- Department of Pharmaceutical Sciences, College of Pharmacy, The University of Oklahoma Health Sciences Center, Oklahoma City, OK, USA
Targeted therapies have become an important therapeutic paradigm for multiple malignancies. The rapid development of resistance to these therapies impedes the successful management of advanced cancer. Due to the redundancy in angiogenic signaling, alternative proangiogenic factors are activated upon treatment with anti-VEGF agents. Higher doses of the agents lead to greater stimulation of compensatory proangiogenic pathways that limit the therapeutic efficacy of VEGF-targeted drugs and produce escape mechanisms for tumor. Evidence suggests that dose intensity and schedules affect the dynamics of the development of this resistance. Thus, an optimal dosing regimen is crucial to maximizing the therapeutic benefit of antiangiogenic agents and limiting treatment resistance. A systems pharmacology approach using multiscale computational modeling can facilitate a mechanistic understanding of these dynamics of angiogenic biomarkers and their impacts on tumor reduction and resistance. Herein, we discuss a systems pharmacology approach integrating the biology of VEGF-targeted therapy resistance, including circulating biomarkers, and pharmacodynamics to enable the optimization of antiangiogenic therapy for therapeutic gains.
Introduction
Therapeutic intervention in diseases takes place within a milieu of factors, including drug pharmacokinetics, signaling pathways, mechanisms of drug action, and compensatory processes. Studying any single pathway, mechanism of action, or interactive process in isolation has limited value in improving our understanding of the complexity of disease physiology. This is evident in the redundancy of signaling networks, feedback, and cross talk between multiple regulatory processes (Moriya et al., 1996; Pawson and Warner, 2007; Logue and Morrison, 2012). A systems approach is required to quantitatively integrate underlying disease and information contributing to treatment response and resistance. Systems pharmacology combines large scale experimental studies, pharmacokinetics, mechanisms of action, signaling pathways, adaptation mechanisms, biomarker, and pharmacodynamic data in a quantitative framework utilizing computational methods. This approach can facilitate understanding of disease systems, their mechanisms of action and pathways, and hypothesis development (Agoram, 2014). Systems pharmacology further offers a tool for translational considerations from non-clinical models to patients, realizing the bench to bedside paradigm (Allerheiligen, 2010; Kreeger and Lauffenburger, 2010; van der Graaf and Benson, 2011; Demin et al., 2013; Rogers et al., 2013; Vicini and van der Graaf, 2013; Visser et al., 2014). Herein we discuss systems pharmacology approaches to achieve a mechanistic understanding of the dynamics of circulatory biomarkers for antiangiogenic agents, thereby guiding selection of doses that can maximize the therapeutic benefits.
Challenges in Antiangiogenic Therapies
Angiogenesis is critical for tumor growth and metastasis. VEGF signaling is an extensively studied pathway for blocking tumor angiogenesis. Several antiangiogenic agents targeting the VEGF-pathways have been approved and are important modalities in the management of advanced cancers. Bevacizumab, a therapeutic antibody targeting VEGF and various VEGF receptor tyrosine kinase inhibitors (TKIs), have shown clinical benefit in solid tumors. However, the benefits of VEGF-targeted agents are short-lived and resistance to anti-VEGF agents rapidly emerges after an initial response phase, leading to restored tumor growth and progression. This rapid development of resistance to therapy constitutes a major clinical obstacle to providing extended therapeutic benefits with this class of drugs. Thus, effective strategies are needed to delay or prevent resistance to VEGF antiangiogenics.
Resistance to antiangiogenic agents arises through multiple mechanisms, including the activation of compensatory responses that are mediated by malignant cells and stroma cells within the microenvironment. Angiogenesis is a highly adaptive biological process. Tumors can resume angiogenesis and progress using diverse angiogenic signaling, including VEGF, FGF, HGF, PDGF, PlGF, and several proangiogenic cytokines. Numerous compensatory angiogenic factors are upregulated upon anti-VEGF therapy in a dose-dependent manner (Ebos et al., 2007), suggesting that dose intensity and frequency influence the development of therapy resistance. Higher doses of anti-VEGF therapy can create favorable conditions for metastasis by upregulating these growth factors (Ebos et al., 2009a). This emphasizes the importance of finding optimal dosing schedules for anti-VEGF therapy. The current dosing approach does not consider the best way to delay or prevent resistance to VEGF-targeted therapy, and thereby improve patient survival beyond a few months (Jubb et al., 2006; Azad et al., 2008; Cannistra, 2008).
Biologically Effective Doses of anti-VEGF Therapy
Oncology drug development often involves the maximum-tolerated dose (MTD)-based paradigm, even when data suggest that a drug maximally inhibits its target at lower doses. The recent analysis by the U.S. Food and Drug Administration (FDA) showed that inappropriate dose selection was the major cause of post-marketing requirements for oncology drugs approved between 2011 and 2013 (Prowell, 2014). Clinically recommended doses are often derived based on their safety profiles. Toxicity has been the primary end point for conventional dose-finding strategies (Parulekar and Eisenhauer, 2004; Le Tourneau et al., 2009). Since antiangiogenic therapies are mostly cytostatic in nature, they do not always conform to the concept that MTD produces maximum benefits (Sleijfer and Wiemer, 2008). Studies have revealed better therapeutic benefits when lower doses of antiangiogenic therapies were used in combination with other treatments (Kabbinavar et al., 2003; Huang et al., 2012). Similar results were observed with other targeted therapies, such as mammalian target of rapamycin kinase inhibitor, in which a lower dose of 25 mg was selected as the recommended dose for treatment after testing 25, 75, and 250 mg doses (Atkins et al., 2004). Likewise, in a study of 24 consecutive Phase I clinical trials, in which 97.7% of participants received targeted agents, patients receiving lower (≤25% MTD) doses responded as well as those patients receiving medium (25–75% MTD) or high (≥75% MTD) doses (Jain et al., 2010). These findings support the concept that higher doses are not necessarily the most effective.
Higher doses of anti-VEGF therapies can lead to pronounced anti-vascular effects and, subsequently, hypoxia in the tumor, e.g., treatment-induced hypoxia. Treatment-induced hypoxia stimulates several compensatory biological processes to circumvent continued VEGF inhibition, leading to resistance to therapy (Harris, 2002; Casanovas et al., 2005; Drevs et al., 2005; Kerbel, 2005; Mizukami et al., 2005; Hendriksen et al., 2009; Casanovas, 2011). This excessive pruning also leads to reduced delivery of therapies into the tumor (Jain, 2005; Van der Veldt et al., 2012; Van der Veldt and Lammertsma, 2014). Tumors have abnormal vasculature, which leads to an abnormal blood supply that produces hypoxic regions in the tumor. Hypoxia has been also implicated in tumor progression by increasing genomic instability (Nelson et al., 2004) and selection of more malignant cancer stem cells with increased metastatic potential (Bottaro and Liotta, 2003; Conley et al., 2012). Therefore, antiangiogenic therapy can produce more challenges than benefits, if it is inappropriately administered (Huang et al., 2013b; Jain, 2013, 2014). This is consistent with RK Jain’s vascular normalization concept, in which the judicious use of antiangiogenic drugs can lead to more efficient delivery of drugs and oxygen to the tumor cells (Jain, 2005). Utilization of the vascular normalization strategy has been shown to improve cancer immunotherapy (Huang et al., 2012, 2013a) and survival in glioblastoma patients (Sorensen et al., 2012; Emblem et al., 2013). Therefore, there is a critical need to find the biologically effective dose (BED) that balances between normalization and excessive anti-vascular effects from antiangiogenic agents, since suboptimal and higher doses can fail to alleviate hypoxia. Further, the BED can minimize stimulation of alternative, compensatory proangiogenic signals in response to treatment-induced hypoxia, and thus limit the rapid development of treatment resistance, extending the therapeutic benefits of antiangiogenic agents (Jubb et al., 2006; Azad et al., 2008; Cannistra, 2008).
