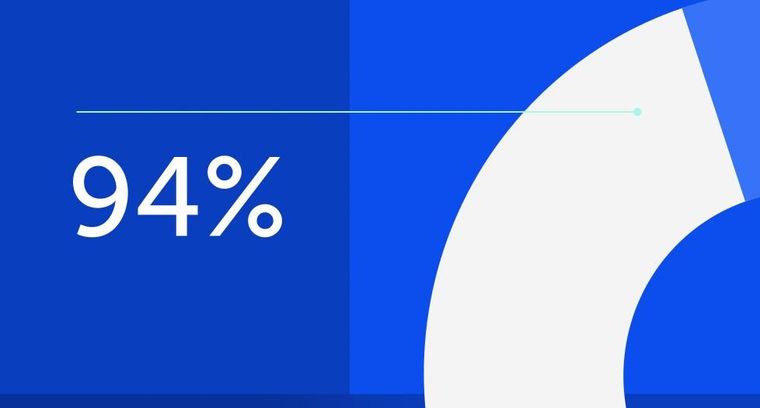
94% of researchers rate our articles as excellent or good
Learn more about the work of our research integrity team to safeguard the quality of each article we publish.
Find out more
ORIGINAL RESEARCH article
Front. Pediatr., 04 October 2023
Sec. Pediatric Infectious Diseases
Volume 11 - 2023 | https://doi.org/10.3389/fped.2023.1233532
This article is part of the Research TopicMethods in Pediatric Infectious Diseases 2022View all 5 articles
Background: Pulse oximeters are not routinely available in outpatient clinics in low- and middle-income countries. We derived clinical scores to identify hypoxemic child pneumonia.
Methods: This was a retrospective pooled analysis of two outpatient datasets of 3–35 month olds with World Health Organization (WHO)-defined pneumonia in Bangladesh and Malawi. We constructed, internally validated, and compared fit & discrimination of four models predicting SpO2 < 93% and <90%: (1) Integrated Management of Childhood Illness guidelines, (2) WHO-composite guidelines, (3) Independent variable least absolute shrinkage and selection operator (LASSO); (4) Composite variable LASSO.
Results: 12,712 observations were included. The independent and composite LASSO models discriminated moderately (both C-statistic 0.77) between children with a SpO2 < 93% and ≥94%; model predictive capacities remained moderate after adjusting for potential overfitting (C-statistic 0.74 and 0.75). The IMCI and WHO-composite models had poorer discrimination (C-statistic 0.56 and 0.68) and identified 20.6% and 56.8% of SpO2 < 93% cases. The highest score stratum of the independent and composite LASSO models identified 46.7% and 49.0% of SpO2 < 93% cases. Both LASSO models had similar performance for a SpO2 < 90%.
Conclusions: In the absence of pulse oximeters, both LASSO models better identified outpatient hypoxemic pneumonia cases than the WHO guidelines. Score external validation and implementation are needed.
The burden of child pneumonia mortality predominantly occurs in low-income and middle-income countries (LMICs) (1). Hypoxemia—a low blood oxyhemoglobin saturation—conveys increased pneumonia mortality risk, yet most children in LMICs lack pulse oximeter access (2, 3). Pulse oximeters identify hypoxemia by non-invasively measuring the peripheral arterial oxyhemoglobin saturation (SpO2) (4). To simplify diagnosis in LMICs the World Health Organization (WHO) Integrated Management of Childhood Illness (IMCI) guidelines consider pneumonia a clinical syndrome (5). Although IMCI recommends oximeter use when available, it also provides guidance for settings without pulse oximetry (5).
There are challenges in the application of the IMCI algorithm for pneumonia management, which have remained largely unchanged since their inception in the mid-1990s. Since then, the guidelines have undergone one technical update that recommends children with chest indrawing but without clinical danger signs no longer require hospital referral (6, 7). Evidence suggests that gaps remain with the IMCI case management strategy, especially regarding use of the algorithm without pulse oximetry. Specifically, research reported IMCI missed ∼70% of outpatient child pneumonia cases with a SpO2 < 90% in Malawi and ∼90% in Bangladesh (8, 9). However, a stronger emphasis on the integration of pulse oximetry into IMCI guidance—while an important next step—is unlikely to immediately solve this issue as healthcare providers in most high pneumonia burden LMICs lack access to pulse oximeter devices in outpatient settings where children usually present to care first. High quality, affordable devices designed for the needs of infants and children in LMICs are not yet available. As a result, healthcare providers instead rely on clinical signs included in the IMCI guidelines to aid in the management of pneumonia cases. As most children first access health systems at outpatient clinics, improving outpatient hypoxemia identification may be key to reducing LMIC pneumonia mortality (4).
