- 1Department of Pediatrics, University of Colorado Anschutz Medical Campus, Aurora, CO, United States
- 2Department of Biostatistics and Informatics, University of Colorado Anschutz Medical Campus, Aurora, CO, United States
- 3Department of Surgery, University of Colorado Anschutz Medical Campus, Aurora, CO, United States
- 4Department of Anesthesia, University of Colorado Anschutz Medical Campus, Aurora, CO, United States
Introduction: Children with single ventricle heart disease (SVHD) experience significant morbidity across systems and time, with 70% of patients experiencing acute kidney injury, 33% neurodevelopmental impairment, 14% growth failure, and 5.5% of patients suffering necrotizing enterocolitis. Proteomics is a method to identify new biomarkers and mechanisms of injury in complex physiologic states.
Methods: Infants with SVHD in the interstage period were compared to similar-age healthy controls. Serum samples were collected, stored at −80°C, and run on a panel of 1,500 proteins in single batch analysis (Somalogic Inc., CO). Partial Least Squares-Discriminant Analysis (PLS-DA) was used to compare the proteomic profile of cases and controls and t-tests to detect differences in individual proteins (FDR <0.05). Protein network analysis with functional enrichment was performed in STRING and Cytoscape.
Results: PLS-DA readily discriminated between SVHD cases (n = 33) and controls (n = 24) based on their proteomic pattern alone (Accuracy = 0.96, R2 = 0.97, Q2 = 0.80). 568 proteins differed between groups (FDR <0.05). We identified 25 up-regulated functional clusters and 13 down-regulated. Active biological systems fell into six key groups: angiogenesis and cell proliferation/turnover, immune system activation and inflammation, altered metabolism, neural development, gastrointestinal system, and cardiac physiology and development.
Conclusions: We report a clear differentiation in the circulating proteome of patients with SVHD and healthy controls with >500 circulating proteins distinguishing the groups. These proteomic data identify widespread protein dysregulation across multiple biologic systems with promising biological plausibility as drivers of SVHD morbidity.
1. Introduction
Single ventricle heart disease (SVHD) occurs in between 2 and 8 in 10,000 live births and is associated with some of the highest morbidity and mortality in patients born with congenital heart disease (CHD) (1). Most patients undergo a staged series of palliative surgical repairs, culminating in total cavopulmonary anastomosis. The first procedure, or stage 1, is performed shortly after birth, stage 2 (Glenn or hemi-Fontan) at 4–6 months of life, and a final stage 3 procedure (Fontan) between 2 and 5 years of age (2). The highest morbidity and mortality period occurs between stages 1 and 2, a particularly vulnerable time termed the “interstage period.” (3) During this time, the single ventricle pumps in parallel to the systemic and pulmonary circulations. The ratio of flow delivered to each circulation is dynamic and dependent on relative vascular resistances. This physiology results in chronic hypoxemia and leaves infants at risk of unbalanced circulation (excess or insufficient pulmonary blood flow), especially during times of illness, fever, dehydration, vagal events, or other effectors of pulmonary and systemic vascular resistance (3, 4).
Single ventricle physiology yields variable, and at times unstable, perfusion and oxygen delivery resulting in both acute and long-term sequelae across organ systems. Excess pulmonary blood flow (PBF) leads to adverse vascular remodeling and in the long-term causes pulmonary hypertension, hypoxemia, venous congestion, and congestive heart failure (5, 6). Conversely, insufficient PBF leads to severe hypoxemia (7). Dysregulated systemic blood flow results in differential organ-specific blood flow and, in combination with baseline hypoxemia, has whole-body downstream consequences. Examples include decreased cerebral blood flow, which correlates with lower IQ (8, 9), mesenteric ischemia resulting in necrotizing enterocolitis (NEC) (10), and chronic kidney disease from episodic low renal blood flow on a background of chronic hypoxia (11). While the multiorgan clinical sequelae of SVHD have been well-described, a gap exists in our understanding of the systemic derangement at the cellular and molecular levels. Moreover, we do not fully understand how individual molecular changes interact across larger biological systems and how those systems drive observed clinical sequelae.
Proteomic techniques may help address this gap. Proteomics allows for large-scale protein exploration to define the extent of individual protein dysregulation experienced by children with SVHD and the underlying biological networks. Our group has begun to utilize “omics” to explore the shifts in the global metabolome and proteome of patients with SVHD. Prior work from our group demonstrated a unique targeted cardiovascular proteomic profile in patients with SVHD compared to healthy controls (12). However, the breadth of circulating protein dysregulation experienced by infants with interstage SVHD has not been well defined. In this study, we performed an expanded analysis of proteins across a broad range of biological systems to characterize the circulating proteome in infants awaiting Stage 2 palliation compared to healthy controls. We hypothesized that the circulating proteome is widely affected by SVHD physiology with diffusely altered biological systems arising from the heart, kidneys, brain, gastrointestinal tract, and immune system.
2. Materials and methods
The Colorado Multiple Institutional Review Board approved this study. Written informed consent was obtained from the study subjects’ parents or legal guardians in all cases. This study is a pre-specified aim of a larger parent study examining longitudinal circulating biomarkers of pulmonary vascular development in SVHD patients.
2.1. Subjects
We prospectively enrolled patients at our center with SVHD in the interstage period prior to their Stage 2 surgical palliation. We defined Stage 2 palliation to include any form of superior cavopulmonary anastomosis (Glenn or Hemi-Fontan operations) regardless of having had a Stage 1 palliation. In addition, we recruited similar-aged control subjects from our institution's broader non-cardiac surgical schedule, who were aged 3 to 12 months and undergoing anesthesia for elective, non-cardiac procedures. We excluded control subjects with known or suspected cardiac, pulmonary, infectious, or genetic abnormalities. Complete details regarding inclusion and exclusion criteria for the parent cohort have previously been published (12).
2.2. Sample collection and processing
All samples were collected under general anesthesia. When possible, systemic venous samples from SVHD subjects were obtained at the time of pre-stage 2 cardiac catheterization. Otherwise, a systemic venous sample was collected in the CVOR prior to the first incision. For control subjects, we obtained systemic venous samples after induction of anesthesia at the time of IV placement. All whole blood samples were processed for serum at the time of collection and then aliquoted into 200 μl samples and frozen at −80°C until analysis.
2.3. Protein quantification assays
We analyzed samples in a single batch using a DNA-based aptamer assay (SomaLogic, Boulder, CO) with a custom panel of 1,500 proteins selected to represent markers of organ function from a wide variety of systems (Supplementary Table S1). For each protein target, chemically labeled nucleotide-antibody pairs were bound to specific epitopes on the protein surface. Complementary nucleotide sequences gave rise to DNA reporter sequences, which were then quantified using real-time PCR (13). The assay measured a dynamic range of absolute protein quantities across 10 logs with a sensitivity down to 125 fM (14). Log-scaled normalized protein expression values were adjusted by a negative control sample. Higher expression values correspond to higher protein levels but are not an absolute quantification of protein concentrations. Given that chronic dysregulation at the organ level may be associated with only modest alterations in the circulating proteome, we elected to err on the side of inclusivity and not impose a low-fold change filter.
2.4. Identification of significant proteins
We used Metaboanalyst 5.0 (www.metaboanalyst.ca, RRID:SCR_015539) (15) to find differences in the proteome between cases and controls. Protein concentrations were log-transformed and auto-scaled (mean-centered and divided by the square root of the standard deviation of each variable) for normalization. We then analyzed the normalized data for global proteome differences using Partial Least Squares-Discriminant Analysis (PLS-DA) followed by model cross-validation (R2, Q2). Variable Importance in Projection (VIP) multivariate regression identified the top 35 proteins distinguishing cases and controls. For cases alone, secondary analyses to assess the association between key covariates and the global proteome were done using Principal Component Analysis (PCA). Covariates tested included sex, ventricular morphology, and history of surgical Stage 1 palliation. As cases and controls were not perfectly matched for age or weight, we sought to rule out a significant proteomic signal related to variation of age and weight. To do so, we performed simple linear regressions in controls, separately comparing each protein with age and weight and correcting for multiple comparisons using the Benjamini-Hochberg method (i.e., False Discovery Rate, FDR) (16). To identify individual proteins that differed between cases and controls, we then performed t-tests on the entire panel to identify all proteins that differed significantly between cases and controls with FDR multiple comparisons correction. We defined the findings to be statistically significant at an FDR <0.05. All further protein analysis was performed on the significantly different proteins alone.
2.5. Protein-protein interaction analysis
Due to the incomplete measurement of the proteome inherent even to large targeted proteomics assays such as ours, we used a network-based method, GenePlexus (17, 18), to infer additional untested proteins strongly related (>99% probability) to the original significant protein list. We then imported this expanded list of statistically significant proteins into STRING (string-db.org, RRID:SCR_005223) (19) to create protein-protein interaction (PPI) networks. Interactions were determined by a combination of evidence sources, including experimental data, co-expression, co-occurrence, gene fusion, neighborhood interactions, and literature text mining. As this work was exploratory in nature, we constrained interactions to “medium confidence” interactions (i.e., setting 0.4 as the minimum required interaction score). We created two primary PPI networks: one for up-regulated and one for down-regulated proteins. To understand functional subnetworks within each larger PPI network, we clustered up-regulated and down-regulated differentially expressed proteins (DEPs) using Markov Clustering (MCL) with an inflation parameter of 3.0. We further clustered large (≥100 proteins) clusters using an inflation parameter of 5.0 to drive cluster granularity, such that no single sub-cluster contained greater than 99 proteins (20). All proteins are referred to by their gene name according to the HUGO Gene Nomenclature Committee naming system (RRID:SCR_012800).
2.6. Functional enrichment analysis: classification of biological networks and systems
Functional enrichment analysis was performed using STRING, specifically looking at the enrichment of Gene Ontology (GO) biological processes. First, we performed GO analysis on the broader up and down-regulated networks as well as each cluster and sub-cluster (21, 22). We then grouped individual GO terms identified at the cluster level into biologically similar groups to better understand the broader biological networks and systems. For grouped GO terms, the lowest p-value in the group is reported. Finally, we imported clusters into Cytoscape (23) (RRID:SCR_003032) to analyze node degrees, a numerical reflection of the connectivity of each protein (node), and edges (total number of connections in the cluster). Cluster visualization was performed in Cytoscape.
3. Results
3.1. Study population
We enrolled 33 patients with SVHD prior to Stage 2 palliation and 24 controls. All patients were included in the analysis and pre-operative systemic vein samples were available for all subjects. SVHD subjects had various underlying diagnoses, the most common being hypoplastic left heart syndrome (HLHS). Of the 33 SVHD patients, 27 were palliated to a single right ventricle, and the remaining six were palliated to a single left ventricle (Table 1). Twenty-two of 33 single ventricle patients had previously undergone a Norwood operation. The remaining 11 patients had either not undergone Stage 1 surgical repair or had a more limited initial palliation (e.g., systemic to pulmonary artery shunting alone or pulmonary artery banding). PCA demonstrated no significant differentiation of the circulating proteome by sex, ventricular morphology, or completion of Stage 1 palliation (Supplementary Figure S1). The most common surgical indications in controls were urogenital malformations or elective urogenital procedures. Owing to the elective nature of these procedures, controls were, on average older and heavier, differences which met statistical significance. The average age at the time of sample collection was 3.41 (±1.19) months in cases and 8.42 (±2.20) months in controls. The average weight was 5.78 (±0.66) kg in cases and 8.07 (±0.89) kg in controls. Simple linear regression analysis identified no statistically significant relationship in any protein with age or weight (FDR <0.05).