Dynamics of Circulating Angiogenic Biomarkers
The transient effects of antiangiogenic therapy predominantly result from a redundancy in the angiogenesis signaling that mediates tumor escape from anti-VEGF therapy. Many of the signaling molecules (circulating angiogenic factors, or CAF) within these compensatory pathways can be detected systemically in patients treated with VEGF-targeting agents. For example, increases in VEGF and PlGF, and decreases in VEGFR2 can be observed. These changes are considered a “class” effect of VEGF-targeted therapies (Jain et al., 2009). Many of these observed CAF changes are recapitulated in tumor-bearing mice in a dose-dependent manner, and are correlated with antitumor activity (Ebos et al., 2007). Thus, CAF are increasingly recognized as important pharmacodynamic biomarkers for better understanding the treatment response and aiding in the identification of the optimal dosing schedules for VEGF-targeted therapy (Huang et al., 2013b). Understanding the molecular interactions between therapy-induced CAFs and resistance to VEGF-targeted agents can inform the development of strategies to delay or overcome resistance to antiangiogenic therapy (Jain et al., 2009; Clarke and Hurwitz, 2013).
These circulating biomarkers are dynamic, altered over the course of treatment by variables including in vivo drug concentrations (PK), changes in the tumors (e.g., antitumor effect and disease progression), the biological turnover of signaling molecules, compensatory mechanisms, tumor-independent CAF induction by normal cells in the host body, and the development of resistance. These diverse contributing factors create uncertainty when attempting to use dynamic biomarkers. Mathematical modeling can play an important role in understanding and utilizing the biomarkers to find the optimum biological dose and schedule which can delay the onset of therapy resistance (Duda et al., 2013). A recent study showcased the utility of computational models in identifying dosing schedules to manipulate the dynamics of the development of resistance to EGFR-targeted therapy (Foo et al., 2012; Dolgin, 2014). A systems pharmacology approach using multiscale computational modeling offers a tool to integrate the biology of response and resistance to VEGF-targeted therapy, including circulatory biomarkers and the pharmacokinetics/pharmacodynamics of antiangiogenic drugs (Figure 1), to optimize therapeutic gains.
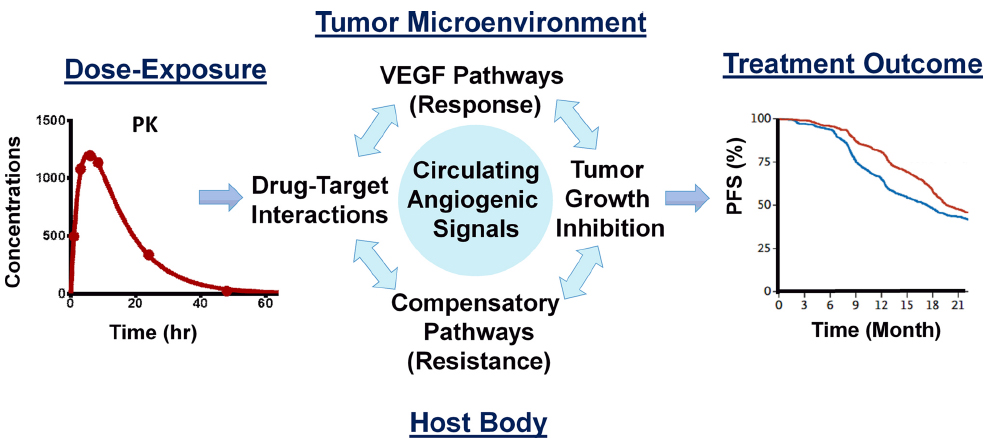
Figure 1. Key components of the systems pharmacology model for anti-VEGF therapy. The model integrates the pharmacokinetics of the drug, antitumor activity, circulating angiogenic biomarkers emanated from host and tumor cells, and therapeutic endpoints based on the drug’s response and compensatory mechanisms within a quantitative framework, to realize a bench to bedside paradigm.
Systems Pharmacology Approach to Antiangiogenic Therapy
The major challenge in developing a systems pharmacology model is how to integrate the dynamics outside the cell (phar macokinetics) with their downstream effects in terms of protein formation or pharmacodynamic effects. PK/PD modeling has been used to explain the relationship between pharmacokinetics and the end downstream effects. What is missing is the mechanistic information in between. Limiting our investigation to antiangiogenic therapy, we anticipate three major challenges to filling this gap: (1) determining the interaction of ligands to their receptors and perturbation by drug molecules, (2) integrating the ensuing signal from these receptors with the downstream protein production machinery, and (3) accounting for interaction between various cell types, that produces pharmacodynamics responses and resistance.
Drug-Target Interaction
Receptor occupancy theory is well-developed and can be readily utilized to integrate this process (Black and Leff, 1983; Black et al., 1985; Mager and Jusko, 2008; Chen et al., 2009; Goodman and Redberg, 2014). We must be mindful that biology is complex and there are many subtypes of ligands, receptors, and co-receptors which have varying degrees of affinity and modulatory functions. Ligand-receptor interaction for angiogenesis involves the VEGF family of ligands (VEGF-A, B, C, D, and PlGF), three main receptors (VEGFR-1, -2, and -3), co-receptors NRP-1 and NRP-2, and heparan sulfate proteoglycans. NRP- and, -2 and proteoglycans play modulatory roles in ligand-receptor interaction; even VEGF-A is alternatively spliced to form VEGFA121, VEGFA145, VEGFA165, and VEGFA189 (Hoeben et al., 2004; Koch et al., 2011; Tugues et al., 2011). Popel and colleagues have contributed extensively to our understanding of the kinetics and interaction of VEGF ligands and receptors (Stefanini et al., 2010; Finley et al., 2011, 2013; Finley and Popel, 2012, 2013; Tan et al., 2013). Although a potential contribution of other ligand and receptor isoforms and families may be recognized, several studies have simplified these interactions by accounting for the most important VEGF ligand, VEGF-A (165), and receptor VEGFR-2 interaction as the major players in angiogenesis (Sharan and Woo, 2014; Zhang et al., 2014). The combination of competitive ligand receptor binding and an inhibitory Hill function model can be used to explain the VEGF-induced VEGFR activation and inhibitor-induced VEGFR inactivation (Sharan and Woo, 2014).