Prior research attempted to determine whether clinical signs accurately identify a SpO2 < 90% in hospitalized children as current guidelines limit severe case definitions to SpO2 measurements <90% (10, 11). While these hospital-based studies report high specificity of clinical signs for a SpO2 < 90%, sensitivity was low (10, 11). Similar studies in outpatient contexts are lacking, and may reveal new evidence than research from hospitals relying on study populations of more severely ill children not representative of outpatient settings. In settings where pulse oximeters are available, recent data also showed elevated mortality among children with a SpO2 90%–92% or 93%, as compared to higher SpO2 levels (8, 12–14). These findings further challenge the current guidelines, which recommend SpO2 < 90% threshold for hospitalization and oxygen treatment in LMICs.
Given the need for healthcare providers in low-resource settings to rely mainly on IMCI-derived clinical signs for outpatient child pneumonia management, we sought to utilize two unique, contemporary outpatient pediatric pneumonia datasets from Bangladesh and Malawi to accomplish three objectives (15, 16). We first sought to evaluate the performance of IMCI clinical signs for identifying hypoxemia at a higher SpO2 threshold (<93%) than recommended. Second, we examined whether other combinations of clinical features not included in IMCI guidelines better identify children with a SpO2 < 93%, followed by development and internal validation of pragmatic hypoxemia clinical scores that could be implemented where pulse oximeters remain unavailable. Third, we repeated these analyses using the currently recommended SpO2 < 90% threshold.
We used data from Malawi and Bangladesh. Malawi is an African country with an under 5 mortality rate of 49/1,000 births (17). This study included 18 clinics in Mchinji and Lilongwe districts with a 1.2 million population catchment area, at 1,000–1,100 m altitude (16). From October 2011 to June 2014, non-physician clinicians and nurses at clinics were IMCI trained and documented care of 0–59 month olds with WHO-defined pneumonia (16). Providers used Acare® pulse oximeters with adult clip probes on the big toe if <2 years old or weighing <10 kg (9). Training, data collection, and supervision methodology has been published (16).
Bangladesh has an under 5 mortality rate of 27.3/1,000 live births (18). Since 2001, Projahnmo, a partnership of Johns Hopkins University with the Government of Bangladesh's Ministry of Health and Family Welfare, International Centre for Diarrhoeal Disease Research, Bangladesh, Shimantik, and the Child Health Research Foundation conducted community-based surveillance in Zakiganj subdistrict of Sylhet district in Bangladesh (15). From May 2015 to September 2017 Projahnmo expanded surveillance into two additional subdistricts (Kanaighat, Beanibazar) and augmented IMCI clinic care of three government Upazila Health Complexes (15). Altogether these subdistricts have a 770,000 population at 17–23 m altitude. Upazila Health Complexes provide outpatient and emergency care and limited inpatient pediatric services. IMCI clinic care was provided by Projahnmo physicians per IMCI guidelines (15). From October 2017 Projahnmo physicians measured the SpO2 of 3–35 month olds with suspected pneumonia using a Masimo Rad-5® pulse oximeter with a LNCS® Y-I wrap sensor on the big toe. Parent study methodology is published (15).
We generated an analytic sample of healthcare visits from Bangladesh and Malawi datasets. Inclusion criteria were: valid SpO2, IMCI-defined non-severe or severe pneumonia (2014 guidelines), (5) and age 3–35 months, as the Bangladesh study population with a SpO2 was limited to this age (19). See Supplementary Material for study definitions. Implicit to this analysis we assumed SpO2 was unavailable and excluded it from pneumonia definitions.
Our primary outcome was a SpO2 < 93%; SpO2 < 90% was secondary. We explored associations between clinical variables and SpO2 ranges to evaluate <93% as the primary outcome. We selected variables a priori: sex, age, weight-for-age z-score (WAZ), chest indrawing, wheezing, severe respiratory distress (grunting, head nodding, nasal flaring, and/or severe fast breathing), cyanosis, fever (temperature ≥38 °C), and WHO-defined general danger signs (stridor, inability to feed/drink, convulsions, and/or lethargy) (8, 18). Severe fast breathing was defined as follows: respiratory rate ≥60 breaths/min for 3–11 month olds, ≥50 breaths/min for 12–59 month olds (20).
