- 1School of Medicine, Jishou University, Jishou, China
- 2Department for Infectious Disease Control and Prevention, Xiangxi Center for Disease Control and Prevention, Jishou, China
Background: Childhood obesity (CO) is an increasing public health issue. Mounting evidence has shown that gut microbiota (GM) is closely related to CO. However, the causal association needs to be treated with caution due to confounding factors and reverse causation.
Methods: Data were obtained from the Microbiome Genome Consortium for GM as well as the Early Growth Genetics Consortium for childhood obesity and childhood body mass index (CBMI). Inverse variance weighted, maximum likelihood, weighted median, and MR.RAPS methods were applied to examine the causal association. Then replication dataset was used to validate the results and reverse Mendelian randomization analysis was performed to confirm the causal direction. Additionally, sensitivity analyses including Cochran's Q statistics, MR-Egger intercept, MR-PRESSO global test, and the leave-one-out analysis were conducted to detect the potential heterogeneity and horizontal pleiotropy.
Results: Our study found suggestive causal relationships between eight bacterial genera and the risk of childhood obesity (five for CO and four for CBMI). After validating the results in the replication dataset, we finally identified three childhood obesity-related GM including the genera Akkermansia, Intestinibacter, and Butyricimonas. Amongst these, the genus Akkermansia was both negatively associated with the risk of CO (OR = 0.574; 95% CI: 0.417, 0.789) and CBMI (β = −0.172; 95% CI: −0.306, −0.039).
Conclusions: In this study, we employed the MR approach to investigate the causal relationship between GM and CO, and discovered that the genus Akkermansia has a protective effect on both childhood obesity and BMI. Our findings may provide a potential strategy for preventing and intervening in CO, while also offering novel insights into the pathogenesis of CO from the perspective of GM.
Introduction
Currently, childhood obesity has become an increasing public health issue throughout the world (1). The global prevalence of obesity has doubled over the past three decades, affecting over 340 million children (2, 3). Previous studies have demonstrated that childhood obesity is associated with the early onset and development of various chronic diseases, such as cardiovascular disease, asthma, and metabolic syndrome (4, 5). Additionally, there is a significant increase in the risk of mental disorders and mortality in adulthood (6). Despite years of investigation and intervention, the underlying mechanism of childhood obesity (CO) has not yet been fully clarified, and its prevalence continues to rise dramatically.
Gut microbiota (GM) plays a vital role in preserving host physiology and homeostasis. Accumulating evidence has demonstrated a strong association between gut microbiota dysbiosis and two forms of obesity: non-disease-induced obesity and genetic obesity (7–9). However, the majority of human-based studies were designed as observational in nature, while others utilized animal models, resulting in diverse findings across studies. In contrast to previous findings, Schwiertz et al. demonstrated a lower Firmicutes/Bacteroidetes ratio in individuals with obesity (10). Andoh et al. identified Coprococcus as a risk factor for obesity (11), while Escobar et al. reported the opposite outcome (12). The observational study design posed challenges in establishing the temporal relationship between exposure and outcome, potentially resulting in reverse causation. Moreover, the results were influenced by confounding factors, including age, dietary patterns, and lifestyle. Lastly, previous research has primarily focused on adults with obesity, while studies involving children are relatively limited. Therefore, caution should be treated when interpreting the association between gut microbiota and childhood obesity.
Mendelian randomization (MR) analysis, which uses genetic variants as instrumental variables (IVs), is a powerful approach to identify and quantify the causal effect of exposure on outcome (13). MR is regarded as the “most natural” randomized controlled trial (RCT) because the alleles from parents to offspring are randomly assigned, freely combined with genotypes remaining stable after birth (14). Its advantages, such as confounding factor minimization and exclusion of reverse causality, make it a valuable tool for causal inference in observational studies. Therefore, our study performed a two-sample bidirectional MR analysis using the genome wide association study (GWAS) summary statistics to explore the causal relationship between GM and CO, which may offer an effective strategy for CO prevention and intervention as well as novel insights to understand the pathogenesis of CO from the perspective of GM.
Materials and methods
Data source
The GM dataset was conducted by the Microbiome Genome (MiBioGen) Consortium including 18,340 trans-ancestral subjects (15). After extracting DNA from fecal samples, data were generated by 16S rRNA gene sequencing in the Illumina platform, targeting variable regions of V1–V2, V3–V4, and V4. Setting SILVA as the reference, all the data were annotated to genus and higher levels to profile the microbial composition (16).
