- 1Department of Emergency and Critical Care Medicine, Shanghai Tenth People's Hospital, Tongji University, Shanghai, China
- 2Department of Experimental and Clinical Pharmacology, College of Pharmacy, University of Minnesota, Minneapolis, MN, United States
In the intensive care unit (ICU) setting, where highly variable and insufficient drug efficacies, as well as frequent and unpredictable adverse drug reactions (ADRs) occur, pharmacogenomics (PGx) offers an opportunity to improve health outcomes. However, PGx has not been fully evaluated in the ICU, partly due to lack of knowledge of how genetic markers may affect drug therapy. To fill in this gap, we conducted a review to summarize the PGx information for the medications commonly encountered in the ICU.
Introduction
Patients in the intensive care unit (ICU) often have multiple serious medical conditions. The critical and complex medical conditions necessitate patients' admission to ICU. Pharmacotherapy is a key component of critical care medicine. ICU patients are vulnerable and cannot afford the comorbidities due to a drug treatment failure. The challenge of pharmacotherapy in the ICU is that patients may concurrently receive the medical treatment of multiple sedative-analgesics, antibiotics, antifungal drugs, anticoagulants, antiarrhythmics, and sometimes paralytics as well as medications to inhibit gastric acid secretion. Critical illness combined with preexisting chronic diseases often results in hepatic or renal insufficiency and, as a consequence, alterations in patient body's disposition and response to drugs. Therefore, the choice of right medication at the right dose is challenging, but essential, to avoid severe and unexpected toxicities and to ensure therapeutic efficacy. Traditional ICU decision-making strategies for treatments depend on the experience of critical care providers, individual patient characteristics and environmental influences. Despite of these efforts, treatment effectiveness varies among ICU patients. The incidence of adverse drug reactions (ADRs) in ICU is much higher than that in other clinical environments (Grenouillet-Delacre et al., 2007; Joshua et al., 2009). Additionally, the expense of pharmaceuticals in the critical care environment is huge. Not only intensive care clinicians but also the pharmaceutical industry are seeking predictors to avoid the risk of ADRs, predict response and reduce the costs of pharmaceuticals in the ICU (Empey, 2010; Patterson et al., 2011). With the emergence and development of human genome sequencing technologies and high throughput genetic analysis, it has been established that genetic variants play a role in an individual's response to drugs. Pharmacogenomics (PGx), a relatively young research field, which aims at deciphering the relationship between individuals' genetic makeup and medication responses, has yield promising results by allowing the prediction of drug responses and the prevention of ADRs, using patient genetic information (Carr and Pirmohamed, 2018). Taking together, incorporating PGx into clinical decision-making in the ICU may improve the patient treatment outcomes significantly (Su et al., 2014; MacKenzie and Hall, 2017). Indeed, PGx has been investigated in a variety of therapeutic areas: cancer, cardiovascular disease, depression, and human immunodeficiency virus infection as well as rheumatologic disorders. The majority of the drugs that are labeled with PGx information by the U.S. Food and Drug Administration (FDA) are used in oncology, cardiology or psychiatry. Considerable PGx research has been conducted in the anesthesia literature (Behrooz, 2015). This data suggests that specific pharmacokinetic genotype screening could personalize the treatment of analgesics used in the ICU (Landau et al., 2008). The potential utility of applying the PGx approach to guide the selection and dosage of drugs for patients who are acutely ill has a promising future.
However, the PGx research has mainly focused on subacute or chronic illnesses. The ICU setting has not been chosen for conducting PGx research or implementation due to a number of challenges, for example, the lack of knowledge on how PGx markers may affect drug therapy in the ICU setting. The complex medical problems seen in the ICU require well-designed studies with adequate power to account for multiple confounding factors. To facilitate the evaluation of PGx in the ICU and to fill in the knowledge gap, we conducted a review of the PGx information for medications that are commonly used in the ICU setting. The choice of drugs to be evaluated was based on two criterion: first, the medications are frequently utilized in the ICU. Secondly, there needs to be substantial literature support of PGx information for these medications, specifically, existence of guidelines, and/or FDA drug labels containing PGx information. Note that the requirement of the second criteria rules out some very commonly used ICU medications, for example, insulin, and hydrocortisone. In addition, we propose several practical steps to encourage PGx research in the ICU.
Pharmacogenomics of Commonly Used ICU Medications
Genetic Terms and Definitions
The Clinical Pharmacogenetics Implementation Consortium (CPIC) is an international consortium with the goal to translate laboratory genetic test information into actionable prescribing suggestions for related medications (Caudle et al., 2014a). The Dutch Pharmacogenetics Working Group (DPWG) was established to develop pharmacogenetics-based therapeutic (dose) recommendations (Swen et al., 2011). These guidelines provide information on the strongest evidence to-date between genetic information and medication usage. They meant to provide support for the usage of PGx information in a specific disease setting. However, none of these guidelines should be viewed as a mandate for a genetic test prior to prescribing medications. CPIC has created standardized terms to be used in their guidelines and in the larger pharmacogenetics community. A variant form of genes is an allele. In star allele nomenclature, alleles are identified through the means of numbers and letters, separated from the gene name by a star. For example: CYP3A5*3 is a typical nonfunctional variant of CYP3A5. CYP3A5*3 identifies the genetic variant at the genomic position 22,893 on chromosome 7 (7q21.1). A variant from A to G creates aberrant splicing of CYP3A5 mRNA, resulting in nonfunctional protein. CYP3A5*3 has a dbSNP number: rs776746. The star allele nomenclature is thought to be faster and easier for non-specialized professionals in identifying important PGx alleles. The star allele nomenclature has been firstly used to identify alleles within the cytochrome P450 (CYP) gene family. The Pharmacogene Variation (PharmVar, at www.PharmVar.org, former at www.cypalleles.ki.se) database is a critical resource for the PGx research. This site provides allele nomenclatures for a collection of very important pharmacogenes (Gaedigk et al., 2018). From that, it spread to all genes that have been studied in PGx (Kalman et al., 2016). The variants of the CYP450 genes (e.g., CYP2D6, CYP2C19, and CYP2C9 genes) were discovered on the basis of the metabolization status variants in medication treated individuals. Caudle et al. worked on the standardization of the PGx terms to capture the phenotypic differences in these gene activities. The phenotypic definitions of ultrarapid metabolizer (UM), rapid metabolizer (RM), extensive metabolizer (EM), intermediate metabolizer (IM), and poor metabolizer (PM) are basing on the amounts and categories of function alleles (increased, normal or decreased). Normal metabolizer (NM) has taken place of EM in CPIC guidelines published after 2017 (Bank et al., 2018). Therefore, for this review as summary sentences, we will use UM, RM, EM/NM, IM, and PM to depict phenotypes of different CYP450 enzymes. The exact definition of genetic effect grouping for each gene can be found in the CPIC term standardization project (Caudle et al., 2017).
Commonly Used ICU Drugs
To summarize the PGx information for the commonly encountered drugs in ICU, we grouped the drugs into five categories: analgesics and sedatives, antifungal drugs, cardiovascular drugs, gastroenterological drugs, and anticonvulsant drugs. Only those genes that have been confirmed to relate to more than one commonly prescribed ICU drugs are listed in Table 1. According to the CPIC guidelines, we develop clinically actionable flow charts (Figure 1) to facility the adaptation of genomic information in clinical decision-making (Caudle et al., 2016).
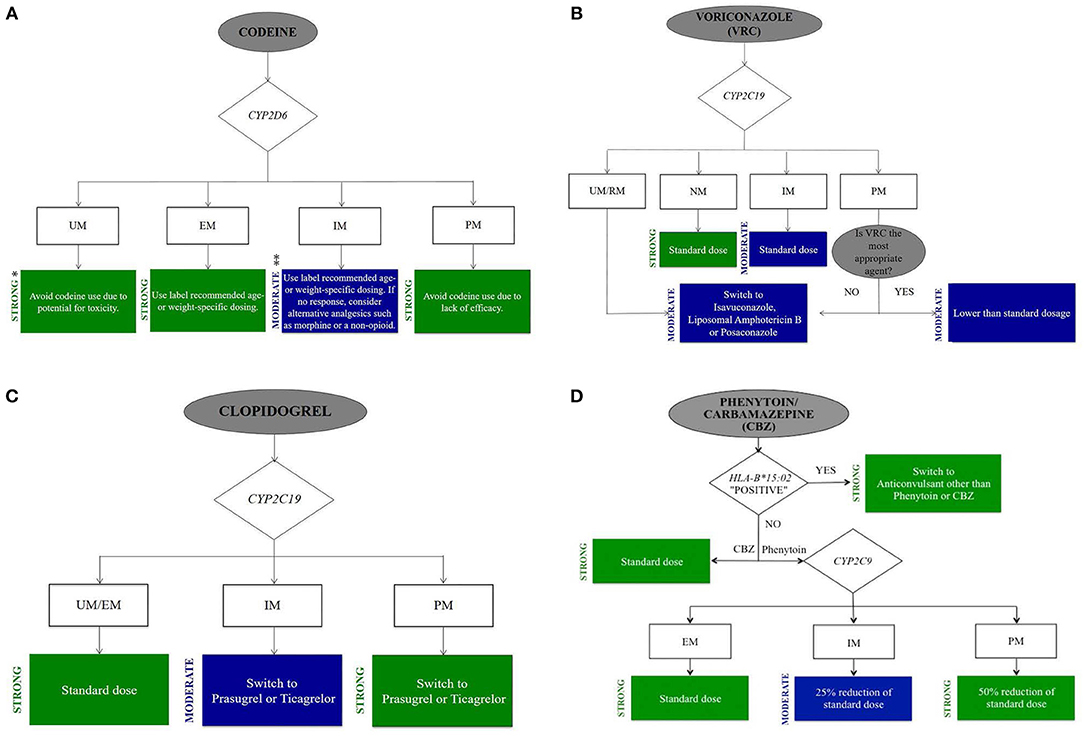
Figure 1. Clinical actionable flow chart of drugs commonly used in ICU. (A) Codeine. (B) Voriconazole. (C) Clopidogrel. (D) Phenytoin/Carbamazepine. Develop clinical actionable flow chart based on CPIC guidelines to facility the adaptation of genomic information in clinical decision-making in ICU. Evidence-based recommendation taken from the online CPIC: Strength of Recommendations, https://cpicpgx.org/strength-of-recommendations/. * “Strong” means “the evidence is high quality and the desirable effects clearly outweigh the undesirable effects.” ** “Moderate” means “there is a close or uncertain balance as to whether the evidence is high quality and the desirable clearly outweigh the undesirable effects.”
Analgesics and Sedatives
Opioid analgesics
Morphine. Because of the global accessibility and extensive clinical experience, morphine has become one of the most widely used opioids. High interindividual differences in analgesia generated by morphine is well-known and a clinically important challenge. There is increasing evidence that the reaction to morphine can be modulated by pharmacokinetic (PK) and pharmacodynamic (PD) related allelic genetic variants.
Variants affecting morphine metabolism
Two major metabolites of morphine are morphine-6-glucuronide (M6G) and morphine-3-glucuronide (M3G). The uridine diphosphate glucuronosyltransferase (UGT) 2B7 is the metabolizing enzyme (Smith, 2009). Among patients receiving morphine therapy with UGT2B7 variants at position −79, the ratios of M6G/morphine and M3G/morphine reduced compared with non-carriers of this polymorphic change (De Gregori et al., 2016). A recent randomized crossover trial in healthy individuals showed no association between different UGT2B7 variants and morphine PK (Nielsen et al., 2017). Research investigating the effects of UGT2B7 genotypes on morphine glucuronidation activity was limited and the results were inconsistent across different study approaches. It has still not yet been completely clear for what affect the genetic variability has on morphine in the acute pain setting. Thus, it is still unclear for UGT2B7 variants' clinical impact and further investigation is needed.
Variants affecting morphine PD
The analgesic effect of morphine is mainly exerted by binding to μ-opioid receptor in the central nervous system. The μ-opioid receptor is encoded by the OPRM1 gene and the A118G nucleotide substitution is the most commonly researched variant. Studies conducted on cancer and postoperative patients treated with morphine showed that individuals who carrying the homozygote G allele had higher pain scores and required higher doses (Nielsen et al., 2015). Huang et al. conducted a meta-analysis comprising more than 4,000 individuals and showed that those who were GG homozygous required 40% higher postoperative morphine. This was mainly because over 40% of Asian population had the G allele compared to only 15% of the European population did (Hwang et al., 2014). COMT enzyme modulates the neurotransmission of norepinephrine and dopamine. Variability in COMT has been proved to affect the efficacy of morphine. For patients who carried the G/G genotype of COMT (rs4680; p.Val158Met), the morphine dose required on day 1 was higher in comparison with those who carried the A/A and A/G genotypes of COMT (Matsuoka et al., 2017). In clinical practice, variability in COMT was related to morphine's central ADRs such as confusion and hallucinations (Ross et al., 2008). A recent postoperative study showed that compared with single genetic variants, combinations of COMT and OPRM1 variants provided a better explanation of the variability in the metabolism of morphine (De Gregori et al., 2016). Hence, several genetic variants may affect protein function synergistically and multi-gene models will be needed in practice.
