- 1Neonatal Intensive Care Unit, The First Affiliated Hospital of Zhengzhou University, Zhengzhou, China
- 2Clinical Treatment and Follow-Up Center for High-Risk Newborns of Henan Province, Zhengzhou, China
- 3Key Laboratory for Prevention and Control of Developmental Disorders, Zhengzhou, China
- 4Computer Science and Technology, University of Science and Technology of China, Hefei, China
- 5Department of Orthopaedics, The First Affiliated Hospital of Zhengzhou University, Zhengzhou, China
- 6Health Care Department, The First Affiliated Hospital of Zhengzhou University, Zhengzhou, China
Objectives: This study aimed to analyze the predictive value of umbilical cord blood Interleukin-6 (UCB IL-6) for the severity-graded BPD and to establish machine learning (ML) predictive models in a Chinese population based on the 2019 NRN evidence-based guidelines.
Methods: In this retrospective analysis, we included infants born with gestational age <32 weeks, who underwent UCB IL-6 testing within 24 h of admission to our NICU between 2020 and 2022. We collected their medical information encompassing the maternal, perinatal, and early neonatal phases. Furthermore, we classified the grade of BPD according to the 2019 NRN evidence-based guidelines. The correlation between UCB IL-6 and the grades of BPD was analyzed. Univariate analysis and ordinal logistic regression were employed to identify risk factors, followed by the development of ML predictive models based on XGBoost, CatBoost, LightGBM, and Random Forest. The AUROC was used to evaluate the diagnostic value of each model. Besides, we generated feature importance distribution plots based on SHAP values to emphasize the significance of UCB IL-6 in the models.
Results: The study ultimately enrolled 414 preterm infants, with No BPD group (n = 309), Grade 1 BPD group (n = 73), and Grade 2–3 BPD group (n = 32). The levels of UCB IL-6 increased with the grades of BPD. UCB IL-6 demonstrated clinical significance in predicting various grades of BPD, particularly in distinguishing Grade 2–3 BPD patients, with an AUROC of 0.815 (95% CI: 0.753–0.877). All four ML models, XGBoost, CatBoost, LightGBM, and Random Forest, exhibited Micro-average AUROC values of 0.841, 0.870, 0.851, and 0.878, respectively. Notably, UCB IL-6 consistently appeared as the most prominent feature across the feature importance distribution plots in all four models.
Conclusion: UCB IL-6 significantly contributes to predicting severity-graded BPD, especially in grade 2–3 BPD. Through the development of four ML predictive models, we highlighted UCB IL-6's importance.
1. Introduction
Bronchopulmonary dysplasia (BPD) is a chronic respiratory disorder originating in the neonatal period and is a major complication among premature infants. BPD results in long-term pulmonary issues, imposing significant economic burdens on both society and families (1). With advancements in neonatal care technology, more premature infants are able to survive, leading to a gradual increase in the global incidence of BPD (2). Effective treatment strategies for BPD are currently lacking (3). The prognosis for newborns with different grades of BPD varies, with a higher likelihood of mortality and neurological damage observed in those with more severe BPD (4). Assessing the severity-graded BPD usually happens late, either by 36 weeks of postnatal menstrual age (PMA) or upon discharge home. This isn't conducive for guiding treatment and devising effective strategies. Hence, early prediction of BPD grade is vital, making the exploration of predictive factors for early grading BPD crucial.
Interleukin-6 (IL-6) is a key member of the cytokine family, participating in cell-to-cell signaling and serving a crucial regulatory function in the immune system. IL-6 can accelerate the progression of lung inflammation (5). Bioinformatics analysis indicates that IL-6 is among the top ten hub genes in BPD, and both mRNA and protein levels of IL-6 are highly expressed in the peripheral blood of newborns with BPD (6). Umbilical cord blood (UCB) is non-invasive for newborns and can be collected early, making it a compelling choice for disease prediction. Previous studies have indicated a link between UCB IL-6 and the occurrence of BPD, suggesting it as a potential biomarker for predicting BPD (7–9). However, the relationship between UCB IL-6 and severity grade of BPD has yet to be investigated.
While numerous prediction tools exist for BPD, there is a scarcity of predictive models specifically addressing the severity grade of BPD (10). Most models still rely on the 2001 NICHD guidelines for assessing and grading BPD. Unfortunately, this standard is no longer applicable, failing to reflect the contemporary neonatal respiratory care practices such as the widespread use of high-flow nasal cannula (11). In 2019, a modern, evidence-based definition of BPD gained widespread recognition. This definition, established by the Neonatal Research Network (NRN), categorizes the grades of BPD based on the respiratory support patterns at PMA 36 weeks (12). When developing predictive models, artificial intelligence (AI) offers increased flexibility, allowing adaptation to diverse data types (13). AI models are particularly adept at capturing complex nonlinear relationships in data, a task that can be challenging for traditional models. In the realm of AI, machine learning (ML) is a key subfield that involves the automated identification of methods and parameters within data to find optimal solutions. Furthermore, ML models hold the potential to establish objective classification standards, thus enhancing the reliability and effectiveness of the models. ML models have emerged as promising predictive tools widely applied in clinical settings (14).
In this study, our aim is to explore the predictive value of UCB IL-6 for grading BPD and to establish effective early predictive models in a Chinese population using ML based on the 2019 NRN standards.
2. Materials and methods
2.1. Patients
We conducted a retrospective cohort study, approved by the Ethics Committee of the First Affiliated Hospital of Zhengzhou University, with the ethics approval number: 2019-KY-95. All participants provided informed consent from their parents. The study's inclusion criteria were as follows: (1) gestational age (GA) at birth of less than 32 weeks, (2) admission to the NICU between January 2020 and December 2022 with survival until PMA 36 weeks, and (3) completion of UCB IL-6 testing within 24 h of admission. Exclusion criteria encompassed (1) significant congenital anomalies or chromosomal abnormalities, (2) death or discharge against medical advice before reaching PMA 36 weeks, and (3) incomplete data. The flow chart is shown in Figure 1.
