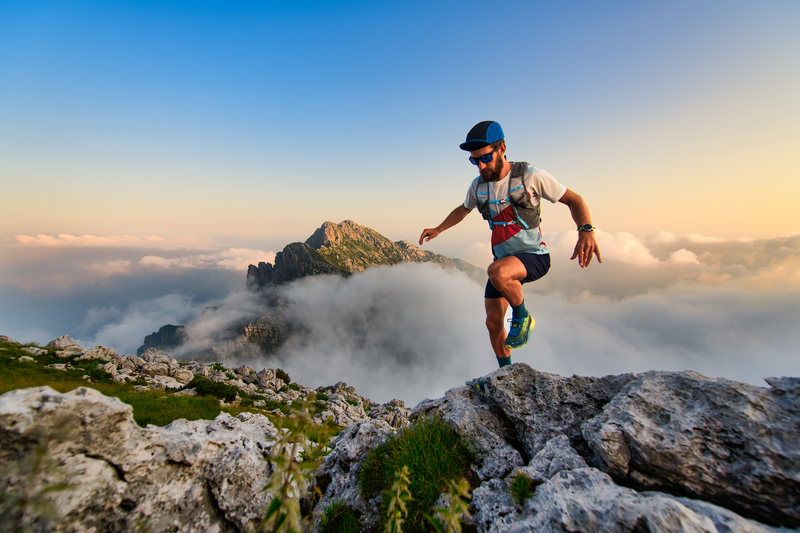
94% of researchers rate our articles as excellent or good
Learn more about the work of our research integrity team to safeguard the quality of each article we publish.
Find out more
ORIGINAL RESEARCH article
Front. Pharmacol. , 04 February 2019
Sec. Respiratory Pharmacology
Volume 10 - 2019 | https://doi.org/10.3389/fphar.2019.00062
This article is part of the Research Topic Drug Prevention and Control of Ventilator-Associated Pneumonia View all 9 articles
A commentary has been posted on this article:
Commentary: Description of Clinical Characteristics of VAP Patients in MIMIC Database
Background: Ventilator-associated pneumonia (VAP) is a common and serious nosocomial infection of intensive-care units (ICUs). Accurate, timely diagnosis enables early VAP patients to receive appropriate therapies and reduce the occurrence of complication. However, so far clinical datas regarding the epidemiology and mortality of VAP are still limited. Medical Information Mart for Intensive Care (MIMIC) database is a free, open and public resource about ICU research database. MIMIC database is a free, open, public database that collects information on more than 40,000 ICU patients who are predominantly white people. Therefore, the purpose of the present study is to observe and describe the clinical characteristics of VAP patients in ICU from the MIMIC database.
Method: A total of 418 patients were enrolled in the study. General information, ventilator use information, microbiology information, antibiotic use information, and some nursing-related information were extracted to describe and analyze the clinical features of VAP patients.
Results: The results of the study showed that patients with one or three pathogens were the most. The main pathogens were YEAST (16.71%), STAPH AUREUS COAG+ (11.63%), Staphylococcus, COAGULASE NEGATIVE (8.68%), GRAM NEGATIVE ROD (S) (6.14%), and Pseudomonas aeruginosa (5.73%). Patients using 4 antibiotics were the most. The top five antibiotics in the largest proportion were synthetic antibacterials (24.66%), peptides (20.13%), cephalosporins (19.60%), penicillins (13.54%), and aminoglycosides (5.27%).
Conclusion: This study summarizes the common pathogens of VAP and the antibiotics commonly used in the treatment of VAP by describing the clinical information of 418 patients with VAP in the MIMIC database. In clinical treatment, we should pay attention to aseptic operation, develop appropriate antibacterial measures, closely monitor the pathogens of VAP infection, and use antibiotics in a timely manner to control the occurrence and development of VAP.