Variants affecting morphine transporters
P-gp is an efflux transporter for morphine that is encoded by the ABCB1 gene. An observational study in cancer patients has found that there is an correlation among ABCB1 variants and altered analgesic responses as well as dose requirements (Lötsch et al., 2009). Campa et al. showed that those with C3435T variant had a substantially increased morphine analgesic effect in pain patients (Campa et al., 2008). In 263 pediatric surgical patients undergoing tonsillectomy, there were higher risks of opioid-related respiratory depression and prolonged hospital stays in children with GG and GA genotypes (Sadhasivam et al., 2015).
Fentanyl. Fentanyl, a first-line analgesic in the ICU, is a synthetic opioid analgesic commonly prescribed for its ability to provide both analgesia and sedation. It is the preference analgesic for patients with kidney failure. In adult critically ill patients, variability in response to fentanyl, including PK and PD, was large (Choi et al., 2016).
Variants affecting fentanyl PK
Fentanyl is N-dealkylated by CYP3A4 and CYP3A5 enzymes into the inactive norfentanyl (Feierman and Lasker, 1996). Variations in therapeutic efficacy of fentanyl can be partly explained by PK factors. However, mixed results were discovered for the CYP3A5*3 variants and its effects on PK. Homozygous CYP3A5*3 gave rise to decreased metabolism of fentanyl, so the toxicity might be identified by genotyping CYP3A5*3 variant (Jin et al., 2005). Barratt et al. however, pointed out that only < 2% of the metabolism of fentanyl was associated with the CYP3A4*22 and CYP3A5*3 variants and the plasma concentrations of fentanyl were not affected (Barratt et al., 2014). Jin M et al. indicated that individuals with homozygous CYP3A5*3 have reduced fentanyl clearance. The fentanyl toxicity was likely to reduce by identifying CYP3A4/5 PM alleles (Ahmed et al., 2005).
Variants affecting fentanyl PD
The effect of fentanyl is exerted through the binding with μ-opioid receptor. Variability of pain sensitivity may be affected by genetic variants in opioid receptors (OPRK1, OPRM1, and OPRD1). Within a total of 189 patients receiving midazolam and fentanyl postoperatively, the reduced analgesic effect of fentanyl and shortened time period to recover consciousness were observed to be associated with the OPRM1 118A>G variant (Wu et al., 2009). Due to decreased expression and activity of p-glycoprotein, ABCB1 variant rs1045642 AA was found associated to fentanyl dosage of pediatric critical ill patients (Horvat et al., 2017). However, Klepstad et al. studied 695 cancer patients with the treatment of fentanyl. There was no association observed between opioid requirements and the genetic variants of OPRM1 and ABCB1 (Klepstad et al., 2011).
Tramadol. Tramadol is an opioid analgesic and a 5-HT transporter inhibitor. The O-demethylated metabolite of tramadol is a potent μ-opioid agonist (Barann et al., 2015). If patients are overdosed on tramadol, they will face a major risk of seizures and respiratory depression. Stamer et al. found respiratory depression in one patient treated with tramadol. Genotyping revealed this patient to be a CYP2D6 UM, which resulted in the increased O-desmethyltramadol (Stamer et al., 2008). The generation of O-desmethyltramadol, active metabolite of tramadol, significantly contributes to the drug's activity (Srinivas, 2015). Tramadol PK are characterized by large interindividual variability, which is partially attributed to variants in CYP2D6. CYP2D6 PMs have lower median plasma area under the curve (AUC) of O-desmethyltramadol in comparison to CYP2D6 NMs (Stamer et al., 2007). As compared with CYP2D6 NMs, PMs more often had reduced analgesia in response to tramadol (Kirchheiner et al., 2008; Xu et al., 2014). The guideline from DPWG recommends PMs to “receive an alternative drug (not oxycodone or codeine) or monitor the clinical symptom of pain relief.” For IMs, the DPWG recommends to “monitor closely the reduced tramadol efficacy and to consider raising the dose of tramadol.” If the reaction is still insufficient, it is recommended to “use an alternative drug (not oxycodone or codeine).” For CYP2D6 UMs, the DPWG recommends, “an alternative drug for tramadol (not oxycodone or codeine) or to decrease the dose 30% and monitor closely for potential ADRs” (Stamer et al., 2007).
Codeine. Codeine is an opioid analgesic to treat mild to moderate pain. A potentially serious ADR of codeine is respiratory depression. Codeine is bioactivated to morphine by the hepatic CYP2D6; thus, codeine's efficacy and safety is dependent on the activity of CYP2D6. PK/PD researchers showed a reduction in morphine concentrations and analgesic effect of codeine in CYP2D6 PMs compared with NMs (Poulsen et al., 1996). In contrast, PK tests of codeine indicated that there were more conversions to morphine within CYP2D6 UMs in comparison with NMs, which leaded to toxic systemic morphine concentrations even when the doses of codeine were low (Kirchheiner et al., 2007). The black box warning of FDA approved drug labeling states that, “respiratory depression and death have occurred in children who received codeine following a tonsillectomy and/or adenoidectomy and who had evidence of being CYP2D6 UMs” (U.S Food and Drug Administration, 2018a). The 2014 CPIC guideline for codeine therapy recommends that for patients who carrying the CYP2D6 PM or UM alleles, “alternative analgesics for codeine should be used.” For IMs, the CPIC guideline recommends, “specific doses based on age or weight.” Alternative analgesics (morphine or a non-opioid) are recommended to non-responders (Figure 1A) (Crews et al., 2014). DPWG guideline recommends alternative analgesics for PMs, IMs, or UMs (Swen et al., 2011).
Oxycodone. Two main metabolites of oxycodone are active oxymorphone and inactive noroxycodone metabolized by CYP2D6 enzyme and CYP3A4 enzyme, respectively. A decrease in the activity of CYP2D6 enzyme reduced the conversion of oxycodone to active oxymorphone (Kummer et al., 2011). The recommendation of the DPWG is to “use an alternative to oxycodone (not codeine or tramadol) in CYP2D6 PMs and IMs or remain constantly alert to deficient relief of pain.” In the case of CYP2D6 UM, “a substitute to oxycodone (not codeine or tramadol) should be used or remain constantly alert to potential ADRs” (de Leon et al., 2003).
Sedatives
Propofol. Propofol is an commonly used anesthetics and sedatives in the ICU. It is glucuronidated and hydroxylated to its inactive metabolite. CYP2B6 enzyme takes the major responsibility for the individuals' variability in hydroxylation rate (Court et al., 2001). Mourão et al. showed that the CYP2B6 c.516G>T genetic variant decreased the metabolism of propofol and accounted for ~7% of the propofol dose variation (Mourão et al., 2016). Mastrogianni et al. indicated that the same CYP2B6 polymorphism was associated with increased propofol concentrations in Greek women (Mastrogianni et al., 2014). The findings of these studies showed that the genetic variations associated with propofol metabolism could improve the prediction of therapeutic response to propofol.
Midazolam. Midazolam is commonly used benzodiazepine for sedation in the emergency departments and ICU. Midazolam is mainly metabolized by CYP3A4/3A5 enzyme to the active metabolite, alpha 1-hydroxymidazolam (Spina and Ensom, 2007). Variability in midazolam metabolism may partly due to genetic variants of the CYP3A alleles (Lin et al., 2002). Individuals with CYP3A5*1/*3 had a higher activity of midazolam hydroxylation in comparison with those who carrying CYP3A5*3/*3 variant (Kuehl et al., 2001). Wong et al. indicated that it was 1.5 times higher of the midazolam clearance in the patients with CYP3A5*1/*3 variant than that in patients with CYP3A5*3/*3 variant (Wong et al., 2004).
Lorazepam. Lorazepam is a benzodiazepine derivative with antianxiety and sedative-hypnotic properties. Lorazepam is mainly cleared by direct glucuronidation (Ameer and Greenblatt, 1981). UGTs primarily facilitate glucuronidation. The major drug substrate for UGT2B15 is lorazepam. Healthy individuals with the UGT2B15*2/*2 genotype had 0.58-fold lower clearance of lorazepam in comparison to UGT2B15*1/*1 genotype carriers, which suggested that the UGT2B15*2 variant might be an important determinant of interindividual PK (Chung et al., 2005).
Diazepam. Diazepam is another sedative of the benzodiazepine family that may be used in the ICU for sedation or anticonvulsant treatment. CYP 3A4 and 2C19 metabolize diazepam into its active metabolites, most importantly desmethyldiazepam (Calcaterra and Barrow, 2014). The marked interindividual variability in diazepam clearance is thought to be partially attributable to CYP2C19 genetic variation. Bertilsson et al. indicated that compared with CYP2C19 PMs, NMs demonstrated higher clearance and shorter elimination half-life (t1/2) of diazepam (Bertilsson et al., 1989). Qin et al. pointed out that the elimination time of diazepam and desmethyldiazepam was prolonged in healthy Chinese subjects with mutated CYP2C19 alleles (Qin et al., 1999). FDA approved label of diazepam states that, “the marked interindividual variability in clearance of diazepam is probably attributable to variability of CYP2C19 and CYP3A4” (U.S Food and Drug Administration, 2018b).
Dexmedetomidine. Dexmedetomidine (DEX), used in the ICU to obtain light sedation and analgesia, is an effective α-2 adrenoreceptor agonist. There is marked variability in the efficiency of DEX to get the goal of light sedation, which has led investigators consider genetic variants. The metabolism of DEX is mainly related to the following genes, CYP2A6, α-2 adrenoreceptor genes (ADRA2A, ADRA2B, ADRA2C) and UGT genes (UGT1A4, UGT2B10) (Holliday et al., 2014). CYP2A6 genetic variation did not affect the DEX clearance significantly in ICU patients (Kohli et al., 2012). The ADRA2A C1291G variant was associated with the prolonged time to take effect and the reduced sedation of individuals treated with DEX (Yagar et al., 2011). Although genetic variations may affect patients' response to DEX, additional investigation is required.
Antipsychotics
Haloperidol
Delirium affects up to 80% of patients in ICU and is a risk for death within the next year. Antipsychotics, particularly haloperidol, are the most commonly used drugs for delirium (Young et al., 2010). Haloperidol is metabolized partially by CYP2D6 to the active metabolite reduced haloperidol (Llerena et al., 1992). The DPWG has made recommendations for therapeutic doses of haloperidol basing on CYP2D6 genotype. For CYP2D6 PMs, it is suggested to “reduce the therapeutic dose to 50% for haloperidol or select a substitute for haloperidol, such as fluphenazine, pimozide, quetiapine, flupenthixol, clozapine, or olanzapine” (Swen et al., 2011).
Antifungal Drugs
Voriconazole
Voriconazole is one of the azole antifungal drugs in the treatment of invasive aspergillosis and other severe fungal infections (Patterson et al., 2016). With wide variability among patients in systemic exposure and a narrow therapeutic range, it is not surprising that patient response is unpredictable and ADRs are common (Andes et al., 2009). Voriconazole concentrations have been associated with drug response and ADRs (e.g., hepatotoxicity, visual disturbances and visual hallucinations) (Pascual et al., 2008). The metabolism enzyme of voriconazole is CYP2C19, which is the principal pathway for elimination (Scholz et al., 2009). The doses of voriconazole needed to achieve targeted plasma concentrations increased in patients with the CYP2C19*17 variant which conveys an UM phenotype in comparison with CYP2C19*1/*1 carriers (NMs) (Weigel et al., 2015). Whereas, individuals carrying one or more CYP2C19*2 or *3 variants are IMs or PMs, respectively and may have higher plasma concentrations. DPWG guideline recommends to “monitor serum concentrations for patients carrying the CYP2C19 PM or IM phenotype” (Swen et al., 2011). Evidence for an association between PM phenotype and ADRs with voriconazole was demonstrated in a case report recently (Lemaitre et al., 2013). An alternative agent is recommended to these patients because of the ADRs resulted by the increased voriconazole concentrations. Basing on this evidence, the CPIC guideline for voriconazole states that, “selecting an alternative agent that is not dependent on the metabolism of CYP2C19 in adults with CYP2C19 UMs, RMs or PMs” (Figure 1B) (Moriyama et al., 2016).