Signal Transduction
Signaling pathways are an important component of a systems pharmacology model, which links receptor-ligand interaction to pharmacodynamic outputs (Iyengar et al., 2012). VEGF binding to its receptors led to the phosphorylation of the tyrosine kinase domain, which in turn initiated the canonical downstream signaling cascades involved in proliferation, migration, survival, and permeability (Tugues et al., 2011). Ordinary differential equation (ODE)-based models, also termed mechanistic or physicochemical models (Birtwistle et al., 2013; Zhang et al., 2014), are often used to describe the canonical signaling cascades. The advantage of this approach being more mechanistic can help a personalized medicine paradigm by incorporating information related to genomic variation and mutation (Iyengar et al., 2012). The limitation of this approach is the currently incomplete mechanistic knowledge of several mediators, signaling processes, and parameter identifiability. Given the incomplete mechanistic knowledge of several mediators and signaling processes, or in the absence of measurement of mediator signaling molecules, a more empirical quantitative logic (QL; Kirouac et al., 2013; Kirouac and Onsum, 2013) or transduction model (Mager and Jusko, 2001) can be utilized to characterize signal transduction. The QL approach has been elegantly explained by Kirouac and Onsum (2013) in building multiscale models which capture the features of oncogenic signaling networks. The transduction model has the flexibility of handling multiscale events with different transit time parameters to account for time needed for signal transduction from receptor-ligand interaction to nucleus, time for cell machinery to form proteins, and to show the pharmacodynamic effects on tumor growth. It is vital to take a balanced approach between mechanistic representation of the signaling pathway and the model’s predictive power (Sharan and Woo, 2014).
Therapeutic and Compensatory Responses to anti-VEGF Therapy
Ideally, signaling events are linked to tumor growth kinetics. Tumor inhibitory effects of anti-VEGF agents can be described by adapting well-established models (Simeoni et al., 2004, 2013; Ribba et al., 2014). In addition to tumor growth inhibition, systemically quantifiable biomarkers such as CAFs can serve as an important measurement to identify disease progression, make dose selections, or stratify patients. Indirect response models can be effectively used to capture inhibition, stimulation, and turnover rates of biomarkers modulation (Mager et al., 2003). We can use non-linear feedback regulation to account for compensatory increases in circulatory biomarkers in response to treatment-induced hypoxia by anti-VEGF agents.
The contributions of host cells and stroma cells within the tumor microenvironment have been increasingly recognized to play an important role in cancer progression and treatment (Ebos et al., 2007, 2009b; Kerbel and Ebos, 2010; Jain, 2013; Stroh et al., 2014). This should be explored using a systems pharmacology model. Antiangiogenic therapies have been shown to upregulate various growth factors in healthy cells and are dose-dependent in non-tumor-bearing mice (Ebos et al., 2007). This dose-dependency is also observed in healthy human volunteers (Lindauer et al., 2010). Thus, it is important to characterize tumor and host cell contributions to CAF modulation and to provide mechanistic information for interpreting biomarker data in respect to antiangiogenic treatments.
Application of a Systems Pharmacology Model of CAFs for Dose Optimization
We have recently developed a systems pharmacology model that uses sunitinib as the test drug to quantify the link between in vivo drug concentrations (PK), target–drug interactions, the biological target pathway, antitumor activity, and compensatory signals leading to treatment resistance (Figure 1). We used the most frequently studied CAFs, including VEGF, PlGF, and sVEGFR2. Our model predictions were consistent with the time- and dose-dependent changes in these hypoxia-derived CAFs following sunitinib given to mice at various dosages (Ebos et al., 2007). We then tested our model within a clinical setting to explain VEGF changes in patients with cancer who experience different treatment outcomes; we found that our predictions were consistent with the observed VEGF changes in patients receiving sunitinib for the treatment of metastatic renal cancer (Kontovinis et al., 2009). The stimulation/inhibition capacity and the hill coefficients of VEGF, PlGF, and sVEGFR2 in mice were similar to those reported in humans, indicating that system-specific parameters for conserved physiological processes such as angiogenesis are comparable across species (Sharan and Woo, 2014).
Our model allows us to delineate CAF changes in the tumor microenvironment and host body during VEGF-targeted therapy and to assess their impacts on tumor response and resistance to therapy. This provided insight into the possible ways to utilize the CAF for better dose guidance of these therapies, either alone or in combination. We found a relationship of tumor reduction and compensatory increase in VEGF and PlGF with increasing sunitinib doses in xenograft mice (Figure 2A). The increase in proangiogenic factors was directly related to the dose, suggesting that these CAF can be used as biomarkers to determine the optimal dose for antiangiogenic drugs. For example, in an A431 xenograft mouse model, the maximum benefit from sunitinib treatment may be achieved at a dose of 20–40 mg/kg/day of sunitinib, as such doses produce no significant changes in VEGF or PlGF levels. Further dose escalation resulted in marginal therapeutic gain (<5%), but significant upregulation of hypoxia-dependent CAF, which may indicate excessive anti-vascular effects. As such, these CAF could be used to construct a therapeutic index for antiangiogenic agents. Figure 2B illustrates this CAF biomarker-based paradigm for dose selections in particular, balancing between antitumor effects and CAF changes. The CAF modulation may serve as a surrogate marker reflecting the anti-vascular effects of antiangiogenic treatment. Ligands of tyrosine kinase receptors have been found to confer resistance by engaging survival signals redundant to those of targeted kinase (Wilson et al., 2012). If we assume that higher changes in compensatory signals are associated with higher likelihood of early onset of resistance, antiangiogenic doses may be increased up to the level at which the CAF increase from their baseline is minimal (e.g., <2-fold for VEGF).
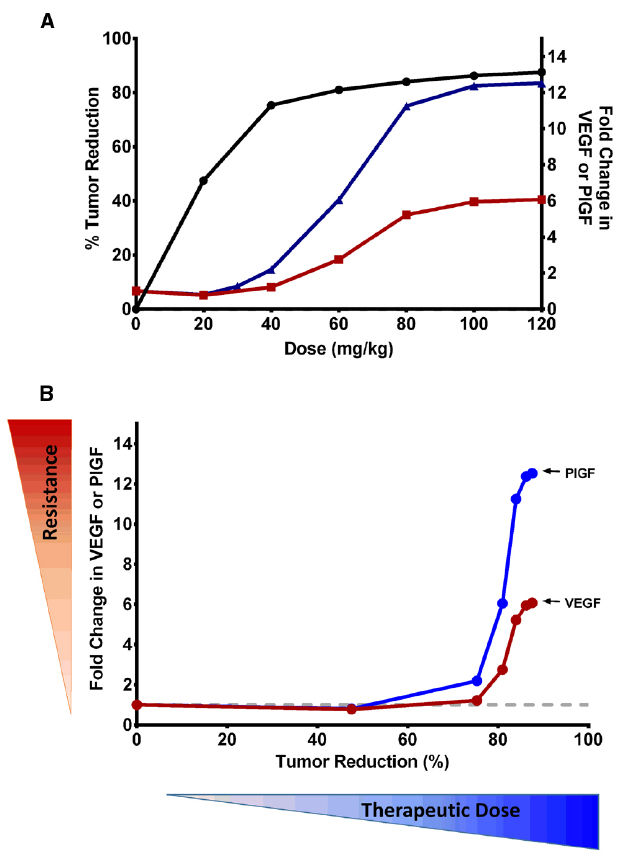
Figure 2. (A) Relationships of therapeutic efficacy and modulation of VEGF and PlGF biomarkers to sunitinib doses. Percentage reduction in tumor volume (•) and fold change in PlGF (▴) and VEGF (▪) are shown at various doses of sunitinib at the end of study. At the dose of 40 mg/kg/day, ∼75% of tumor volume was reduced, with minimal upregulation of hypoxia-dependent CAF. Further dose escalation resulted in marginal therapeutic gain (<5%), but significant upregulation of CAF, which may indicate excessive anti-vascular effects. (B) Utilization of CAF biomarkers in the selection of biological dose of antiangiogenic drugs. The fold change in VEGF and PlGF may serve as a surrogate marker for excessive anti-vascular effects and, in turn, potential for emerging resistance. This illustrates how the biologically effective dose may be selected in a manner which does not invoke significant hypoxia and involves little stimulation of hypoxia-dependent CAF. Monitoring multiple CAFs will be advantageous, as each factor has a different dynamic range. PlGF has a wider dynamic range than VEGF, and results in higher fold change at the same dose. This provides an advantage over VEGF, because PlGF changes are more likely detectable even at lower doses.