Ventilator-associated pneumonia (VAP) is a common and serious nosocomial infection of intensive-care units (ICUs) (Legras et al., 1998; Urli et al., 2002). The incidence of VAP is 15.6% worldwide, among which the highest incidence is 19.4% in Europe, followed by 16.0% in Asia Pacific, 13.8% in Latin America, and 13.5% in the United States (Kollef et al., 2014). The occurrence of VAP not only prolongs ventilatory support, but also prolongs the stays in ICU and hospital, which would increase healthcare cost and result in a poorer prognosis (Kollef et al., 2012; Craven et al., 2013; Mathai et al., 2015).
Antibiotics are important means for the treatment of VAP. As it is previously reported, early VAP is associated with more antibiotic-sensitive microorganisms, while resistant strains are frequently observed with late-onset VAP (Tseng et al., 2012). Because of the emergence of resistant strains, VAP remains a major cause of morbidity and mortality among critically ill patients and incurs excessive medical expenses for institutions. Accurate, timely diagnosis enables early VAP patients to receive appropriate therapies and reduce the occurrence of complication. However, so far clinical datas regarding the epidemiology and mortality of VAP are still limited (Yue et al., 2015).
Medical Information Mart for Intensive Care (MIMIC) database is a free, open and public resource about ICU research database. MIMIC database was published in 2006 by the computational physiology laboratory of the Massachusetts Institute of Technology (MIT), the Beth Israel Dikang Medical Center (BIDMC) and Philips Medical Center. It has attracted more and more researchers from academia and industry to utilize the database for medical research (Dejam et al., 2014; Lee et al., 2015). Therefore, the purpose of the present study is to observe and describe the clinical characteristics of VAP patients in ICU from the MIMIC database.
Funded by the National Institutes of Health (NIH), the MIMIC database was jointly published by the Computational Physiology Laboratory of MIT, Beth Israel Dikon Medical Center, and Philips Medical, it Collected and sorted out the clinical diagnosis and treatment information on more than 40,000 real patients who are predominantly white people living in the intensive care unit of the Beacon Israel Dikang Medical Center from 2001 to 2012 (Saeed et al., 2011). The database has well solved the current situation of clinical medical workers suffering from the scientific research without a large number of systematic clinical diagnosis and treatment data. The MIMIC database is constantly updated and the latest version is currently MIMIC-III v1.4 (Zhang, 2015; Johnson et al., 2016). This study mainly builds the library and extracts the information data of relevant patients from the MIMIC database through PostgreSQL software (version 9.6).
We extracted the hadm_id of 564 person-times inpatients with VAP diagnosis from the MIMIC database by using of icd9 code (99731: Ventilator associated pneumonia). To ensure the association of cases with VAP, we searched for VAP-related keywords (VAP or “ventilator-associated pneumonia” or “venting-associated pneumonia”) in the noteevents of these cases, the number of cases with keywords was 418 person-times (407 patients, 9 of whom were hospitalized 2 times, and 1 was hospitalized 3 times). The final search was performed on November 2, 2018. These 418-person-time patients are the subject of our research. Taking hadm_id of 418 person-times as index, we extracted the patient’s general information, ventilator use information, microbiology information, antibiotic use information, and some nursing related information from the corresponding tables to describe and analyze the clinical characteristics of VAP patients. The project was approved by the institutional review boards of the MIT and Beth Israel Deaconess Medical Center (BIDMC) and was granted a waiver of informed consent.
Continuous variables were expressed as mean ± SD, the distribution of data was represented by pie charts, and the specific states of different indicators were displayed in a bar chart.
Basic situation of MIMIC-III database: The clinical data were derived from the Internal Medicine ICU (MICU), Cardiac Surgery ICU (CSRU), Surgical ICU (SICU), Cardiology ICU (CCU), Trauma Surgery ICU (TSICU), and Neonatal ICU (NICU), a total of 26 tables including patient demographics, laboratory test results, drug use records, medical records, and information on out-of-hospital deaths, physiological waveforms, etc., such as: ADMISSIONS, CHARTEVENTS, D-ITEMS, DIAGNOSES_ICD, etc.
