diverse ancestry. Blood 130, 1209–1212. doi: 10.1182/blood-2017-05-782383
Ng, P. C. (2003). SIFT: predicting amino acid changes that affect protein function. Nucleic Acids Res. 31, 3812–3814. doi: 10.1093/nar/gkg509
Relling, M. V., Klein, T. E. (2009). CPIC: Clinical Pharmacogenetics Implementation Consortium of the pharmacogenomics research network. Clin. Pharmacol. Ther. 89, 464–467. doi: 10.1038/clpt.2010.279
Relling, M. V., Gardner, E. E., Sandborn, W. J., Schmiegelow, K., Pui, C.-H., Yee, S. W., et al. (2013). Clinical Pharmacogenetics Implementation Consortium guidelines for thiopurine methyltransferase genotype and thiopurine dosing: 2013 update. Clin. Pharmacol. Ther. 93, 324–325. doi: 10.1038/clpt.2013.4
Relling, M. V., Schwab, M., Whirl-Carrillo, M., Suarez-Kurtz, G., Pui, C.-H., Stein, C. M., et al. (2018). Clinical Pharmacogenetics Implementation Consortium (CPIC) guideline for thiopurine dosing based on TPMT and NUDT15 genotypes: 2018 update. Clin. Pharmacol. Ther. 105, 1095–1105. doi: 10.1002/cpt.1304
Robarge, J. D., Li, L., Desta, Z., Nguyen, A., Flockhart, D. A. (2007). The star-allele nomenclature: retooling for translational genomics. Clin. Pharmacol. Ther. 82, 244–248. doi: 10.1038/sj.clpt.6100284
Robin, X., Turck, N., Hainard, A., Tiberti, N., Lisacek, F., Sanchez, J.-C., et al. (2011). pROC: an open-source package for R and S+ to analyze and compare ROC curves. BMC Bioinforma. 12, 77. doi: 10.1186/1471-2105-12-77
Seo, H., Kwon, E. J., You, Y.-A., Park, Y., Min, B. J., Yoo, K., et al. (2018). Deleterious genetic variants in ciliopathy genes increase risk of ritodrine-induced cardiac and pulmonary side effects. BMC Med. Genomics 11, 31. doi: 10.1186/s12920-018-0323-4
Shah, R. R., Shah, D. R. (2012). Personalized medicine: is it a pharmacogenetic mirage? Br J Clin. Pharmacol. 74, 698–721. doi: 10.1111/j.1365-2125.2012.04328.x
Stephens, M., Scheet, P. (2005). Accounting for decay of linkage disequilibrium in haplotype inference and missing-data imputation. Am. J. Hum. Genet. 76, 449–462. doi: 10.1086/428594
Stephens, M., Smith, N. J., Donnelly, P. (2001). A new statistical method for haplotype reconstruction from population data. Am. J. Hum. Genet. 68, 978–989. doi: 10.1086/319501
Supandi, S., Harahap, Y., Harmita, H., Andalusia, R. (2018). Quantification of 6-mercaptopurine and its metabolites in patients with acute lympoblastic leukemia using dried blood spots and UPLC-MS/MS. Sci. Pharm. 86, 18–18. doi: 10.3390/scipharm86020018
Vogenberg, F. R., Barash, C. I., Pursel, M. (2010). Personalized medicine, part 1: evolution and development into theranostics. Pharm. Ther. 35, 560–576. Retrieved from https://www.ptcommunity.com/.
Wang, K., Li, M., Hakonarson, H. (2010). ANNOVAR: functional annotation of genetic variants from high-throughput sequencing data. Nucleic Acids Res. 38, e164–e164. doi: 10.1093/nar/gkq603
Whirl-Carrillo, M., McDonagh, E. M., Hebert, J. M., Gong, L., Sangkuhl, K., Thorn, C. F., et al. (2012). Pharmacogenomics knowledge for personalized medicine. Clin. Pharmacol. Ther. 92, 414–417. doi: 10.1038/clpt.2012.96
Witte, J. S. (2012). Rare genetic variants and treatment response: sample size and analysis issues. Stat. Med. 31, 3041–3050. doi: 10.1002/sim.5428
Yang, J. J., Landier, W., Yang, W., Liu, C., Hageman, L., Cheng, C., et al. (2015). Inherited NUDT15 variant is a genetic determinant of mercaptopurine intolerance in children with acute lymphoblastic leukemia. JCO 33, 1235–1242. doi: 10.1200/JCO.2014.59.4671
Yang, S.-K., Hong, M., Baek, J., Choi, H., Zhao, W., Jung, Y., et al. (2014). A common missense variant in NUDT15 confers susceptibility to thiopurine-induced leukopenia. Nat. Genet. 46, 1017–1020. doi: 10.1038/ng.3060
Zgheib, N. K., Akika, R., Mahfouz, R., Aridi, C. A., Ghanem, K. M., Saab, R., et al. (2016). NUDT15 and TPMT genetic polymorphisms are related to 6-mercaptopurine intolerance in children treated for acute lymphoblastic leukemia at the Children’s Cancer Center of Lebanon. Pediatr. Blood Cancer 64, 146–150. doi: 10.1002/pbc.26189
Keywords: 6-mercaptopurine, drug toxicity, variant burden, pharmacogenetics, pharmacogenomics, next-generation sequencing, Nudix Hydrolase 15 (NUDT15), Thiopurine S-Methyltransferase (TPMT)
Citation: Park Y, Kim H, Choi JY, Yun S, Min B-J, Seo M-E, Im HJ, Kang HJ and Kim JH (2019) Star Allele-Based Haplotyping versus Gene-Wise Variant Burden Scoring for Predicting 6-Mercaptopurine Intolerance in Pediatric Acute Lymphoblastic Leukemia Patients. Front. Pharmacol. 10:654. doi: 10.3389/fphar.2019.00654
Received: 14 January 2019; Accepted: 20 May 2019;
Published: 11 June 2019.
Edited by:
Martin A. Kennedy, University of Otago, New ZealandReviewed by:
Chakradhara Rao, Satyanarayana Uppugunduri, Université de Genève, SwitzerlandCopyright © 2019 Park, Kim, Choi, Yun, Min, Seo, Im, Kang and Kim. This is an open-access article distributed under the terms of the Creative Commons Attribution License (CC BY). The use, distribution or reproduction in other forums is permitted, provided the original author(s) and the copyright owner(s) are credited and that the original publication in this journal is cited, in accordance with accepted academic practice. No use, distribution or reproduction is permitted which does not comply with these terms.
*Correspondence: Ju Han Kim, anVoYW5Ac251LmFjLmty
Disclaimer: All claims expressed in this article are solely those of the authors and do not necessarily represent those of their affiliated organizations, or those of the publisher, the editors and the reviewers. Any product that may be evaluated in this article or claim that may be made by its manufacturer is not guaranteed or endorsed by the publisher.
Research integrity at Frontiers
Learn more about the work of our research integrity team to safeguard the quality of each article we publish.