2.2. Data collection and UCB IL-6 determinations
We retrospectively obtained clinical data from the hospital's electronic medical record system, including maternal factors, delivery characteristics, neonatal factors, respiratory support and initial laboratory tests. Respiratory support details encompassed initial modes of continuous positive airway pressure (CPAP), initial inspiratory oxygen concentration (FiO2), and invasive ventilation. Initial laboratory tests covered UCB IL-6, N-terminal pro-brain natriuretic peptide (NT-proBNP), white blood cell count (WBC), pH value, chloride ion concentration, sodium ion concentration, and blood glucose levels, all collected within 24 h of admission.
The detection of UCB IL-6 concentration was performed using a multiplex microsphere flow cytometry assay (BD FACSCantoTM II flow cytometer manufactured by BD Company, Qingdao, China). The normal range for UCB IL-6 is 0–5.4 pg/ml.
Pregnancy induced hypertension is defined as systolic blood pressure of 140 mmHg or higher, diastolic blood pressure of 90 mmHg or higher, or the presence of both after 20 weeks of gestation (15). Gestational diabetes mellitus (GDM) refers to any degree of impaired glucose tolerance during pregnancy, regardless of whether diabetes was present prior to pregnancy (16). Preterm rupture of membranes (PROM) is defined as the rupture of fetal membranes before the onset of labor (17). Fetal growth restriction is defined as an estimated fetal weight or abdominal circumference below the 10th percentile for GA on ultrasound (18). According to the Fenton Chart, a birth weight (BW) at or below the 10th percentile of the average BW for the same GA is classified as small for gestational age (SGA) (19). All this data was obtained from the obstetric electronic medical records on the day of birth.
Respiratory distress syndrome (RDS) and intraventricular hemorrhage (IVH) are defined according to standard criteria (20, 21). Respiratory failure (RF) was diagnosed based on clinical features and laboratory results (22). White matter injury (WMI) diagnosis involved the use of Doppler ultrasound imaging. Hemodynamically significant patent ductus arteriosus (HsPDA) is defined as a ductal diameter of 1.5 mm or larger, and the diagnosis is confirmed through echocardiography, which shows abnormal blood flow from the aorta to the pulmonary artery (23, 24). Neonatal sepsis (NS) diagnosis includes both culture-based and culture-independent methods (25). All the aforementioned clinical complications were collected within 7 days.
2.3. Definition of study outcomes
2.3.1. Primary outcome
In our study, the primary outcome focuses on diagnosing and grading BPD. According to the 2019 NRN evidence-based guidelines (12), severity-graded definitions of BPD are based on the mode of respiratory support at PMA 36 weeks, regardless of prior or current oxygen therapy: No BPD, no support; Grade 1 BPD, nasal cannula at flow rates ≤2 L/min; Grade 2 BPD, nasal cannula at flow rates >2 L/min or noninvasive positive airway pressure; and Grade 3 BPD, invasive mechanical ventilation. Since there were only two cases of Grade 3 BPD, we combined them with the Grade 2 BPD cases, creating a single group referred to as Grade 2–3 BPD.
2.3.2. Secondary outcome
Furthermore, to explore the impact of different grades of BPD on clinical burden, we conducted supplementary analyses on length of hospital stay, total cost, and corrected age at discharge. Hospital length of stay is the entire duration from admission to discharge, measured in days. The total cost we obtained represents all expenses documented in the hospital's records during hospitalization. Corrected age at discharge was calculated by subtracting the number of weeks a child was born prematurely to her/his chronological age at the time of discharge to home (26).
2.4. Data analysis
For normally distributed numerical variables, we utilized mean ± SD for presentation. Conversely, for numerical variables that did not follow a normal distribution, we opted the median (P25–P75) for representation. Additionally, to meet the normal distribution assumptions, logarithmic transformations were applied to the values of UCB IL-6 and NT-proBNP using the natural logarithm (base e). Normally distributed variables were analyzed using analysis of variance (ANOVA), while non-normally distributed variables were assessed using the Kruskal-Wallis H test. For variables with significant P values, further pairwise comparisons were conducted. Categorical data were expressed as counts (%), and intergroup comparisons were performed using either the chi-square test or Fisher's exact test, as appropriate. Subsequently, the main objective was to assess the correlation between UCB IL-6 and the severity grades of BPD through logistic regression analysis.
Factors with P values <0.10 were selected for regression analysis. Univariate and multivariate ordinal logistic regression analyses were performed. Predictive models were established using all statistically significant risk factors (P < 0.05) identified through the multivariate analysis.
2.5. Model development and evaluation
2.5.1. Model development
In the field of ML, ensemble learning algorithms are widely acknowledged as superior to traditional single-model methods. The two main branches of ensemble learning are boosting and bagging, with XGBoost, LightGBM, CatBoost, GBDT, and AdaBoost belonging to boosting algorithms, while Random Forest represents the bagging method. AdaBoost, functioning as a standalone model, is limited to binary classification and cannot be employed for multi-class tasks. Notably, XGBoost, LightGBM, and Catboost are all improved versions of GBDT. Therefore, in this study, we employed the four most commonly used methods in similar research endeavors (27–29): XGBoost, LightGBM, Catboost, and RF.