A total of 418 patients were included in the study. Table 1 summarized the basic characteristics of males and females in baseline data. The average age of male patients was 58.22 years and that of female patients was 64.63 years. The proportion of white individuals was the highest among males and females, followed by blacks. In terms of marital status, the proportion of married people was the highest, followed by singles, same situation for both males and females. In terms of insurance, there were a total of five types of insurance among these patients. The proportion of these insurances, in descending order: Medicare, Private, Medicaid, Government, and Self Pay. In terms of oral care, the head of a bed up, parenteral nutrition, and Sputum suction, the proportion of patients who have not taken measures were larger. The average using time for ventilator was 7.59 h of male patients and was 6.67 h of females. Both males and females had a highest proportion of survival.
Figure 1 summarized the number of pathogenic bacteria carried in each patient. There were 82 patients carried only one pathogen, 76 patients carried two pathogens, and 82 carried three pathogens. One patient carried 15 pathogens, more than any single patient. Figure 2 summarized the proportion of various pathogens in these VAP patients. The top five pathogens with the highest proportion, in descending order: YEAST (16.71%), STAPH AUREUS COAG+ (11.63%), Staphylococcus, COAGULASE NEGATIVE (8.68%), GRAM NEGATIVE ROD (S) (6.14%), and Pseudomonas aeruginosa (5.73%), the rest were other pathogenic bacteria (52.83%).
Figure 1. Distribution of number of pathogenic bacteria in each VAP patients in intensive care medical database III (MIMIC-III).
Figure 2. Distribution of bacterial species in VAP patients in intensive care medical database III (MIMIC-III).
Figure 3 summarized the number of antibiotics used in each patient. Four people used only one antibiotic, and one person used 19 antibiotics, which was the patient with the highest number of antibiotics. The largest number of patients used 5 antibiotics, a total of 83 people.
Figure 3. Distribution of number of antibiotics in each VAP patient in intensive care medical database III (MIMIC-III).
Figure 4 summarized the categories of antibiotic used by each patient. Four people used only one categories of antibiotics, and one used 10 categories of antibiotics, which was the patient with the most categories of antibiotics. The largest number of patients used 4 categories of antibiotics, a total of 122 people. Figure 5 summarized the proportion of each categorie of antibiotic in these VAP patients. The top five antibiotics in the largest proportion were, in order, synthetic antibacterials (24.66%), peptides (20.13%), cephalosporins (19.60%), penicillins (13.54%), and aminoglycosides (5.27%), the rest were other antibiotics (16.80%), mainly including antifungal drugs, carbapenems, macrolides, β-lactams, antituberculosis drugs and anti-leprosy drugs, lincomycin, clindamycin, and tetracyclines.
Figure 4. Distribution of categories of antibiotics in each VAP patient in intensive care medical database III (MIMIC-III).
Figure 5. Distribution of categories of antibiotics in VAP patients in intensive care medical database III (MIMIC-III).
In this study, the basic information and medication status of patients with VAP were extracted and described in the latest version of the multi-parameter intensive care database MIMIC-III. The MIMIC-III database provides relevant data reference for clinicians and engineers who want to solve clinical research problems. This study is mainly to describe the bacterial infection and antibiotic use in patients with VAP. The results of the study showed that patients with one or three pathogens were the most. The main pathogens were YEAST, STAPH AUREUS COAG+, Staphylococcus, COAGULASE NEGATIVE, GRAM NEGATIVE ROD (S) and Pseudomonas aeruginosa. Patients using 4 antibiotics were the most. The top five antibiotics in the largest proportion were synthetic antibacterials (24.66%), peptides (20.13%), cephalosporins (19.60%), penicillins (13.54%), and aminoglycosides (5.27%).
Mechanical ventilation is an important measure to rescue critically ill patients. VAP is a common complication in mechanical ventilation and a special type of hospital-acquired pneumonia. Patients admitted to the ICU are critically ill and have low immunity. Various invasive procedures create conditions for bacterial infection. In order to prevent the occurrence of stress ulcers, severe patients often use antacids to increase the pH level of gastric juice and create conditions for bacterial growth. Mechanical ventilation destroys the defense barrier of the respiratory tract, making it easy for exogenous bacteria and pathogenic bacteria in the body to enter the bronchial and lung tissues. This makes the lungs susceptible to infection by pathogenic bacteria.