Increasing sunitinib doses also led to VEGF and PlGF stimulation with different magnitudes (Figure 2B). This differential stimulation of pro-angiogenic factors can be exploited to facilitate dose finding. Given the inter-individual variability and heterogeneity in tumor response, monitoring multiple biomarkers, rather than relying on a single marker, would be advantageous. We found that PlGF changes were ∼2-fold higher than VEGF changes at the same dose (Sharan and Woo, 2014). This finding suggests that PlGF has a wider dynamic range than VEGF, and can ensure better detection of its change even at lower doses. Thus, monitoring PlGF and VEGF can aid in ensuring that the therapy does not fall below the minimum effective dose nor go above the excessive anti-vasculature dose. This finding is consistent with the recent study in which increased PlGF, but not VEGF, was associated with patients responding to cediranib (Batchelor et al., 2013). While we illustrated the CAF-based dose-finding strategy using VEGF and PlGF, other CAF could be used, as different tumor types and drug targets can invoke different CAF dynamics. Many concepts and the mathematical framework are broadly applicable among several tumor types and different antiangiogenic agents, and could serve as a paradigm for determining the optimal dose of targeted therapies.
Antiangiogenic therapies are often administered in combination with chemotherapy. There is increasing interest in combining antiangiogenics with other targeted therapies in order to improve therapeutic outcomes. However, since the clinical doses of many targeted therapies are determined based on MTD rather than BED, we cannot easily deduce the dosage and schedules of combinations from single agent studies. When antiangiogenic therapies are combined with drugs of same class, excessive overlapping toxicities have resulted (Azad et al., 2008, 2009). In addition, antiangiogenic therapies at higher doses could reduce the efficacy of concomitant cytotoxic agents, most likely due to reduced drug delivery by excessive vessel pruning (Van der Veldt et al., 2012). The CAF biomarker-based approach could also be useful for determining the optimal dose of combination therapy. In combination therapy, the role of antiangiogenic drugs may be focused on vascular normalization. Other therapeutics can be targeted toward killing tumor cells. In such cases, it is desirable for antiangiogenic drugs to be administered at lower doses at which the stimulation of compensatory signaling is minimal.
Conclusion
Therapy-induced CAF can be effectively utilized as pharmacodynamic biomarkers to find the optimal biological dose for antiangiogenic drugs. This will ensure that the therapy maintains minimum effective dose levels, without invoking much compensatory response from the system. Routine incorporation of biomarkers into future clinical trials will be critical for the optimization of anti-VEGF agents and development of next generation of antiangiogenic regimens. Biomarker studies can be augmented by imaging studies, such as dynamic contrast-enhanced MRI (DCE-MRI), or other imaging techniques to monitor vessel integrity, permeability of blood vessels, and tumor perfusion (Murukesh et al., 2010). Thus, future strategies will require circulating biomarkers and imaging with an integrated multi-scale computational tool to guide optimal dose selection for antiangiogenic agents. As more data become available in the future, with the advance of high throughput methods like genomic data, the main challenge will be vertically integrating those data. Systems pharmacology will offer a tool to vertically integrate knowledge from pharmacokinetics, mechanisms of action, genomics, biomarkers, toxicokinetics, and pharmacodynamics. This approach yields an informed perspective from which we can streamline drug discovery and development. The knowledge gained from this approach can provide an in-depth understanding and, hence, a better approach for achieving enduring therapeutic benefits from antiangiogenic therapy.
Conflict of Interest Statement
The authors declare that the research was conducted in the absence of any commercial or financial relationships that could be construed as a potential conflict of interest.
Acknowledgments
Research reported in this publication was supported by the National Institutes of General Medical Sciences of the National Institutes of Health under Award Number P20GM103639. The content is solely the responsibility of the authors and does not necessarily represent the official views of the National Institutes of Health.
References
Agoram, B. (2014). Evaluating systems pharmacology models is different from evaluating standard pharmacokinetic-pharmacodynamic models. CPT Pharmacometrics Syst. Pharmacol. 3, e101. doi: 10.1038/psp.2013.77
Pubmed Abstract | Pubmed Full Text | CrossRef Full Text | Google Scholar
Allerheiligen, S. R. (2010). Next-generation model-based drug discovery and development: quantitative and systems pharmacology. Clin. Pharmacol. Ther. 88, 135–137. doi: 10.1038/clpt.2010.81
Pubmed Abstract | Pubmed Full Text | CrossRef Full Text | Google Scholar
Atkins, M. B., Hidalgo, M., Stadler, W. M., Logan, T. F., Dutcher, J. P., Hudes, G. R., et al. (2004). Randomized phase II study of multiple dose levels of CCI-779, a novel mammalian target of rapamycin kinase inhibitor, in patients with advanced refractory renal cell carcinoma. J. Clin. Oncol. 22, 909–918. doi: 10.1200/JCO.2004.08.185
Pubmed Abstract | Pubmed Full Text | CrossRef Full Text | Google Scholar
Azad, N. S., Aragon-Ching, J. B., Dahut, W. L., Gutierrez, M., Figg, W. D., Jain, L., et al. (2009). Hand-foot skin reaction increases with cumulative sorafenib dose and with combination anti-vascular endothelial growth factor therapy. Clin. Cancer Res. 15, 1411–1416. doi: 10.1158/1078-0432.CCR-08-1141