We evaluated missingness using a 5% threshold. We used Chi-squared and Fisher's exact tests for proportions, Wilcoxon rank-sum for non-parametric data, and Student's t-test for normally distributed data comparisons. We reviewed individual-level variables and their associated SpO2 < 93% and <90% predictive quality. For SpO2 < 93% and <90% model development we randomly split the dataset into derivation (70%) and validation (30%) sets balanced by outcome and country. For each model we fit a logistic regression model with hypoxemia as the binary outcome measure. We allowed selection of country as a fixed effect to account for significant differences by country. Thereafter, we used a random intercept in each post selection model to control for country after interrogating the suitability of this approach with the Hausman test (21). All analyses were by Stata 16.1 (StataCorp, College Station, TX).
The IMCI model reflects 2014 IMCI referral criteria (Supplementary Material) (5). The WHO-composite model is a composite of four WHO guidelines (5, 22–24). We fit both models using the logistic command for implementing multivariable, maximum-likelihood logit models to obtain odds ratios (and 95% confidence intervals) comparing the odds of hypoxemia vs. non-hypoxemia.
We used the least absolute shrinkage and selection operator (LASSO) reduction method, testing two selection modes [(1) 10-fold cross-validation selection and (2) adaptive selection] using the derivation dataset to develop both models from an expanded variable list of the IMCI and WHO-composite models (Supplementary Material) (25, 26). For Independent LASSO we used singular variables (i.e., independent) whereas for Composite LASSO we used composite variables for “danger signs” (WHO-defined general danger signs) and “severe respiratory distress.” For both models we compared the two methods using the C-statistic based on predicted estimates, sensitivity, and specificity. If we found no statistical difference between methods, we used the selection results from the simplest model to implement an unsupervised approach (i.e., selection of the full variable rather than one category of a three-category variable) to refit both models.
We compared discriminatory power and model fit [C-statistic and Bayesian Information Criteria (BIC)] of the four maximum-likelihood logit models using the derivation dataset models (27, 28). Using an unsupervised approach (i.e., if only two age groups were selected, we retained all age categories), each of the LASSO model covariates were kept on the log scale, rounded to the nearest 0.5, and doubled to form an integer (14, 29, 30). We then split the score into approximately equally sized quintiles to create hypoxemia risk categories.
Using the validation dataset, LASSO model scores were estimated by child, and score discriminatory power to identify children with and without hypoxemia was determined. Scores were not developed using IMCI and WHO-composite models. The C-statistic, sensitivity, specificity, positive and negative predictive value (PPV and NPV), and positive and negative likelihood ratios (LR+ and LR−) were compared across all models. We adjusted C-statistics for optimism by bootstrapping (200 repetitions) to account for any overfitting (31). A C-statistic 0.71–0.80 was considered moderate and >0.80 as excellent discriminatory power (32). We applied the same analysis methodology for SpO2 < 90%.
We included 12,712 pneumonia cases; 63.6% (n = 8,081) were from Bangladesh (Supplementary Material). Table 1 shows all participant characteristics by SpO2. A SpO2 < 93% was in 10.4% (1,328/12,712) of cases and 63.6% (845/1,328) of SpO2 < 93% cases were in Malawi. Most SpO2 < 93% cases had non-severe pneumonia (1,065/1,328; 81.4%) and, without a SpO2 measurement, were hospitalization ineligible per IMCI guidelines. A larger proportion of severe (263/1,198, 21.9%) than non-severe (1,065/11,514, 9.2%) cases had a SpO2 < 93%. A SpO2 < 90% was in 4.6% (602/12,712) of cases and in 3.8% (434/11,514) with non-severe disease. 2014 IMCI hospital referral criteria missed 72.0% (434/602) of SpO2 < 90% cases. While Bangladesh and Malawi case characteristics differed in frequency, other than WAZ < −3 and danger signs, they had similar crude associations with a SpO2 < 93% (Supplementary Material).
We assessed the relationship between referral criteria and hypoxemia at SpO2 < 90%, 90%–92%, and <93% for the IMCI and WHO-composite models (Table 2). WAZ ≤ −3, in both models, was associated with a SpO2 90%–92% and <93% but not <90%. In the WHO-composite model severe respiratory distress was associated with an increased adjusted odds of an abnormal SpO2 regardless of SpO2 range. While in the IMCI model danger signs were associated with hypoxemia, including respiratory distress in the WHO-composite model attenuated its effect at each SpO2 threshold. Respiratory distress was associated with each SpO2 threshold in the WHO-composite model.
Table 2. Association of IMCI guideline and WHO-composite models with SpO2 ranges (full dataset, N = 12,712).