Toxins and invasive enzymes secreted by these bacteria can destroy the body’s normal immune activity by damaging human white blood cells and macrophages, leading to VAP (Kradin and Digumarthy, 2017). The common pathogens of VAP are STAPH AUREUS COAG+, Staphylococcus, GRAM NEGATIVE ROD (S), and Pseudomonas aeruginosa (Djordjevic et al., 2017), which are consistent with the results of our study.
The occurrence of VAP in ICU patients is responsible for greater morbidity, prolonged ICU stay, and exceeded medical expenses (Vandana Kalwaje and Rello, 2018). On a per case basis, case VAP is associated with additional hospital costs of approximately US$ 40,000 (Warren et al., 2003; Zimlichman et al., 2013). Based on the timing of onset, VAP can be divided into early-onset VAP, occurring within4 to 5 days after intubation and mainly caused by community pathogens with a favorable pattern of antibiotic sensitivity (e.g., Haemophilus influenzae, Streptococcus pneumoniae, and anaerobes of the oral cavity), and late-onset VAP, often caused by multidrug-resistant (MDR) pathogens (e.g., Staphylococcus aureus, Pseudomonas aeruginosa, Acinetobacter baumannii, and Enterobacteriaceae), which were selected by exposure to broad-spectrum antibiotics (Giard et al., 2008; Shorr et al., 2009).
Antibiotics are the most important means of preventing and treating VAP, so it is important to determine the early diagnosis of VAP and early antibiotic treatment. However, early diagnosis of VAP has become difficult due to the early lack of microbiological analysis and highly specific radiological signs of VAP (Bassetti et al., 2012). Therefore, the adequacy of empirical antibacterial therapy in the early stage is highly predictive of hospital survival (Agrafiotis et al., 2011). For patients with VAP who have a microbiologically determined diagnosis, appropriate broad-spectrum antibiotics must be administered immediately. In our study, a total of 418 hospitalizations of 407 VAP patients in MIMIC database were analyzed. These VAP patients received a total of 13 antibiotic treatments, and the top five antibiotics with the highest frequency were synthetic antibacterials (24.66%), peptides (20.13%), cephalosporins (19.60%), penicillins (13.54%), and aminoglycosides (5.27%), others were followed by antifungal agents (5.19%), carbapenems (4.82%), macrolides (3.42%), beta lactams (1.24%), antituberculosis drugs and anti-leprosy drugs (1.24%), lincomycin and clindamycin (0.49%), and tetracyclines (0.37%). These antibiotics kill bacteria by destroying their cell walls or cell membranes (Běhal, 2002). The results of this study directly reflect the antibiotic use of VAP patients in the MIMIC-III database.
The MIMIC-III database is the first large ICU database that is open to the public free of charge. It has a real large data set with rich types of medical data, providing high-quality data resources for clinical research, mining and building a knowledge base. Effectively assist clinical decision making. This study was subject to some limitations: First, we extract data from MIMIC data, which is a retrospective study with selective bias. Second, this study mainly involved white people, blacks and Asians are very few. Due to ethnic differences, the results of this study need to be further verified in other ethnic groups. Third, we did not conduct influencing factor analysis and predictive analysis for this disease. Influencing factors analysis and predictive analysis can better remind people how to prevent the disease from onset; predict the progression of the disease according to the patient’s condition and basic physiological characteristics, provide patients with better prognosis and rational allocation of medical resources. This will be the main direction of our next research.
This study summarizes the common pathogens of VAP and the antibiotics commonly used in the treatment of VAP by describing the clinical information of 418 patients with VAP in the MIMIC database. In clinical treatment, we should pay attention to aseptic operation, develop appropriate antibacterial measures, closely monitor the pathogens of VAP infection, and use antibiotics in a timely manner to control the occurrence and development of VAP.
All authors listed have made a substantial, direct and intellectual contribution to the work, and approved it for publication.
