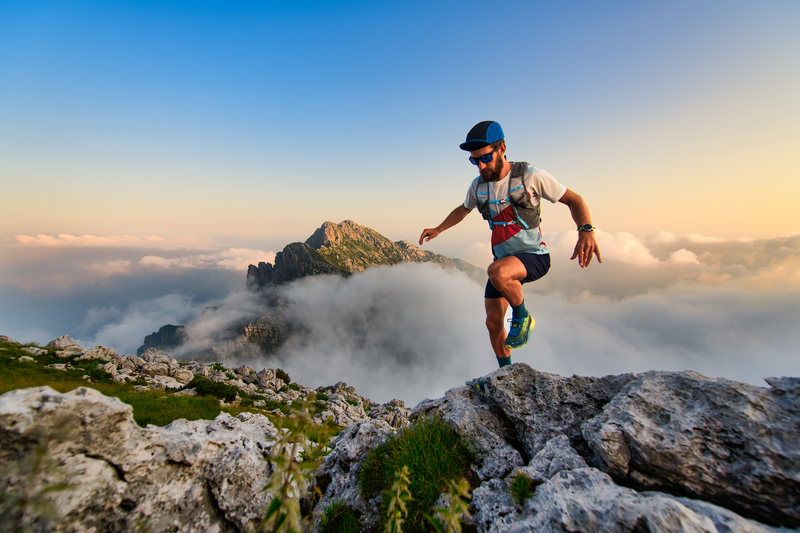
95% of researchers rate our articles as excellent or good
Learn more about the work of our research integrity team to safeguard the quality of each article we publish.
Find out more
BRIEF RESEARCH REPORT article
Front. Pediatr.
Sec. Pediatric Critical Care
Volume 13 - 2025 | doi: 10.3389/fped.2025.1544404
This article is part of the Research Topic Advances in Pediatric Rehabilitation Clinical Trials: Design, Methods, and Analysis View all 8 articles
The final, formatted version of the article will be published soon.
You have multiple emails registered with Frontiers:
Please enter your email address:
If you already have an account, please login
You don't have a Frontiers account ? You can register here
Introduction: Mobile monitoring devices offer an opportunity to characterize physical health recovery in children who survive critical illness.Methods: To validate the BioIntelliSense BioButton® as a pediatric activity monitor, we studied healthy children (2-17 years-old) who wore the BioButton® device and an ActiGraph wGT3X-BT accelerometer, and a study team member documented activity in 1-minute intervals (gold standard) during 45 minutes of scripted activities. In two-thirds of the cohort (derivation cohort), we identified BioButton activity count thresholds to differentiate activity levels based on highest Youden indices. Thresholds were applied to the remainder of the cohort (validation cohort) to determine sensitivity and specificity (95% confidence interval [CI]). We also evaluated BioButton activity designations compared with accelerometer designations and calculated agreement between BioButton-measured body position and the activity log.Results: Forty-five participants provided a median 43 (IQR 41, 44) analyzable minutes. Sensitivity and specificity of derived BioButton thresholds were 0.78 (95%CI 0.69, 0.88) and 0.95 (95%CI 0.90, 0.97) to identify moderate or vigorous activity (MVPA) and 0.91 (95%CI 0.87, 0.95) and 0.98 (95%CI 0.98, 0.98) to identify sedentary behavior. Sensitivity and specificity compared with the accelerometer were 0.52 (95%CI 0.45-0.60) and 0.88 (95%CI (95%CI 0.84, 0.93) to identify MVPA and 0.92 (95%CI 0.89-0.96) and 0.70 (95%CI 0.67, 0.73) to identify sedentary behavior. The BioButton accurately identified position during 1,125 of 1,432 (78.6%) minutes.Discussion: The BioButton device accurately identified physical activity and body position in children and may be a useful tool to quantify physical activity as an outcome in future trials.
Keywords: Exercise, sedentary behavior, Patient outcome assessment (MeSH), pediatrics - children, Critical care outcomes
Received: 12 Dec 2024; Accepted: 13 Mar 2025.
Copyright: © 2025 Petruccelli, Miller, Greer, Sauceda, Watson, Mourani and Maddux. This is an open-access article distributed under the terms of the Creative Commons Attribution License (CC BY). The use, distribution or reproduction in other forums is permitted, provided the original author(s) or licensor are credited and that the original publication in this journal is cited, in accordance with accepted academic practice. No use, distribution or reproduction is permitted which does not comply with these terms.
* Correspondence:
Aline B Maddux, Pediatrics, Section of Critical Care, University of Colorado School of Medicine, Aurora, Colorado, United States
Disclaimer: All claims expressed in this article are solely those of the authors and do not necessarily represent those of their affiliated organizations, or those of the publisher, the editors and the reviewers. Any product that may be evaluated in this article or claim that may be made by its manufacturer is not guaranteed or endorsed by the publisher.
Research integrity at Frontiers
Learn more about the work of our research integrity team to safeguard the quality of each article we publish.