Cardiovascular Drugs
Anticoagulant drugs
Warfarin. As an oral anticoagulant used worldwide, warfarin is used to prevent and treat thromboembolic disorders in the ICU. The therapeutic index is narrow and interindividual variability in dose requirements is wide. The length of time to identify the ideal personalized dose can be as long as weeks to months resulting in the increased risk of thromboembolism or bleeding for patients. So warfarin dosing is a proverbial challenge (Kearon et al., 2016). The genes with the strongest literature support in affecting warfarin PK are CYP2C9, CYP4F2, and VKORC1. These genes when combined with clinical factors explain around 50% of dose variability.
Warfarin is a racemic mixture of the R and S stereoisomers and S-warfarin has 3–5 times more effect on inhabitation of VKORC1 than R-warfarin (Choonara et al., 1986). The predominant metabolic pathway of S-warfarin is via the CYP2C9 enzyme. Patients who carry more than one variant CYP2C9 alleles have reduced S-warfarin clearance. Studies in vitro and in vivo showed the metabolism of S-warfarin could be impaired by the presence of CYP2C9*2 by the range of from 30% to 40% and *3 alleles by the range of from 80 to 90% (Lee et al., 2002; Gage et al., 2017). There was a greater risk for patients carrying 1 or 2 copies of CYP2C9*2 or *3 (PMs) to bleed during warfarin therapy in comparison with patients carrying homozygous CYP2C9*1 alleles (NMs). The required doses to achieve similar anticoagulation levels for patients carrying 1 or 2 copies of CYP2C9*2 or *3 (PMs) were lower and the required time for them to meet a stable international normalized ratio (INR) was longer (Pirmohamed et al., 2013).
Warfarin anticoagulation is mediated through inhibition of VKORC1 which results in lower amounts of decreased vitamin K and reduced active clotting factors. As a rate-limiting enzyme, VKORC1 directly influences the efficiency of warfarin. Individuals who carrying one or two−1639A alleles of the VKORC1 gene required lower doses of warfarin than those with−1639G/G alleles (Limdi et al., 2010).
The amount of reduced vitamin K and active clotting factors are decreased due to the transform of reduced vitamin K to hydroxyl-vitamin K1 by CYP4F2 enzyme. The CYP4F2*3 variant (rs2108622; c.1297G>A) was associated with reduced function resulting in approximate 10% increased warfarin dosage in A allele carriers (Gage et al., 2017).
The initial dosing of warfarin varies widely among individuals and it is affected by several clinical factors, including but not limited to race, age, gender, body weight, concomitant medications (e.g., factor Xa inhibitors), comorbidities, diet, as well as genetic factors. In general, the biggest proportion of known dose variability can be explained by VKORC1 and CYP2C9 gene variants. According to the 2017 update of CPIC guideline in regard to the application of PGx tests in dosing of warfarin, it takes more time (>2–4 weeks) for patients who carry reduced function genotypes such as CYP2C9 *1/*3, *2/*2, *2/*3, and *3/*3 to obtain maximum INR than patients without these variants. The VKORC1 variant (c.-1639G>A) profoundly influences the sensitivity of warfarin thereby carriers have lower dose requirements. The CYP4F2 variant is also included in the dosing calculator and has been shown to improve dose predictions (Sun et al., 2016).
Antiplatelet drugs
Clopidogrel. Antiplatelet agent clopidogrel is commonly prescribed for acute coronary syndromes. It is an oral prodrug, activated via hepatic biotransformation to the active metabolite, which prohibits platelet activation and aggregation in a selective and irreversible way. Two oxidative steps involving CYP enzymes are required to form the active metabolite. CYP2C19 is the predominant enzyme. Individuals carrying CYP2C19*2 or *3 alleles (PMs) have reduced active clopidogrel metabolite formation and greater platelet aggregation in comparison with CYP2C19*1 homozygotes (NMs) (Hulot et al., 2011). These low function alleles increase the risk for poor clopidogrel response and the ADRs (Cavallari et al., 2018). The FDA approved clopidogrel black box warning indicates the decreased effectiveness in patients carrying PM alleles (U.S Food and Drug Administration, 2018c). If no contraindication exists, alternative antiplatelet medications (e.g., prasugrel) are recommended to CYP2C19 PMs/IMs by the CPIC dosing guideline for clopidogrel. Individuals homozygous or heterozygous for CYP2C19*17 (UMs) have increased active clopidogrel metabolite formation and decreased platelet aggregation. Dosage of clopidogrel for CYP2C19 UMs is recommended by the CPIC guideline (Figure 1C) (Scott et al., 2013). DPWG guideline recommends “an alternative drug for the CYP2C19 PM and IM phenotypes” (Swen et al., 2011). Clopidogrel also undergoes extensive hydrolytic metabolism to the inactive metabolite by hepatic carboxylesterase 1 (CES1) (Tang et al., 2006). CES1 genetic variation may result in the deficient CES1 hydrolysis activity. The hydrolysis of clopidogrel was marked impaired in patients with the D260fs and G143E CES1 genetic variants (Zhu et al., 2013). Hence, it may be associated with the increased plasma concentrations of active metabolite of clopidogrel.
Antiarrhythmic drugs
Metoprolol. Metoprolol is a beta-blocker often used as an antiarrhythmic. It undergoes α-hydroxylation and O-demethylation as a substrate of CYP2D6 enzyme. CYP2D6 genetic variants have a significant impact on metoprolol PK. CYP2D6 PMs had significantly higher metoprolol plasma concentrations and a prolonged half-life (t1/2) compared to other CYP2D6 phenotypes, despite the same doses of metoprolol administered to PMs and non-PMs (Rau et al., 2009). CYP2D6 PMs also appeared to have increased risk of metoprolol toxicity (Isbister et al., 2016). The DPWG guideline recommends “a reduced dose of metoprolol by 70–75% or an alternative agent, such as bisoprolol or carvedilol to PMs with heart failure.” In PMs with other indications, the DPWG guideline suggests to “remain alert to ADRs, such as cold extremities and bradycardia, or choose alternative drugs, such as bisoprolol and atenolol.” In IMs with heart failure, “a 50% reduction of metoprolol dose or selection of alternative drugs, such as bisoprolol and carvedilol,” is recommended. While in IMs with other indications, the guideline recommends to “remain alert to ADRs or select alternative drugs” (Rau et al., 2009). UMs are recommended to “an alternative agent (e.g., bisoprolol or carvedilol for heart failure, atenolol or bisoprolol for other indications) or titration of the metoprolol dose to 1.5 times higher than the normal dose needed for efficacy” (Goryachkina et al., 2008; Swen et al., 2011).
Carvedilol. Carvedilol is another commonly prescribed beta-blocker in cardiovascular medicine. CYP2D6 is carvedilol's primary metabolizing enzyme. CYP2D6 alleles and carvedilol PK are significantly associated with each other. In healthy volunteers, however, there was no association between the CYP2D6 genotypes and cardiovascular indicators (e.g., blood pressure, heart rate). In the same study, ADRB1 Gly49 was found to be associated with increased carvedilol effects on exercise heart rates (Sehrt et al., 2011). The DPWG guideline recommends that no action is needed for carvedilol and CYP2D6 genotype. For CYP2D6 PMs, DPWG states that, “the plasma concentration of carvedilol can be elevated, but this does not result in an increase of ADRs” (Swen et al., 2011). The FDA-approved drug labeling for carvedilol states that, “plasma concentrations of carvedilol may be higher in CYP2D6 PMs compared to NMs,” but does not discuss altering carvedilol dosing based on a patient's CYP2D6 genotype (U.S Food and Drug Administration, 2017a). No CPIC guideline is available for recommendations.
Propafenone. Propafenone is an antiarrythmic used for the prevention of the recurrence of atrial fibrillation in individuals with episodic atrial fibrillation and no underlying structural heart disease and in the management of paroxysmal supraventricular tachycardia and atrial flutter. The metabolizing enzymes for propafenone are CYP3A4, CYP2D6, and CYP1A2 enzymes. Multiple studies have showed that genetic variants of CYP2D6 gene influence the plasma concentrations of propafenone. Standard doses of propafenone in CYP2D6 PMs may lead to higher plasma parent drug concentrations in comparison with NMs. The drug label approved by FDA for propafenone recommends the dosing regimen is the same for all patients (CYP2D6 PMs and NMs) (Rouini and Afshar, 2017). The DPWG guidelines recommend, “CYP2D6 PMs receive a 70% reduction in initial dose, plus electrocardiogram and plasma concentration monitoring.” For CYP2D6 IMs and UMs, the guideline states that data which calculates the dose adjustment is insufficient and recommends that, “the drug dose adjustment should be based on plasma concentration and the electrocardiogram, or it is encouraged to choose alternative drugs, such as sotalol, quinidine, disopyramide, or amiodarone” (Mörike et al., 2008).
Vasoactive drugs
Vasopressin. Vasopressin is recommended to treat septic shock. More than 10% of patients treated with vasopressors develop life-threatening ADRs, including myocardial infarction, limb and mesenteric ischemia. Anantasit et al. indicated that there were close associations between serious ADRs and a variant near the AVPR1b gene, the AA allele at rs28418396 (Anantasit et al., 2014). No prescribing recommendations based on PGx are available to date.
Statins
Simvastatin. Simvastatin is one of the HMG-CoA reductase inhibitors used to reduce cholesterol. Skeletal muscle toxicity is a rare but a serious statin-related ADR occurring in 1–5% of subjects. OATP1B1, encoded by SLCO1B1 gene, is a hepatic transporter which facilitates statin uptake by the liver (Couvert et al., 2008). SLCO1B1 variants significantly altered the PK of simvastatin (Niemi, 2010). SLCO1B1*5, *15, and *17 genotypes were associated with lower level of plasma clearance of simvastatin. A single coding variant in SLCO1B1, rs4149056T>C, was associated with the higher plasma concentration of simvastatin, and hence the risk of ADRs. Pasanen et al. pointed out that compared to the patients with TT homozygous at rs4149056 in SLCO1B1, the active simvastatin was significantly higher in those with CC homozygous (Pasanen et al., 2006). Nearly 1/4 of the general population is a carrier of C genotype. The variant has also been associated with lower simvastatin adherence presumably due to the development of muscle toxicity (Voora et al., 2009). In the Study of the Effectiveness of Additional Reductions in Cholesterol and Homocysteine (SEARCH), the association between rs4149056 SNP and myopathy was observed. This study also showed an association between this SNP and the cholesterol-lowering effects of simvastatin. Another noncoding SNP, rs4363657, which is in almost complete linkage disequilibrium with rs4149056, was also found to be strongly associated with statin-induced myopathy (Group et al., 2008). The simvastatin CPIC guideline recommends, “the SLCO1B1 genotype be used to identify individuals at greater risk for simvastatin related myopathy while receiving a lower dose of simvastatin (e.g., 40 mg daily).” In individuals heterozygous or homozygous for the C allele, the guideline recommends, “to use lower simvastatin doses or consider alternative statins like pravastatin and rosuvastatin” (Ramsey et al., 2014). A simvastatin drug-drug interaction occurring in individuals with this variant may enhance the risk for myopathy.
Gastroenterological Drugs
Proton pump inhibitors
Proton pump inhibitors (PPIs) are frequently used to patients in the ICU to prevent stress ulcers and treating gastrointestinal bleeding. Up to 20% of patients who received PPIs orally could not achieve ideal acid suppression (Shimatani et al., 2006). More than 80% of the PPIs (e.g., pantoprazole, lansoprazole, omeprazole, esomeprazole, but not rabeprazole) are metabolized by CYP2C19 and CYP3A4 enzyme (Furuta et al., 2005). Genetic variations of the CYP2C19 enzyme may result in differences among PPIs in response. In CYP2C19 NMs treated with PPIs orally, the plasma concentration of PPIs was decreased compared to PMs (Chaudhry et al., 2008). The clearance of lansoprazole, omeprazole, and pantoprazole were higher in CYP2C19 RMs compared to PMs, resulting in decreased plasma concentration of parent (Shin and Kim, 2013). CYP2C19 RM genotype was found the most relevant PGx factor in clinical practice (Shimatani et al., 2006). The DPWG recommends for those with the CYP2C19 UM phenotype, “extra caution during treatment due to a higher risk of insufficient response to omeprazole, esomeprazole, lansoprazole, and pantoprazole.” Further recommendation is to increase the doses by 200, 50–100, 100–200, and 400%, respectively (Swen et al., 2011). The CPIC guideline for CYP2C19 and PPIs (e.g., esomeprazole, omeprazole, etc.) is in progress.