This study was supported by the National Social Science Foundation of China (No. 16BGL183).
The authors declare that the research was conducted in the absence of any commercial or financial relationships that could be construed as a potential conflict of interest.
Agrafiotis, M., Siempos, I. I., Ntaidou, T. K., and Falagas, M. E. (2011). Attributable mortality of ventilator-associated pneumonia: a meta-analysis. Int. J. Tuberc. Lung Dis. 15, 1154–1163. doi: 10.5588/ijtld.10.0498
Bassetti, M., Taramasso, L., Giacobbe, D. R., and Pelosi, P. (2012). Management of ventilator-associated pneumonia: epidemiology, diagnosis and antimicrobial therapy. Expert Rev. Anti Infect. Ther. 10, 585–596. doi: 10.1586/eri.12.36
Běhal, V. (2002). Antibiotics. Biotechnol. Annu. Rev. 8, 227–265. doi: 10.1016/S1387-2656(02)08010-9
Craven, D. E., Lei, Y., Ruthazer, R., Sarwar, A., and Hudcova, J. (2013). Incidence and outcomes of ventilator-associated tracheobronchitis and pneumonia. Am. J. Med. 126, 542–549. doi: 10.1016/j.amjmed.2012.12.012
Dejam, A., Malley, B. E., Feng, M., Cismondi, F., Park, S., Samani, S., et al. (2014). The effect of age and clinical circumstances on the outcome of red blood cell transfusion in critically ill patients. Crit. Care 18:487. doi: 10.1186/s13054-014-0487-z
Djordjevic, Z. M., Folic, M. M., and Jankovic, S. M. (2017). Distribution and antibiotic susceptibility of pathogens isolated from adults with hospital-acquired and ventilator-associated pneumonia in intensive care unit. J. Infect. Public Health 10, 740–744. doi: 10.1016/j.jiph.2016.11.016
Giard, M., Lepape, A., Allaouchiche, B., Guerin, C., Lehot, J. J., Robert, M. O., et al. (2008). Early- and late-onset ventilator-associated pneumonia acquired in the intensive care unit: comparison of risk factors. J. Crit. Care 23, 27–33. doi: 10.1016/j.jcrc.2007.08.005
Johnson, A. E., Pollard, T. J., Shen, L., Lehman, L. W., Feng, M., Ghassemi, M., et al. (2016). MIMIC-III, a freely accessible critical care database. Sci. Data 3:160035. doi: 10.1038/sdata.2016.35
Kollef, M. H., Chastre, J., Fagon, J. Y., François, B., Niederman, M. S., Rello, J., et al. (2014). Global prospective epidemiologic and surveillance study of ventilator-associated pneumonia due to Pseudomonas aeruginosa. Crit. Care Med. 42, 2178–2187. doi: 10.1097/CCM.0000000000000510
Kollef, M. H., Hamilton, C. W., and Ernst, F. R. (2012). Economic impact of ventilator-associated pneumonia in a large matched cohort. Infect. Control Hosp. Epidemiol. 33, 250–256. doi: 10.1086/664049
Kradin, R. L., and Digumarthy, S. (2017). The pathology of pulmonary bacterial infection. Semin. Diagn. Pathol. 34, 498–509. doi: 10.1053/j.semdp.2017.06.001
Lee, J., de Louw, E., Niemi, M., Nelson, R., Mark, R. G., Celi, L. A., et al. (2015). Association between fluid balance and survival in critically ill patients. J. Intern. Med. 277, 468–477. doi: 10.1111/joim.12274
Legras, A., Malvy, D., Quinioux, A. I., Villers, D., Bouachour, G., Robert, R., et al. (1998). Nosocomial infections: prospective survey of incidence in five French intensive care units. Intensiv. Care Med. 24, 1040–1046. doi: 10.1007/s001340050713
Mathai, A. S., Phillips, A., Kaur, P., and Isaac, R. (2015). Incidence and attributable costs of ventilator-associated pneumonia (VAP) in a tertiary-level intensive care unit (ICU) in northern India. J. Infect. Public Health 8, 127–135. doi: 10.1016/j.jiph.2014.07.005