GWAS summary statistics for outcomes were extracted from the Early Growth Genetics (EGG) Consortium including childhood obesity and childhood body mass index (BMI). Specifically, CO cases were defined as children whose BMI-for-age were ≥95th percentile at any time from 2 to 18 years old, while controls were individuals whose BMI-for-age were less than the 50th percentile consistently throughout childhood for all available measures. The growth chart and the criteria of childhood obesity was based on the Centers for Disease Control and Prevention in the United States (17). The discovery dataset was collected from a pooled dataset of 30 multiple ancestry cohorts including 28,604 subjects (13,005 cases and 15,599 controls) (18). The replication dataset was obtained from a meta-analysis of 14 studies consisting of 13,848 subjects (5,530 cases and 8,318 controls) (19). For childhood BMI, the phenotype analyzed in both discovery and replication dataset was BMI at the latest time point during childhood, transformed into sex- and age-adjusted standard deviation scores. The discovery dataset consisted of a GWAS meta-analysis of 26 studies, encompassing 39,620 children aged 2–10 years old (20). The replication dataset comprised another GWAS meta-analysis of 20 studies, involving 35,668 children aged 3–10 years old (21). Detailed information on exposure and outcome GWAS datasets was summarized in Supplementary Table S1.
Instrumental variables
To satisfy the three key assumptions of MR analysis (Figure 1), five steps were applied to select the optimal IVs: (1) SNPs under a locus-wide significance threshold of P < 1 × 10−05 were obtained as candidate IVs related to GM (22). (2) PLINK clumping method (r2 < 0.001, clump window <10,000 kb) was performed to ensure the IVs were independent (23). (3) SNPs that are palindromic and those with minor allele frequencies below 0.01 were eliminated. (4) The proxy SNPs (r2 > 0.8) were selected based on 1,000 Genome project's European population after removing the SNPs closely related to the outcome phenotype (P < 5 × 10−08) (24). (5) SNPs with F-statistics < 10 were excluded to avoid weak IV bias (25).
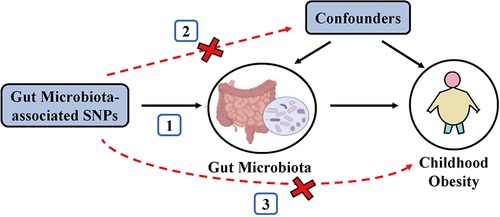
Figure 1. Schematic representation of the MR analysis. The three assumptions of MR are as follows: (1) Instrumental variables must be associated with gut microbiota, (2) instrumental variables must not be associated with confounders; and (3) instrumental variables must influence disease outcomes only through gut microbiota, not through other pathways.
Statistical analyses
To detect the causal associations between exposure (GM) and outcomes (CO and CBMI), the inverse-variance weighted (IVW) method was used as the primary MR analysis method. The IVW method, an extension of the Wald estimator for estimating causal effects, constrains the intercept to zero. It calculates the total causal effect using a weighted linear regression model with the weight coefficient (26). IVW results were corrected for multiple comparisons applying the q-value procedure (q < 0.1), while P < 0.05 but q > 0.1 was considered to have a suggestive association (27). The analysis was initially performed in the discovery set and subsequently validated in the replication set.
To evaluate the robustness of our study, we also performed several other MR methods including Maximum Likelihood (MaxLik), Weighted Median (WM), and MR robust adjusted profile score (MR.RAPS). The MaxLik method estimates the parameter values by maximizing the likelihood function with small standard errors (28). The WM method increases the power of causal effect when more than 50% of IVs are valid (29). MR.RAPS provides robust estimates to account for systematic and idiosyncratic pleiotropy, even in the presence of weak IVs (30). Reverse MR analysis was employed to confirm the causal direction. It followed similar methods as forward MR, but with CO/CBMI as the exposures and GM as the outcome.
Cochran's IVW Q statistics and leave-one-out analysis were employed to evaluate potential heterogeneity. MR-Egger intercept and MR Pleiotropy RESidual Sum and Outlier (MR-PRESSO) global test were conducted to detect whether directional horizontal pleiotropy is driving the results of MR analyses (31, 32).
The flowchart of our study was shown in Figure 2. All MR analyses were conducted using the “TwoSampleMR”, “MRPRESSO”, and “qvalue” packages in R software.