Derivation (n = 3,813) and validation (n = 8,899) datasets have similar patient characteristic distributions (Supplementary Material, all p > 0.05). Table 3 presents adjusted odds ratios (aORs) for SpO2 < 93% using model-specific predictors from the derivation dataset. In the independent LASSO model, individual predictor scores ranged from −1 to 3. The composite LASSO model scores ranged from −1 to 4. Overall predictive performance of the independent (C-statistic = 0.774) and composite LASSO models (C-statistic = 0.773) was moderate (Table 3) and did not differ in discriminatory power (p = 0.480), with total score ranges in the Supplementary Material. Model fit improved from the IMCI (BIC 5,536.5) and WHO-composite models (BIC 5,155.9) to the independent (BIC 4,650.6) and composite LASSO models (BIC 4,712.2). Overall, the independent (C-statistic = 0.774) and composite LASSO models (C-statistic = 0.773) better discriminated between children with and without a SpO2 < 93% than the IMCI (C-statistic = 0.661) and WHO-composite models (C-statistic = 0.734). SpO2 < 90% score development and cross-model comparison are in the Supplementary Material.
In the validation dataset, independent (C-statistic = 0.745) and composite LASSO model (C-statistic = 0.752) scores moderately discriminated between SpO2 < 93% and ≥94% cases (Figure 1). When examining the C-statistics adjusted for optimism, there was minimal C-statistic change for both scores (Table 4), and independent and composite LASSO model discriminatory power did not differ (p = 0.480). For the independent and composite LASSO models about 4% of children with a score in the first stratum had SpO2 < 93% compared to >35% in the last stratum (Table 4).
Figure 1. Comparison of ROC curves for identifying SpO2 < 93% cases (validation dataset). ROC, receiver operating characteristic curve; SpO2, peripheral arterial oxyhemoglobin saturation; IMCI, integrated management of childhood illnesses; WHO, World Health Organization; LASSO, least absolute shrinkage and selection operator reduction method.
Among IMCI and WHO-composite model classified cases, 23% and 24% had a SpO2 < 93%, while among non-cases 9% and 6% had a SpO2 < 93% (Table 4). The ability to discriminate between SpO2 < 93% and ≥94% cases using the IMCI (C-statistic = 0.563) and WHO-composite model (C-statistic = 0.677) criteria was low. Based on model fit and discrimination, the composite LASSO model was the most predictive of SpO2 < 93%, identifying 49.0% of SpO2 < 93% cases. The independent LASSO model performed similarly, followed by WHO-composite and IMCI models. SpO2 < 90% results on score validation and ability to discriminate hypoxemia from non-hypoxemia cases are in the Supplementary Material.
The Supplementary Material includes the diagnostic performance of individual clinical signs for a SpO2 < 93% and <90% using the validation datasets.
Using pooled data from 12,712 IMCI-defined child pneumonia cases evaluated at 21 clinics in Malawi and Bangladesh we examined WHO IMCI guideline hypoxemia identification performance and developed and internally validated clinical hypoxemia scores for use in LMICs where pulse oximeters suitable for pediatric outpatient care are scarce. Our findings suggest more hypoxemic children could be identified during outpatient care lacking pulse oximeters if additional signs of respiratory distress are incorporated into IMCI. Notably, >80% of hypoxemic cases with a SpO2 < 93% and >70% with a SpO2 < 90% were misclassified by IMCI as ineligible for hospital referral when pulse oximeters are unavailable. The independent LASSO model added age, severe respiratory distress, chest indrawing, cyanosis, fever, and wheezing parameters into the base IMCI guideline model and better identified children with a SpO2 < 93% than the IMCI and WHO-composite models. The composite LASSO model's simplified composite variables may facilitate implementation. Both LASSO models also achieved excellent discrimination of SpO2 < 90% cases.
Unlike other studies evaluating hypoxemia predictors we focused on ambulatory rather than hospital settings. This distinction is important as our findings should therefore be generalizable to outpatient settings without oximeters and with lower hypoxemia prevalence than hospitals (33, 34). Notably, individual variable sensitivity and specificity for hypoxemia are largely similar to hospital-based studies (11). However, our WHO-composite model and two LASSO models have good to excellent discriminatory values distinguishing between hypoxemic and non-hypoxemic pneumonia cases, contrasting to other work with smaller samples that limited analyses (11). An exception is a large, multi-center, hospital-based Nigerian study that found a combination of respiratory distress, inability to feed, cyanosis, lethargy and severe tachypnea had lower discrimination (C-statistic = 0.655) for SpO2 < 90% amongst respiratory and non-respiratory cases than in our data (10). The lower C-statistic may reflect the authors inclusion of older children <15 years.