Ondansetron
Ondansetron is prescribed to prevent vomiting and postoperative nausea in the ICU (Apfelbaum et al., 2013). CYP2D6 is the primary metabolizing enzyme of ondansetron (Sanwald et al., 1996). There is a link between the variability in efficacy of ondansetron and the CYP2D6 genotype. The evidence was observed that antiemetic effect of ondansetron was decreased in CYP2D6 UMs treated for chemotherapy-induced and postoperative nausea or vomiting (Stamer et al., 2011). According to the CPIC guideline for ondansetron, if the genotype of CYP2D6 is known, “Alternative 5-HT3 receptor antagonist antiemetics not metabolized by CYP2D6 (e.g., granisetron) should be considered in CYP2D6 UMs” (Bell et al., 2017). Compared with CYP2D6 NMs, the incidence of vomiting in IMs and PMs were the same (Kaiser et al., 2002). There is no dose reduction recommended in FDA labeling. Besides CYP2D6 genetic variants, the ABCB1 gene was implicated in affecting the response to ondansetron (Lehmann et al., 2013; He et al., 2014). 5-HT3 receptor antagonist, such as ondansetron, could prolong the QT interval. There is a fatal risk of polymorphic ventricular tachycardia in the patients with the syndrome of congenital prolongation of the QT interval of the electrocardiogram. Therefore, potentially increased blood levels of ondansetron in CYP2D6 IMs or PMs might be at an even greater risk for QT interval prolongation (Zuo et al., 2014). In this case, ondansetron should not be used in these patients.
Anticonvulsant Drugs
Phenytoin
Phenytoin is an anticonvulsant medication that is used for both focal and generalized status epilepticus. It has a narrow therapeutic index, nonlinear PK and large variability among patients. Phenytoin has several dose and concentration-related ADRs (e.g., neurologic, hypotension and arrhythmias) and initial maintenance dose selection is important. One enzyme involved in the metabolism of phenytoin is CYP2C9, and variant CYP2C9 alleles can impact the concentrations of phenytoin. Clearance rates of phenytoin in individuals with reduced activity CYP2C9 variants may be reduced. Meanwhile, patients with this variant will face a greater risk for dose related side effects (Caudle et al., 2014b). Phenytoin maintenance doses were lower in heterozygous individuals who carried 1 reduced activity allele and lower by 31–52% for individuals who carried 2 reduced activity CYP2C9 alleles compared to CYP2C9*1/*1 (NM) (van der Weide et al., 2001). To avoid excessive blood concentrations, a decrease of 25% in the starting maintenance dose for CYP2C9 IMs (CYP2C9*1/*3 and CYP2C9*1/*2 genotypes) is recommended in the CPIC guideline. For CYP2C9 PMs (CYP2C9*2/*2, CYP2C9*2/*3, CYP2C9*3/*3), a decrease of 50% of starting maintenance dose should be considered (Caudle et al., 2014b). DPWG guideline has the same recommendations with the CPIC guideline that, “to reduce the starting maintenance dose of phenytoin basing on CYP2C9 genotype and monitor serum concentrations and observe closely for ADRs” (Swen et al., 2011).
Variants in the HLA-B gene are exposed to a greater risk of serious phenytoin-induced cutaneous ADRs: toxic epidermal necrolysis (TEN) and Stevens-Johnson syndrome (SJS). The rates of death for SJS and TEN are approximately 5% and 30% respectively; sepsis related to loss of skin integrity is considered to be the critical cause of death (Roujeau and Stern, 1994). The incidence of phenytoin induced SJS and TEN was high in HLA-B*15:02 allele carriers. HLA-B*15:02 allele with high frequency in Han Chinese and other Asians can increase the chance of suffering from SJS/TEN (Hung et al., 2010). HLA-B*15:02 allele is distinct in race and regional distribution. Southeast Asia population has a frequency of HLA-B*15:02 allele as high as >15% (Chung et al., 2004). It is estimated that the frequency of HLA-B*15:02 allele in Yunnan province of China is as high as 36%. There is high risk for these serious cutaneous ADRs in individuals carrying one or two HLA-B*15:02 alleles. The guideline recommends “using an alternative anticonvulsant (not carbamazepine) only when the advantages of treating the disease obviously outweigh the disadvantages” (Figure 1D) (Caudle et al., 2014b). The FDA also makes recommendations that individuals who carrying HLA-B*15:02 allele should avoid to use phenytoin (U.S Food and Drug Administration, 2017b).
Carbamazepine
Carbamazepine is the commonly used antiepileptic drugs in the treatment of generalized and tonic-clonic seizures as well as neuropathic pain. Similar to phenytoin, the most severe manifestations of carbamazepine induced cutaneous ADRs is SJS/TEN. The CPIC guideline and FDA recommend to screen the HLA-B*15:02 variant in the populations at risk before starting carbamazepine (Figure 1D) (Leckband et al., 2013). The risk in the Chinese population is 10 times greater than in Caucasians. It is not recommended for carbamazepine-naive individuals who possess one or two HLA-B*15:02 alleles to take carbamazepine. The frequency of the HLA-A*31:01 variant in Han Chinese populations, Northern European populations and Japanese populations is 2, 2–5, and 9%, respectively (Wen et al., 2008; Schmidt et al., 2009; Ozeki et al., 2011). McCormack et al. found that this allele was significantly associated with carbamazepine-induced hypersensitivity reactions among subjects of Northern European ancestry. The presence of the allele increased the risk from 5.0 to 26.0%, whereas its absence reduced the risk from 5.0 to 3.8% (McCormack et al., 2011).
Valproic acid
Valproic acid is an anticonvulsant drug used in status epilepticus and to treat epilepsy. Valproic acid is metabolized by CYP450 enzymes (CYP2A6, CYP2B6, CYP2C9, and CYP3A5) and also by the UDT enzymes (e.g., UGT1A6, UGT2B7, UGT2B15, etc.) (DeVane, 2003). Valproic acid has a narrow therapeutic range and high interindividual variability in PK and PD (Chadwick, 1985). PGx studies of valproic acid are comparatively few in comparison with that of carbamazepine and phenytoin. A study showed an association between UGT1A6 variants and higher valproic acid maintenance doses as compared with children without variant alleles (Guo et al., 2012). UGT2B7 is also involved in the glucuronidation of valproic acid. Chung et al. found that there was a noteworthy but nonsignificant growth in the AUC of valproate in the patients with increased UGT2B7*2 alleles (Chung et al., 2008). About 6–14% of interindividual variability in valproic acid PK can be explained by CYP2B6 variant alleles, CYP2A6 and CYP2C9 genes. Children who carried the CYP2A6*4, CYP2B6*6, or CYP2C9*3 alleles was likely to obtain high concentrations of valproic acid (Jiang and Wang, 2004).
PGx in the Context of Drug-Drug Interactions
Most ICU patients have multiple medical problems and require treatments with numerous medications, often new to their therapeutic regimens. Five main CYP enzymes (CYP2D6, CYP2C9, CYP2C19, CYP3A4, and CYP1A2) are involved in the metabolism of over 90% of therapeutic drugs (Rendic and Guengerich, 2015). If CYP isoforms is inhibited or induced by one drug, the metabolic status of other drugs will likely be changed in vivo, which can result in decreased therapeutic efficacy or acute drug toxicity (Doucet et al., 2002). Thus, the potential risks of polypharmacy related ADRs resulted from drug-drug interaction are critical in ICU patients (Empey, 2010). For example, voriconazole is an effective inhibitor of CYP enzymes (e.g., CYP2B6, CYP2C9, CYP2C19, and CYP3A) and meanwhile is metabolized by several CYP enzymes (Brüggemann et al., 2009). Combination use of high dose phenytoin or carbamazepine (CYP enzyme inducers) with voriconazole significantly decreased mean AUC and Cmax of voriconazole, potentially reducing its effectiveness (Li et al., 2017). On the other hand, CYP2C19 inhibitors (e.g., cimetidine and omeprazole) may result in greater increases in voriconazole concentrations in patients (Bouatou et al., 2014; Qi et al., 2017). Further, CYP interaction of voriconazole with midazolam caused decreased clearance of midazolam (Saari et al., 2006). Another ICU drug-drug interaction example is between PPIs and clopidogrel, which are commonly co-prescribed in order to minimize antiplatelet treatment-related gastrointestinal bleeding. Some of these PPIs, such as omeprazole, are inhibitors of CYP2C19, so use of PPIs may affect clopidogrel response (Scott et al., 2013). Both concomitant medication of clopidogrel with a high omeprazole dose (80 mg) and taking clopidogrel and omeprazole separated lead to decreases of the plasma concentrations of the active metabolite of clopidogrel compared to clopidogrel administered alone (Angiolillo et al., 2011). These results suggested the metabolic drug-drug interaction between PPIs and clopidogrel.
In addition to drug-drug interaction, our review shows that the effect of a drug can be affected by PGx as well. Therefore, to interpret and subsequently predict a drug effect in the ICU, one needs to consider drug-drug interaction and drug-gene interaction (PGx). For example, metoprolol is metabolized predominantly by CYP2D6, a highly polymorphic gene. Meantime, CYP2D6 can be inhibited by a number of drugs, such as quinidine, fluoxetine, paroxetine, and H1-receptor antagonists. Therefore, the FDA approved drug labeling for metoprolol states that, “CYP2D6 PMs, and EMs who concomitantly use CYP2D6 inhibiting drugs will have increased metoprolol blood levels” (U.S Food and Drug Administration, 2018d). Here, the drug label includes both the genetic effect in CYP2D6 PMs and the drug-drug interaction effect in CYP2D6 EMs. Diphenhydramine is a widely used classic H1-receptor antagonist that has been shown to inhibit the CYP2D6 in vitro (Hamelin et al., 1998). Indeed, diphenhydramine coadministration significantly increased the peak concentration of metoprolol in CYP2D6 EMs (Hamelin et al., 2000). This is just an example where enhanced understanding of PGx in the context of drug-drug interactions can help optimize the dosing of these drugs as well as enable dosage adjustments to prevent toxicities with other drugs.
Discussion
Application of PGx in the ICU
PGx is an evolving field that provides the promise of personalized selection of medication and optimized dosage to maximize therapeutic response and to minimize ADRs. In the ICU, where insufficient drug efficacy, polypharmacy and ADRs are challenges faced by clinicians, PGx guided prescribing may be of immense value. Specific areas to consider include:
Personalized Dosing: Studying the interplay between PGx, population PK and clinical PK might most effectively advance PGx for personalized dosing. The warfarin dose calculator at the warfarin dosing.org website is commonly used for prediction of warfarin doses and uses genotypes and clinical factors in its estimates (Johnson et al., 2017). This approach should be evaluated for other medications used in the ICU as we gain scientific information on PGx.
Decrease ADRs: Many factors are involved in the variation of the efficacy or ADRs to pharmacotherapy. The effect of genetics on drug PK/PD is increasingly being appreciated. PGx provides clinicians in the ICU with additional information to assess the potential for efficacy and/or toxicity of medications. For instance, there was a high risk of the phenytoin induced SJS or TEN in individuals who carry one or two HLA-B*15:02 alleles (van der Weide et al., 2001). The clinical implementation of PGx knowledge is expected to decrease ADRs by improving the selection of medicines who are likely to be safe and selecting the optimum dose for efficacy in critically ill patients (Empey, 2010).
Save costs and time to optimize therapy: The goal of PGx is to facilitate to select the right medication and the tailored dosage. The implementation of PGx in the ICU has the potential to significantly reduce the costs and time. According to Desta et al., the H. pylori infection eradication rate of the tailored treatment basing on PPIs PGx was higher and the final cost per patient for successful eradication did not increase (Desta et al., 2002). These estimates indicate that the determination of CYP2C19 genotype prior to PPI therapy is cost-effective. In 12 large academic institutions in the U.S., genotype-guided antiplatelet treatments were found to decrease the incidence of cardiovascular events and increase the cost-effectiveness in patients who underwent percutaneous coronary interventions (Deiman et al., 2016). Different genotyping/phenotyping analysis, like those for CYP2D6, have already been successfully applied in oncology practice and are becoming increasingly indicated in psychiatric practice. This is particularly true when variants in one gene were shown to be important in the PK and response to multiple drugs. Several PGx guided treatments have been demonstrated to have high cost-effectiveness in chronic disease settings (Empey et al., 2017). Given that some of these drugs are commonly used in the ICU (e.g., CYP2C19 genotype for voriconazole, clopidogrel, PPIs, diazepam treatment), it is possible that the inclusion of PGx information for patients in the ICU could also be cost-effective. The cost-effectiveness of genotyping in guiding choice of multiple medications for critically ill patients requires further scientific study.