3.2. Comparison of case and control proteomic patterns and differential protein expression
PLS-DA demonstrated clear discrimination between SVHD cases and controls based on their proteomic patterns alone (Accuracy = 0.96, R2 = 0.97, Q2 = 0.80) (Figure 1A). VIP identified the 35 proteins contributing most to the predictive power of the PLS-DA model and thus on proteome differentiation (Figure 1B). Thirty of the top 35 proteins were up-regulated in SVHD patients, functioning across multiple biological systems. The biological processes most represented include angiogenesis, immune system regulation, and neuronal morphogenesis. The protein with the single highest effect in distinguishing cases and controls was NPPB, coding for B-type Natriuretic Peptide (BNP). T-tests showed 568 proteins differed significantly between groups at FDR <0.05. In total, 366 proteins were up-regulated, and 202 were down-regulated, demonstrating an overall trend toward protein up-regulation in SVHD patients.
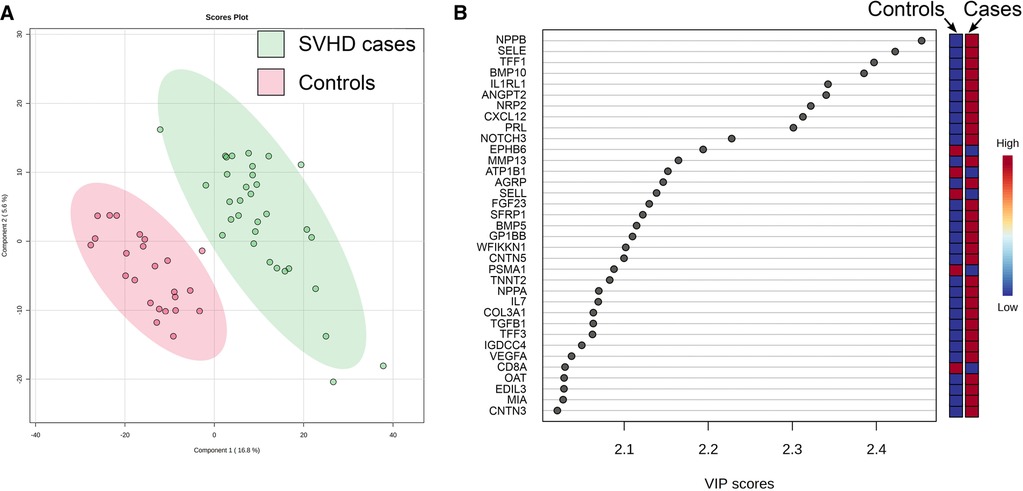
Figure 1. (A) Partial least squares-determinant analysis (PLS-DA) readily discriminated between SVHD cases (n = 33) and controls (n = 24) based on their proteomic pattern alone. Each circle represents the distilled proteome of one subject. (B) Variable Importance in Projection (VIP) analysis shows the 35 proteins with the highest effect in distinguishing the proteomes of SVHD cases and controls. Relative up and down-regulation of each protein (high vs. low) demonstrates an overall trend toward up-regulation in SVHD cases.
3.3. Protein-protein interaction analysis and functional enrichment
First, the original list of significant proteins was expanded using the network-based computational method, GenePlexus. GenePlexus identified 19 additional proteins that had a >99% probability of being associated with the original set. We imported these 587 DEPs into STRING and generated a network with 586 nodes and 7,047 edges. The PPI enrichment for the network, a value estimating the number of observed vs. expected protein interactions for a specific network size, was found to be highly statistically significant (p < 1 × 10−16).
Next, we performed a more in-depth analysis of the up and down-regulated protein lists separately. Running GenePlexus on each of these lists identified 20 additional proteins to the up-regulated protein set, for a total of 386 up-regulated proteins. No additional proteins were identified in the down-regulated group. To discover the active biological systems in our cases, we performed MCL on the up and down-regulated networks separately. MCL identified 25 major (≥3 nodes) clusters in up-regulated proteins (Table 2) and 13 major clusters in down-regulated proteins (Table 3). We grouped enriched GO terms across six broad categories (Figure 2). Most unified GO groups consisted of multiple clusters. A list of unifying GO terms assigned to each cluster can be found in Supplementary Tables S2 and S3.
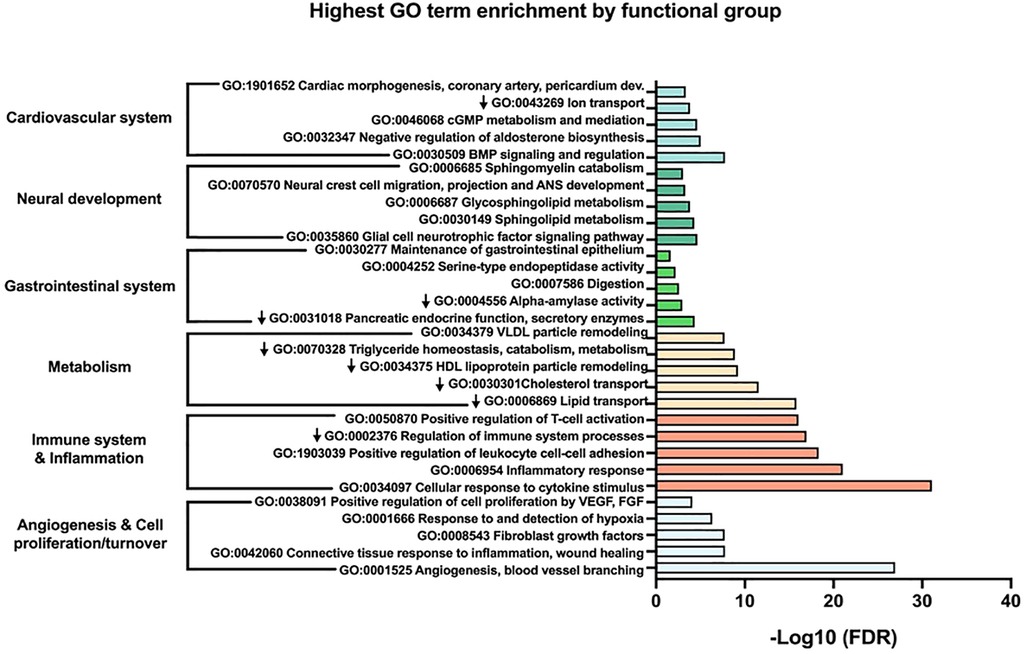
Figure 2. The top five most significant gene ontology (GO) terms within each larger functional group are shown. Down arrows signify GO terms from down-regulated clusters.
3.4. Angiogenesis and cell proliferation/turnover
The largest and most highly connected protein cluster in our network, up-regulated cluster 1, centered on angiogenesis and cell proliferation/turnover (Figure 3). Cluster 1 had 112 proteins by initial clustering. Using an inflation factor of 5, we generated four sub-clusters, with cluster U1a remaining large at 89 proteins. This large sub-cluster comprised angiogenic factors (Figure 3, cluster U1a). There are no down-regulated clusters mediating angiogenesis. The remaining sub-clusters were centered on cell proliferation and extracellular matrix (ECM) changes (Figure 3, clusters U1b-d). Two additional clusters also contained ECM proteins (Figure 3, clusters U25, D2).
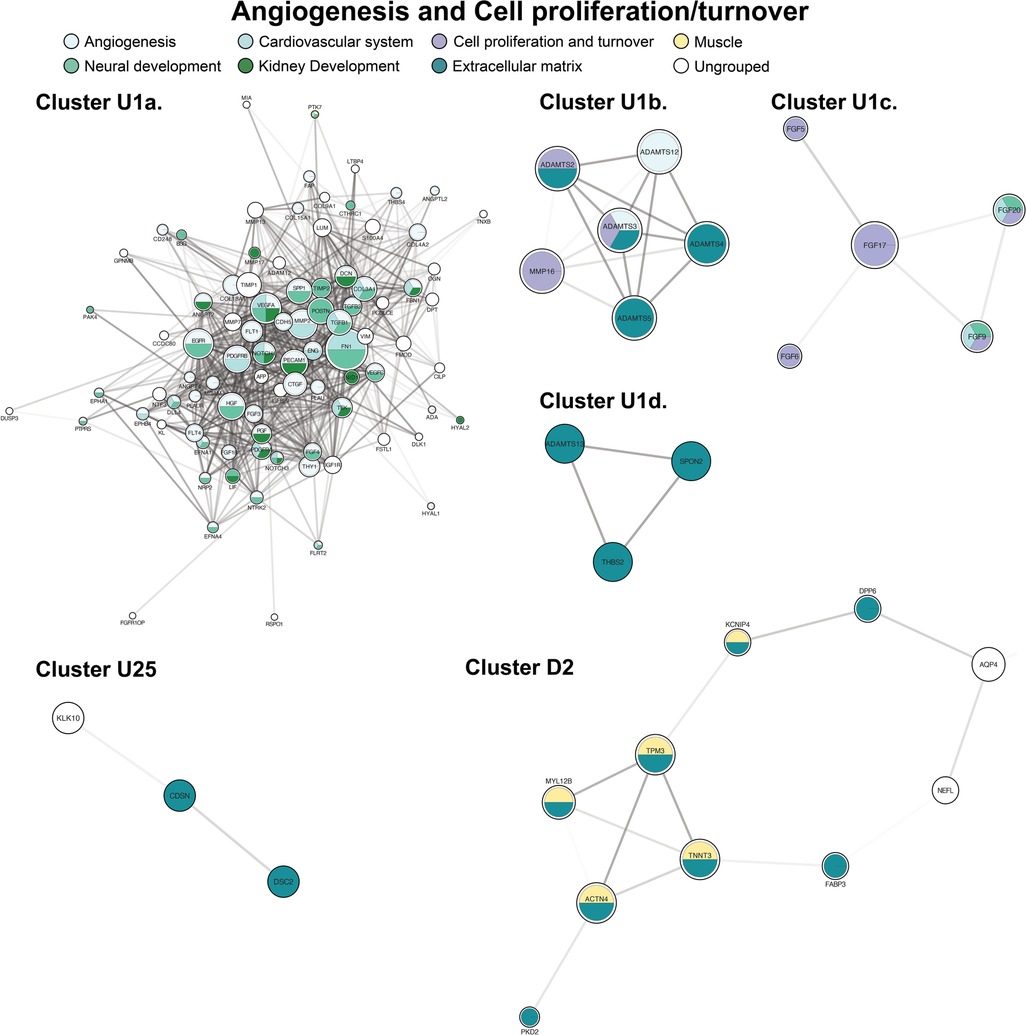
Figure 3. Clusters of angiogenesis and cell proliferation and turnover. Clusters with a “U” designation were up-regulated and clusters with a “D” designation were down-regulated. Figure protein (node) size is reflective of the degree of connectivity of the protein. Transparency of connecting lines (edges) is reflective of the “interaction score”, or the relative confidence in interaction by STRING.
3.5. Immune system activation and inflammation
The second largest cluster, cluster U2, contained 69 proteins and focused on immune system regulation with co-expression of inflammatory cascades (Figure 4, cluster U2). A predominant group of proteins acted as activators of the adaptive immune system. Two down-regulated clusters were also highly enriched by adaptive and innate immune system mediators (Figure 4, clusters D1, D4). Apoptotic proteins were included within several clusters (principally Figure 4, clusters U1, U13). In contrast, antioxidants were among the proteins expressed in the principal down-regulated immune cluster (Figure 4, cluster D1).