Ten-fold cross-validation was utilized to train and evaluate our four models. The dataset was split into ten uniform partitions using stratified sampling, maintaining consistent proportions for each category within every partition, mirroring the original dataset. Each model underwent ten rounds of training and evaluation, with nine subsets used for training and one subset for testing in each iteration. This tenfold process ensured each subset was used for testing once. The final evaluation represented the average of these ten assessment rounds, providing a more stable assessment of model performance by reducing the impact of random data partitioning. To address the imbalance in sample sizes among different classes in our dataset, we applied balanced sampling to maintain consistent training across all categories. Additionally, we conducted a grid hyperparameter search to optimize the models' performance.
2.5.2. Model evaluation
We used Receiver Operating Characteristic (ROC) curves and Area Under the Curve (AUC) to assess the models’ classification performance. We not only utilized Macro-average ROC for an overall evaluation but also employed Micro-average ROC to mitigate class imbalance, offering a more accurate reflection of the models' predictive capabilities. Apart from these metrics, we also utilized F1 score, precision, recall and accuracy to assess the models' performance. Furthermore, we calculated SHAP values and generated feature importance distribution plots to evaluate the significance of UCB IL-6 in each model.
2.5.3. Experiment setting
All our experiments were based on Python 3.8, utilizing the following libraries: scikit-learn 0.24.1, XGBoost, LightGBM, Catboost, and GridSearch. We set the random seed to 42. The trained models and inference evaluation codes have been uploaded to GitHub. You can click the following link to view them (https://github.com/1183371714/GBD).
3. Results
3.1. Demographics and clinical burden
The research ultimately included 414 preterm infants, with merely two cases falling into the Grade 3 BPD category. All patients were divided into three groups: the No BPD group (n = 309), the Grade 1 BPD group (n = 73), and the Grade 2–3 BPD group (n = 32). The demographic characteristics and clinical burden of the patients are detailed in Table 1. It's clear that infants with more severe BPD tend to have a lower GA, and those in the No BPD group have the highest BW. Additionally, Grade 2–3 BPD infants have a higher proportion of males compared to the No BPD group. Notably, the analysis of differences in clinical burdens among the three groups indicates that groups with higher BPD grades experience heavier clinical burdens.
In the univariate analysis, infants with Grade 2–3 BPD were less likely to receive antenatal corticosteroid treatment. After birth, significant differences were observed among the different groups in Apgar scores and rates of asphyxia. Infants with a higher grade of BPD exhibited lower Apgar scores and a greater likelihood of requiring resuscitation for asphyxia. The risk factors during the antepartum and perinatal periods are listed in Table 2.
Patients with No BPD initially require the lowest FiO2 and are most likely to receive initial breathing support through CPAP. Grade 2–3 BPD patients require the highest FiO2 and are most likely to receive initial support through invasive ventilation. Compared to the No BPD group, Grade 2–3 BPD patients are more likely to receive surfactant therapy. Among the three groups, Grade 2–3 BPD patients have the highest levels of initial NT-proBNP and UCB IL-6, while having the lowest pH values. Children in the No BPD group have the lowest likelihood of experiencing pulmonary hemorrhage and HsPDA. The more severe the condition, the higher the likelihood of RF, pulmonary hypertension, and IVH. The early postnatal clinical characteristics are presented in Table 3.
3.2. The predictive value of UCB IL-6 levels for the severity grade of BPD
The comparison of UCB IL-6 levels among different BPD groups is illustrated in Figure 2. Significant differences (P < 0.05) were observed between any two groups, with higher UCB IL-6 levels in the groups with more severe BPD.
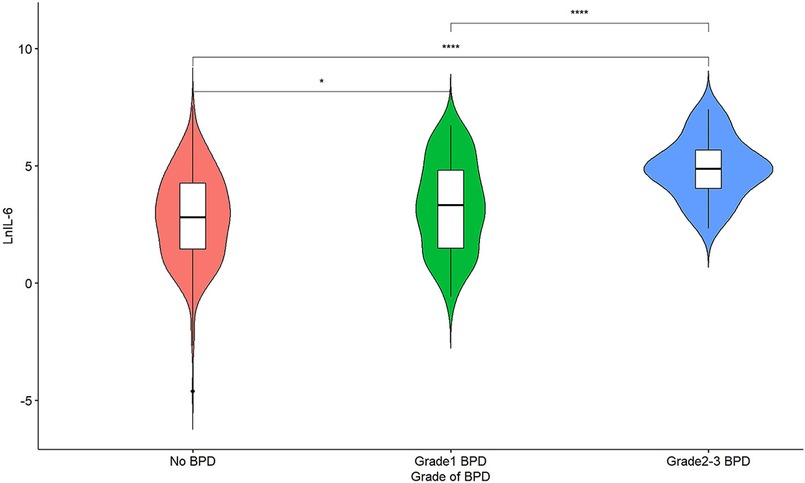
Figure 2. A violin plot of lnIL-6 levels among different grade groups of BPD; *represents P < 0.05, and ****represents P < 0.0001.
UCB IL-6 levels were not effective in distinguishing between infants with Grade 1 BPD and those with No BPD. However, as illustrated in Figure 3, in ROC curve a, UCB IL-6 effectively distinguishes infants with Grade 2–3 BPD from those with No BPD, with an AUC of 0.815 (95% CI: 0.753–0.877). In ROC curve b, UCB IL-6 demonstrates predictive potential for distinguishing between less severe (Grade 0–1) and more severe (Grade 2–3) BPD, supported by an AUC of 0.800 (95% CI: 0.738–0.862). The optimal cutoff value for UCB IL-6 in both cases was 47.70 pg/ml. The sensitivity was 81.3% in both cases, with specificities of 70.2% and 68.3%, respectively. Within the subgroup of BPD patients, ROC curve c demonstrates UCB IL-6's predictive value for higher-grade BPD (Grade 2–3 BPD) with an AUC of 0.737 (95% CI: 0.642–0.831). The optimal cutoff value was 76.71 pg/ml, providing a sensitivity of 71.9% and specificity of 69.9%. In ROC curve d, the ROC curve demonstrates the predictive ability of UCB IL-6 for BPD occurrence. The AUC was calculated as 0.644 (95% CI: 0.582–0.706), with an optimal cutoff value of 90.92 pg/ml. Sensitivity and specificity were 41.9% and 81.2%, respectively.