Saeed, M., Villarroel, M., Reisner, A. T., Clifford, G., Lehman, L. W., Moody, G., et al. (2011). Multiparameter intelligent monitoring in intensive care II: a public-access intensive care unit database. Crit. Care Med. 39, 952–960. doi: 10.1097/CCM.0b013e31820a92c6
Shorr, A. F., Zilberberg, M. D., and Kollef, M. (2009). Cost-effectiveness analysis of a silver-coated endotracheal tube to reduce the incidence of ventilator-associated pneumonia. Infect. Control Hosp. Epidemiol. 30, 759–763. doi: 10.1086/599005
Tseng, C. C., Huang, K. T., Chen, Y. C., Wang, C. C., Liu, S. F., Tu, M. L., et al. (2012). Factors predicting ventilator dependence in patients with ventilator-associated pneumonia. ScientificWorldJournal 2012:1. doi: 10.1100/2012/547241
Urli, T., Perone, G., Acquarolo, A., Zappa, S., Antonini, B., and Candiani, A. (2002). Surveillance of infections acquired in intensive care: usefulness in clinical practice. J. Hosp. Infect. 52, 130–135. doi: 10.1053/jhin.2002.1271
Vandana Kalwaje, E., and Rello, J. (2018). Management of ventilator-associated pneumonia: need for a personalized approach. Expert Rev. Anti Infect. Ther. 16, 641–653. doi: 10.1080/14787210.2018.1500899
Warren, D. K., Shukla, S. J., Olsen, M. A., Kollef, M. H., Hollenbeak, C. S., Cox, M. J., et al. (2003). Outcome and attributable cost of ventilator-associated pneumonia among intensive care unit patients in a suburban medical center. Crit. Care Med. 31, 1312–1317. doi: 10.1097/01.ccm.0000063087.93157.06
Yue, M., Liu, F., Zhao, L., Zhang, F., and Wang, C. (2015). A multicenter clinical study of bundle treatment for moderate or severe acute respiratory distress syndrome. Zhonghua Wei Zhong Bing Ji Jiu Yi Xue 27, 601–605. doi: 10.3760/cma.j.issn.2095-4352.2015.07.012
Zhang, Z. (2015). Accessing critical care big data: a step by step approach. J. Thorac. Dis. 7, 238–242. doi: 10.3978/j.issn.2072-1439.2015.02.14
Keywords: ventilator-associated pneumonia, VAP, antibiotic, pathogenic bacteria, MIMIC database
Citation: Liu Q, Yang J, Zhang J, Zhao F, Feng X, Wang X and Lyu J (2019) Description of Clinical Characteristics of VAP Patients in MIMIC Database. Front. Pharmacol. 10:62. doi: 10.3389/fphar.2019.00062
Received: 21 November 2018; Accepted: 18 January 2019;
Published: 04 February 2019.
Edited by:
Xian-Tao Zeng, Wuhan University, ChinaCopyright © 2019 Liu, Yang, Zhang, Zhao, Feng, Wang and Lyu. This is an open-access article distributed under the terms of the Creative Commons Attribution License (CC BY). The use, distribution or reproduction in other forums is permitted, provided the original author(s) and the copyright owner(s) are credited and that the original publication in this journal is cited, in accordance with accepted academic practice. No use, distribution or reproduction is permitted which does not comply with these terms.
*Correspondence: Xue Wang, d2FuZ3h1ZWpkX2pkXzIwMDhAMTI2LmNvbQ== Jun Lyu, bHVqdW4yMDA2QHhqdHUuZWR1LmNu
†These authors have contributed equally to this work
Disclaimer: All claims expressed in this article are solely those of the authors and do not necessarily represent those of their affiliated organizations, or those of the publisher, the editors and the reviewers. Any product that may be evaluated in this article or claim that may be made by its manufacturer is not guaranteed or endorsed by the publisher.
Research integrity at Frontiers
Learn more about the work of our research integrity team to safeguard the quality of each article we publish.