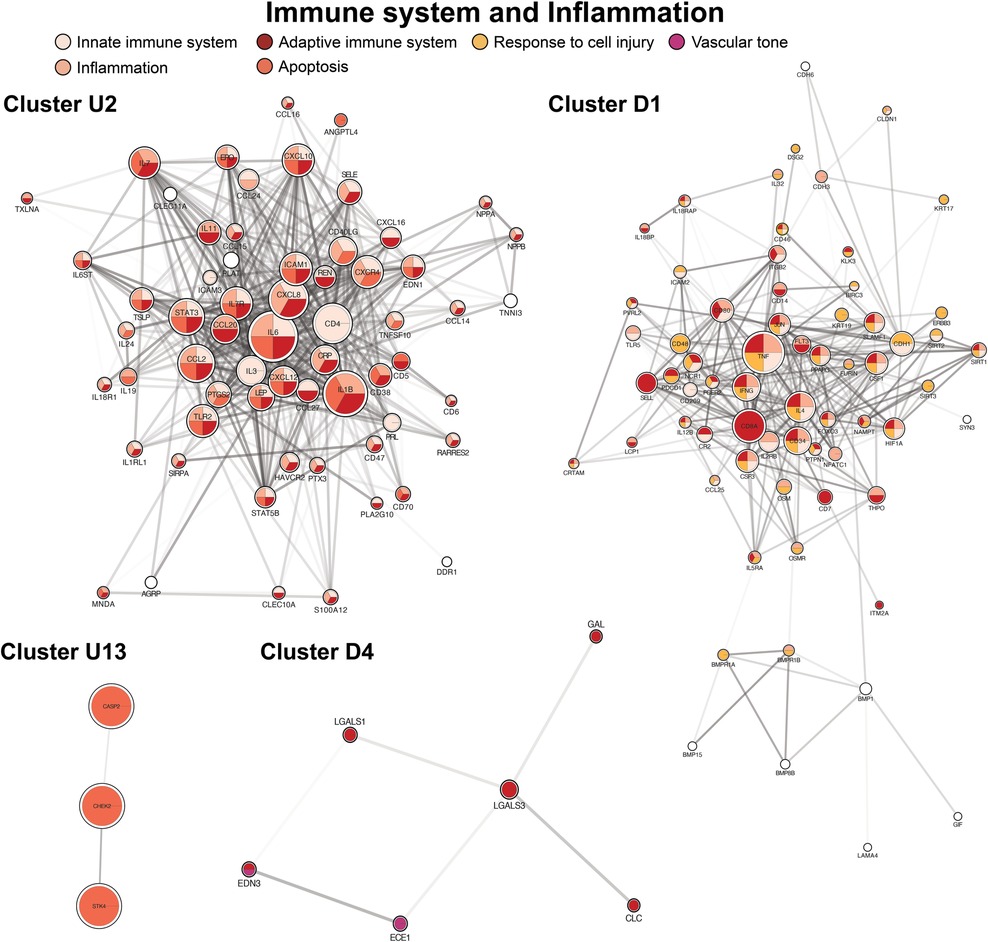
Figure 4. Clusters of immune system regulation, inflammation, response to cell injury (antioxidants), and apoptosis. Cluster formatting is described in Figure 3.
3.6. Altered metabolism
Key metabolic processes appeared in both the up-regulated and down-regulated protein networks. The primary groups governed lipid and carbohydrate biosynthesis, processing, and transport (Figure 5, clusters U4, U19, D3). The second most represented metabolism pathways centered on carbohydrate metabolism and digestion with both up and down-regulators (Figure 5, clusters U5, D7, D13). We also identified smaller, distinct down-regulated clusters for deranged amino acids (e.g., tryptophan) and nicotinate and nicotinamide metabolism (Supplementary Figure S2).
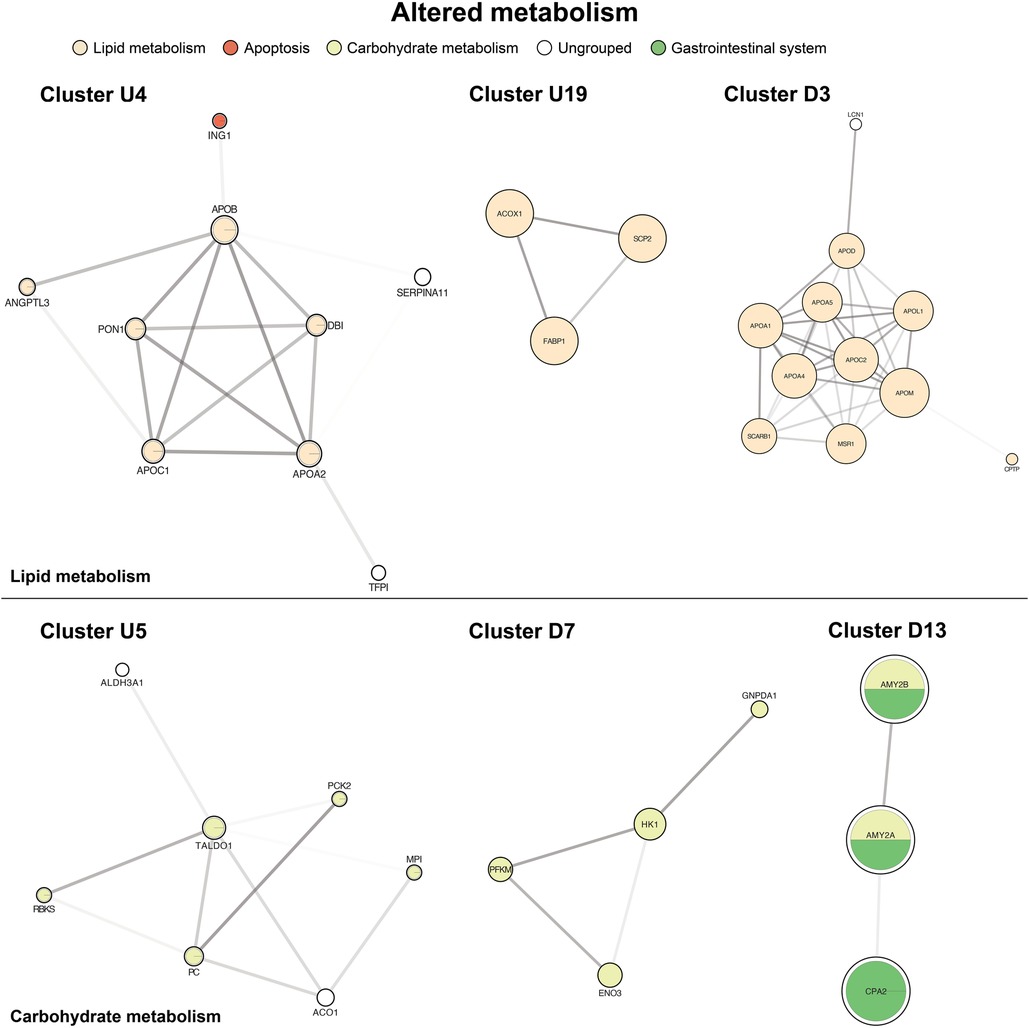
Figure 5. Of altered metabolism. The top panel is centered on lipid metabolism (U4, U19, D3). The bottom panel shows carbohydrate metabolism and digestion (U5, D7, D13). Cluster formatting is described in Figure 3.
3.7. Gastrointestinal system
Four up-regulated clusters involved the gastrointestinal system, mainly surrounding the intestinal mucosa, with functions in stabilizing the mucus gel overlying the gastrointestinal mucosa and promoting epithelial cell mobility to aid in healing (Figure 6, cluster U20). A second set of proteins bind bile acids and recycle them in ileal enterocytes (Figure 6, cluster U21). Finally, we identified two clusters involving pancreatic inflammation and function (Figure 6, cluster U8 and Figure 5, cluster D13).
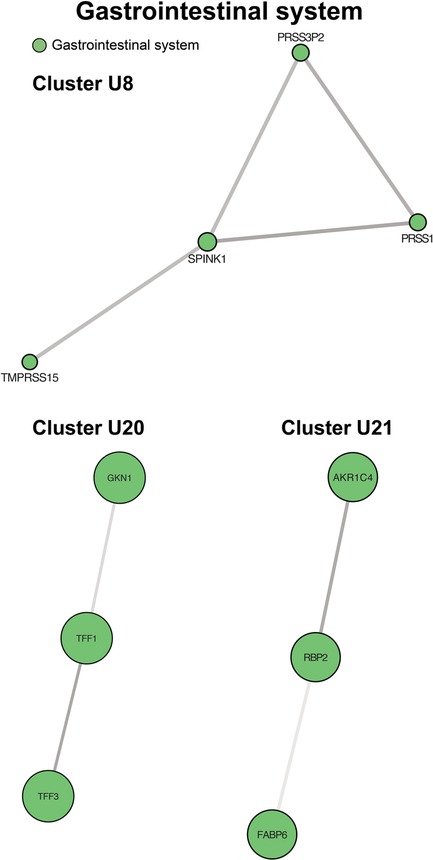
Figure 6. Clusters of gastrointestinal system processes. Cluster U8 contains genes involved in pancreatic inflammation. Cluster U20 centers on maintenance and protection of the gastrointestinal mucosa. Cluster U21 contains proteins controlling bile acid recycling. Cluster formatting is described in Figure 3.
3.8. Neural development
Six unique clusters focused on processes within the nervous system (Figure 7, clusters U6, U9, U15, U16, U24, D5), and our largest cluster (U1a) was also highly enriched in neurologic processes. The most frequent processes in GO enrichment were axonogenesis and axon guidance with expression across both the central and peripheral nervous systems. We also observed multiple clusters involving axon myelination through sphingolipid metabolism (Figure 7, cluster U16) in the axonal myelin sheath and plasma membranes of the CNS. One cluster was not enriched by GO terms but contained individual proteins associated with cerebral plaque formation and autoimmune neuropathy (Figure 7, cluster U15).