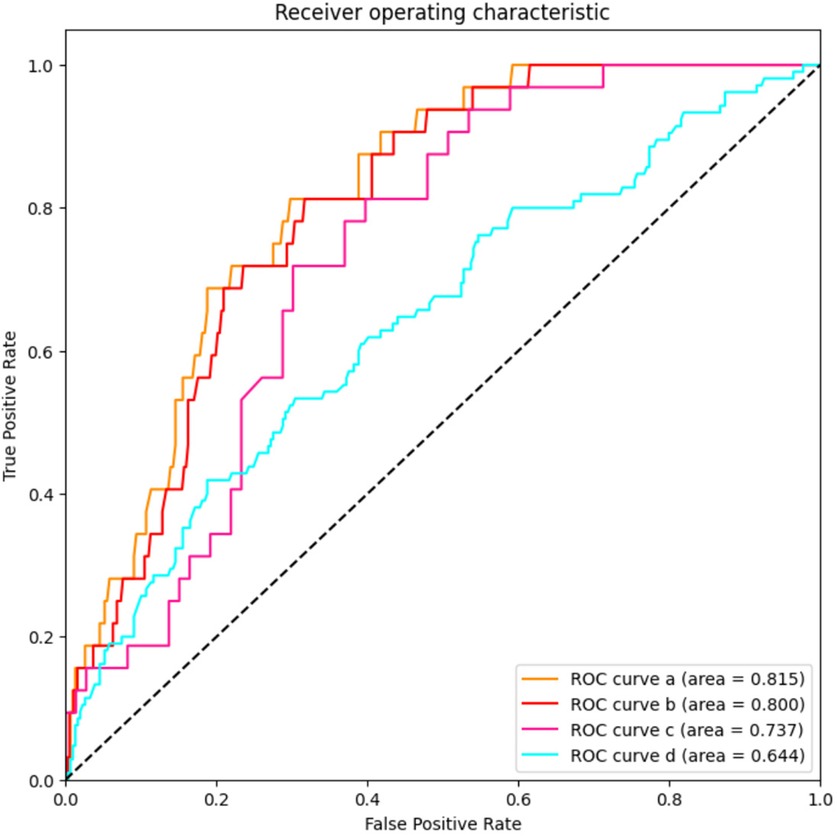
Figure 3. The predictive value of umbilical cord blood IL-6 levels for the grade of BPD. AUC stands for area under the receiver operating characteristic curve; and BPD stands for bronchopulmonary dysplasia. ROC curve a: LnIL-6 levels for distinguishes infants with Grade 2–3 BPD from those with No BPD; ROC curve b: LnIL-6 levels for distinguishing between less severe (Grade 0–1) and more severe (Grade 2–3) BPD; ROC curve c: LnIL-6 levels’ predictive value for higher-grade BPD (Grade 2–3 BPD) in patients with BPD; ROC curve d: LnIL-6 levels for prediction of BPD occurrence.
3.3. Multivariable analysis
The results of the ordinal logistic regression analysis are presented in Table 4. Patients were categorized based on severity grade as follows: No BPD as 0, Grade 1 BPD as 1, and Grade 2–3 BPD as 2. According to the logistic regression analysis, UCB IL-6, NT-proBNP, pH value, Apgar scores at 1 and 5 min, perinatal asphyxia, initial FiO2, invasive ventilation, surfactant therapy, HsPDA, RF, and IVH were identified as independent risk factors for grading BPD.
3.4. Model performance and feature importance distribution
Twelve clinical factors with significant differences in multivariate analysis, as well as key factors (gender, GA and BW), were incorporated into ML models. ROC curves were derived through ten-fold cross-validation. The Micro-average AUROC values for the XGBoost, CatBoost, LightGBM, and Random Forest models were 0.841, 0.870, 0.851, and 0.878, respectively (Figure 4). Detailed metrics for these four models can be found in Supplementary Table 1.
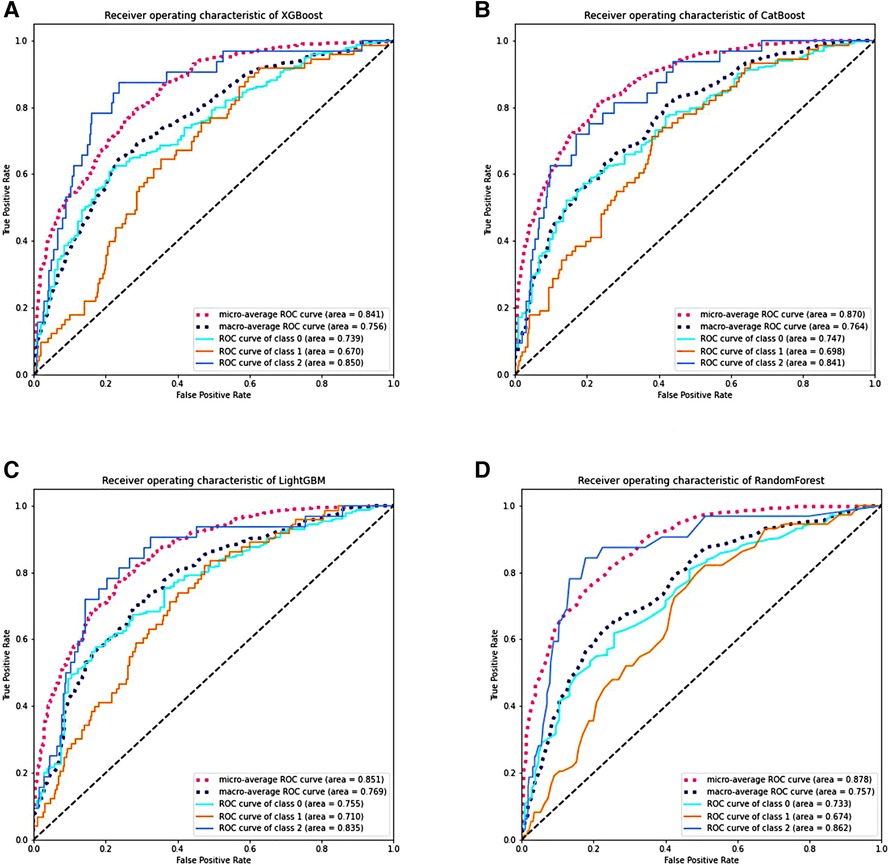
Figure 4. The performance of various machine learning models through ten-fold cross-validation. (A–D) Display the ROC curves of the XGBoost, CatBoost, LightGBM, and RF models. Class 0 represents No BPD; class 1 represents Grade 1 BPD, and class 2 represents Grade 2–3 BPD. RF stands for random forest, ROC stands for receiver operating characteristic, AUC stands for area under the receiver operating characteristic curve, and BPD stands for bronchopulmonary dysplasia.