Azad, N. S., Posadas, E. M., Kwitkowski, V. E., Steinberg, S. M., Jain, L., Annunziata, C. M., et al. (2008). Combination targeted therapy with sorafenib and bevacizumab results in enhanced toxicity and antitumor activity. J. Clin. Oncol. 26, 3709–3714. doi: 10.1200/JCO.2007.10.8332
Pubmed Abstract | Pubmed Full Text | CrossRef Full Text | Google Scholar
Batchelor, T. T., Gerstner, E. R., Emblem, K. E., Duda, D. G., Kalpathy-Cramer, J., Snuderl, M., et al. (2013). Improved tumor oxygenation and survival in glioblastoma patients who show increased blood perfusion after cediranib and chemoradiation. Proc. Natl. Acad. Sci. U.S.A. 110, 19059–19064. doi: 10.1073/pnas.1318022110
Pubmed Abstract | Pubmed Full Text | CrossRef Full Text | Google Scholar
Birtwistle, M. R., Mager, D. E., and Gallo, J. M. (2013). Mechanistic vs. Empirical network models of drug action. CPT Pharmacometrics Syst. Pharmacol. 2, e72. doi: 10.1038/psp.2013.51
Pubmed Abstract | Pubmed Full Text | CrossRef Full Text | Google Scholar
Black, J. W., and Leff, P. (1983). Operational models of pharmacological agonism. Proc. R. Soc. Lond. B Biol. Sci. 220, 141–162. doi: 10.1098/rspb.1983.0093
Pubmed Abstract | Pubmed Full Text | CrossRef Full Text | Google Scholar
Black, J. W., Leff, P., Shankley, N. P., and Wood, J. (1985). An operational model of pharmacological agonism: the effect of E/[A] curve shape on agonist dissociation constant estimation. Br. J. Pharmacol. 84, 561–571. doi: 10.1111/j.1476-5381.1985.tb12941.x
Pubmed Abstract | Pubmed Full Text | CrossRef Full Text | Google Scholar
Bottaro, D. P., and Liotta, L. A. (2003). Cancer: out of air is not out of action. Nature 423, 593–595. doi: 10.1038/423593a
Pubmed Abstract | Pubmed Full Text | CrossRef Full Text | Google Scholar
Cannistra, S. A. (2008). Challenges and pitfalls of combining targeted agents in phase I studies. J. Clin. Oncol. 26, 3665–3667. doi: 10.1200/JCO.2008.17.2676
Pubmed Abstract | Pubmed Full Text | CrossRef Full Text | Google Scholar
Casanovas, O. (2011). The adaptive stroma joining the antiangiogenic resistance front. J. Clin. Invest. 121, 1244–1247. doi: 10.1172/JCI46430
Pubmed Abstract | Pubmed Full Text | CrossRef Full Text | Google Scholar
Casanovas, O., Hicklin, D. J., Bergers, G., and Hanahan, D. (2005). Drug resistance by evasion of antiangiogenic targeting of VEGF signaling in late-stage pancreatic islet tumors. Cancer Cell 8, 299–309. doi: 10.1016/j.ccr.2005.09.005
Pubmed Abstract | Pubmed Full Text | CrossRef Full Text | Google Scholar
Chen, W. W., Schoeberl, B., Jasper, P. J., Niepel, M., Nielsen, U. B., Lauffenburger, D. A., et al. (2009). Input-output behavior of ErbB signaling pathways as revealed by a mass action model trained against dynamic data. Mol. Syst. Biol. 5, 239. doi: 10.1038/msb.2008.74
Pubmed Abstract | Pubmed Full Text | CrossRef Full Text | Google Scholar
Clarke, J. M., and Hurwitz, H. I. (2013). Understanding and targeting resistance to anti-angiogenic therapies. J. Gastrointest. Oncol 4, 253–263. doi: 10.3978/j.issn.2078-6891.2013.036
Pubmed Abstract | Pubmed Full Text | CrossRef Full Text | Google Scholar
Conley, S. J., Gheordunescu, E., Kakarala, P., Newman, B., Korkaya, H., Heath, A. N., et al. (2012). Antiangiogenic agents increase breast cancer stem cells via the generation of tumor hypoxia. Proc. Natl. Acad. Sci. U.S.A. 109, 2784–2789. doi: 10.1073/pnas.1018866109
Pubmed Abstract | Pubmed Full Text | CrossRef Full Text | Google Scholar
Demin, O., Karelina, T., Svetlichniy, D., Metelkin, E., Speshilov, G., Demin, O., et al. (2013). Systems pharmacology models can be used to understand complex pharmacokinetic-pharmacodynamic behavior: an example using 5-lipoxygenase inhibitors. CPT Pharmacometrics Syst. Pharmacol. 2, e74. doi: 10.1038/psp.2013.49
Pubmed Abstract | Pubmed Full Text | CrossRef Full Text | Google Scholar
Dolgin, E. (2014). The mathematician versus the malignancy. Nat. Med. 20, 460–463. doi: 10.1038/nm0514-460
Pubmed Abstract | Pubmed Full Text | CrossRef Full Text | Google Scholar
Drevs, J., Zirrgiebel, U., Schmidt-Gersbach, C. I., Mross, K., Medinger, M., Lee, L., et al. (2005). Soluble markers for the assessment of biological activity with PTK787/ZK 222584 (PTK/ZK), a vascular endothelial growth factor receptor (VEGFR) tyrosine kinase inhibitor in patients with advanced colorectal cancer from two phase I trials. Ann. Oncol. 16, 558–565. doi: 10.1093/annonc/mdi118
Pubmed Abstract | Pubmed Full Text | CrossRef Full Text | Google Scholar
Duda, D. G., Munn, L. L., and Jain, R. K. (2013). Can we identify predictive biomarkers for antiangiogenic therapy of cancer using mathematical modeling? J. Natl. Cancer Inst. 105, 762–765. doi: 10.1093/jnci/djt114
Pubmed Abstract | Pubmed Full Text | CrossRef Full Text | Google Scholar
Ebos, J. M., Lee, C. R., Christensen, J. G., Mutsaers, A. J., and Kerbel, R. S. (2007). Multiple circulating proangiogenic factors induced by sunitinib malate are tumor-independent and correlate with antitumor efficacy. Proc. Natl. Acad. Sci. U.S.A. 104, 17069–17074. doi: 10.1073/pnas.0708148104
Pubmed Abstract | Pubmed Full Text | CrossRef Full Text | Google Scholar
Ebos, J. M., Lee, C. R., Cruz-Munoz, W., Bjarnason, G. A., Christensen, J. G., and Kerbel, R. S. (2009a). Accelerated metastasis after short-term treatment with a potent inhibitor of tumor angiogenesis. Cancer Cell 15, 232–239. doi: 10.1016/j.ccr.2009.01.021
Pubmed Abstract | Pubmed Full Text | CrossRef Full Text | Google Scholar
Ebos, J. M., Lee, C. R., and Kerbel, R. S. (2009b). Tumor and host-mediated pathways of resistance and disease progression in response to antiangiogenic therapy. Clin. Cancer Res. 15, 5020–5025. doi: 10.1158/1078-0432.CCR-09-0095
Pubmed Abstract | Pubmed Full Text | CrossRef Full Text | Google Scholar
Emblem, K. E., Mouridsen, K., Bjornerud, A., Farrar, C. T., Jennings, D., Borra, R. J., et al. (2013). Vessel architectural imaging identifies cancer patient responders to anti-angiogenic therapy. Nat. Med. 19, 1178–1183. doi: 10.1038/nm.3289
Pubmed Abstract | Pubmed Full Text | CrossRef Full Text | Google Scholar