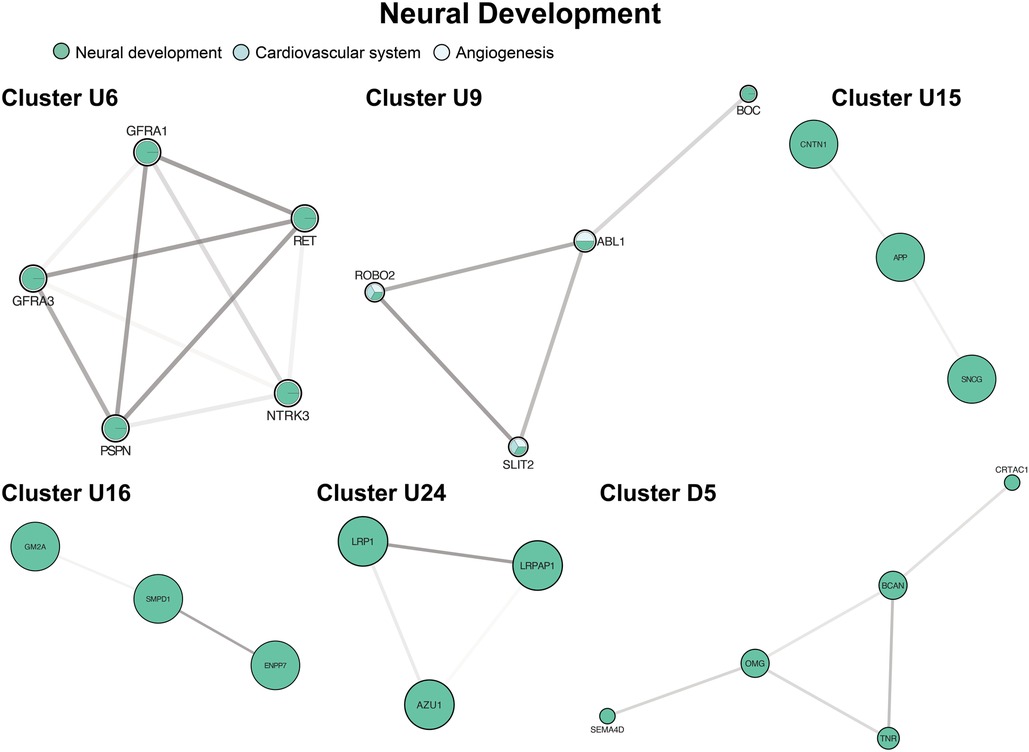
Figure 7. Clusters of neural development. Clusters U6, U9, U24, D5 involve drivers of axonogenesis and axon guidance. Cluster U15 contains individual proteins associated with cerebral plaque formation and autoimmune neuropathy Cluster U16 contains mediators of myelination. Cluster formatting is described in Figure 3.
3.9. Cardiovascular system
The third largest up-regulated cluster (Figure 8, cluster U3) and a large fraction of clusters U1a and U9 concerned cardiac morphogenesis from vascular to valvular structures and cardiomyocyte proliferation and differentiation. Cluster U3 was also enriched in cardiac morphogenesis at the embryonic level through endocardial cushion development, forming the trabecular muscles, septum, and pericardium. Clusters U1a and U9 had additional proteins active during the embryonic period, contributing to forming the aortic, mitral, and pulmonic valves. Cluster U3 was also enriched in cortisol and aldosterone biosynthesis. Additional clusters mediated vascular tone through direct action on the vasculature and heart and through ion transport (Figure 8, cluster U7 and Figure 4, cluster D4).
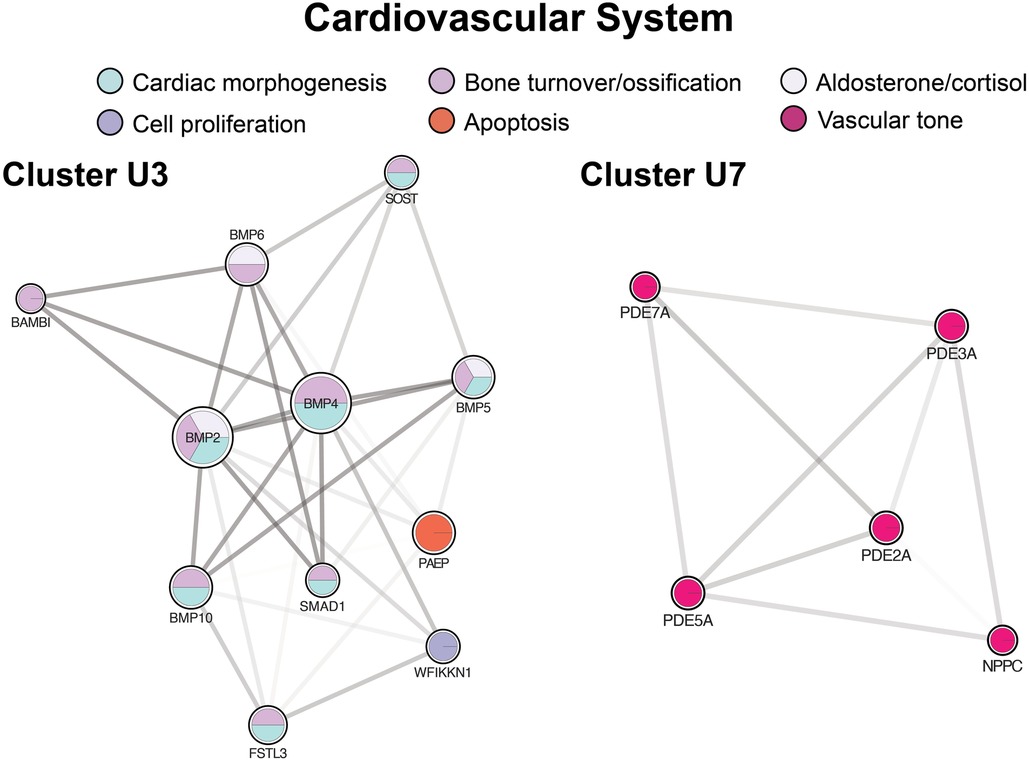
Figure 8. Clusters regulating the cardiovascular system. Cluster U3 centers of cardiac morphogenesis. Cluster U7 includes mediators of vascular tone. Cluster formatting is described in Figure 3.
4. Discussion
Single ventricle heart disease patients experience serious morbidity with clinical sequelae across organ systems. However, little is understood about the type and extent of molecular dysregulation SVHD patients experience. In this study, we analyzed serum to report a clear difference in the circulating proteomic fingerprint of infants with SVHD in the interstage period compared to similar-aged healthy controls. Furthermore, the proteome dysregulation they experience extends beyond cardiovascular regulation to include alterations in six primary areas: angiogenesis and cell proliferation/turnover, immune system regulation and inflammation, altered metabolism, gastrointestinal systems, neural development, and cardiac development.
4.1. Angiogenesis and cell proliferation/turnover
The largest and most highly interconnected cluster within our altered SVHD proteome centered on angiogenesis with functional enrichment of blood vessel formation across organs, including coronary vein and coronary artery morphogenesis, and glomerular capillary formation. One of the most potent drivers of angiogenesis, VEGFA, was at the center of this cluster. High levels of angiogenesis promoters in SVHD patients have been documented by others, including Dodge-Khatami et al., who reported elevated levels of VEGFA across the stages of SVHD palliation, peaking after Stage 2, followed by a slow decline until a nadir after completion of Stage 3 (24). In counterpoint, Bartoli et al. conducted a study similar to ours from samples drawn at the pre-Fontan catheterization and found levels of VEGFA to be downregulated compared to age matched-healthy controls despite up-regulation of numerous other pro-angiogenic factors (25). This discrepancy raises the question of whether the role of different angiogenic drivers may dominate at different stages of palliation. Further, we note that we cannot determine the origin of VEGFA from our circulating biomarker study, and consider it would be either systemic or pulmonary. In the systemic circulation, interstage physiology is marked by relative local tissue hypoxia secondary to arterial hypoxemia coupled with potentially compromised systemic cardiac output as the single ventricle pumps two effective cardiac outputs. The origin of VEGFA in this case could be systemic and hypoxia-driven, as local tissue hypoxia drives compensatory angiogenesis to overcome inadequate blood and oxygen supply (26). Interestingly, one of the most potent hypoxia-driven activators of VEGFA, HIF-1α, was not significantly different between our cases and controls, however, several other detectors of hypoxia, ENG and TGFB3, were. VEGFA may also have hypoxia-independent activation (27). The pulmonary endothelium experiences a marked shift in pressure and flow as the pulmonary vascular physiology transitions from a pulsatile flow (interstage) to a low-pressure, low-velocity continuous venous flow (Glenn). This discrepancy in pulmonary perfusion may explain both the increased VEGFA in our pre-Stage 2 cohort and the decreased VEGFA in the post-Stage 2 patients reported by the Bartoli group. Literature outside of CHD has shown changes in flow and pressure to directly alter protein synthesis at the pulmonary vascular endothelium, though not of angiogenic factors specifically (28). Notably, angiogenesis is not necessarily protective, as it also drives the growth of abnormal vasculature through the creation of systemic venous collaterals and multiple aortopulmonary collateral arteries (29).
We also demonstrated multiple fibroblast growth factors and metalloproteinases that function in tandem with VEGFA to achieve angiogenesis and other vascular changes. Proteins identified have roles in controlling the assembly and disassembly of the extracellular matrix (ECM) and collagen scaffolding, which support both the growth of new vessels and general cellular growth and repair. Notable proteins include FGF9 and FGF20, with roles in ECM changes in both the nervous and cardiovascular systems. Fibroblast growth factors have also been implicated as both a cause of pulmonary arterial hypertension (30, 31) as well as some evidence of, at times, protective effects (32). Our findings here are in alignment with our prior work and that of other groups in that SVHD patients have elevated circulating fibroblast growth factors, which may partly explain their proclivity to develop pulmonary hypertension (12, 33).
4.2. Immune system regulation and inflammation
Prior work by our group using a targeted cardiovascular protein panel demonstrated down-regulation of circulating pro-inflammatory cytokines within this cohort. Specific examples included interleukin (IL) 18, IL1 receptor ligand 2, tumor necrosis receptor superfamily member 13B, CXC motif chemokine 1, and leukocyte immunoglobulin-like receptor subfamily B1 (12). In our current study, we re-demonstrated lower levels of these specific proteins (with the exception of IL18 and leukocyte immunoglobulin-like receptor subfamily B1, which were not different between groups). There were a few other notable discrepancies between studies including discordant up vs. down regulation of ICAM3 and CD40LG (both up-regulated here). We suspect these differences to be secondary to differences and the relative quantification techniques across proteomics platforms. However, globally our expanded analysis includes additional members of the above immune pathways, demonstrating an overall trend toward up-regulation of pro-inflammatory cytokines. Included among the up-regulated proteins are proteins driving cytokine production, signaling, and response to cytokine activation. We also demonstrated an up-regulation of pro-apoptotic factors and a few instances of down-regulation of protective antioxidant factors. Inflammatory activation is well-described in post-surgical and post-bypass patients (34, 35), but our patients were, on average, 3–6 months out from initial palliative interventions. Therefore, the up-regulation of pro-inflammatory cytokines points to a broad chronic inflammatory state. The etiology of this is likely multifactorial with chronic poor oxygen delivery as a major mediator. Hypoxemia through chronic intermittent hypoxia (e.g., obstructive sleep apnea) has been shown to alter inflammatory mediators, including tumor necrosis factor (TNF)-α, interleukin (IL)-6 (36). Similarly, non-SVHD forms of chronic continuous hypoxemia (e.g., chronic obstructive pulmonary disease) have been shown to be altered at the microRNA level, though to a lesser degree than intermittent hypoxia (37). Our findings align with the results of studies of clinically available inflammatory markers that have identified elevated levels of inflammation in adults with CHD (38, 39). In adults, those with higher levels had worse functional status and exercise capacity and greater risk for death or non-elective cardiovascular hospitalization (40). The role and harm of inflammatory mediators in the pediatric CHD population will be an important area for ongoing investigation.