Based on SHAP values, Figure 5 illustrates the feature importance distribution for each model. It is evident that UCB IL-6 is the most crucial feature across all models.
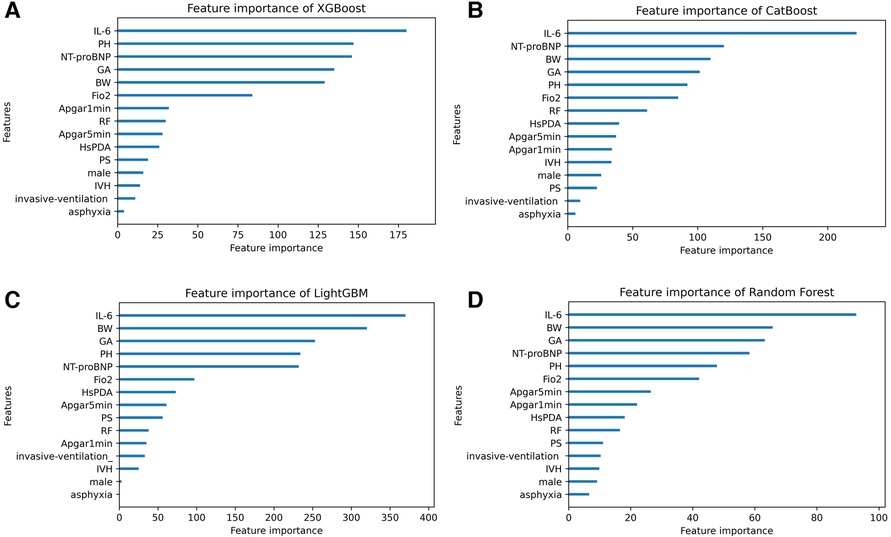
Figure 5. The feature importance distribution charts of four machine learning models. (A–D) Display the feature importance distribution charts of the XGBoost, CatBoost, LightGBM, and RF models. IL-6 umbilical cord blood interleukin-6, BW birth weight, GA gestational age, PH pH value, FiO2 fraction of inspiration O2, RF respiratory failure, HsPDA hemodynamically significant patent ductus arteriosus, IVH intraventricular hemorrhage, PS pulmonary surfactant.
4. Discussion
We verified the predictive significance of UCB IL-6 for severity-graded BPD among a Chinese cohort, in accordance with the 2019 NRN guidelines. Based on the results of univariate analysis, UCB IL-6 levels exhibited an increase in tandem with the grades of BPD. Our study demonstrated that UCB IL-6 predicts the occurrence of BPD. Additionally, it predicts the presence of Grades 2–3 BPD when compared to either No BPD or Grade 1 BPD. What's more, ordinal logistic regression analyses indicated that UCB IL-6 is a predictive factor for grading BPD. UCB IL-6 predicts Grade 2–3 BPD better than Grade 1 BPD, as do the four ML models we developed. It was observed that the UCB IL-6 factor consistently ranked as the top feature in the feature importance distribution charts of the four models.
The 2001 NICHD criteria for BPD are outdated due to advancements in respiratory support methods. The 2018 NICHD criteria appear to be somewhat complex. The 2019 NRN guidelines are easy and effective, and using them to assess the severity-graded BPD can provide the most accurate predictions regarding infant outcomes at 18–26 months of corrected age (12). Based on the 2019 NRN standards, it was found that infants classified with a higher grade BPD have a significantly higher risk of requiring tracheostomy or facing mortality compared to those with a lower grade of BPD (30). Early prediction of severity-graded BPD under the 2019 NRN standards is necessary, as it can predict clinical burden, guide treatment, and inform follow-up strategies.
Yan et al. (6) found that the higher the grade of BPD, the higher the concentration of peripheral blood IL-6. Cansu Yılmaz et al. (31) found that elevated levels of IL-6 in tracheal aspirates of newborns were associated with the severity grade of BPD. In contrast, our study focuses on UCB, obtained at an earlier time point, and poses lower invasiveness to the neonates. IL-6 may play a pivotal role in the pathogenesis of BPD. One possible explanation is that activation of IL-6 signaling in macrophages by high oxygen impairs the homeostasis of alveolar type II epithelial cells and disrupts the formation of elastic fibers, thereby inhibiting lung growth (32). Additionally, studies suggest that the crosstalk between inflammation and cell death might be associated with oxygen-induced lung injury in BPD. Future therapeutic approaches for BPD should be centered on suppressing the expression of cytokines (33). Zhang et al. (34) revealed that BPD might be influenced by the inflammatory response to the gut microbiota. Further in-depth research and exploration are necessary to elucidate the specific underlying mechanisms.