Despite all the promises, PGx has not been studied or implemented extensively in the ICU setting to date, due in part to the hurdles presented. Results of diagnostic tests to identify genotypes would need to be available quickly and clinicians would need to understand how to interpret and apply the results in order to guide therapy efficiently. Secondly, PK/PD parameters can be affected by the changing blood volume, severity of illness, and not only acute but also chronic diseases impacting organ function. To date, most PGx studies were conducted in healthy volunteers with single specific disease states, not critically ill patients. It is unlikely accurate to forecast or monitor drug response basing on the PGx knowledge generated in healthy individuals or individuals with chronic illness. Thirdly, it is common in the ICU for patients to be treated with more than 5 drugs. The interpretation of PGx in the ICU will be further complicated by the existence of polypharmacy and numerous potential drug-drug interactions. Fourth, due to our limited knowledge of the mechanisms of ADRs, assessing how many ADRs are associated with genetic variations is still unknown. And finally, the PGx study end points assessed were often related to PK, which may or may not reflect the clinical response/toxicity of patients.
Future Perspective
With the improved accuracy and rapidly declining costs of large-scale genome testing and development of cost-effective array-based tests, technical advancement has gradually overcome the first challenge (Johnson et al., 2012; Schildcrout et al., 2012). Indeed, it is increasingly easy, cheap and straightforward to perform PGx tests in clinical practice (particularly those around metabolism gene and drug transporters) considering the commercialization and routine utility of PGx tests. In spite of the recent increase in the knowledge of PGx used for targeted therapeutics, acutely ill patients in the critical care environment fail to be routinely evaluated for genetic variants to forecast the efficacy of the treatment. The CPIC/DPWG guidelines and FDA labeling clearly support using data when it is available. To date, the number of PGx studies done in the ICU setting remains small. More research is needed in this particularly challenging area to gather the evidence and justify widespread PGx screening in the ICU. It is challenging for critical care researchers to design and conduct studies on gene association that are adequately controlled but also possess the power to correctly assess findings and apply the PGx information clinically. The proper time point to order the PGx tests or whether it should be done in all patients is rarely discussed. Given the complexity and the hurdles in studying and implementing PGx in the ICU setting, we propose the following critical steps for practitioners. First of all, perform preemptive genotyping and construct clinical recommendation tool in the electronic medical record. This will allow the timely and accurate application of genotype information, which can be used for prescribing decisions at the point-of-action. Secondly, focus on key gene products and evaluate them as a panel. The evidence to date (as reviewed in this manuscript) supports the genotyping of key metabolic enzymes to guide pharmacotherapy of commonly used ICU drugs. The predictive value of a single genetic variant with regard to drug response is often limited, and combinations of multiple genetic variants may be involved. Thirdly, develop clinically actionable flow charts (Figure 1) to facility the adaptation of genomic information into clinical decision-making. In the ICU, a clinically actionable flow chart should consider both gene-drug interactions and drug-drug interactions when recommending medications to treat an ICU patient. And finally, offer basic and advanced PGx education to all healthcare professionals caring for ICU patients so the interprofessional team understands the clinical application of PGx and how it can further improve patient outcomes. In summary, PGx is moving forward from the bench to the bedside and will offer new insight to assist clinicians in optimizing pharmacotherapy in the ICU. Further research is needed to define cost-effective, clinically actionable recommendations in the ICU, but the interest and potential of precision medicine to improve pharmacotherapy is compelling.
Author Contributions
SZ and RH conceived and designed the study. SZ conducted the literature search and drafted the manuscript; SZ, DS, PJ, and RH edited and approved the manuscript.
Funding
SZ is supported by a research grant from Shanghai Municipal Commission of Health and Family Planning Research Project of China (20164Y0170), a grant from “the Fundamental Research Funds for the Central Universities of China” (22120180394), and a research grant “Pandeng Plan” from Shanghai Tenth People's Hospital of China (04.01.16.025). RH receives a NIH/NCI grant [1R01CA204856-01A1] and a research grant from the Avon Foundation for Women. She also received support from the NIH/NIGMS [grants K08GM089941, U01GM61393] and the NIH/NCI [grant R21 CA139278].
Conflict of Interest Statement
The authors declare that the research was conducted in the absence of any commercial or financial relationships that could be construed as a potential conflict of interest.
Abbreviations
ICU, intensive care unit; PGx, pharmacogenomics; ADR, adverse drug reaction; FDA, food and drug administration; CPIC, clinical pharmacogenetics implementation consortium; DPWG, royal dutch association for the advancement of pharmacy-pharmacogenetics working group; CYP, cytochrome P450; UM, ultrarapid metabolizer; RM, rapid metabolizer; EM, extensive metabolizer; NM, normal metabolizer; IM, Intermediate metabolizer; PM, poor metabolizer; PK, pharmacokinetics; PD, pharmacodynamics; M3G, morphine-3-glucuronide; M6G, morphine-6-glucuronide; UGT, uridine diphosphate glucuronosyltransferase; AUC, area under the curve; DEX, Dexmedetomidine; INR, international normalized ratio; PPIs, proton pump inhibitors; TEN, toxic epidermal necrolysis; SJS, stevens-johnson syndrome.
References
Ahmed, A. E., Jacob, S., Campbell, G. A., Harirah, H. M., Perez-Polo, J. R., and Johnson, K. M. (2005). Fetal origin of adverse pregnancy outcome: the water disinfectant by-product chloroacetonitrile induces oxidative stress and apoptosis in mouse fetal brain. Brain Res. Dev. Brain Res. 159, 1–11. doi: 10.1016/j.devbrainres.2005.05.008
Ameer, B., and Greenblatt, D. J. (1981). Lorazepam: a review of its clinical pharmacological properties and therapeutic uses. Drugs 21, 162–200.
Anantasit, N., Boyd, J. H., Walley, K. R., and Russell, J. A. (2014). Serious adverse events associated with vasopressin and norepinephrine infusion in septic shock. Crit. Care Med. 42, 1812–1820. doi: 10.1097/CCM.0000000000000333
Andes, D., Pascual, A., and Marchetti, O. (2009). Antifungal therapeutic drug monitoring: established and emerging indications. Antimicrob. Agents Chemother. 53, 24–34. doi: 10.1128/AAC.00705-08
Angiolillo, D. J., Gibson, C. M., Cheng, S., Ollier, C., Nicolas, O., Bergougnan, L., et al. (2011). Differential effects of omeprazole and pantoprazole on the pharmacodynamics and pharmacokinetics of clopidogrel in healthy subjects: randomized, placebo-controlled, crossover comparison studies. Clin. Pharmacol. Ther. 89, 65–74. doi: 10.1038/clpt.2010.219
Apfelbaum, J. L., Silverstein, J. H., Chung, F. F., Connis, R. T., Fillmore, R. B., and Hunt, S. E. (2013). Practice guidelines for postanesthetic care an updated report by the american society of anesthesiologists task force on postanesthetic care. Anesthesiology 118, 291–307. doi: 10.1097/ALN.0b013e31827773e9
Bank, P. C. D., Caudle, K. E., Swen, J. J., Gammal, R. S., Whirl-Carrillo, M., Klein, T. E., et al. (2018). Comparison of the guidelines of the clinical pharmacogenetics implementation consortium and the dutch pharmacogenetics working group. Clin. Pharmacol. Ther. 103, 599–618. doi: 10.1002/cpt.762
Barann, M., Stamer, U. M., Lyutenska, M., Stuber, F., Bonisch, H., and Urban, B. (2015). Effects of opioids on human serotonin transporters. Naunyn Schmiedebergs. Arch. Pharmacol. 388, 43–49. doi: 10.1007/s00210-014-1056-3
Barratt, D. T., Bandak, B., Klepstad, P., Dale, O., Kaasa, S., Christrup, L. L., et al. (2014). Genetic, pathological and physiological determinants of transdermal fentanyl pharmacokinetics in 620 cancer patients of the EPOS study. Pharmacogenet. Genom. 24, 185–194. doi: 10.1097/FPC.0000000000000032
Behrooz, A. (2015). Pharmacogenetics and anaesthetic drugs: implications for perioperative practice. Ann. Med. Surg. 4, 470–474. doi: 10.1016/j.amsu.2015.11.001
Bell, G. C., Caudle, K. E., Whirl-Carrillo, M., Gordon, R. J., Hikino, K., Prows, C. A., et al. (2017). Clinical pharmacogenetics implementation consortium (CPIC) guideline for CYP2D6 genotype and use of ondansetron and tropisetron. Clin. Pharmacol. Ther. 102, 213–218. doi: 10.1002/cpt.598
Bertilsson, L., Henthorn, T. K., Sanz, E., Tybring, G., Sawe, J., and Villen, T. (1989). Importance of genetic factors in the regulation of diazepam metabolism: relationship to S-mephenytoin, but not debrisoquin, hydroxylation phenotype. Clin. Pharmacol. Ther. 45, 348–355.
Bouatou, Y., Samer, C. F., Ing Lorenzini, K. R., Daali, Y., Daou, S., Fathi, M., et al. (2014). Therapeutic drug monitoring of voriconazole: a case report of multiple drug interactions in a patient with an increased CYP2C19 activity. AIDS Res. Ther. 11:25. doi: 10.1186/1742-6405-11-25
Brüggemann, R. J., Alffenaar, J. W., Blijlevens, N. M., Billaud, E. M., Kosterink, J. G., Verweij, P. E., et al. (2009). Clinical relevance of the pharmacokinetic interactions of azole antifungal drugs with other coadministered agents. Clin. Infect. Dis. 48, 1441–1458. doi: 10.1086/598327
Calcaterra, N. E., and Barrow, J. C. (2014). Classics in chemical neuroscience: diazepam (valium). ACS Chem. Neurosci. 5, 253–260. doi: 10.1021/cn5000056
Campa, D., Gioia, A., Tomei, A., Poli, P., and Barale, R. (2008). Association of ABCB1/MDR1 and OPRM1 gene polymorphisms with morphine pain relief. Clin. Pharmacol. Ther. 83, 559–566. doi: 10.1038/sj.clpt.6100385
Carr, D. F., and Pirmohamed, M. (2018). Biomarkers of adverse drug reactions. Exp. Biol. Med. (Maywood).243, 291–299. doi: 10.1177/1535370217733425
Caudle, K. E., Dunnenberger, H. M., Freimuth, R. R., Peterson, J. F., Burlison, J. D., Whirl-Carrillo, M., et al. (2017). Standardizing terms for clinical pharmacogenetic test results: consensus terms from the Clinical Pharmacogenetics Implementation Consortium (CPIC). Genet. Med. 19, 215–223. doi: 10.1038/gim.2016.87
Caudle, K. E., Gammal, R. S., Whirl-Carrillo, M., Hoffman, J. M., Relling, M. V., and Klein, T. E. (2016). Evidence and resources to implement pharmacogenetic knowledge for precision medicine. Am. J. Health Syst. Pharm. 73, 1977–1985. doi: 10.2146/ajhp150977
Caudle, K. E., Klein, T. E., Hoffman, J. M., Muller, D. J., Whirl-Carrillo, M., Gong, L., et al. (2014a). Incorporation of pharmacogenomics into routine clinical practice: the clinical pharmacogenetics implementation consortium (CPIC) Guideline Development Process. Curr. Drug Metab. 15, 209–217. doi: 10.2174/1389200215666140130124910
Caudle, K. E., Rettie, A. E., Whirl-Carrillo, M., Smith, L. H., Mintzer, S., Lee, M. T., et al. (2014b). Clinical pharmacogenetics implementation consortium guidelines for CYP2C9 and HLA-B genotypes and phenytoin dosing. Clin. Pharmacol. Ther. 96, 542–548. doi: 10.1038/clpt.2014.159
Cavallari, L. H., Lee, C. R., Beitelshees, A. L., Cooper-DeHoff, R. M., Duarte, J. D., Voora, D., et al. (2018). Multisite investigation of outcomes with implementation of CYP2C19 genotype-guided antiplatelet therapy after percutaneous coronary intervention. JACC Cardiovasc. Int. 11, 181–191. doi: 10.1016/j.jcin.2017.07.022
Chadwick, D. W. (1985). Concentration-effect relationships of valproic acid. Clin. Pharmacokinet. 10, 155–163. doi: 10.2165/00003088-198510020-00003
Chaudhry, A. S., Kochhar, R., and Kohli, K. K. (2008). Genetic polymorphism of CYP2C19 & therapeutic response to proton pump inhibitors. Indian J. Med. Res. 127, 521–530.