Immune regulators were expressed across both the up and down-regulated proteomes and across both the innate and adaptive immune systems. Changes in the adaptive immune system were more prominent, suggesting more chronic immune activation. We identified particular enrichment of mediators of T-cell activation, a phenomenon which has been well-described in other immune-activating chronic illnesses, including HIV and hepatitis C (41). Chronic immune activation is also associated with T-lymphocyte depletion (42, 43), consistent with described lymphopenia in many SVHD infants before both the stage 2 and stage 3 operations (44). A complicating factor in assessing the adaptive immune system alteration is the frequency of thymectomy in SVHD patients, as median sternotomy often requires the removal of the thymic tissue. Stages 1 and 2 typically occur during the first year of life, a critical period for thymic lymphocyte production (45, 46). Thymectomy has been associated with premature immunosenescence of peripheral T-cell populations both in the short- and long-term, effects that appear permanent in some studies (46, 47). There are also likely connections between the development of the cardiovascular and immune systems, which may cloud the assessment of immune function. Many of the most common genetic syndromes linked to (non-SVHD) CHD (e.g., Trisomy 21, DiGeorge syndrome, Noonan syndrome) have associated immune deficiencies or senescence, as well as increased risk of the malignancies of the hematopoietic system, affecting immunoregulatory cell populations (45, 48).
4.3. Altered metabolism
Altered cholesterol metabolism has previously been documented in hypoxia, secondary to oncologic processes with notable hepatic tissue hypoxia driving excessive hepatic steatosis (49) as well as lipogenesis, extracellular uptake of fatty acids, and lipogenesis (50). However, it should be noted that these are often more extreme forms of hypoxia, and the role of lower-grade chronic hypoxia on metabolism in CHD is not understood. Evidence of altered cholesterol metabolism has been identified directly in SVHD patients, though in the relatively normoxic post-Fontan period. In fact, these patients often experience metabolic dysregulation quite remotely, in many cases years out from the Fontan operation (51, 52). Specifically, post-Fontan patients have hypocholesterolemia, possibly due to hepatic insufficiency. Decreased cholesterol synthesis and resultant hypocholesterolemia is hypothesized as an early marker of liver disease, as it is associated with liver fibrosis even without correlative transaminases or gamma-glutamyl transpeptidase (GGT) elevation. To our knowledge, there has not been a study of cholesterol levels or synthesis earlier in the course of SVHD, nor the interstage period specifically. We found mixed results in proteins governing cholesterol synthesis. We demonstrated a down-regulation of numerous apolipoproteins, but circulating lipoproteins, including VLDL, LDL, and HDL, were well-represented in both up and down-regulated networks. We noted up-regulation of cholesterol and fat absorption markers with proteins driving bile acid binding and enterohepatic bile acid recycling (FABP6, AKR1C4), suggesting an overall increase in cholesterol and fat absorption capacity. This aligns with the findings of Whiteside et al., who showed an up-regulation of cholesterol absorption efficiency and low synthesis in Fontan patients (51). It has been posited that cholesterol absorption is up-regulated in an attempt to compensate for poor synthetic capacity in SVHD livers.
Additional metabolism pathways center on carbohydrate metabolism. First, several proteins central to carbohydrate digestion were down-regulated with low levels of amylases A1 and A2, though it is unclear how this may affect carbohydrate metabolism overall. The proteins trended slightly towards increased gluconeogenesis and decreased glycolysis, with a number of gluconeogenic proteins up-regulated (e.g., fructose-1,6-bisphosphatase and glucose-6-phosphate isomerase) and down-regulated proteins driving glycolysis (e.g., hexokinase 1 and 2, glucose-6-phosphate dehydrogenase). Prior metabolic evaluation of Fontan patients has shown no significant differences in glycolysis metabolites apart from lactate, which was elevated in Fontan patients when compared to healthy controls (53). Conclusions from our study are similarly limited as a number of proteins did not meet statistical significance, and a more complete mapping of gluconeogenic and glycolytic pathways is needed.
Additional protein derangements involving amino acid (e.g., tryptophan), nicotinate, and nicotinamide metabolism were noted and merit investigation, though outside the scope of this manuscript. Amino acid metabolism may be of particular interest, as we have previously demonstrated low circulating amino acids during the interstage period (54, 55).
4.4. Gastrointestinal systems
Regulators of the gastrointestinal system were well-represented, with particular emphasis on the intestines and pancreas. Perfusion insults to the intestines in the acute post-operative period result in high morbidity and, when severe, contribute to the development of necrotizing enterocolitis (56). Patients with HLHS, the most common diagnosis in our cohort, are at the highest risk of developing NEC among all congenital heart disease patients (10). In the sub-acute post-operative period, patients frequently experience feeding intolerance and growth failure (57) and in the long term, patients are at risk of developing protein-losing enteropathy (PLE). PLE is thought to occur secondary to alterations in mesenteric blood flow, systemic inflammation, neurohormonal activation, and protein glycosylation (58), many of which were demonstrated in our cohort. We identified up-regulation of a number of protective proteins. In particular, trefoil factors 1 and 3 (TFF1, TFF3) stabilize the mucus gel overlying the gastrointestinal mucosa, providing a physical barrier against noxious agents (59). TFF3 further supports the mucosal barrier by promoting the mobility of epithelial cells in the healing process (60). It is therefore conceivable that the up-regulation of these proteins represents an adaptive response to low levels of mesenteric ischemia and inflammation recognized in our other cluster sets.
There was also an up-regulation of various pancreatic enzymes, including endopeptidases, (e.g., trypsins 1 and 2), and carboxypeptidases (e.g., CPA2). In sum, this may imply an upregulation of protein digestion or markers of pancreatic hypoperfusion, inflammation, and/or injury. CPA2 has also been considered as a possible biomarker for pancreatic injury in rats, with preliminary studies showing a higher sensitivity of detection and of sustained increases in plasma observed over a longer time period when compared to clinically available biomarkers, lipase and amylase (61). It should be noted, however, that the difference in age along with likely differences in oral-motor skills between healthy controls and our SVHD cases may result in a difference in overall diet (e.g., primarily breast milk/formula diet vs. a diet including solid foods). As a difference in dietary intake may affect the proteome (62, 63), and particularly digestive enzymes, we are cautious to draw conclusions in this area without further studies
4.5. Neural development
Neurologic and neurodevelopmental impairment is well-documented in patients with SVHD, with as many as one-third of patients demonstrating moderate to severe impairment by IQ testing (64). Neurodevelopmental deficits occur across developmental domains, including fine and gross motor skills, language, and memory (8). Patients may also experience higher level deficits in executive function, social cognition, and impulse and emotional control (8, 65, 66). The causes are thought to be multifactorial, including shared genetic origins of fetal heart and brain development, hypoxemia beginning in the fetal period, chronically low cardiac output, ischemic injuries from cardiopulmonary bypass, injurious events peri-operatively (e.g., intracranial hemorrhage, seizure), and prolonged hospital stays resulting in increased noxious exposures and decreased developmental stimulation (67–69). Our network was highly enriched by up-regulated proteins clustering around processes within the nervous system. The highest degrees of enrichment were in axonogenesis, axon morphogenesis, and axon guidance enrichment across both the central and peripheral nervous systems, with notable processes including retinal ganglion axon guidance, olfactory bulb interneuron development, and motor neuron axon migration. Proteins within the contactin gene family (CNTN1, CNTN3, CNTN4, CNTN5) were nearly universally up-regulated. Notably, CNTN3 and CNTN5 were among the top 35 VIP proteins, along with NOTCH3 of the NOTCH signaling pathway, with which contactin acts as a ligand (70). The contactin family is an influential immunoglobulin superfamily with roles in axon outgrowth, guidance, and neuronal differentiation (71). The group has also been implicated in hippocampal synaptic plasticity and memory in adult mice (72).
Additionally, the network enrichment in CNS axon myelination through sphingolipid metabolism is of particular interest as myelination has been shown to correlate with intelligence with significant crossover in attributed genes (73). Further, delayed myelination is associated with developmental delay and behavioral impairments and in fact, long-term white matter alterations are a hallmark of brain imaging studies in SVHD patients (74). Although the cause is unknown, risk factors include perinatal inflammatory insults and fluctuating cerebral oxygenation on a background of chronic hypoxemia (75). The up-regulation of these processes in SVHD patients may therefore reflect a response to delayed myelination secondary to hypoxemic and inflammatory insults. However, our SVHD cohort skewed slightly younger than the healthy controls and thus perhaps experienced increased myelination owing to their younger age. Nevertheless, the myelination process should extend to at least two to three years of age, so both cohorts were well within the timeframe of active myelinating processes (76).
We were also interested in identifying biomarkers of neurologic injury. Prior work identified high levels of S100β, a glial-derived protein marker of cerebral ischemia, in HLHS patients (77, 78). Our protein panel did not test for S100β specifically but did target various other proteins in the S100 protein family and found similar elevation. The challenge with clinical translation of S100β has been the existence of extracerebral sources, confounding its utility as a marker of specific neurologic injury. It is unclear if similar proteins in the S100 family suffer from the same complication, but this would be an important area of investigation. Interestingly, commonly studied markers of neurologic injury were not similarly up-regulated. Specifically, GFAP was not significantly different between groups, and NEFL was down-regulated. These biomarkers peak with acute injurious events followed by a decline (on the order of months) and thus may not have been at chronically elevated levels even in patients with prior neurologic insults (79).
4.6. Cardiovascular system
We found mediation of the cardiovascular system at multiple levels, including cardiac morphogenesis, mediators of vascular tone and contractility through ion transport, and direct vasoactive proteins. Four key signaling pathways were well represented in the up-regulated protein network: NOTCH, TGFβ, insulin-like growth factor (IGF), and bone morphogenetic protein (BMP). NOTCH and BMP work synchronously in developing valvular structures such as the aortic and mitral valves (80–82). Together with TGFβ, they also drive cardiac morphogenesis at the embryonic level through endocardial cushion development, forming the trabecular muscles (BMP 5,10) and pericardium (BMP 2,5), among other structures (82, 83). In particular, NOTCH signaling is crucial for regulating a subset of endocardial cells in the primary heart tube (81). IGF, along with BMP, is responsible for cardiac growth through the proliferation of cardiomyocytes. Among this pathway, IGF2 has been identified as the most important mitogen for cardiomyocytes (84). Although our panel did not test for IGF2 itself, its receptor, as well as key binding proteins, were significantly higher in our SVHD cohort, suggesting activation of the pathway. Although the exact roles of proteins within these signaling pathways are still being elucidated, their general importance to the embryonic and fetal morphogenesis of the heart is understood. What is less clear is how these signaling pathways may be altered postnatally in patients with CHD and, further, how they may change at the various stages of intervention and beyond. Traditionally, cardiomyocytes were thought to be strictly postmitotic after birth, with further myocardial growth occurring by cell hypertrophy alone (85). However, growing work suggests possible cardiomyocyte regenerative ability at low levels. A number of genes and signaling pathways have been posited as potential drivers of regeneration. BMP, TGFβ, and NOTCH signaling have been identified as regenerative signaling pathways at sites of cardiomyocyte injury in non-mammalian animal models (86, 87). Mammalian studies have identified TNNI3K, a kinase interacting with TNNI3 (up-regulated in our cohort), as capable of cardiac stem cell dedifferentiation, conferring a regenerative capacity to the heart (88). The changes in these pathways and their possible roles in cardiomyocyte regeneration have not been well-studied in humans and, to our knowledge, not studied at all in CHD patients, specifically. SVHD and CHD patients may have abnormal circulating levels of these proteins at baseline, however, the broad up-regulation of several key signaling pathways in our SVHD patients raises the question of a possible role in cardiac growth and recovery in response to changing physiology.