It is also important our results are interpreted within the broader child pneumonia context of known mortality risk factors. In the IMCI and WHO-composite models WAZ ≤ −3 was not associated with a SpO2 < 90%, yet is a known mortality risk factor (14, 35). Conversely wheezing was retained in both LASSO models but, when identified alone without accompanying respiratory distress, it is not associated with radiographic pneumonia or in-hospital mortality (Supplementary Material) (14, 36). Isolated wheeze usually reflects milder, self-limited viral illness (e.g., bronchiolitis) and is susceptible to non-differential misclassification when confused with transmitted upper respiratory sounds.
While our pooling of data from studies in South Asia and Africa aimed to improve generalizability, there are several differences between the settings and studies that may be limitations. Malawi data had a higher frequency of hypoxemia, danger signs, and respiratory distress than Bangladesh data. Alternatively, WAZ ≤ −3 was more frequent in Bangladesh than Malawi. These differences, in part, may reflect higher HIV and malaria prevalence or modestly higher altitude in Malawi. These diseases increase susceptibility for hypoxemia or, for malaria, increase the frequency of signs overlapping with IMCI pneumonia (37). Although the designs differed, all personnel were similarly trained per IMCI and followed similar data collection procedures. Two different pulse oximeter devices were used in each study. In Malawi, healthcare workers used Acare pulse oximeters with the adult clip probes while in Bangladesh study staff used Masimo Rad5 pulse oximeters with wrap sensors. Although the devices differed, healthcare workers followed similar measurement procedures that relied on SpO2 measurement of the child's big toe, which mitigated probe fit issues with the Acare device. Additionally, while pulse oximeter manufacturer algorithms for SpO2 calculation may differ, we do not expect systematic, clinically relevant differences in population-level SpO2 summary statistics between these devices as both were used similarly on patients by frequently supervised healthcare workers, met ISO 80601-2-61:2017 requirements for the basic safety and essential accuracy performance of pulse oximeters during laboratory testing, are CE marked, and commercially available. Lastly, the under 5 mortality rate in Malawi is higher than Bangladesh, (17, 18) which aligns with our results suggesting Malawi cases were more severe. Nevertheless, we attempted to account for unmeasured confounders from epidemiological, health system, and methodological differences by fitting models with a country variable.
We recognize that the future use of a clinical hypoxemia score would require further external validation and evaluation of implementation acceptability, feasibility, fidelity, and other early implementation indicators. Broader use of such clinical scores largely depend on health system capacity—for example, developing and maintaining well-trained clinical staff who can recognize the clinical features that comprise the score and who accept new guidelines, local expertise for overseeing and advising any adapted implementation, and, most importantly, resources such as oxygen and antibiotics to act on these symptoms and improve care with resultant improved outcomes. To optimize implementation further studies are needed to examine and adapt to changes to clinical procedure flows as well as assessment and adaptation of health system dynamics that include the capacity to handle additional referrals. These steps should be conducted within the context of strengthening the outpatient system for subsequent pulse oximeter implementation when appropriate, high quality devices for infants and children become accessible (38).
In sum, these findings may improve hypoxemic pneumonia identification in clinics either without pulse oximetry at all or lacking pulse oximeters suitable for pediatric use. Given a SpO2 < 93% is highly associated with mortality, (3, 12, 14) earlier hypoxemic case identification with successful referral may reduce fatality. While these models could advance care for hypoxemic children they are an inadequate substitution for pulse oximeters. Pulse oximetry scale up in ambulatory settings must be prioritized, as should pulse oximeter device development targeted for young children in LMICs. Where pulse oximetry is unavailable for children, our models suggest children with chest indrawing and/or with other signs of respiratory distress could be referred. To successfully implement these models ambulatory health workers of varying pediatric experience will need further training to recognize signs of respiratory distress in children. Understanding how these changes may affect referral quality, uptake, and hospitalizations is critical. Next steps include external validation and research evaluating implementation feasibility of the hypoxemia scores.
The datasets presented in this article are not readily available because of country limits on data availability. Requested to access the datasets should be directed to EM,ZW1jY29sbDNAamhtaS5lZHU=.
The studies involving humans were approved by the National Health Sciences Research Committee of Malawi, the Ethics Committee of University College London, and The Bangladesh Medical Research Counsel, International Centre for Diarrhoeal Diseases Research, Johns Hopkins Bloomberg School of Public Health and School of Medicine Institutional Review Boards. Caregiver written consent was required in Bangladesh but not Malawi. The studies were conducted in accordance with the local legislation and institutional requirements. Written informed consent for participation in this study was provided by the participants’ legal guardians/next of kin.