Most predictive models traditionally relied on statistical methods. However, there is a recent surge in utilizing AI techniques to develop BPD prediction models. For example, a study integrated clinical data and genomics to construct a ML model for predicting BPD and severe BPD, achieving an AUC of 0.872 (35). In 2023, Wen He and colleagues developed multiple ML models for predicting BPD severity using clinical data, with the highest AUC reaching 0.86 (10). However, it's important to note that their criteria for diagnosing and grading BPD were based on the 2001 NICHD guidelines, which might not fully account for advances in respiratory support technology today, limiting the practical use of their models. In contrast, our models adhere to the more up-to-date 2019 NRN standards, making them more suitable for clinical application. Furthermore, our models include new predictive factors—NT-proBNP and UCB IL-6. Notably, UCB IL-6, being non-invasive, consistently held the top rank in the feature importance distribution charts within all four models, signaling its significance.
Other than UCB IL-6, we observed that in our four models, the top five factors in terms of importance also include BW, GA, NT-proBNP, pH value, and FiO2. Factors that impede alveolar maturation, such as BW and GA, are considered crucial elements in the pathology of BPD. Previous studies have demonstrated that NT-proBNP levels can predict moderate-to-severe BPD or mortality (36–38). Given that, in this study, based on the 2019 NRN criteria, NT-proBNP remains a significant risk factor for grading BPD, further attention should be directed towards understanding how NT-proBNP influences infant lung development. According to our study, timely correction of premature infants' pH levels and prevention of acidosis are crucial. Additionally, we noticed high initial FiO2 levels as an independent risk. One possible reason is that elevated oxygen triggers reactive oxygen species, harming the lungs (39).
However, our study has limitations. The small number of patients with Grade 3 BPD in our level III NICU forces us to combine it with Grade 2 BPD, representing a more severe condition. Therefore, we are unable to predict the likelihood of an infant developing grade 3 BPD specifically; instead, we can only predict whether the newborn is at a more severe level of BPD. Being retrospective, confounding factors weren't controlled. Additionally, our data were collected from a single center, potentially not representing all patients. Future research requires a prospective study with a larger sample size, multiple centers, and external validation to incorporate UCB IL-6 in predicting preterm infant outcomes.
5. Conclusion
Our research focused on preterm infants with GA less than 32 weeks. We developed four ML models and found that UCB IL-6 plays a crucial role in predicting the severity grade of BPD.
Data availability statement
The raw data supporting the conclusions of this article will be made available by the authors, without undue reservation.
Ethics statement
The studies involving humans were approved by the Ethics Committee of the First Affiliated Hospital of Zhengzhou University. The studies were conducted in accordance with the local legislation and institutional requirements. Written informed consent for participation in this study was provided by the participants’ legal guardians/next of kin.
Author contributions
LG: Data curation, Formal Analysis, Investigation, Methodology, Visualization, Writing – original draft. PY: Formal Analysis, Methodology, Visualization, Writing – review and editing. CL: Methodology, Writing – review and editing. ML: Methodology, Writing – review and editing. ZS: Methodology, Writing – review and editing. XC: Methodology, Writing – review and editing. JZ: Data curation, Formal Analysis, Writing – review and editing. WC: Data curation, Formal Analysis, Writing – review and editing. MR: Data curation, Formal Analysis, Writing – review and editing. LZ: Data curation, Formal Analysis, Writing – review and editing. BW: Data curation, Writing – review and editing. QZ: Project administration, Supervision, Writing – review and editing.
Funding
The author(s) declare financial support was received for the research, authorship, and/or publication of this article.
All phases of this study were supported by National Health Commission Medical and Health Science and Technology Development Center, No. WA2020HK41 (to QZ); Overseas Research and Training Project of Health Science and Technology Talents of Henan Province, No. HWYX2019066 (to QZ); and Provincial Natural Science Fund for General Projects, No. 232300420045 (to QZ).
Acknowledgments
We thank all our colleagues in the Neonatal Intensive Care Unit of the First Affiliated Hospital of Zhengzhou University, for their support of our project.
Conflict of interest
The authors declare that the research was conducted in the absence of any commercial or financial relationships that could be construed as a potential conflict of interest.
Publisher's note
All claims expressed in this article are solely those of the authors and do not necessarily represent those of their affiliated organizations, or those of the publisher, the editors and the reviewers. Any product that may be evaluated in this article, or claim that may be made by its manufacturer, is not guaranteed or endorsed by the publisher.