BMP signaling is also central to GO enrichment of vascular tone mediators. Specifically, there was overall down-regulation (up-regulation of negative regulators) of aldosterone and cortisol, perhaps attributable to volume overload frequently experienced by interstage patients. Vasoactive proteins were enriched through negative regulators of transmembrane ion and cation (calcium) transport, affecting smooth muscle contraction. Interestingly, the same protein network demonstrated expression in striated muscle and sarcomeres and thus may have a role in cardiac contractility. Vascular permeability and resistance proteins were highly enriched through up-regulation of phosphodiesterase (PDE) signaling driving cGMP and cAMP catabolism. As cGMP and cAMP lead to vasodilation, up-regulation of PDEs causing increased catabolism of both has the end physiologic effect of vasoconstriction (89). This is deleterious in the pulmonary vasculature, where it is a primary cause of pulmonary hypertension. We demonstrated up-regulation of several phosphodiesterases, including PDE5, the therapeutic target of medical therapy for pulmonary hypertension, which works to inhibit PDE5, thereby increasing cGMP and vasodilation. Prior work has shown elevated PDE5 in SVHD patients with heart failure (90), but it is more commonly experienced following Stage 2 palliation where it is especially harmful as it hinders passive pulmonary blood flow. Our findings indicate that drivers of pulmonary hypertension may begin earlier in the course of palliation.
4.7. Additional enriched pathways
We identified several other areas of enrichment within our proteome that are outside the scope of this manuscript but may warrant future investigation. Most notable was a particularly high degree of bone ossification and bone turnover/resorption enrichment and, when considered alongside upregulated calcitriol metabolism, renal phosphate excretion, calcium transport, and calcium absorption, suggest an osteogenic focus.
4.8. Limitations
A limitation of this work is the difference in age and weight of our cases and controls. Although our inclusion criteria for age and weight were identical in both groups, patients in the control group tended to be undergoing elective and less time-sensitive surgical procedures. In standard clinical practice, this often leads to delaying procedures, which resulted in a skew of our control group to be slightly older and, therefore, heavier. To clarify the magnitude of this effect, we analyzed proteins against age and weight in our control group, who ranged from 5.12 to 11.66 months and 6.58–10.38 kg. Across these time and weight ranges, we found no effect of age or weight, which is reassuring against a large signal from either driving our observed differences in the proteomes. However, this does not preclude small age/weight effects, as it did not include the full age and weight range of all included subjects. The lack of studies on the effects of age on protein levels remains a gap in our field that merits dedicated investigation. We were unable to control for age or weight in our full analysis, as the case and control groups would split on that metric. Second, although our custom protein panel is a relatively large-scale assay, it still represents a subset of the total human proteome and does not include analysis of downstream proteome mediators (e.g., nucleic acids, metabolites, glycosylation, etc.). While the human proteome's exact size is unknown, estimates in the literature suggest there are approximately 20,000 total proteins (91–93). We therefore estimate that our targeted protein assay tests 7%–10% of all human proteins. It has been hypothesized that the detectable circulating proteome is smaller than the total proteome, but this value has not been quantified. Therefore, our study likely represents a higher fraction of proteins available in the blood. Additionally, the custom nature of our protein panel could introduce bias. To obviate this, we selected proteins of potential significance in cardiovascular, neurologic, and kidney function. In an exploratory effort, we also chose a variety of proteins of unknown significance to our patient population. An additional limitation is that our study does not identify a singular role of any one protein because many proteins tested are involved in multiple signaling pathways and function in more than one organ system. Further, individual proteins can be assigned to more than one GO term, introducing potential overlap with other functional groups. Finally, our data include a large number of proteins with p values between 0.05 and 1.1873e -13 between the various groups. Traditional Bonferroni correction is likely overly conservative and thus would underestimate the number of proteins affected during the interstage period (type 2 error). We therefore chose the less-conservative Benjamini-Hochberg (FDR) correction, and thus studies using absolute quantification methodologies in larger populations will be important to validate these results. Future work will also be needed to evaluate altered proteins’ changes over time and with subsequent interventions, including Stage 3 palliation and beyond. As our study shows multi-organ effects, evaluating these biological systems at the tissue level via an animal model will be necessary to understand mechanisms of dysregulation. Additionally, it will be important to collect clinical outcomes data for correlation and to begin to understand causation.
5. Conclusions
We report that the proteome of interstage SVHD subjects is readily distinguishable from healthy controls. This builds upon prior work, which demonstrated differences in the cardiovascular proteome in this cohort. Here, we present an expanded proteomic profile broadly dysregulated across multiple biological systems, including the nervous system, heart, vasculature, and gastrointestinal systems, with a high degree of activation of inflammatory cascades, underscoring the stress imposed by interstage SVHD physiology. Furthermore, this work demonstrates the degree of chronic protein dysregulation experienced by SVHD patients, even at a time of relative health within the course of palliation. This is an important step in understanding the sequelae of SVHD at the biochemical level, which is necessary to identify possible targets for prognostication and therapeutic intervention. In addition, the breadth of the biochemical changes highlights the importance of a systems-based approach in defining complex interactions not well captured by single biomarker strategies.
Data availability statement
The original contributions presented in the study are included in the article/supplementary materials, further inquiries can be directed to the corresponding author.
Ethics statement
The studies involving humans were approved by Colorado Multiple Institutional Review Board. The studies were conducted in accordance with the local legislation and institutional requirements. Written informed consent for participation in this study was provided by the participants’ legal guardians/next of kin.
Author contributions
LT: Data curation, Formal Analysis, Visualization, Writing – original draft, Writing – review & editing. CM: Formal Analysis, Methodology, Software, Writing – original draft, Writing – review & editing. KW: Conceptualization, Writing – review & editing. LK: Data curation, Investigation, Project administration, Writing – review & editing. SN: Writing – review & editing, Formal Analysis, Software. EA: Writing – review & editing, Data curation, Investigation. MDM: Conceptualization, Writing – review & editing, Funding acquisition. MM: Writing – review & editing, Conceptualization, Methodology. MT: Investigation, Writing – review & editing. GM: Conceptualization, Investigation, Writing – review & editing. BF: Conceptualization, Formal Analysis, Funding acquisition, Investigation, Methodology, Supervision, Writing – original draft, Writing – review & editing. JD: Formal Analysis, Writing – review & editing, Conceptualization, Funding acquisition, Investigation, Methodology, Supervision, Writing – original draft.
Funding
The author(s) declare financial support was received for the research, authorship, and/or publication of this article.
American Heart Association 18IPA34170070 (JD). American Heart Association 20CDA35310498 (BF). NIH/NHLBI K23HL163490 (BF). NIH R38HL143511 (LT). Additional Ventures Tools and Tech Expansion Award (BF, JD and MDM). Jayden DeLuca Foundation (BF).
Acknowledgments
We thank Kim Pham, RN, and the rest of the staff at Children's Hospital Colorado Translational Research Center for their contributions in conducting this study.
Conflict of interest
The authors declare that the research was conducted in the absence of any commercial or financial relationships that could be construed as a potential conflict of interest.
Publisher's note
All claims expressed in this article are solely those of the authors and do not necessarily represent those of their affiliated organizations, or those of the publisher, the editors and the reviewers. Any product that may be evaluated in this article, or claim that may be made by its manufacturer, is not guaranteed or endorsed by the publisher.
Supplementary material
The Supplementary Material for this article can be found online at: https://www.frontiersin.org/articles/10.3389/fped.2023.1308700/full#supplementary-material
References
1. Gilboa SM, Salemi JL, Nembhard WN, Fixler DE, Correa A. Mortality resulting from congenital heart disease among children and adults in the United States, 1999 to 2006. Circulation. (2010) 122(22):2254–63. doi: 10.1161/CIRCULATIONAHA.110.947002
2. Ohye RG, Schranz D, D’Udekem Y. Current therapy for hypoplastic left heart syndrome and related single ventricle lesions. Circulation. (2016) 134(17):1265–79. doi: 10.1161/CIRCULATIONAHA.116.022816
3. Ghanayem NS, Allen KR, Tabbutt S, Atz AM, Clabby ML, Cooper DS, et al. Interstage mortality after the Norwood procedure: results of the multicenter single ventricle reconstruction trial. J Thorac Cardiovasc Surg. (2012) 144(4):896–906. doi: 10.1016/j.jtcvs.2012.05.020
4. Anderson JB, Beekman RH 3rd, Kugler JD, Rosenthal GL, Jenkins KJ, Klitzner TS, et al. Improvement in interstage survival in a national pediatric cardiology learning network. Circ Cardiovasc Qual Outcomes. (2015) 8(4):428–36. doi: 10.1161/CIRCOUTCOMES.115.001956
5. Kulik TJ. Pulmonary blood flow and pulmonary hypertension: is the pulmonary circulation flowophobic or flowophilic? Pulm Circ. (2012) 2(3):327–39. doi: 10.4103/2045-8932.101644
6. Khan M, Bordes SJ, Murray IV, Sharma S. Physiology, pulmonary vasoconstriction. Statpearls. Treasure Island, FL (2023).
7. Kogon BE, Plattner C, Leong T, Simsic J, Kirshbom PM, Kanter KR. The bidirectional glenn operation: a risk factor analysis for morbidity and mortality. J Thorac Cardiovasc Surg. (2008) 136(5):1237–42. doi: 10.1016/j.jtcvs.2008.05.017
8. du Plessis K, d'Udekem Y. The neurodevelopmental outcomes of patients with single ventricles across the lifespan. Ann Thorac Surg. (2019) 108(5):1565–72. doi: 10.1016/j.athoracsur.2019.05.043
9. Sun L, Macgowan CK, Sled JG, Yoo SJ, Manlhiot C, Porayette P, et al. Reduced fetal cerebral oxygen consumption is associated with smaller brain size in fetuses with congenital heart disease. Circulation. (2015) 131(15):1313–23. doi: 10.1161/CIRCULATIONAHA.114.013051
10. Spinner JA, Morris SA, Nandi D, Costarino AT, Marino BS, Rossano JW, et al. Necrotizing enterocolitis and associated mortality in neonates with congenital heart disease: a multi-institutional study. Pediatr Crit Care Med. (2020) 21(3):228–34. doi: 10.1097/PCC.0000000000002133
11. Morgan C, Al-Aklabi M, Garcia Guerra G. Chronic kidney disease in congenital heart disease patients: a narrative review of evidence. Can J Kidney Health Dis. (2015) 2:27. doi: 10.1186/s40697-015-0063-8
12. Frank BS, Khailova L, Silveira L, Mitchell MB, Morgan GJ, Hsieh EWY, et al. Proteomic profiling identifies key differences between inter-stage infants with single ventricle heart disease and healthy controls. Transl Res. (2021) 229:24–37. doi: 10.1016/j.trsl.2020.10.001
13. Gold L, Ayers D, Bertino J, Bock C, Bock A, Brody EN, et al. Aptamer-based multiplexed proteomic technology for biomarker discovery. PLoS One. (2010) 5(12):e15004. doi: 10.1371/journal.pone.0015004
15. Pang Z, Chong J, Zhou G, de Lima Morais DA, Chang L, Barrette M, et al. Metaboanalyst 5.0: narrowing the gap between raw spectra and functional insights. Nucleic Acids Res. (2021) 49(W1):W388–W96. doi: 10.1093/nar/gkab382
16. Benjamini YH Y. Controlling the false discovery rate: a practical and powerful approach to multiple testing. J R Stat Soc Series B Stat Methodol. (1995) 57(1):289–300. doi: 10.1111/j.2517-6161.1995.tb02031.x
17. Liu R, Mancuso CA, Yannakopoulos A, Johnson KA, Krishnan A. Supervised learning is an accurate method for network-based gene classification. Bioinformatics. (2020) 36(11):3457–65. doi: 10.1093/bioinformatics/btaa150
18. Mancuso CA, Liu R, Krishnan A. Pygeneplexus: a python package for gene discovery using network-based machine learning. Bioinformatics. (2023) 39(2):1–3. doi: 10.1093/bioinformatics/btad064
19. Szklarczyk D, Franceschini A, Wyder S, Forslund K, Heller D, Huerta-Cepas J, et al. STRING V10: protein-protein interaction networks, integrated over the tree of life. Nucleic Acids Res. (2015) 43(Database issue):D447–52. doi: 10.1093/nar/gku1003
20. Shih YK, Parthasarathy S. Identifying functional modules in interaction networks through overlapping markov clustering. Bioinformatics. (2012) 28(18):i473–i9. doi: 10.1093/bioinformatics/bts370
21. Ashburner M, Ball CA, Blake JA, Botstein D, Butler H, Cherry JM, et al. Gene ontology: tool for the unification of biology. The gene ontology consortium. Nat Genet. (2000) 25(1):25–9. doi: 10.1038/75556
22. Ontology C G, Aleksander SA, Balhoff J, Carbon S, Cherry JM, Drabkin HJ, et al. The gene ontology knowledgebase in 2023. Genetics. (2023) 224(1):1–14.