EM had full access to data in the study and takes full responsibility for the integrity of the data and the accuracy of the data analysis. EM conceived the study idea and HS, SH, and EM designed the study; HS analyzed the data; SH, HS, and EM drafted and revised the manuscript. All authors contributed to the article and approved the submitted version.
We offer our thanks to all of the caregivers and children participating in this research in Bangladesh and Malawi. In Bangladesh we would like to thank the Projahnmo Study Group field and data management staff, the Ministry of Health and Family Welfare, Government of Bangladesh. In Malawi we would like to thank Mr. Charles Makwenda and Rashid Deula for leading efforts in data collection and curation of the original study, the Republic of Malawi Ministry of Health and the communities and traditional authorities of Lilongwe and Mchinji districts of Malawi, and the dedication and hard work of our field and data collection staff. Lastly, we thank GlaxoSmithKline, Bill & Melinda Gates Foundation, and the National Institute of Health their support of this study.
The authors declare that the research was conducted in the absence of any commercial or financial relationships that could be construed as a potential conflict of interest.
All claims expressed in this article are solely those of the authors and do not necessarily represent those of their affiliated organizations, or those of the publisher, the editors and the reviewers. Any product that may be evaluated in this article, or claim that may be made by its manufacturer, is not guaranteed or endorsed by the publisher.
The Supplementary Material for this article can be found online at: https://www.frontiersin.org/articles/10.3389/fped.2023.1233532/full#supplementary-material
1. GBD 2016 Lower Respiratory Infections Collaborators. Estimates of the global, regional, and national morbidity, mortality, and aetiologies of lower respiratory infections in 195 countries, 1990–2016: a systematic analysis for the global burden of disease study 2016. Lancet Infect Dis. (2018) 18(11):1191–210. doi: 10.1016/S1473-3099(18)30310-4
2. Ginsburg AS, Van Cleve WC, Thompson MIW, English M. Oxygen and pulse oximetry in childhood pneumonia: a survey of healthcare providers in resource-limited settings. J Trop Pediatr. (2012) 58(5):389–93. doi: 10.1093/tropej/fmr103
3. Lazzerini M, Sonego M, Pellegrin MC. Hypoxaemia as a mortality risk factor in acute lower respiratory infections in children in low and middle-income countries: systematic review and meta-analysis. PLoS One. (2015) 10(9):e0136166. doi: 10.1371/journal.pone.0136166
4. McCollum ED, King C, Colbourn T, Graham H, Bernstein M, Wilson IH, et al. Pulse oximetry in paediatric primary care in low-income and middle-income countries. Lancet Respir Med. (2019) 7(12):1001–2. doi: 10.1016/S2213-2600(19)30358-3
5. World Health Organization. Integrated management of childhood illness (IMCI): Chart booklet. 2nd ed Geneva, Switzerland: WHO Press (2014). 80.
6. Simon JL, Daelmans B, Boschi-Pinto C, Aboubaker S, Were W. Child health guidelines in the era of sustainable development goals. BMJ. (2018) 362:bmj.k3151. doi: 10.1136/bmj.k3151
7. Mulholland K. Problems with the WHO guidelines for management of childhood pneumonia. Lancet Glob Health. (2018) 6(1):e8–9. doi: 10.1016/S2214-109X(17)30468-0
8. McCollum ED, Ahmed S, Roy AD, Islam AA, Schuh HB, King C, et al. Risk and accuracy of outpatient-identified hypoxaemia for death among suspected child pneumonia cases in rural Bangladesh: a multifacility prospective cohort study. Lancet Respir Med. (2023) 11(9):769–81. S2213-2600(23)00098-X 37037207