Finley, S. D., Dhar, M., and Popel, A. S. (2013). Compartment model predicts VEGF secretion and investigates the effects of VEGF trap in tumor-bearing mice. Front. Oncol. 3:196. doi: 10.3389/fonc.2013.00196
Pubmed Abstract | Pubmed Full Text | CrossRef Full Text | Google Scholar
Finley, S. D., Engel-Stefanini, M. O., Imoukhuede, P. I., and Popel, A. S. (2011). Pharmacokinetics and pharmacodynamics of VEGF-neutralizing antibodies. BMC Syst. Biol. 5:193. doi: 10.1186/1752-0509-5-193
Pubmed Abstract | Pubmed Full Text | CrossRef Full Text | Google Scholar
Finley, S. D., and Popel, A. S. (2012). Predicting the effects of anti-angiogenic agents targeting specific VEGF isoforms. AAPS J. 14, 500–509. doi: 10.1208/s12248-012-9363-4
Pubmed Abstract | Pubmed Full Text | CrossRef Full Text | Google Scholar
Finley, S. D., and Popel, A. S. (2013). Effect of tumor microenvironment on tumor VEGF during anti-VEGF treatment: systems biology predictions. J. Natl. Cancer Inst. 105, 802–811. doi: 10.1093/jnci/djt093
Pubmed Abstract | Pubmed Full Text | CrossRef Full Text | Google Scholar
Foo, J., Chmielecki, J., Pao, W., and Michor, F. (2012). Effects of pharmacokinetic processes and varied dosing schedules on the dynamics of acquired resistance to erlotinib in EGFR-mutant lung cancer. J. Thorac. Oncol. 7, 1583–1593. doi: 10.1097/JTO.0b013e31826146ee
Pubmed Abstract | Pubmed Full Text | CrossRef Full Text | Google Scholar
Goodman, S. N., and Redberg, R. F. (2014). Opening the FDA black box. JAMA 311, 361–363. doi: 10.1001/jama.2013.283946
Pubmed Abstract | Pubmed Full Text | CrossRef Full Text | Google Scholar
Harris, A. L. (2002). Hypoxia–a key regulatory factor in tumour growth. Nat. Rev. Cancer 2, 38–47. doi: 10.1038/nrc704
Pubmed Abstract | Pubmed Full Text | CrossRef Full Text | Google Scholar
Hendriksen, E. M., Span, P. N., Schuuring, J., Peters, J. P., Sweep, F. C., Van Der Kogel, A. J., et al. (2009). Angiogenesis, hypoxia and VEGF expression during tumour growth in a human xenograft tumour model. Microvasc. Res. 77, 96–103. doi: 10.1016/j.mvr.2008.11.002
Pubmed Abstract | Pubmed Full Text | CrossRef Full Text | Google Scholar
Hoeben, A., Landuyt, B., Highley, M. S., Wildiers, H., Van Oosterom, A. T., and De Bruijn, E. A. (2004). Vascular endothelial growth factor and angiogenesis. Pharmacol. Rev. 56, 549–580. doi: 10.1124/pr.56.4.3
Pubmed Abstract | Pubmed Full Text | CrossRef Full Text | Google Scholar
Huang, Y., Goel, S., Duda, D. G., Fukumura, D., and Jain, R. K. (2013a). Vascular normalization as an emerging strategy to enhance cancer immunotherapy. Cancer Res. 73, 2943–2948. doi: 10.1158/0008-5472.CAN-12-4354
Pubmed Abstract | Pubmed Full Text | CrossRef Full Text | Google Scholar
Huang, Y., Stylianopoulos, T., Duda, D. G., Fukumura, D., and Jain, R. K. (2013b). Benefits of vascular normalization are dose and time dependent–letter. Cancer Res. 73, 7144–7146. doi: 10.1158/0008-5472.CAN-13-1989
Pubmed Abstract | Pubmed Full Text | CrossRef Full Text | Google Scholar
Huang, Y., Yuan, J., Righi, E., Kamoun, W. S., Ancukiewicz, M., Nezivar, J., et al. (2012). Vascular normalizing doses of antiangiogenic treatment reprogram the immunosuppressive tumor microenvironment and enhance immunotherapy. Proc. Natl. Acad. Sci. U.S.A. 109, 17561–17566. doi: 10.1073/pnas.1215397109
Pubmed Abstract | Pubmed Full Text | CrossRef Full Text | Google Scholar
Iyengar, R., Zhao, S., Chung, S. W., Mager, D. E., and Gallo, J. M. (2012). Merging systems biology with pharmacodynamics. Sci. Transl. Med. 4, 126ps127. doi: 10.1126/scitranslmed.3003563
Pubmed Abstract | Pubmed Full Text | CrossRef Full Text | Google Scholar
Jain, R. K. (2005). Normalization of tumor vasculature: an emerging concept in antiangiogenic therapy. Science 307, 58–62. doi: 10.1126/science.1104819
Pubmed Abstract | Pubmed Full Text | CrossRef Full Text | Google Scholar
Jain, R. K. (2013). Normalizing tumor microenvironment to treat cancer: bench to bedside to biomarkers. J. Clin. Oncol. 31, 2205–2218. doi: 10.1200/JCO.2012.46.3653
Pubmed Abstract | Pubmed Full Text | CrossRef Full Text | Google Scholar
Jain, R. K. (2014). An indirect way to tame cancer. Sci. Am. 310, 46–53. doi: 10.1038/scientificamerican0214-46
Pubmed Abstract | Pubmed Full Text | CrossRef Full Text | Google Scholar
Jain, R. K., Duda, D. G., Willett, C. G., Sahani, D. V., Zhu, A. X., Loeffler, J. S., et al. (2009). Biomarkers of response and resistance to antiangiogenic therapy. Nat. Rev. Clin. Oncol. 6, 327–338. doi: 10.1038/nrclinonc.2009.63
Pubmed Abstract | Pubmed Full Text | CrossRef Full Text | Google Scholar
Jain, R. K., Lee, J. J., Hong, D., Markman, M., Gong, J., Naing, A., et al. (2010). Phase I oncology studies: evidence that in the era of targeted therapies patients on lower doses do not fare worse. Clin. Cancer Res. 16, 1289–1297. doi: 10.1158/1078-0432.CCR-09-2684
Pubmed Abstract | Pubmed Full Text | CrossRef Full Text | Google Scholar
Jubb, A. M., Oates, A. J., Holden, S., and Koeppen, H. (2006). Predicting benefit from anti-angiogenic agents in malignancy. Nat. Rev. Cancer 6, 626–635. doi: 10.1038/nrc1946
Pubmed Abstract | Pubmed Full Text | CrossRef Full Text | Google Scholar
Kabbinavar, F., Hurwitz, H. I., Fehrenbacher, L., Meropol, N. J., Novotny, W. F., Lieberman, G., et al. (2003). Phase II, randomized trial comparing bevacizumab plus fluorouracil (FU)/leucovorin (LV) with FU/LV alone in patients with metastatic colorectal cancer. J. Clin. Oncol. 21, 60–65. doi: 10.1200/JCO.2003.10.066
Pubmed Abstract | Pubmed Full Text | CrossRef Full Text | Google Scholar
Kerbel, R. S. (2005). Therapeutic implications of intrinsic or induced angiogenic growth factor redundancy in tumors revealed. Cancer Cell 8, 269–271. doi: 10.1016/j.ccr.2005.09.016
Pubmed Abstract | Pubmed Full Text | CrossRef Full Text | Google Scholar
Kerbel, R. S., and Ebos, J. M. (2010). Peering into the aftermath: the inhospitable host? Nat. Med. 16, 1084–1085. doi: 10.1038/nm1010-1084