23. Shannon P, Markiel A, Ozier O, Baliga NS, Wang JT, Ramage D, et al. Cytoscape: a software environment for integrated models of biomolecular interaction networks. Genome Res. (2003) 13(11):2498–504. doi: 10.1101/gr.1239303
24. Dodge-Khatami A, Sreeram N, de Mol BA, Bennink GB. Systemic plasma vascular endothelial growth factor levels as a marker for increased angiogenesis during the single ventricle surgical pathway. Interact Cardiovasc Thorac Surg. (2003) 2(4):458–61. doi: 10.1016/S1569-9293(03)00113-0
25. Bartoli CR, Hennessy-Strahs S, Dowling RD, Gaynor JW, Glatz AC. Abnormalities in the von Willebrand-angiopoietin axis contribute to dysregulated angiogenesis and angiodysplasia in children with a glenn circulation. JACC Basic Transl Sci. (2021) 6(3):222–35. doi: 10.1016/j.jacbts.2020.12.014
26. Gerritsen ME. Angiogenesis. In: Tuma RF, Duran WN, Ley K, editors. Microcirculation. San Francisco, CA: Academic Press (2008). p. 351–83.
27. Maira MAP MS, Fabbro D, Garcia-Echeverria C. Cancer biology. In: Taylor JB, Triggle DJ, editors. Comprehensive medicinal chemistry II. Basel, Switzerland: Elsevier (2007). p. 1–31.
28. Dschietzig T, Richter C, Bartsch C, Bohme C, Heinze D, Ott F, et al. Flow-induced pressure differentially regulates endothelin-1, urotensin II, adrenomedullin, and relaxin in pulmonary vascular endothelium. Biochem Biophys Res Commun. (2001) 289(1):245–51. doi: 10.1006/bbrc.2001.5946
29. Spearman AD, Ginde S. Pulmonary vascular sequelae of palliated single ventricle circulation: arteriovenous malformations and aortopulmonary collaterals. J Cardiovasc Dev Dis. (2022) 9(9):309. doi: 10.3390/jcdd9090309
30. Benisty JI, McLaughlin VV, Landzberg MJ, Rich JD, Newburger JW, Rich S, et al. Elevated basic fibroblast growth factor levels in patients with pulmonary arterial hypertension. Chest. (2004) 126(4):1255–61. doi: 10.1378/chest.126.4.1255
31. Yeo Y, Yi ES, Kim JM, Jo EK, Seo S, Kim RI, et al. FGF12 (fibroblast growth factor 12) inhibits vascular smooth muscle cell remodeling in pulmonary arterial hypertension. Hypertension. (2020) 76(6):1778–86. doi: 10.1161/HYPERTENSIONAHA.120.15068
32. Woo KV, Shen IY, Weinheimer CJ, Kovacs A, Nigro J, Lin CY, et al. Endothelial FGF signaling is protective in hypoxia-induced pulmonary hypertension. J Clin Invest. (2021) 131(17):e141467. doi: 10.1172/JCI141467
33. Sandeep N, Uchida Y, Ratnayaka K, McCarter R, Hanumanthaiah S, Bangoura A, et al. Characterizing the angiogenic activity of patients with single ventricle physiology and aortopulmonary collateral vessels. J Thorac Cardiovasc Surg. (2016) 151(4):1126–35.e2. doi: 10.1016/j.jtcvs.2015.10.001
34. Kozik DJ, Tweddell JS. Characterizing the inflammatory response to cardiopulmonary bypass in children. Ann Thorac Surg. (2006) 81(6):S2347–54. doi: 10.1016/j.athoracsur.2006.02.073
35. Kolcz J, Karnas E, Madeja Z, Zuba-Surma EK. The cardioprotective and anti-inflammatory effect of inhaled nitric oxide during fontan surgery in patients with single ventricle congenital heart defects: a prospective randomized study. J Intensive Care. (2022) 10(1):48. doi: 10.1186/s40560-022-00639-y
36. Levy P, Pepin JL, Arnaud C, Tamisier R, Borel JC, Dematteis M, et al. Intermittent hypoxia and sleep-disordered breathing: current concepts and perspectives. Eur Respir J. (2008) 32(4):1082–95. doi: 10.1183/09031936.00013308
37. Lacedonia D, Scioscia G, Pia Palladino G, Gallo C, Carpagnano GE, Sabato R, et al. MicroRNA expression profile during different conditions of hypoxia. Oncotarget. (2018) 9(80):35114–22. doi: 10.18632/oncotarget.26210
38. Riehle C, Bauersachs J. Key inflammatory mechanisms underlying heart failure. Herz. (2019) 44(2):96–106. doi: 10.1007/s00059-019-4785-8
39. Sorriento D, Iaccarino G. Inflammation and cardiovascular diseases: the most recent findings. Int J Mol Sci. (2019) 20(16):1–4. doi: 10.3390/ijms20163879
40. Opotowsky AR, Valente AM, Alshawabkeh L, Cheng S, Bradley A, Rimm EB, et al. Prospective cohort study of C-reactive protein as a predictor of clinical events in adults with congenital heart disease: results of the Boston adult congenital heart disease biobank. Eur Heart J. (2018) 39(34):3253–61. doi: 10.1093/eurheartj/ehy362
41. Gonzalez VD, Falconer K, Blom KG, Reichard O, Morn B, Laursen AL, et al. High levels of chronic immune activation in the T-cell compartments of patients coinfected with hepatitis C virus and human immunodeficiency virus type 1 and on highly active antiretroviral therapy are reverted by alpha interferon and ribavirin treatment. J Virol. (2009) 83(21):11407–11. doi: 10.1128/JVI.01211-09
42. Abdel-Hakeem MS, Manne S, Beltra JC, Stelekati E, Chen Z, Nzingha K, et al. Author correction: epigenetic scarring of exhausted T cells hinders memory differentiation upon eliminating chronic antigenic stimulation. Nat Immunol. (2021) 22(11):1465. doi: 10.1038/s41590-021-01057-2
43. Taeschler P, Adamo S, Deng Y, Cervia C, Zurbuchen Y, Chevrier S, et al. T-cell recovery and evidence of persistent immune activation 12 months after severe COVID-19. Allergy. (2022) 77(8):2468–81. doi: 10.1111/all.15372
44. Kovacikova L, Krasnanova V, Skrak P, Zahorec M, Kantorova A, Semberova J, et al. Immune abnormalities in patients with single ventricle circulation precede the fontan procedure. World J Pediatr Congenit Heart Surg. (2017) 8(6):672–82. doi: 10.1177/2150135117732529
45. Wienecke LM, Cohen S, Bauersachs J, Mebazaa A, Chousterman BG. Immunity and inflammation: the neglected key players in congenital heart disease? Heart Fail Rev. (2022) 27(5):1957–71. doi: 10.1007/s10741-021-10187-6
46. Roosen J, Oosterlinck W, Meyns B. Routine thymectomy in congenital cardiac surgery changes adaptive immunity without clinical relevance. Interact Cardiovasc Thorac Surg. (2015) 20(1):101–6. doi: 10.1093/icvts/ivu343
47. Afifi A, Raja SG, Pennington DJ, Tsang VT. For neonates undergoing cardiac surgery does thymectomy as opposed to thymic preservation have any adverse immunological consequences? Interact Cardiovasc Thorac Surg. (2010) 11(3):287–91. doi: 10.1510/icvts.2010.237172
48. Calcagni G, Unolt M, Digilio MC, Baban A, Versacci P, Tartaglia M, et al. Congenital heart disease and genetic syndromes: new insights into molecular mechanisms. Expert Rev Mol Diagn. (2017) 17(9):861–70. doi: 10.1080/14737159.2017.1360766
49. Cao R, Zhao X, Li S, Zhou H, Chen W, Ren L, et al. Hypoxia induces dysregulation of lipid metabolism in HepG2 cells via activation of HIF-2alpha. Cell Physiol Biochem. (2014) 34(5):1427–41. doi: 10.1159/000366348
50. Mylonis I, Simos G, Paraskeva E. Hypoxia-inducible factors and the regulation of lipid metabolism. Cells. (2019) 8(3):214–30. doi: 10.3390/cells8030214
51. Whiteside W, Tan M, Ostlund RE Jr., Yu S, Ma L, Rocchini A. Altered cholesterol metabolism and hypocholesterolemia in patients with single ventricle following fontan palliation. J Pediatr. (2016) 171:73–7. doi: 10.1016/j.jpeds.2015.12.038
52. Lubert AM, Alsaied T, Palermo JJ, Anwar N, Urbina EM, Brown NM, et al. Fontan-associated dyslipidemia. J Am Heart Assoc. (2021) 10(7):e019578. doi: 10.1161/JAHA.120.019578
53. Motoki N, Motoki H, Utsumi M, Yamazaki S, Obinata H, Takei K, et al. Identification of metabolomic profile related to adult fontan pathophysiology. Int J Cardiol Heart Vasc. (2021) 37:100921. doi: 10.1016/j.ijcha.2021.100921
54. Frank BS, Khailova L, Dekermanjian J, Mitchell MB, Morgan GJ, Twite M, et al. Interstage single ventricle heart disease infants show dysregulation in multiple metabolic pathways: targeted metabolomics analysis. JACC Adv. (2023) 2(1):1–13. doi: 10.1016/j.jacadv.2022.100169
55. Sabapathy D, Klawitter J, Silveira L, Khailova L, Mitchell MB, Morgan GJ, et al. Activation of kynurenine pathway of tryptophan metabolism after infant cardiac surgery with cardiopulmonary bypass: a prospective cohort study. Metabolomics. (2020) 16(9):93. doi: 10.1007/s11306-020-01714-x
56. Kargl S, Maier R, Gitter R, Pumberger W. Necrotizing enterocolitis after open cardiac surgery for congenital heart defects–a serious threat. Klin Padiatr. (2013) 225(1):24–8. doi: 10.1055/s-0032-1331724
57. Weiss SL, Gossett JG, Kaushal S, Wang D, Backer CL, Wald EL. Comparison of gastrointestinal morbidity after Norwood and hybrid palliation for complex heart defects. Pediatr Cardiol. (2011) 32(4):391–8. doi: 10.1007/s00246-010-9864-9
58. Ostrow AM, Freeze H, Rychik J. Protein-losing enteropathy after fontan operation: investigations into possible pathophysiologic mechanisms. Ann Thorac Surg. (2006) 82(2):695–700. doi: 10.1016/j.athoracsur.2006.02.048
59. Poulsen SS, Kissow H, Hare K, Hartmann B, Thim L. Luminal and parenteral TFF2 and TFF3 dimer and monomer in two models of experimental colitis in the rat. Regul Pept. (2005) 126(3):163–71. doi: 10.1016/j.regpep.2004.09.007
60. Wright NA. Aspects of the biology of regeneration and repair in the human gastrointestinal tract. Philos Trans R Soc Lond B Biol Sci. (1998) 353(1370):925–33. doi: 10.1098/rstb.1998.0257