9. McCollum ED, King C, Deula R, Zadutsa B, Mankhambo L, Nambiar B, et al. Pulse oximetry for children with pneumonia treated as outpatients in rural Malawi. Bull World Health Organ. (2016) 94(12):893–902. doi: 10.2471/BLT.16.173401
10. Graham H, Bakare AA, Ayede AI, Oyewole OB, Gray A, Peel D, et al. Hypoxaemia in hospitalised children and neonates: a prospective cohort study in Nigerian secondary-level hospitals. EClinicalMedicine. (2019) 16:51–63. doi: 10.1016/j.eclinm.2019.10.009
11. Zhang L, Mendoza-Sassi R, Santos JCH, Lau J. Accuracy of symptoms and signs in predicting hypoxaemia among young children with acute respiratory infection: a meta-analysis [review article]. Int J Tuberc Lung Dis. (2011) 15(3):317–25.21333097
12. Colbourn T, King C, Beard J, Phiri T, Mdala M, Zadutsa B, et al. Predictive value of pulse oximetry for mortality in infants and children presenting to primary care with clinical pneumonia in rural Malawi: a data linkage study. PLoS Med. (2020) 17(10):e1003300. doi: 10.1371/journal.pmed.1003300
13. Hooli S, King C, Zadutsa B, Nambiar B, Makwenda C, Masache G, et al. The epidemiology of hypoxemic pneumonia among young infants in Malawi. Am J Trop Med Hyg. (2020) 102(3):676–83. doi: 10.4269/ajtmh.19-0516
14. Hooli S, Colbourn T, Lufesi N, Costello A, Nambiar B, Thammasitboon S, et al. Predicting hospitalised paediatric pneumonia mortality risk: an external validation of RISC and mRISC, and local tool development (RISC-Malawi) from Malawi. PLoS One. (2016) 11(12):e0168126. doi: 10.1371/journal.pone.0168126
15. Baqui AH, McCollum ED, Saha SK, Roy AK, Chowdhury NH, Harrison M, et al. Pneumococcal conjugate vaccine impact assessment in Bangladesh. Gates Open Res. (2018) 2:21. doi: 10.12688/gatesopenres.12805.1
16. McCollum ED, Nambiar B, Deula R, Zadutsa B, Bondo A, King C, et al. Impact of the 13-valent pneumococcal conjugate vaccine on clinical and hypoxemic childhood pneumonia over three years in central Malawi: an observational study. PLoS One. (2017) 12(1):e0168209. doi: 10.1371/journal.pone.0168209
17. UNICEF DATA. Malawi (MWI)—demographics, health & infant mortality. Available at: https://data.unicef.org/country/mwi/ (Cited Jun 24, 2020).
18. UNICEF DATA. Bangladesh (BGD)—demographics, health & infant mortality. Available at: https://data.unicef.org/country/bgd/ (Cited Jun 19, 2020).
19. McCollum ED, Ahmed S, Roy AD, Chowdhury NH, Schuh HB, Rizvi SJR, et al. Effectiveness of the 10-valent pneumococcal conjugate vaccine against radiographic pneumonia among children in rural Bangladesh: a case-control study. Vaccine. (2020) 38(42):6508–16. doi: 10.1016/j.vaccine.2020.08.035
20. Fox MP, Thea DM, Sadruddin S, Bari A, Bonawitz R, Hazir T, et al. Low rates of treatment failure in children aged 2-59 months treated for severe pneumonia: a multisite pooled analysis. Clin Infect Dis. (2013) 56(7):978–87. doi: 10.1093/cid/cis1201
21. McNeish D, Kelley K. Fixed effects models versus mixed effects models for clustered data: reviewing the approaches, disentangling the differences, and making recommendations. Psychol Methods. (2019) 24(1):20–35. doi: 10.1037/met0000182
22. World Health Organization. Oxygen therapy for children: A manual for health workers. Geneva, Switzerland: WHO Press (2016). p. 57. Available at: https://apps.who.int/iris/handle/10665/204584 (Cited Dec 20, 2021).
23. World Health Organization, Department of Child and Adolescent Health and Development. Emergency triage assessment and treatment (ETAT): Facilitator guide. Geneva, Switzerland: World Health Organization, Dept. of Child and Adolescent Health and Development (2005). Available at: http://site.ebrary.com/id/10277787 (Cited Jul 13, 2018).