Choi, L., Ferrell, B. A., Vasilevskis, E. E., Pandharipande, P. P., Heltsley, R., Ely, E. W., et al. (2016). Population pharmacokinetics of fentanyl in the critically Ill. Crit. Care Med. 44, 64–72. doi: 10.1097/CCM.0000000000001347
Choonara, I. A., Haynes, B. P., Cholerton, S., Breckenridge, A. M., and Park, B. K. (1986). Enantiomers of warfarin and vitamin K1 metabolism. Br. J. Clin. Pharmacol. 22, 729–732
Chung, J. Y., Cho, J. Y., Yu, K. S., Kim, J. R., Jung, H. R., Lim, K. S. Effect of the UGT2B15 genotype on the pharmacokinetics, pharmacodynamics, drug interactions of intravenous lorazepam in healthy volunteers., et al. (2005). Clin. Pharmacol. Ther. 77, 486–494. doi: 10.1016/j.clpt.2005.02.006
Chung, J. Y., Cho, J. Y., Yu, K. S., Kim, J. R., Lim, K. S., Sohn, D. R., et al. (2008). Pharmacokinetic and pharmacodynamic interaction of lorazepam and valproic acid in relation to UGT2B7 genetic polymorphism in healthy subjects. Clin. Pharmacol. Ther. 83, 595–600. doi: 10.1038/sj.clpt.6100324
Chung, W. H., Hung, S. I., Hong, H. S., Hsih, M. S., Yang, L. C., Ho, H. C., et al. (2004). Medical genetics: a marker for Stevens-Johnson syndrome. Nature 428:486. doi: 10.1038/428486a
Court, M. H., Duan, S. X., Hesse, L. M., Venkatakrishnan, K., and Greenblatt, D. J. (2001). Cytochrome P-450 2B6 is responsible for interindividual variability of propofol hydroxylation by human liver microsomes. Anesthesiology 94, 110–119.
Couvert, P., Giral, P., Dejager, S., Gu, J., Huby, T., Chapman, M. J., et al. (2008). Association between a frequent allele of the gene encoding OATP1B1 and enhanced LDL-lowering response to fluvastatin therapy. Pharmacogenomics 9, 1217–1227. doi: 10.2217/14622416.9.9.1217
Crews, K. R., Gaedigk, A., Dunnenberger, H. M., Leeder, J. S., Klein, T. E., Caudle, K. E., et al. (2014). Clinical pharmacogenetics implementation consortium guidelines for cytochrome P450 2D6 genotype and codeine therapy: 2014 update. Clin. Pharmacol. Ther. 95, 376–382. doi: 10.1038/clpt.2013.254
De Gregori, M., Diatchenko, L., Ingelmo, P. M., Napolioni, V., Klepstad, P., Belfer, I., et al. (2016). Human genetic variability contributes to postoperative morphine consumption. J. Pain 17, 628–636. doi: 10.1016/j.jpain.2016.02.003
de Leon, J., Dinsmore, L., and Wedlund, P. (2003). Adverse drug reactions to oxycodone and hydrocodone in CYP2D6 ultrarapid metabolizers. J. Clin. Psychopharmacol. 23, 420–421. doi: 10.1097/01.jcp.0000085421.74359.60
Deiman, B. A., Tonino, P. A., Kouhestani, K., Schrover, C. E., Scharnhorst, V., Dekker, L. R., et al. (2016). Reduced number of cardiovascular events and increased cost-effectiveness by genotype-guided antiplatelet therapy in patients undergoing percutaneous coronary interventions in the Netherlands. Neth. Heart J. 24, 589–599. doi: 10.1007/s12471-016-0873-z
Desta, Z., Zhao, X., Shin, J. G., and Flockhart, D. A. (2002). Clinical significance of the cytochrome P450 2C19 genetic polymorphism. Clin. Pharmacokinet. 41, 913–958. doi: 10.2165/00003088-200241120-00002
DeVane, C. L. (2003). Pharmacokinetics, drug interactions, and tolerability of valproate. Psychopharmacol. Bull. 37(Suppl. 2), 25–42.
Doucet, J., Jego, A., Noel, D., Geffroy, C. E., Capet, C., Coquard, A., et al. (2002). Preventable and non-preventable risk factors for adverse drug events related to hospital admissions in the elderly - A prospective study. Clin. Drug Investig. 22, 385–392. doi: 10.2165/00044011-200222060-00006
Empey, P. E. (2010). Genetic predisposition to adverse drug reactions in the intensive care unit. Crit. Care Med. 38(6 Suppl.), S106–S116. doi: 10.1097/CCM.0b013e3181de09f8
Empey, P. E., Stevenson, J. M., Tuteja, S., Weitzel, K. W., Angiolillo, D. J., Beitelshees, A. L., et al. (2017). Multisite investigation of strategies for the implementation of CYP2C19 genotype-guided antiplatelet therapy. Clin. Pharmacol. Ther. 104, 664–674. doi: 10.1002/cpt.1006
Feierman, D. E., and Lasker, J. M. (1996). Metabolism of fentanyl, a synthetic opioid analgesic, by human liver microsomes. Role of CYP3A4. Drug Metab. Dispos. 24, 932–939
Furuta, T., Shirai, N., Sugimoto, M., Nakamura, A., Hishida, A., and Ishizaki, T. (2005). Influence of CYP2C19 pharmacogenetic polymorphism on proton pump inhibitor-based therapies. Drug Metab. Pharmacokinet. 20, 153–167. doi: 10.2133/dmpk.20.153
Gaedigk, A., Ingelman-Sundberg, M., Miller, N. A., Leeder, J. S., Whirl-Carrillo, M., and Klein, T. E. (2018). The Pharmacogene variation (PharmVar) consortium: incorporation of the human cytochrome P450 (CYP) Allele Nomenclature Database. Clin. Pharmacol. Ther. 103, 399–401. doi: 10.1002/cpt.910
Gage, B. F., Bass, A. R., Lin, H., Woller, S. C., Stevens, S. M., Al-Hammadi, N., et al. (2017). Effect of genotype-guided warfarin dosing on clinical events and anticoagulation control among patients undergoing hip or knee arthroplasty the GIFT randomized clinical trial. J. Am. Med. Assoc. 318, 1115–1124. doi: 10.1001/jama.2017.11469
Goryachkina, K., Burbello, A., Boldueva, S., Babak, S., Bergman, U., and Bertilsson, L. (2008). CYP2D6 is a major determinant of metoprolol disposition and effects in hospitalized Russian patients treated for acute myocardial infarction. Eur. J. Clin. Pharmacol. 64, 1163–1173. doi: 10.1007/s00228-008-0525-3
Grenouillet-Delacre, M., Verdoux, H., Moore, N., Haramburu, F., Miremont-Salamé, G., Etienne, G., et al. (2007). Life-threatening adverse drug reactions at admission to medical intensive care: a prospective study in a teaching hospital. Intens. Care Med. 33, 2150–2157. doi: 10.1007/s00134-007-0787-8
Group, S. C., Link, E., Parish, S., Armitage, J., Bowman, L., Heath, S., et al. (2008). SLCO1B1 variants and statin-induced myopathy–a genomewide study. N. Engl. J. Med. 359, 789–799. doi: 10.1056/NEJMoa0801936
Guo, Y., Hu, C., He, X., Qiu, F., and Zhao, L. (2012). Effects of UGT1A6, UGT2B7, and CYP2C9 genotypes on plasma concentrations of valproic acid in Chinese children with epilepsy. Drug Metab. Pharmacokinet. 27, 536–542. doi: 10.2133/dmpk.DMPK-11-NT-144
Hamelin, B. A., Bouayad, A., Drolet, B., Gravel, A., and Turgeon, J. (1998). In vitro characterization of cytochrome P450 2D6 inhibition by classic histamine H-1 receptor antagonists. Drug Metab. Dispos. 26, 536–539.
Hamelin, B. A., Bouayad, A., Methot, J., Jobin, J., Desgagnes, P., Poirier, P., et al. (2000). Significant interaction between the nonprescription antihistamine diphenhydramine and the CYP2D6 substrate metoprolol in healthy men with high or low CYP2D6 activity. Clin. Pharmacol. Ther. 67, 466–477. doi: 10.1067/mcp.2000.106464
He, H., Yin, J. Y., Xu, Y. J., Li, X., Zhang, Y., Liu, Z. G., et al. (2014). Association of ABCB1 polymorphisms with the efficacy of ondansetron in chemotherapy-induced nausea and vomiting. Clin. Ther. 36, 1242–1252. doi: 10.1016/j.clinthera.2014.06.016
Holliday, S. F., Kane-Gill, S. L., Empey, P. E., Buckley, M. S., and Smithburger, P. L. (2014). Interpatient variability in dexmedetomidine response: a survey of the literature. Sci. World J. 2014:805013. doi: 10.1155/2014/805013
Horvat, C. M., Au, A. K., Conley, Y. P., Kochanek, P. M., Li, L., Poloyac, S. M., et al. (2017). ABCB1 genotype is associated with fentanyl requirements in critically ill children. Pediatr. Res. 82, 29–35. doi: 10.1038/pr.2017.103
Hulot, J. S., Collet, J. P., Cayla, G., Silvain, J., Allanic, F., Bellemain-Appaix, A., et al. (2011). CYP2C19 but not PON1 genetic variants influence clopidogrel pharmacokinetics, pharmacodynamics, and clinical efficacy in post-myocardial infarction patients. Circ. Cardiovasc. Interv. 4, 422–428. doi: 10.1161/CIRCINTERVENTIONS.111.963025
Hung, S. I., Chung, W. H., Liu, Z. S., Chen, C. H., Hsih, M. S., Hui, R. C., et al. (2010). Common risk allele in aromatic antiepileptic-drug induced Stevens-Johnson syndrome and toxic epidermal necrolysis in Han Chinese. Pharmacogenomics 11, 349–356. doi: 10.2217/pgs.09.162
Hwang, I. C., Park, J. Y., Myung, S. K., Ahn, H. Y., Fukuda, K., and Liao, Q. (2014). OPRM1 A118G gene variant and postoperative opioid requirement: a systematic review and meta-analysis. Anesthesiology 121, 825–834. doi: 10.1097/ALN.0000000000000405
Isbister, G. K., Ang, K., Gorman, K., Cooper, J., Mostafa, A., and Roberts, M. S. (2016). Zero-order metoprolol pharmacokinetics after therapeutic doses: severe toxicity and cardiogenic shock. Clin. Toxicol. 54, 881–885. doi: 10.1080/15563650.2016.1209768
Jiang, D. C., and Wang, L. (2004). Population pharmacokinetic model of valproate and prediction of valproate serum concentrations in children with epilepsy. Acta Pharmacol. Sin. 25, 1576–1583.
Jin, M., Gock, S. B., Jannetto, P. J., Jentzen, J. M., and Wong, S. H. (2005). Pharmacogenomics as molecular autopsy for forensic toxicology: genotyping cytochrome P450 3A4*1B and 3A5*3 for 25 fentanyl cases. J. Anal. Toxicol. 29, 590–598.
Johnson, J. A., Burkley, B. M., Langaee, T. Y., Clare-Salzler, M. J., Klein, T. E., and Altman, R. B. (2012). Implementing personalized medicine: development of a cost-effective customized pharmacogenetics genotyping array. Clin. Pharmacol. Ther. 92, 437–439. doi: 10.1038/clpt.2012.125
Johnson, J. A., Caudle, K. E., Gong, L., Whirl-Carrillo, M., Stein, C. M., Scott, S. A., et al. (2017). Clinical pharmacogenetics implementation consortium (CPIC) guideline for pharmacogenetics-guided warfarin dosing: 2017 Update. Clin. Pharmacol. Ther. 102, 397–404. doi: 10.1002/cpt.668
Joshua, L., Devi, P., and Guido, S. (2009). Adverse drug reactions in medical intensive care unit of a tertiary care hospital. Pharmacoepidemiol. Drug Saf. 18, 639–645. doi: 10.1002/pds.1761
Kaiser, R., Sezer, O., Papies, A., Bauer, S., Schelenz, C., Tremblay, P. B., et al. (2002). Patient-tailored antiemetic treatment with 5-hydroxytryptamine type 3 receptor antagonists according to cytochrome P-450 2D6 genotypes. J. Clin. Oncol. 20, 2805–2811. doi: 10.1200/Jco.2002.09.064
Kalman, L. V., Agúndez, J., Appell, M. L., Black, J. L., Bell, G. C., Boukouvala, S., et al. (2016). Pharmacogenetic allele nomenclature: international workgroup recommendations for test result reporting. Clin. Pharmacol. Ther. 99, 172–185. doi: 10.1002/cpt.280
Kearon, C., Akl, E. A., Ornelas, J., Blaivas, A., Jimenez, D., Bounameaux, H., et al. (2016). Antithrombotic therapy for VTE disease: CHEST guideline and expert panel report. Chest 149, 315–352. doi: 10.1016/j.chest.2015.11.026
Kirchheiner, J., Keulen, J. T., Bauer, S., Roots, I., and Brockmöller, J. (2008). Effects of the CYP2D6 gene duplication on the pharmacokinetics and pharmacodynamics of tramadol. J. Clin. Psychopharmacol. 28, 78–83. doi: 10.1097/JCP.0b013e318160f827
Kirchheiner, J., Schmidt, H., Tzvetkov, M., Keulen, J. T., Lötsch, J., Roots, I., et al. (2007). Pharmacokinetics of codeine and its metabolite morphine in ultra-rapid metabolizers due to CYP2D6 duplication. Pharmacogenomics J.7, 257–265. doi: 10.1038/sj.tpj.6500406
Klepstad, P., Fladvad, T., Skorpen, F., Bjordal, K., Caraceni, A., Dale, O., et al. (2011). Influence from genetic variability on opioid use for cancer pain: a European genetic association study of 2294 cancer pain patients. Pain 152, 1139–1145. doi: 10.1016/j.pain.2011.01.040
Kohli, U., Pandharipande, P., Muszkat, M., Sofowora, G. G., Friedman, E. A., Scheinin, M., et al. (2012). CYP2A6 genetic variation and dexmedetomidine disposition. Eur. J. Clin. Pharmacol. 68, 937–942. doi: 10.1007/s00228-011-1208-z
Kuehl, P., Zhang, J., Lin, Y., Lamba, J., Assem, M., Schuetz, J., et al. (2001). Sequence diversity in CYP3A promoters and characterization of the genetic basis of polymorphic CYP3A5 expression. Nat. Genet. 27, 383–391. doi: 10.1038/86882
Kummer, O., Hammann, F., Moser, C., Schaller, O., Drewe, J., and Krahenbuhl, S. (2011). Effect of the inhibition of CYP3A4 or CYP2D6 on the pharmacokinetics and pharmacodynamics of oxycodone. Eur. J. Clin. Pharmacol. 67, 63–71. doi: 10.1007/s00228-010-0893-3
Landau, R., Kern, C., Columb, M. O., Smiley, R. M., and Blouin, J. L. (2008). Genetic variability of the mu-opioid receptor influences intrathecal fentanyl analgesia requirements in laboring women. Pain 139, 5–14. doi: 10.1016/j.pain.2008.02.023
Leckband, S. G., Kelsoe, J. R., Dunnenberger, H. M., George, AL Jr., Tran, E., Berger, R., et al. (2013). Clinical pharmacogenetics implementation consortium guidelines for HLA-B genotype and carbamazepine dosing. Clin. Pharmacol. Ther. 94, 324–328. doi: 10.1038/clpt.2013.103
Lee, C. R., Goldstein, J. A., and Pieper, J. A. (2002). Cytochrome P450 2C9 polymorphisms: a comprehensive review of the in-vitro and human data. Pharmacogenetics 12, 251–263.