Pubmed Abstract | Pubmed Full Text | CrossRef Full Text | Google Scholar
Kirouac, D. C., Du, J. Y., Lahdenranta, J., Overland, R., Yarar, D., Paragas, V., et al. (2013). Computational modeling of ERBB2-amplified breast cancer identifies combined ErbB2/3 blockade as superior to the combination of MEK and AKT inhibitors. Sci. Signal. 6, ra68. doi: 10.1126/scisignal.2004008
Pubmed Abstract | Pubmed Full Text | CrossRef Full Text | Google Scholar
Kirouac, D. C., and Onsum, M. D. (2013). Using network biology to bridge pharmacokinetics and pharmacodynamics in oncology. CPT Pharmacometrics Syst. Pharmacol. 2, e71. doi: 10.1038/psp.2013.38
Pubmed Abstract | Pubmed Full Text | CrossRef Full Text | Google Scholar
Koch, S., Tugues, S., Li, X., Gualandi, L., and Claesson-Welsh, L. (2011). Signal transduction by vascular endothelial growth factor receptors. Biochem. J. 437, 169–183. doi: 10.1042/BJ20110301
Pubmed Abstract | Pubmed Full Text | CrossRef Full Text | Google Scholar
Kontovinis, L. F., Papazisis, K. T., Touplikioti, P., Andreadis, C., Mouratidou, D., and Kortsaris, A. H. (2009). Sunitinib treatment for patients with clear-cell metastatic renal cell carcinoma: clinical outcomes and plasma angiogenesis markers. BMC Cancer 9:82. doi: 10.1186/1471-2407-9-82
Pubmed Abstract | Pubmed Full Text | CrossRef Full Text | Google Scholar
Kreeger, P. K., and Lauffenburger, D. A. (2010). Cancer systems biology: a network modeling perspective. Carcinogenesis 31, 2–8. doi: 10.1093/carcin/bgp261
Pubmed Abstract | Pubmed Full Text | CrossRef Full Text | Google Scholar
Le Tourneau, C., Lee, J. J., and Siu, L. L. (2009). Dose escalation methods in phase I cancer clinical trials. J. Natl. Cancer Inst. 101, 708–720. doi: 10.1093/jnci/djp079
Pubmed Abstract | Pubmed Full Text | CrossRef Full Text | Google Scholar
Lindauer, A., Di Gion, P., Kanefendt, F., Tomalik-Scharte, D., Kinzig, M., Rodamer, M., et al. (2010). Pharmacokinetic/pharmacodynamic modeling of biomarker response to sunitinib in healthy volunteers. Clin. Pharmacol. Ther. 87, 601–608. doi: 10.1038/clpt.2010.20
Pubmed Abstract | Pubmed Full Text | CrossRef Full Text | Google Scholar
Logue, J. S., and Morrison, D. K. (2012). Complexity in the signaling network: insights from the use of targeted inhibitors in cancer therapy. Genes Dev. 26, 641–650. doi: 10.1101/gad.186965.112
Pubmed Abstract | Pubmed Full Text | CrossRef Full Text | Google Scholar
Mager, D. E., and Jusko, W. J. (2001). Pharmacodynamic modeling of time-dependent transduction systems. Clin. Pharmacol. Ther. 70, 210–216. doi: 10.1067/mcp.2001.118244
Pubmed Abstract | Pubmed Full Text | CrossRef Full Text | Google Scholar
Mager, D. E., and Jusko, W. J. (2008). Development of translational pharmacokinetic-pharmacodynamic models. Clin. Pharmacol. Ther. 83, 909–912. doi: 10.1038/clpt.2008.52
Pubmed Abstract | Pubmed Full Text | CrossRef Full Text | Google Scholar
Mager, D. E., Wyska, E., and Jusko, W. J. (2003). Diversity of mechanism-based pharmacodynamic models. Drug Metab. Dispos. 31, 510–518. doi: 10.1124/dmd.31.5.510
Pubmed Abstract | Pubmed Full Text | CrossRef Full Text | Google Scholar
Mizukami, Y., Jo, W. S., Duerr, E. M., Gala, M., Li, J., Zhang, X., et al. (2005). Induction of interleukin-8 preserves the angiogenic response in HIF-1alpha-deficient colon cancer cells. Nat. Med. 11, 992–997. doi: 10.1038/nm1294
Pubmed Abstract | Pubmed Full Text | CrossRef Full Text | Google Scholar
Moriya, S., Kazlauskas, A., Akimoto, K., Hirai, S., Mizuno, K., Takenawa, T., et al. (1996). Platelet-derived growth factor activates protein kinase C epsilon through redundant and independent signaling pathways involving phospholipase C gamma or phosphatidylinositol 3-kinase. Proc. Natl. Acad. Sci. U.S.A. 93, 151–155. doi: 10.1073/pnas.93.1.151
Pubmed Abstract | Pubmed Full Text | CrossRef Full Text | Google Scholar
Murukesh, N., Dive, C., and Jayson, G. C. (2010). Biomarkers of angiogenesis and their role in the development of VEGF inhibitors. Br. J. Cancer 102, 8–18. doi: 10.1038/sj.bjc.6605483
Pubmed Abstract | Pubmed Full Text | CrossRef Full Text | Google Scholar
Nelson, D. A., Tan, T. T., Rabson, A. B., Anderson, D., Degenhardt, K., and White, E. (2004). Hypoxia and defective apoptosis drive genomic instability and tumorigenesis. Genes Dev. 18, 2095–2107. doi: 10.1101/gad.1204904
Pubmed Abstract | Pubmed Full Text | CrossRef Full Text | Google Scholar
Parulekar, W. R., and Eisenhauer, E. A. (2004). Phase I trial design for solid tumor studies of targeted, non-cytotoxic agents: theory and practice. J. Natl. Cancer Inst. 96, 990–997. doi: 10.1093/jnci/djh182
Pubmed Abstract | Pubmed Full Text | CrossRef Full Text | Google Scholar
Pawson, T., and Warner, N. (2007). Oncogenic re-wiring of cellular signaling pathways. Oncogene 26, 1268–1275. doi: 10.1038/sj.onc.1210255
Pubmed Abstract | Pubmed Full Text | CrossRef Full Text | Google Scholar
Prowell, T. (2014). An audience with tatiana prowell. Interviewed by Asher Mullard. Nat. Rev. Drug Discov. 13, 410–411. doi: 10.1038/nrd4335
Pubmed Abstract | Pubmed Full Text | CrossRef Full Text | Google Scholar
Ribba, B., Holford, N. H., Magni, P., Troconiz, I., Gueorguieva, I., Girard, P., et al. (2014). A review of mixed-effects models of tumor growth and effects of anticancer drug treatment used in population analysis. CPT Pharmacometrics Syst. Pharmacol. 3, e113. doi: 10.1038/psp.2014.12
Pubmed Abstract | Pubmed Full Text | CrossRef Full Text | Google Scholar
Rogers, M., Lyster, P., and Okita, R. (2013). NIH support for the emergence of quantitative and systems pharmacology. CPT Pharmacometrics Syst. Pharmacol. 2, e37. doi: 10.1038/psp.2013.13
Pubmed Abstract | Pubmed Full Text | CrossRef Full Text | Google Scholar
Sharan, S., and Woo, S. (2014). Quantitative insight in utilizing circulating angiogenic factors as biomarkers for antiangiogenic therapy: systems pharmacology approach. CPT Pharmacometrics Syst. Pharmacol. 3, e139. doi: 10.1038/psp.2014.36