24. World Health Organization. Pocket book of hospital care for children: Guidelines for the management of common illnesses with limited resources. 1st ed. Geneva, Switzerland: WHO Press (2005). p. 365. Available at: http://apps.who.int/iris/bitstream/handle/10665/43206/9241546700.pdf?sequence=1
25. Zou H. The adaptive LASSO and its oracle properties. J Am Stat Assoc. (2006) 101(476):1418–29. doi: 10.1198/016214506000000735
26. Tibshirani R. Regression shrinkage and selection via the LASSO: a retrospective. J R Stat Soc Series B Stat Methodol. (2011) 73(3):273–82. doi: 10.1111/j.1467-9868.2011.00771.x
27. Neath AA, Cavanaugh JE. The Bayesian information criterion: background, derivation, and applications. WIRES Comput Stat. (2012) 4(2):199–203. doi: 10.1002/wics.199
28. Schwarz G. Estimating the dimension of a model. Ann Stat. (1978) 6(2):461–4. doi: 10.1214/aos/1176344136
29. Le Gall JR, Lemeshow S, Saulnier F. A new simplified acute physiology score (SAPS II) based on a European/North American multicenter study. JAMA. (1993) 270(24):2957–63. doi: 10.1001/jama.1993.03510240069035
30. Harrell FE, Lee KL, Mark DB. Multivariable prognostic models: issues in developing models, evaluating assumptions and adequacy, and measuring and reducing errors. Stat Med. (1996) 15(4):361–87. doi: 10.1002/(SICI)1097-0258(19960229)15:4%3C361::AID-SIM168%3E3.0.CO;2-4
31. Smith GCS, Seaman SR, Wood AM, Royston P, White IR. Correcting for optimistic prediction in small data sets. Am J Epidemiol. (2014) 180(3):318–24. doi: 10.1093/aje/kwu140
32. Austin PC, Steyerberg EW. Interpreting the concordance statistic of a logistic regression model: relation to the variance and odds ratio of a continuous explanatory variable. BMC Med Res Methodol. (2012) 12:82. doi: 10.1186/1471-2288-12-82
33. Rahman AE, Hossain AT, Nair H, Chisti MJ, Dockrell D, Arifeen SE, et al. Prevalence of hypoxaemia in children with pneumonia in low-income and middle-income countries: a systematic review and meta-analysis. Lancet Glob Health. (2022) 10(3):e348–59. doi: 10.1016/S2214-109X(21)00586-6
34. Subhi R, Adamson M, Campbell H, Weber M, Smith K, Duke T, et al. The prevalence of hypoxaemia among ill children in developing countries: a systematic review. Lancet Infect Dis. (2009) 9(4):219–27. doi: 10.1016/S1473-3099(09)70071-4
35. Deardorff KV, McCollum ED, Ginsburg AS. Pneumonia risk stratification scores for children in low-resource settings: a systematic literature review. Pediatr Infect Dis J. (2018) 37(8):743–8. doi: 10.1097/INF.0000000000001883
36. McCollum ED, Park DE, Watson NL, Fancourt NSS, Focht C, Baggett HC, et al. Digital auscultation in PERCH: associations with chest radiography and pneumonia mortality in children. Pediatr Pulmonol. (2020) 55(11):3197–208. doi: 10.1002/ppul.25046
37. Källander K, Nsungwa-Sabiiti J, Peterson S. Symptom overlap for malaria and pneumonia–policy implications for home management strategies. Acta Trop. (2004) 90(2):211–4. doi: 10.1016/j.actatropica.2003.11.013
38. Rahman AE, Mhajabin S, Dockrell D, Nair H, El Arifeen S, Campbell H. Correction to: managing pneumonia through facility-based integrated management of childhood management (IMCI) services: an analysis of the service availability and readiness among public health facilities in Bangladesh. BMC Health Serv Res. (2021) 21(1):872. doi: 10.1186/s12913-021-06898-z
Keywords: hypoxia, clinical decision rules, pediatrics, primary health care, low-income countries, respiratory tract infection (RTI)
Citation: Schuh HB, Hooli S, Ahmed S, King C, Roy AD, Lufesi N, Islam AA, Mvalo T, Chowdhury NH, Ginsburg AS, Colbourn T, Checkley W, Baqui AH and McCollum ED (2023) Clinical hypoxemia score for outpatient child pneumonia care lacking pulse oximetry in Africa and South Asia. Front. Pediatr. 11:1233532. doi: 10.3389/fped.2023.1233532
Received: 2 June 2023; Accepted: 7 September 2023;
Published: 4 October 2023.
Edited by:
Zhongjie Shi, Wayne State University, United StatesReviewed by:
Rashmi Ranjan Das, All India Institute of Medical Sciences Bhubaneswar, India© 2023 Schuh, Hooli, Ahmed, King, Roy, Lufesi, Islam, Mvalo, Chowdhury, Ginsburg, Colbourn, Checkley, Baqui and McCollum. This is an open-access article distributed under the terms of the Creative Commons Attribution License (CC BY). The use, distribution or reproduction in other forums is permitted, provided the original author(s) and the copyright owner(s) are credited and that the original publication in this journal is cited, in accordance with accepted academic practice. No use, distribution or reproduction is permitted which does not comply with these terms.
*Correspondence: Eric D. McCollum ZW1jY29sbDNAamhtaS5lZHU=
†These authors have contributed equally to this work and share first authorship
Disclaimer: All claims expressed in this article are solely those of the authors and do not necessarily represent those of their affiliated organizations, or those of the publisher, the editors and the reviewers. Any product that may be evaluated in this article or claim that may be made by its manufacturer is not guaranteed or endorsed by the publisher.
Research integrity at Frontiers
Learn more about the work of our research integrity team to safeguard the quality of each article we publish.