Lehmann, A. S., Renbarger, J. L., McCormick, C. L., Topletz, A. R., Rouse, C., and Haas, D. M. (2013). Pharmacogenetic predictors of nausea and vomiting of pregnancy severity and response to antiemetic therapy: a pilot study. BMC Pregnancy Childb. 13:132. doi: 10.1186/1471-2393-13-132
Lemaitre, F., Barbaz, M., Scailteux, L. M., Uhel, F., Tadié, J. M., Verdier, M. C., et al. (2013). A case-report of unpredictable and massive voriconazole intoxication in a patient with extensive CYP2C19 and CYP2C9 polymorphisms. Drug Metab. Pharmacokinet. 28, 439–441. doi: 10.2133/dmpk.DMPK-13-NT-007
Li, T. Y., Liu, W., Chen, K., Liang, S. Y., and Liu, F. (2017). The influence of combination use of CYP450 inducers on the pharmacokinetics of voriconazole: a systematic review. J. Clin. Pharm. Ther. 42, 135–146. doi: 10.1111/jcpt.12493
Limdi, N. A., Wadelius, M., Cavallari, L., Eriksson, N., Crawford, D. C., Lee, M. T., et al. (2010). Warfarin pharmacogenetics: a single VKORC1 polymorphism is predictive of dose across 3 racial groups. Blood 115, 3827–3834. doi: 10.1182/blood-2009-12-255992
Lin, Y. S., Dowling, A. L., Quigley, S. D., Farin, F. M., Zhang, J., Lamba, J., et al. (2002). Co-regulation of CYP3A4 and CYP3A5 and contribution to hepatic and intestinal midazolam metabolism. Mol. Pharmacol. 62, 162–172. doi: 10.1124/mol.62.1.162
Llerena, A., Alm, C., Dahl, M. L., Ekqvist, B., and Bertilsson, L. (1992). Haloperidol disposition is dependent on debrisoquine hydroxylation phenotype. Ther. Drug Monit. 14, 92–97.
Lötsch, J., von Hentig, N., Freynhagen, R., Griessinger, N., Zimmermann, M., Doehring, A., et al. (2009). Cross-sectional analysis of the influence of currently known pharmacogenetic modulators on opioid therapy in outpatient pain centers. Pharmacogenet. Genom. 19, 429–436. doi: 10.1097/FPC.0b013e32832b89da
MacKenzie, M., and Hall, R. (2017). Pharmacogenomics and pharmacogenetics for the intensive care unit: a narrative review. Can. J. Anaesth. 64, 45–64. doi: 10.1007/s12630-016-0748-1
Mastrogianni, O., Gbandi, E., Orphanidis, A., Raikos, N., Goutziomitrou, E., Kolibianakis, E. M., et al. (2014). Association of the CYP2B6 c.516G>T polymorphism with high blood propofol concentrations in women from northern Greece. Drug Metab. Pharmacokinet. 29, 215–218. doi: 10.2133/dmpk.DMPK-13-NT-092
Matsuoka, H., Makimura, C., Koyama, A., Fujita, Y., Tsurutani, J., Sakai, K., et al. (2017). Prospective replication study implicates the catechol-O-methyltransferase Val(158)Met polymorphism as a biomarker for the response to morphine in patients with cancer. Biomed. Rep. 7, 380–384. doi: 10.3892/br.2017.963
McCormack, M., Alfirevic, A., Bourgeois, S., Farrell, J. J., Kasperaviciute, D., Carrington, M., et al. (2011). HLA-A*3101 and carbamazepine-induced hypersensitivity reactions in Europeans. N. Engl. J. Med. 364, 1134–1143. doi: 10.1056/NEJMoa1013297
Mörike, K, Kivistö, K. T., Schaeffeler, E., Jägle, C., Igel, S., Drescher, S., et al. (2008). Propafenone for the prevention of atrial tachyarrhythmias after cardiac surgery: a randomized, double-blind placebo-controlled trial. Clin. Pharmacol. Ther. 84, 104–110. doi: 10.1038/sj.clpt.6100473
Moriyama, B., Obeng, A. O., Barbarino, J., Penzak, S. R., Henning, S. A., Scott, S. A., et al. (2016). Clinical pharmacogenetics implementation consortium (CPIC) guidelines for CYP2C19 and voriconazole Therapy. Clin. Pharmacol. Ther. 102, 45–51. doi: 10.1002/cpt.583
Mourão, A. L., de Abreu, F. G., and Fiegenbaum, M. (2016). Impact of the Cytochrome P450 2B6 (CYP2B6) Gene Polymorphism c.516G>T (rs3745274) on propofol dose variability. Eur. J. Drug Metab. Pharmacokinet. 41, 511–515. doi: 10.1007/s13318-015-0289-y
Nielsen, L. M., Olesen, A. E., Branford, R., Christrup, L. L., Sato, H., and Drewes, A. M. (2015). Association between human pain-related genotypes and variability in opioid analgesia: an updated review. Pain Pract. 15, 580–594. doi: 10.1111/papr.12232
Nielsen, L. M., Sverrisdóttir, E., Stage, T. B., Feddersen, S., Brøsen, K., Christrup, L. L., et al. (2017). Lack of genetic association between OCT1, ABCB1, and UGT2B7 variants and morphine pharmacokinetics. Eur. J. Pharm. Sci. 99, 337–342. doi: 10.1016/j.ejps.2016.12.039
Niemi, M. (2010). Transporter pharmacogenetics and statin toxicity. Clin. Pharmacol. Ther. 87, 130–133. doi: 10.1038/clpt.2009.197
Ozeki, T., Mushiroda, T., Yowang, A., Takahashi, A., Kubo, M., Shirakata, Y., et al. (2011). Genome-wide association study identifies HLA-A*3101 allele as a genetic risk factor for carbamazepine-induced cutaneous adverse drug reactions in Japanese population. Hum. Mol. Genet. 20, 1034–1041. doi: 10.1093/hmg/ddq537
Pasanen, M. K., Neuvonen, M., Neuvonen, P. J., and Niemi, M. (2006). SLCO1B1 polymorphism markedly affects the pharmacokinetics of simvastatin acid. Pharmacogenet. Genom. 16, 873–879. doi: 10.1097/01.fpc.0000230416.82349.90
Pascual, A., Calandra, T., Bolay, S., Buclin, T., Bille, J., and Marchetti, O. (2008). Voriconazole therapeutic drug monitoring in patients with invasive mycoses improves efficacy and safety outcomes. Clin. Infect. Dis. 46, 201–211. doi: 10.1086/524669
Patterson, S. D., Cohen, N., Karnoub, M., Truter, S. L., Emison, E., Khambata-Ford, S., et al. (2011). Prospective-retrospective biomarker analysis for regulatory consideration: white paper from the industry pharmacogenomics working group. Pharmacogenomics 12, 939–951. doi: 10.2217/pgs.11.52
Patterson, T. F., Thompson, G. R. 3rd, Denning, D. W., Fishman, J. A., Hadley, S., Herbrecht, R., et al. (2016). Practice guidelines for the diagnosis and management of aspergillosis: 2016 update by the infectious diseases society of america. Clin. Infect. Dis. 63, e1–e60. doi: 10.1093/cid/ciw326
Pirmohamed, M., Burnside, G., Eriksson, N., Jorgensen, A. L., Toh, C. H., Nicholson, T., et al. (2013). A randomized trial of genotype-guided dosing of warfarin. N. Engl. J. Med. 369, 2294–2303. doi: 10.1056/NEJMoa1311386
Poulsen, L., Brøsen, K., Arendt-Nielsen, L., Gram, L. F., Elbaek, K., and Sindrup, S. H. (1996). Codeine and morphine in extensive and poor metabolizers of sparteine: pharmacokinetics, analgesic effect and side effects. Eur. J. Clin. Pharmacol. 51, 289–295
Qi, F., Zhu, L., Li, N., Ge, T., Xu, G., and Liao, S. (2017). Influence of different proton pump inhibitors on the pharmacokinetics of voriconazole. Int. J. Antimicrob. Agents 49, 403–409. doi: 10.1016/j.ijantimicag.2016.11.025
Qin, X. P., Xie, H. G., Wang, W., He, N., Huang, S. L., Xu, Z. H., et al. (1999). Effect of the gene dosage of CgammaP2C19 on diazepam metabolism in Chinese subjects. Clin. Pharmacol. Ther. 66, 642–646. doi: 10.1016/S0009-9236(99)90075-9
Ramsey, L. B., Johnson, S. G., Caudle, K. E., Haidar, C. E., Voora, D., Wilke, R. A., et al. (2014). The clinical pharmacogenetics implementation consortium guideline for SLCO1B1 and simvastatin-induced myopathy: 2014 update. Clin. Pharmacol. Ther. 96, 423–428. doi: 10.1038/clpt.2014.125
Rau, T., Wuttke, H., Michels, L. M., Werner, U., Bergmann, K., Kreft, M., et al. (2009). Impact of the CYP2D6 genotype on the clinical effects of metoprolol: a prospective longitudinal study. Clin. Pharmacol. Ther. 85, 269–272. doi: 10.1038/clpt.2008.218
Rendic, S., and Guengerich, F. P. (2015). Survey of human oxidoreductases and cytochrome P450 enzymes involved in the metabolism of xenobiotic and natural chemicals. Chem. Res. Toxicol. 28, 38–42. doi: 10.1021/tx500444e
Ross, J. R., Riley, J., Taegetmeyer, A. B., Sato, H., Gretton, S., du Bois, R. M., et al. (2008). Genetic variation and response to morphine in cancer patients: catechol-O-methyltransferase and multidrug resistance-1 gene polymorphisms are associated with central side effects. Cancer 112, 1390–1403. doi: 10.1002/cncr.23292
Rouini, M. R., and Afshar, M. (2017). Effect of CYP2D6 polymorphisms on the pharmacokinetics of propafenone and its two main metabolites. Therapie 72, 373–382. doi: 10.1016/j.therap.2016.10.005
Roujeau, J. C., and Stern, R. S. (1994). Severe adverse cutaneous reactions to drugs. N. Engl. J. Med. 331, 1272–1285. doi: 10.1056/NEJM199411103311906
Saari, T. I., Laine, K., Leino, K., Valtonen, M., Neuvonen, P. J., and Olkkola, K. T. (2006). Effect of voriconazole on the pharmacokinetics and pharmacodynamics of intravenous and oral midazolam. Clin. Pharmacol. Ther. 79, 362–370. doi: 10.1016/j.clpt.2005.12.305
Sadhasivam, S., Chidambaran, V., Zhang, X., Meller, J., Esslinger, H., Zhang, K., et al. (2015). Opioid-induced respiratory depression: ABCB1 transporter pharmacogenetics. Pharmacogenomics J.15, 119–126. doi: 10.1038/tpj.2014.56
Sanwald, P., David, M., and Dow, J. (1996). Characterization of the cytochrome P450 enzymes involved in the in vitro metabolism of dolasetron - Comparison with other indole-containing 5-HT3 antagonists. Drug Metabol. Disposit. 24, 602–609.