Pubmed Abstract | Pubmed Full Text | CrossRef Full Text | Google Scholar
Simeoni, M., De Nicolao, G., Magni, P., Rocchetti, M., and Poggesi, I. (2013). Modeling of human tumor xenografts and dose rationale in oncology. Drug Discov. Today Technol. 10, e365–e372. doi: 10.1016/j.ddtec.2012.07.004
Pubmed Abstract | Pubmed Full Text | CrossRef Full Text | Google Scholar
Simeoni, M., Magni, P., Cammia, C., De Nicolao, G., Croci, V., Pesenti, E., et al. (2004). Predictive pharmacokinetic-pharmacodynamic modeling of tumor growth kinetics in xenograft models after administration of anticancer agents. Cancer Res. 64, 1094–1101. doi: 10.1158/0008-5472.CAN-03-2524
Pubmed Abstract | Pubmed Full Text | CrossRef Full Text | Google Scholar
Sleijfer, S., and Wiemer, E. (2008). Dose selection in phase I studies: why we should always go for the top. J. Clin. Oncol. 26, 1576–1578. doi: 10.1200/JCO.2007.15.5192
Pubmed Abstract | Pubmed Full Text | CrossRef Full Text | Google Scholar
Sorensen, A. G., Emblem, K. E., Polaskova, P., Jennings, D., Kim, H., Ancukiewicz, M., et al. (2012). Increased survival of glioblastoma patients who respond to antiangiogenic therapy with elevated blood perfusion. Cancer Res. 72, 402–407. doi: 10.1158/0008-5472.CAN-11-2464
Pubmed Abstract | Pubmed Full Text | CrossRef Full Text | Google Scholar
Stefanini, M. O., Wu, F. T., Mac Gabhann, F., and Popel, A. S. (2010). Increase of plasma VEGF after intravenous administration of bevacizumab is predicted by a pharmacokinetic model. Cancer Res. 70, 9886–9894. doi: 10.1158/0008-5472.CAN-10-1419
Pubmed Abstract | Pubmed Full Text | CrossRef Full Text | Google Scholar
Stroh, M., Duda, D. G., Takimoto, C. H., Yamazaki, S., and Vicini, P. (2014). Translation of anticancer efficacy from nonclinical models to the clinic. CPT Pharmacometrics Syst. Pharmacol. 3, e128. doi: 10.1038/psp.2014.28
Pubmed Abstract | Pubmed Full Text | CrossRef Full Text | Google Scholar
Tan, W. H., Popel, A. S., and Mac Gabhann, F. (2013). Computational model of VEGFR2 pathway to ERK activation and modulation through receptor trafficking. Cell. Signal. 25, 2496–2510. doi: 10.1016/j.cellsig.2013.08.015
Pubmed Abstract | Pubmed Full Text | CrossRef Full Text | Google Scholar
Tugues, S., Koch, S., Gualandi, L., Li, X., and Claesson-Welsh, L. (2011). Vascular endothelial growth factors and receptors: anti-angiogenic therapy in the treatment of cancer. Mol. Aspects Med. 32, 88–111. doi: 10.1016/j.mam.2011.04.004
Pubmed Abstract | Pubmed Full Text | CrossRef Full Text | Google Scholar
van der Graaf, P. H., and Benson, N. (2011). Systems pharmacology: bridging systems biology and pharmacokinetics-pharmacodynamics (PKPD) in drug discovery and development. Pharm. Res. 28, 1460–1464. doi: 10.1007/s11095-011-0467-9
Pubmed Abstract | Pubmed Full Text | CrossRef Full Text | Google Scholar
Van der Veldt, A. A., and Lammertsma, A. A. (2014). In vivo imaging as a pharmacodynamic marker. Clin. Cancer Res. 20, 2569–2577. doi: 10.1158/1078-0432.CCR-13-2666
Pubmed Abstract | Pubmed Full Text | CrossRef Full Text | Google Scholar
Van der Veldt, A. A., Lubberink, M., Bahce, I., Walraven, M., De Boer, M. P., Greuter, H. N., et al. (2012). Rapid decrease in delivery of chemotherapy to tumors after anti-VEGF therapy: implications for scheduling of anti-angiogenic drugs. Cancer Cell 21, 82–91. doi: 10.1016/j.ccr.2011.11.023
Pubmed Abstract | Pubmed Full Text | CrossRef Full Text | Google Scholar
Vicini, P., and van der Graaf, P. H. (2013). Systems pharmacology for drug discovery and development: paradigm shift or flash in the pan? Clin. Pharmacol. Ther. 93, 379–381. doi: 10.1038/clpt.2013.40
Pubmed Abstract | Pubmed Full Text | CrossRef Full Text | Google Scholar
Visser, S. A., De Alwis, D. P., Kerbusch, T., Stone, J. A., and Allerheiligen, S. R. (2014). Implementation of quantitative and systems pharmacology in large pharma. CPT Pharmacometrics Syst. Pharmacol. 3, e142. doi: 10.1038/psp.2014.40
Pubmed Abstract | Pubmed Full Text | CrossRef Full Text | Google Scholar
Wilson, T. R., Fridlyand, J., Yan, Y., Penuel, E., Burton, L., Chan, E., et al. (2012). Widespread potential for growth-factor-driven resistance to anticancer kinase inhibitors. Nature 487, 505–509. doi: 10.1038/nature11249
Pubmed Abstract | Pubmed Full Text | CrossRef Full Text | Google Scholar
Zhang, X. Y., Birtwistle, M. R., and Gallo, J. M. (2014). A general network pharmacodynamic model-based design pipeline for customized cancer therapy applied to the VEGFR pathway. CPT Pharmacometrics Syst. Pharmacol. 3, e92. doi: 10.1038/psp.2013.65
Pubmed Abstract | Pubmed Full Text | CrossRef Full Text | Google Scholar
Keywords: systems pharmacology, biomarkers, dose selection, antiangiogenic therapy, resistance, targeted therapies, biologically effective dose, bed
Citation: Sharan S and Woo S (2015) Systems pharmacology approaches for optimization of antiangiogenic therapies: challenges and opportunities. Front. Pharmacol. 6:33. doi: 10.3389/fphar.2015.00033
Received: 05 September 2014; Accepted: 06 February 2015;
Published online: 20 February 2015.
Edited by:
Tarek A. Leil, Bristol-Myers Squibb, USAReviewed by:
Xiaogang Wu, Institute for Systems Biology, USAJeffrey S. Barrett, Sanofi Pharmaceuticals, USA
Copyright © 2015 Sharan and Woo. This is an open-access article distributed under the terms of the Creative Commons Attribution License (CC BY). The use, distribution or reproduction in other forums is permitted, provided the original author(s) or licensor are credited and that the original publication in this journal is cited, in accordance with accepted academic practice. No use, distribution or reproduction is permitted which does not comply with these terms.
*Correspondence: Sukyung Woo, Department of Pharmaceutical Sciences, College of Pharmacy, The University of Oklahoma Health Sciences Center, CPB 331, 1110 N. Stonewall Avenue, Oklahoma City, OK 73117, USA e-mail: sukyung-woo@ouhsc.edu