61. Vlasakova K, Steinhilber A, Bailey WJ, Erdos Z, Haag H, Joos T, et al. Plasma biomarkers TAP, CPA1, and CPA2 for the detection of pancreatic injury in rat: the development of a novel multiplex IA-LC-MS/MS assay and biomarker performance evaluation. Arch Toxicol. (2023) 97(3):769–85. doi: 10.1007/s00204-022-03425-9
62. Einer C, Hohenester S, Wimmer R, Wottke L, Artmann R, Schulz S, et al. Data on chow, liver tissue and mitochondrial fatty acid compositions as well as mitochondrial proteome changes after feeding mice a western diet for 6-24 weeks. Data Brief. (2017) 15:163–9. doi: 10.1016/j.dib.2017.09.019
63. Gebert N, Cheng CW, Kirkpatrick JM, Di Fraia D, Yun J, Schadel P, et al. Region-specific proteome changes of the intestinal epithelium during aging and dietary restriction. Cell Rep. (2020) 31(4):107565. doi: 10.1016/j.celrep.2020.107565
64. Goldberg CS, Schwartz EM, Brunberg JA, Mosca RS, Bove EL, Schork MA, et al. Neurodevelopmental outcome of patients after the fontan operation: a comparison between children with hypoplastic left heart syndrome and other functional single ventricle lesions. J Pediatr. (2000) 137(5):646–52. doi: 10.1067/mpd.2000.108952
65. Pike NA, Roy B, Gupta R, Singh S, Woo MA, Halnon NJ, et al. Brain abnormalities in cognition, anxiety, and depression regulatory regions in adolescents with single ventricle heart disease. J Neurosci Res. (2018) 96(6):1104–18. doi: 10.1002/jnr.24215
66. Sarrechia I, Miatton M, De Wolf D, Francois K, Gewillig M, Meyns B, et al. Neurocognitive development and behaviour in school-aged children after surgery for univentricular or biventricular congenital heart disease. Eur J Cardiothorac Surg. (2016) 49(1):167–74. doi: 10.1093/ejcts/ezv029
67. International Cardiac Collaborative on Neurodevelopment I. Impact of operative and postoperative factors on neurodevelopmental outcomes after cardiac operations. Ann Thorac Surg. (2016) 102(3):843–9. doi: 10.1016/j.athoracsur.2016.05.081
68. Goldberg CS, Lu M, Sleeper LA, Mahle WT, Gaynor JW, Williams IA, et al. Factors associated with neurodevelopment for children with single ventricle lesions. J Pediatr. (2014) 165(3):490–6.e8. doi: 10.1016/j.jpeds.2014.05.019
69. Patt E, Singhania A, Roberts AE, Morton SU. The genetics of neurodevelopment in congenital heart disease. Can J Cardiol. (2023) 39(2):97–114. doi: 10.1016/j.cjca.2022.09.026
70. Hu QD, Ang BT, Karsak M, Hu WP, Cui XY, Duka T, et al. F3/contactin acts as a functional ligand for notch during oligodendrocyte maturation. Cell. (2003) 115(2):163–75. doi: 10.1016/S0092-8674(03)00810-9
71. Bizzoca A, Corsi P, Gennarini G. The mouse F3/contactin glycoprotein: structural features, functional properties and developmental significance of its regulated expression. Cell Adh Migr. (2009) 3(1):53–63. doi: 10.4161/cam.3.1.7462
72. Gulisano W, Bizzoca A, Gennarini G, Palmeri A, Puzzo D. Role of the adhesion molecule F3/contactin in synaptic plasticity and memory. Mol Cell Neurosci. (2017) 81:64–71. doi: 10.1016/j.mcn.2016.12.003
73. Schmitt JE, Raznahan A, Liu S, Neale MC. The genetics of cortical myelination in young adults and its relationships to cerebral surface area, cortical thickness, and intelligence: a magnetic resonance imaging study of twins and families. Neuroimage. (2020) 206:116319. doi: 10.1016/j.neuroimage.2019.116319
74. Williamson BJ, Barnes-Davis ME, Vannest J, Anixt JS, Heydarian HC, Kuan L, et al. Altered white matter connectivity in children with congenital heart disease with single ventricle physiology. Sci Rep. (2023) 13(1):1318. doi: 10.1038/s41598-023-28634-9
75. van Tilborg E, Achterberg EJM, van Kammen CM, van der Toorn A, Groenendaal F, Dijkhuizen RM, et al. Combined fetal inflammation and postnatal hypoxia causes myelin deficits and autism-like behavior in a rat model of diffuse white matter injury. Glia. (2018) 66(1):78–93. doi: 10.1002/glia.23216
76. Williamson JM, Lyons DA. Myelin dynamics throughout life: an ever-changing landscape? Front Cell Neurosci. (2018) 12:424. doi: 10.3389/fncel.2018.00424
77. Bokesch PM, Appachi E, Cavaglia M, Mossad E, Mee RB. A glial-derived protein, S100B, in neonates and infants with congenital heart disease: evidence for preexisting neurologic injury. Anesth Analg. (2002) 95(4):889–92. doi: 10.1213/00000539-200210000-00018
78. Vaage J, Anderson R. Biochemical markers of neurologic injury in cardiac surgery: the rise and fall of S100beta. J Thorac Cardiovasc Surg. (2001) 122(5):853–5. doi: 10.1067/mtc.2001.119055
79. Shahim P, Politis A, van der Merwe A, Moore B, Ekanayake V, Lippa SM, et al. Time course and diagnostic utility of NfL, tau, GFAP, and UCH-L1 in subacute and chronic TBI. Neurology. (2020) 95(6):e623–e36. doi: 10.1212/WNL.0000000000009985
80. Frieden LA, Townsend TA, Vaught DB, Delaughter DM, Hwang Y, Barnett JV, et al. Regulation of heart valve morphogenesis by eph receptor ligand, ephrin-A1. Dev Dyn. (2010) 239(12):3226–34. doi: 10.1002/dvdy.22458
81. Pedrazzini T. Control of cardiogenesis by the notch pathway. Trends Cardiovasc Med. (2007) 17(3):83–90. doi: 10.1016/j.tcm.2007.01.003
82. Garside VC, Chang AC, Karsan A, Hoodless PA. Co-ordinating notch, BMP, and TGF-beta signaling during heart valve development. Cell Mol Life Sci. (2013) 70(16):2899–917. doi: 10.1007/s00018-012-1197-9
83. Yamagishi T, Ando K, Nakamura H. Roles of TGFbeta and BMP during valvulo-septal endocardial cushion formation. Anat Sci Int. (2009) 84(3):77–87. doi: 10.1007/s12565-009-0027-0
84. Diaz Del Moral S, Benaouicha M, Munoz-Chapuli R, Carmona R. The insulin-like growth factor signalling pathway in cardiac development and regeneration. Int J Mol Sci. (2021) 23(1):234–45. doi: 10.3390/ijms23010234
85. Sedmera D, Thompson RP. Myocyte proliferation in the developing heart. Dev Dyn. (2011) 240(6):1322–34. doi: 10.1002/dvdy.22650
86. Wu CC, Kruse F, Vasudevarao MD, Junker JP, Zebrowski DC, Fischer K, et al. Spatially resolved genome-wide transcriptional profiling identifies BMP signaling as essential regulator of zebrafish cardiomyocyte regeneration. Dev Cell. (2016) 36(1):36–49. doi: 10.1016/j.devcel.2015.12.010
87. Gemberling M, Bailey TJ, Hyde DR, Poss KD. The zebrafish as a model for complex tissue regeneration. Trends Genet. (2013) 29(11):611–20. doi: 10.1016/j.tig.2013.07.003
88. Patterson M, Barske L, Van Handel B, Rau CD, Gan P, Sharma A, et al. Frequency of mononuclear diploid cardiomyocytes underlies natural variation in heart regeneration. Nat Genet. (2017) 49(9):1346–53. doi: 10.1038/ng.3929
89. Krawutschke C, Koesling D, Russwurm M. Cyclic GMP in vascular relaxation: export is of similar importance as degradation. Arterioscler Thromb Vasc Biol. (2015) 35(9):2011–9. doi: 10.1161/ATVBAHA.115.306133
90. Garcia AM, Nakano SJ, Karimpour-Fard A, Nunley K, Blain-Nelson P, Stafford NM, et al. Phosphodiesterase-5 is elevated in failing single ventricle myocardium and affects cardiomyocyte remodeling in vitro. Circ Heart Fail. (2018) 11(9):e004571. doi: 10.1161/CIRCHEARTFAILURE.117.004571
91. Ponomarenko EA, Poverennaya EV, Ilgisonis EV, Pyatnitskiy MA, Kopylov AT, Zgoda VG, et al. The size of the human proteome: the width and depth. Int J Anal Chem. (2016) 2016:7436849. doi: 10.1155/2016/7436849
92. Kim MS, Pinto SM, Getnet D, Nirujogi RS, Manda SS, Chaerkady R, et al. A draft map of the human proteome. Nature. (2014) 509(7502):575–81. doi: 10.1038/nature13302
Keywords: biomarkers, congenital heart disease, congenital heart defect, Glenn, hypoplastic left heart syndrome, inflammation, protein dysregulation, single ventricle palliation
Citation: Thomson LM, Mancuso CA, Wolfe KR, Khailova L, Niemiec S, Ali E, DiMaria M, Mitchell M, Twite M, Morgan G, Frank BS and Davidson JA (2023) The proteomic fingerprint in infants with single ventricle heart disease in the interstage period: evidence of chronic inflammation and widespread activation of biological networks. Front. Pediatr. 11:1308700. doi: 10.3389/fped.2023.1308700
Received: 6 October 2023; Accepted: 20 November 2023;
Published: 8 December 2023.
Edited by:
Petru Liuba, Lund University, SwedenReviewed by:
Tina Oak Findley, University of Texas Health Science Center at Houston, United StatesAndrew D. Spearman, Medical College of Wisconsin, United States
© 2023 Thomson, Mancuso, Wolfe, Khailova, Niemiec, Ali, DiMaria, Mitchell, Twite, Morgan, Frank and Davidson. This is an open-access article distributed under the terms of the Creative Commons Attribution License (CC BY). The use, distribution or reproduction in other forums is permitted, provided the original author(s) and the copyright owner(s) are credited and that the original publication in this journal is cited, in accordance with accepted academic practice. No use, distribution or reproduction is permitted which does not comply with these terms.
*Correspondence: Lindsay M. Thomson lindsay.thomson@childrenscolorado.org
†These authors have contributed equally to this work and share senior authorship