Schildcrout, J. S., Denny, J. C., Bowton, E., Gregg, W., Pulley, J. M., Basford, M. A., et al. (2012). Optimizing drug outcomes through pharmacogenetics: a case for preemptive genotyping. Clin. Pharmacol. Ther. 92, 235–242. doi: 10.1038/clpt.2012.66aa
Schmidt, A. H., Baier, D., Solloch, U. V., Stahr, A., Cereb, N., Wassmuth, R., et al. (2009). Estimation of high-resolution HLA-A, -B, -C, -DRB1 allele and haplotype frequencies based on 8862 German stem cell donors and implications for strategic donor registry planning. Hum. Immunol. 70, 895–902. doi: 10.1016/j.humimm.2009.08.006
Scholz, I., Oberwittler, H., Riedel, K. D., Burhenne, J., Weiss, J., Haefeli, W. E., et al. (2009). Pharmacokinetics, metabolism and bioavailability of the triazole antifungal agent voriconazole in relation to CYP2C19 genotype. Br. J. Clin. Pharmacol. 68, 906–915. doi: 10.1111/j.1365-2125.2009.03534.x
Scott, S. A., Sangkuhl, K., Stein, C. M., Hulot, J. S., Mega, J. L., Roden, D. M., et al. (2013). Clinical pharmacogenetics implementation consortium guidelines for CYP2C19 genotype and clopidogrel therapy: 2013 update. Clin. Pharmacol. Ther. 94, 317–323. doi: 10.1038/clpt.2013.105
Sehrt, D., Meineke, I., Tzvetkov, M., Gültepe, S., and Brockmöller, J. (2011). Carvedilol pharmacokinetics and pharmacodynamics in relation to CYP2D6 and ADRB pharmacogenetics. Pharmacogenomics 12, 783–795. doi: 10.2217/pgs.11.20
Shimatani, T., Inoue, M., Kuroiwa, T., Xu, J., Mieno, H., Nakamura, M., et al. (2006). Acid-suppressive effects of rabeprazole, omeprazole, and lansoprazole at reduced and standard doses: a crossover comparative study in homozygous extensive metabolizers of cytochrome P450 2C19. Clin. Pharmacol. Ther. 79, 144–152. doi: 10.1016/j.clpt.2005.09.012
Shin, J. M., and Kim, N. (2013). Pharmacokinetics and pharmacodynamics of the proton pump inhibitors. J. Neurogastroenterol. Motil. 19, 25–35. doi: 10.5056/jnm.2013.19.1.25
Smith, H. S. (2009). Opioid metabolism. Mayo Clin. Proc. 84, 613–624. doi: 10.1016/S0025-6196(11)60750-7
Spina, S. P., and Ensom, M. H. (2007). Clinical pharmacokinetic monitoring of midazolam in critically ill patients. Pharmacotherapy 27, 389–398. doi: 10.1592/phco.27.3.389
Srinivas, N. R. (2015). Differential consequences of tramadol in overdosing: dilemma of a polymorphic cytochrome P450 2D6-mediated substrate. J. Pain Palliat. Care Pharmacother. 29, 272–275. doi: 10.3109/15360288.2015.1047555
Stamer, U. M., Lee, E. H., Rauers, N. I., Zhang, L., Kleine-Brueggeney, M., Fimmers, R., et al. (2011). CYP2D6-and CYP3A-dependent enantioselective plasma concentrations of ondansetron in postanesthesia care. Anesth. Analg. 113, 48–54. doi: 10.1213/ANE.0b013e31821d01bc
Stamer, U. M., Musshoff, F., Kobilay, M., Madea, B., Hoeft, A., and Stuber, F. (2007). Concentrations of tramadol and O-desmethyltramadol enantiomers in different CYP2D6 genotypes. Clin. Pharmacol. Ther. 82, 41–47. doi: 10.1038/sj.clpt.6100152
Stamer, U. M., Stüber, F., Muders, T., and Musshoff, F. (2008). Respiratory depression with tramadol in a patient with renal impairment and CYP2D6 gene duplication. Anesth. Analg. 107, 926–929. doi: 10.1213/ane.0b013e31817b796e
Su, S. C., Chung, W. H., and Hung, S. I. (2014). Digging up the human genome: current progress in deciphering adverse drug reactions. Biomed Res. Int. 2014:824343. doi: 10.1155/2014/824343
Sun, X., Yu, W. Y., Ma, W. L., Huang, L. H., and Yang, G. P. (2016). Impact of the CYP4F2 gene polymorphisms on the warfarin maintenance dose: a systematic review and meta-analysis. Biomed. Rep. 4, 498–506. doi: 10.3892/br.2016.599
Swen, J. J., Nijenhuis, M., de Boer, A., Grandia, L., Maitland-van der Zee, A. H., Mulder, H., et al. (2011). Pharmacogenetics: from bench to byte-an update of guidelines. Clin. Pharmacol. Ther. 89, 662–673. doi: 10.1038/clpt.2011.34
Tang, M., Mukundan, M., Yang, J., Charpentier, N., LeCluyse, E. L., Black, C., et al. (2006). Antiplatelet agents aspirin and clopidogrel are hydrolyzed by distinct carboxylesterases, and clopidogrel is transesterificated in the presence of ethyl alcohol. J. Pharmacol. Exp. Ther. 319, 1467–1476. doi: 10.1124/jpet.106.110577
U.S Food and Drug Administration (2017a). DRUG LABEL INFORMATION, 12 CLINICAL PHARMACOLOGY, 12.3 Pharmacokinetics; CARVEDILOL- Carvedilol Tablet, Film Coated. Available online at: https://dailymed.nlm.nih.gov/dailymed/drugInfo.cfm?setid=dc6a2d33-aa04-4a04-9cde-901b744d24ca
U.S Food and Drug Administration (2017b). DRUG LABEL INFORMATION, 5 WARNINGS AND PRECAUTIONS, 5.3 Serious Dermatologic Reactions; PHENYTOIN SODIUM- Phenytoin Sodium Injection. Available online at: https://dailymed.nlm.nih.gov/dailymed/drugInfo.cfm?setid=035a8d4e-2063-4240-83cb-d7eebcabe301#LINK_601801c5-035a-4e3a-9964-a1ed82a8ca52
U.S Food and Drug Administration (2018a). DRUG LABEL INFORMATION; ACETAMINOPHEN AND CODEINE PHOSPHATE- Acetaminophen and Codeine Phosphate Liquid. Available online at: https://dailymed.nlm.nih.gov/dailymed/drugInfo.cfm?setid=7bca1d24-a317-44c4-8db2-68030eb961ed
U.S Food and Drug Administration (2018b). DRUG LABEL INFORMATION; DIASTAT- Diazepam Gel. Available online at: https://dailymed.nlm.nih.gov/dailymed/drugInfo.cfm?setid=a84a73a7-8e3a-4985-8be0-04b3028f5e49
U.S Food and Drug Administration (2018c). DRUG LABEL INFORMATION; CLOPIDOGREL- Clopidogrel Bisulfate Tablet, Film Coated. Available online at: https://dailymed.nlm.nih.gov/dailymed/drugInfo.cfm?setid=edae8df1-caf9-ff72-1304-5ae8b355f8e7
U.S Food and Drug Administration (2018d). DRUG LABEL INFORMATION, 7 DRUG INTERACTIONS, 7.2 CYP2D6 Inhibitors; TOPROL XL- Metoprolol Succinate Tablet. Available online at: https://dailymed.nlm.nih.gov/dailymed/drugInfo.cfmsetid=4a5762c6-d7a2-4e4c-10b7-8832b36fa5f4
van der Weide, J., Steijns, L. S., van Weelden, M. J., and de Haan, K. (2001). The effect of genetic polymorphism of cytochrome P450 CYP2C9 on phenytoin dose requirement. Pharmacogenetics 11, 287–291.
Voora, D., Shah, S. H., Spasojevic, I., Ali, S., Reed, C. R., Salisbury, B. A., et al. (2009). The SLCO1B1*5 genetic variant is associated with statin-induced side effects. J. Am. Coll. Cardiol. 54, 1609–1616. doi: 10.1016/j.jacc.2009.04.053
Weigel, J. D., Hunfeld, N. G., Koch, B. C., Egal, M., Bakker, J., van Schaik, R. H., et al. (2015). Gain-of-function single nucleotide variants of the CYP2C19 gene (CYP2C19*17) can identify subtherapeutic voriconazole concentrations in critically ill patients: a case series. Intensive Care Med. 41, 2013–2014. doi: 10.1007/s00134-015-4002-z
Wen, S. H., Lai, M. J., and Yang, K. L. (2008). Human leukocyte antigen-A, -B, and -DRB1 haplotypes of cord blood units in the Tzu Chi Taiwan Cord Blood Bank. Hum. Immunol. 69, 430–436. doi: 10.1016/j.humimm.2008.05.012
Wong, M., Balleine, R. L., Collins, M., Liddle, C., Clarke, C. L., and Gurney, H. (2004). CYP3A5 genotype and midazolam clearance in Australian patients receiving chemotherapy. Clin. Pharmacol. Ther. 75, 529–538. doi: 10.1016/j.clpt.2004.02.005
Wu, W. D., Wang, Y., Fang, Y. M., and Zhou, H. Y. (2009). Polymorphism of the micro-opioid receptor gene (OPRM1 118A>G) affects fentanyl-induced analgesia during anesthesia and recovery. Mol. Diagn. Ther. 13, 331–337. doi: 10.2165/11317320-000000000-00000
Xu, J., Zhang, X. C., Lv, X. Q., Xu, Y. Y., Wang, G. X., Jiang, B., et al. (2014). Effect of the cytochrome P450 2D6*10 genotype on the pharmacokinetics of tramadol in post-operative patients. Pharmazie 69, 138–141.
Yagar, S., Yavaş, S., and Karahalil, B. (2011). The role of the ADRA2A C1291G genetic polymorphism in response to dexmedetomidine on patients undergoing coronary artery surgery. Mol. Biol. Rep. 38, 3383–3389. doi: 10.1007/s11033-010-0446-y
Young, J., Murthy, L., Westby, M., Akunne, A., and O'Mahony, R. (2010). Diagnosis, prevention, and management of delirium: summary of NICE guidance. BMJ 341:c3704. doi: 10.1136/bmj.c3704
Zhu, H. J., Wang, X., Gawronski, B. E., Brinda, B. J., Angiolillo, D. J., and Markowitz, J. S. (2013). Carboxylesterase 1 as a determinant of clopidogrel metabolism and activation. J. Pharmacol. Exp. Ther. 344, 665–672. doi: 10.1124/jpet.112.201640
Zuo, P., Haberer, L. J., Fang, L., Hunt, T. L., Ridgway, D., and Russo, M. W. (2014). Integration of modeling and simulation to support changes to ondansetron dosing following a randomized, double-blind, placebo-, and active-controlled thorough QT study. J. Clin. Pharmacol. 54, 1221–1229. doi: 10.1002/jcph.322
Keywords: pharmacogenomics, personalized medicine, pharmacogenetics, intensive care unit, adverse drug reaction
Citation: Zhou S, Skaar DJ, Jacobson PA and Huang RS (2018) Pharmacogenomics of Medications Commonly Used in the Intensive Care Unit. Front. Pharmacol. 9:1436. doi: 10.3389/fphar.2018.01436
Received: 03 August 2018; Accepted: 20 November 2018;
Published: 04 December 2018.
Edited by:
Ulrich M. Zanger, Dr. Margarete Fischer-Bosch Institut für Klinische Pharmakologie (IKP), GermanyReviewed by:
Andrew A. Somogyi, University of Adelaide, AustraliaMiia Turpeinen, University of Oulu, Finland
Copyright © 2018 Zhou, Skaar, Jacobson and Huang. This is an open-access article distributed under the terms of the Creative Commons Attribution License (CC BY). The use, distribution or reproduction in other forums is permitted, provided the original author(s) and the copyright owner(s) are credited and that the original publication in this journal is cited, in accordance with accepted academic practice. No use, distribution or reproduction is permitted which does not comply with these terms.
*Correspondence: R. Stephanie Huang, cnNodWFuZ0B1bW4uZWR1