Supplementary material
The Supplementary Material for this article can be found online at: https://www.frontiersin.org/articles/10.3389/fped.2023.1301376/full#supplementary-material
References
1. Stoll BJ, Hansen NI, Bell EF, Shankaran S, Laptook AR, Walsh MC, et al. Neonatal outcomes of extremely preterm infants from the NICHD neonatal research network. Pediatrics. (2010) 126:443–56. doi: 10.1542/peds.2009-2959
2. Stoll BJ, Hansen NI, Bell EF, Walsh MC, Carlo WA, Shankaran S, et al. Trends in care practices, morbidity, and mortality of extremely preterm neonates, 1993-2012. J Am Med Assoc. (2015) 314:1039–51. doi: 10.1001/jama.2015.10244
3. Emma W, Anne G. Advances in treating bronchopulmonary dysplasia. Expert Rev Respir Med. (2019) 13:727–35. doi: 10.1080/17476348.2019.1637738
4. Zhang H, Dysart K, Kendrick DE, Li L, Das A, Hintz SR, et al. Prolonged respiratory support of any type impacts outcomes of extremely low birth weight infants. Pediatr Pulmonol. (2018) 53:1447–55. doi: 10.1002/ppul.24124
5. Hsiao CC, Chang JC, Tsao LY, Yang RC, Chen HN, Lee CH, et al. Correlates of elevated interleukin-6 and 8-hydroxy-2'-deoxyguanosine levels in tracheal aspirates from very low birth weight infants who develop bronchopulmonary dysplasia. Pediatr Neonatol. (2017) 58:63–9. doi: 10.1016/j.pedneo.2016.01.004
6. Yan W, Jiang M, Zheng J. Identification of key pathways and differentially expressed genes in bronchopulmonary dysplasia using bioinformatics analysis. Biotechnol Lett. (2020) 42:2569–80. doi: 10.1007/s10529-020-02986-y
7. Hagman C, Björklund LJ, Bjermer L, Hansen-Pupp I, Tufvesson E. Perinatal inflammation relates to early respiratory morbidity and lung function at 12 years of age in children born very preterm. Acta Paediatr. (2021) 110:2084–92. doi: 10.1111/apa.15815
8. Wang M, Luo C, Shi Z, Cheng X, Lei M, Cao W, et al. The relationship between cord blood cytokine levels and perinatal characteristics and bronchopulmonary dysplasia: a case-control study. Front Pediatr. (2022) 10:807932. doi: 10.3389/fped.2022.807932
9. Zhong XQ, Yan Q, Chen ZG, Jia CH, Li XH, Liang ZY, et al. Umbilical cord blood-derived exosomes from very preterm infants with bronchopulmonary dysplasia impaired endothelial angiogenesis: roles of exosomal microRNAs. Front Cell Dev Biol. (2021) 9:637248. doi: 10.3389/fcell.2021.637248
10. He W, Zhang L, Feng R, Fang WH, Cao Y, Sun SQ, et al. Risk factors and machine learning prediction models for bronchopulmonary dysplasia severity in the Chinese population. World J Pediatr. (2023) 19:568–76. doi: 10.1007/s12519-022-00635-0
11. Poindexter BB, Feng R, Schmidt B, Aschner JL, Ballard RA, Hamvas A, et al. Comparisons and limitations of current definitions of bronchopulmonary dysplasia for the prematurity and respiratory outcomes program. Ann Am Thorac Soc. (2015) 12:1822–30. doi: 10.1513/AnnalsATS.201504-218OC
12. Jensen EA, Dysart K, Gantz MG, McDonald S, Bamat NA, Keszler M, et al. The diagnosis of bronchopulmonary dysplasia in very preterm infants. An evidence-based approach. Am J Respir Crit Care Med. (2019) 200:751–9. doi: 10.1164/rccm.201812-2348OC
13. Doborjeh M, Doborjeh Z, Merkin A, Bahrami H, Sumich A, Krishnamurthi R, et al. Personalised predictive modelling with brain-inspired spiking neural networks of longitudinal MRI neuroimaging data and the case study of dementia. Neural Netw. (2021) 144:522–39. doi: 10.1016/j.neunet.2021.09.013
14. Yu X, Wu R, Ji Y, Feng Z. Bibliometric and visual analysis of machine learning-based research in acute kidney injury worldwide. Front Public Health. (2023) 11:1136939. doi: 10.3389/fpubh.2023.1136939
15. American College of Obstetricians and Gynecologists. Gestational hypertension and preeclampsia: ACOG practice bulletin summary, number 222. Obstet Gynecol. (2020) 135:1492–5. doi: 10.1097/AOG.0000000000003892
16. Bental Y, Reichman B, Shiff Y, Weisbrod M, Boyko V, Lerner-Geva L, et al. Impact of maternal diabetes mellitus on mortality and morbidity of preterm infants (24-33 weeks’ gestation). Pediatrics. (2011) 128:e848–55. doi: 10.1542/peds.2010-3443
17. American College of Obstetricians and Gynecologists. Practice bulletin no. 172: premature rupture of membranes. Obstet Gynecol. (2016) 128:e165–77. doi: 10.1097/AOG.0000000000001712
18. Martins JG, Biggio JR, Abuhamad A. Society for maternal-fetal medicine consult series #52: diagnosis and management of fetal growth restriction: (replaces clinical guideline number 3, April 2012). Am J Obstet Gynecol. (2020) 223:B2–B17. doi: 10.1016/j.ajog.2020.05.010
19. Fenton TR. A new growth chart for preterm babies: Babson and Benda’s chart updated with recent data and a new format. BMC Pediatr. (2003) 3:13. doi: 10.1186/1471-2431-3-13
20. Sweet DG, Carnielli VP, Greisen G, Hallman M, Klebermass-Schrehof K, Ozek E, et al. European consensus guidelines on the management of respiratory distress syndrome: 2022 update. Neonatology. (2023) 120:3–23. doi: 10.1159/000528914
21. Lampe R, Rieger-Fackeldey E, Sidorenko I, Turova V, Botkin N, Eckardt L, et al. Assessing key clinical parameters before and after intraventricular hemorrhage in very preterm infants. Eur J Pediatr. (2020) 179:929–37. doi: 10.1007/s00431-020-03585-9
22. Zhang J, Luo C, Lei M, Shi Z, Cheng X, Wang L, et al. Development and validation of a nomogram for predicting bronchopulmonary dysplasia in very-low-birth-weight infants. Front Pediatr. (2021) 9:648828. doi: 10.3389/fped.2021.648828
23. Shennan AT, Dunn MS, Ohlsson A, Lennox K, Hoskins EM. Abnormal pulmonary outcomes in premature infants: prediction from oxygen requirement in the neonatal period. Pediatrics. (1988) 82:527–32. doi: 10.1542/peds.82.4.527
24. Sung SI, Chang YS, Chun JY, Yoon SA, Yoo HS, Ahn SY, et al. Mandatory closure versus nonintervention for patent ductus arteriosus in very preterm infants. J Pediatr. (2016) 177:66–71.e1. doi: 10.1016/j.jpeds.2016.06.046
25. Shane AL, Sánchez PJ, Stoll BJ. Neonatal sepsis. Lancet. (2017) 390:1770–80. doi: 10.1016/S0140-6736(17)31002-4
26. Harel-Gadassi A, Friedlander E, Yaari M, Bar-Oz B, Eventov-Friedman S, Mankuta D, et al. Developmental assessment of preterm infants: chronological or corrected age? Res Dev Disabil. (2018) 80:35–43. doi: 10.1016/j.ridd.2018.06.002
27. Karabacak M, Margetis K. Precision medicine for traumatic cervical spinal cord injuries: accessible and interpretable machine learning models to predict individualized in-hospital outcomes. Spine J. (2023) 23:S1529–9430. doi: 10.1016/j.spinee.2023.08.009
28. Shen Z, Chen H, Wang W, Xu W, Zhou Y, Weng Y, et al. Machine learning algorithms as early diagnostic tools for pancreatic fistula following pancreaticoduodenectomy and guide drain removal: a retrospective cohort study. Int J Surg. (2022) 102:106638. doi: 10.1016/j.ijsu.2022.106638
29. Han H, Oh J. Application of various machine learning techniques to predict obstructive sleep apnea syndrome severity. Sci Rep. (2023) 13:6379. doi: 10.1038/s41598-023-33170-7
30. Vyas-Read S, Logan JW, Cuna AC, Machry J, Leeman KT, Rose RS, et al. A comparison of newer classifications of bronchopulmonary dysplasia: findings from the children’s hospitals neonatal consortium severe BPD group. J Perinatol. (2022) 42:58–64. doi: 10.1038/s41372-021-01178-4
31. Yılmaz C, Köksal N, Özkan H, Dorum BA, Bağcı O. Low serum IGF-1 and increased cytokine levels in tracheal aspirate samples are associated with bronchopulmonary dysplasia. Turk J Pediatr. (2017) 59:122–9. doi: 10.24953/turkjped.2017.02.003
32. Hirani D, Alvira CM, Danopoulos S, Milla C, Donato M, Tian L, et al. Macrophage-derived IL-6 trans-signalling as a novel target in the pathogenesis of bronchopulmonary dysplasia. Eur Respir J. (2022) 59:2002248. doi: 10.1183/13993003.02248-2020
33. Li H, Wang G, Lin S, Wang C, Zha J. Loss of interleukin-6 enhances the inflammatory response associated with hyperoxia-induced lung injury in neonatal mice. Exp Ther Med. (2019) 17:3101–7. doi: 10.3892/etm.2019.7315
34. Zhang Z, Jiang J, Li Z, Wan W. The change of cytokines and gut microbiome in preterm infants for bronchopulmonary dysplasia. Front Microbiol. (2022) 13:804887. doi: 10.3389/fmicb.2022.804887
35. Dai D, Chen H, Dong X, Chen J, Mei M, Lu Y, et al. Bronchopulmonary dysplasia predicted by developing a machine learning model of genetic and clinical information. Front Genet. (2021) 12:689071. doi: 10.3389/fgene.2021.689071
36. Khan S, Concina VA, Schneider D, Westgate P, Arriagada S, Bada H. Role of NT-proBNP in the prediction of moderate to severe bronchopulmonary dysplasia in preterm infants. Pediatr Pulmonol. (2020) 55:376–82. doi: 10.1002/ppul.24610
37. Potsiurko S, Dobryanskyy D, Sekretar L. Patent ductus arteriosus, systemic NT-proBNP concentrations and development of bronchopulmonary dysplasia in very preterm infants: retrospective data analysis from a randomized controlled trial. BMC Pediatr. (2021) 21:286. doi: 10.1186/s12887-021-02750-9
38. Alonso-Ojembarrena A, Méndez-Abad P, Alonso-Quintela P, Zafra-Rodríguez P, Oulego-Erroz I, Lubián-López SP. Lung ultrasound score has better diagnostic ability than NT-proBNP to predict moderate-severe bronchopulmonary dysplasia. Eur J Pediatr. (2022) 181:3013–21. doi: 10.1007/s00431-022-04491-y
Keywords: interleukin-6, bronchopulmonary dysplasia, biomarker, machine learning, umbilical cord blood
Citation: Gao L, Yang P, Luo C, Lei M, Shi Z, Cheng X, Zhang J, Cao W, Ren M, Zhang L, Wang B and Zhang Q (2023) Machine learning predictive models for grading bronchopulmonary dysplasia: umbilical cord blood IL-6 as a biomarker. Front. Pediatr. 11:1301376. doi: 10.3389/fped.2023.1301376
Received: 24 September 2023; Accepted: 27 November 2023;
Published: 15 December 2023.
Edited by:
Shi Yuan, Children’s Hospital of Chongqing Medical University, ChinaReviewed by:
Guang Yue, Chengdu Women’s and Children’s Central Hospital, ChinaFan Wu, Third Affiliated Hospital of Guangzhou Medical University, China
© 2023 Gao, Yang, Luo, Lei, Shi, Cheng, Zhang, Cao, Ren, Zhang, Wang and Zhang. This is an open-access article distributed under the terms of the Creative Commons Attribution License (CC BY). The use, distribution or reproduction in other forums is permitted, provided the original author(s) and the copyright owner(s) are credited and that the original publication in this journal is cited, in accordance with accepted academic practice. No use, distribution or reproduction is permitted which does not comply with these terms.
*Correspondence: Qian Zhang zhangqian629@zzu.edu.cn
†These authors have contributed equally to this work and share first authorship