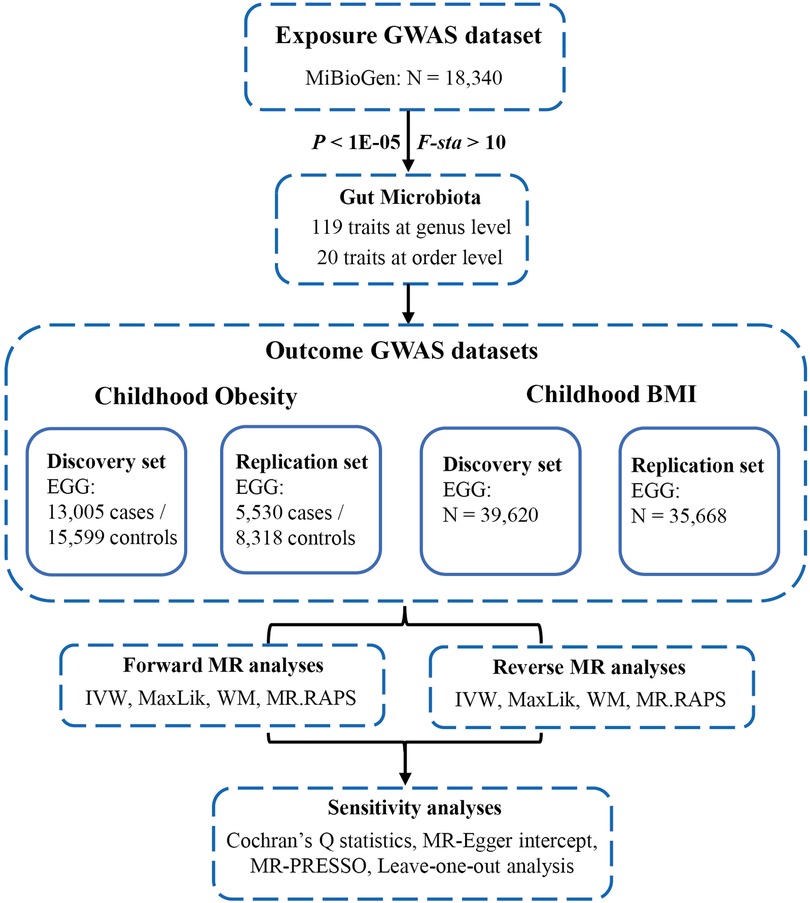
Figure 2. Flowchart of this study. GWAS, genome-wide association studies; MiBioGen, microbiome genome; EGG, early growth genetics; MR, mendelian randomization; IVW, inverse-variance weighted; MaxLik, maximum likelihood; WM, weighted median; MR.RAPS, MR robust adjusted profile score; MR-PRESSO, MR Pleiotropy RESidual Sum and Outlier.
Results
The selected GM instruments based on the criteria containing a total of 8,763 SNPs associated with 119 bacterial genera and 20 bacterial orders. The characters of the selected IVs were presented in Supplementary Table S2.
Childhood obesity
At the genus level, by using the IVW method, we found a suggestive causal association of increase in Eubacterium (eligens group) (OR = 1.710; 95% CI: 1.028, 2.845; P = 0.039) and higher risk of CO, while genetically increased in Akkermansia (OR = 0.574; 95% CI: 0.417, 0.789; P < 0.001), Coprococcus1 (OR = 0.689; 95% CI: 0.487, 0.977; P = 0.036), Eubacterium (oxidoreducens group) (OR = 0.710; 95% CI: 0.511, 0.985; P = 0.040), and Roseburia (OR = 0.665; 95% CI: 0.484, 0.915; P = 0.012) were related to protective effects on CO (Figure 3A). After multiple comparison corrected, we still found a significant causal effect of increased Akkermansia on the lower risk of CO (q = 0.048), the replication dataset also validates this causal relationship (OR = 0.743; 95% CI: 0.586, 0.941; P = 0.014; Figure 3C). Details of all the IVW results in discovery and replication datasets were shown in Supplementary Table S3, S4. The F-statistics ranged from 21.46 to 25.48 among all the results above. Additionally, causal associations between GM and CO risk were found in more than three MR methods (Table 1, Supplementary Figure S1), including IVW, MaxLik, WM, and MR.RAPS methods. However, at the order level, all the P values (ranging from 0.053 to 0.932) were greater than 0.05, indicating no significant associations between bacterial orders and CO (Supplementary Table S5).
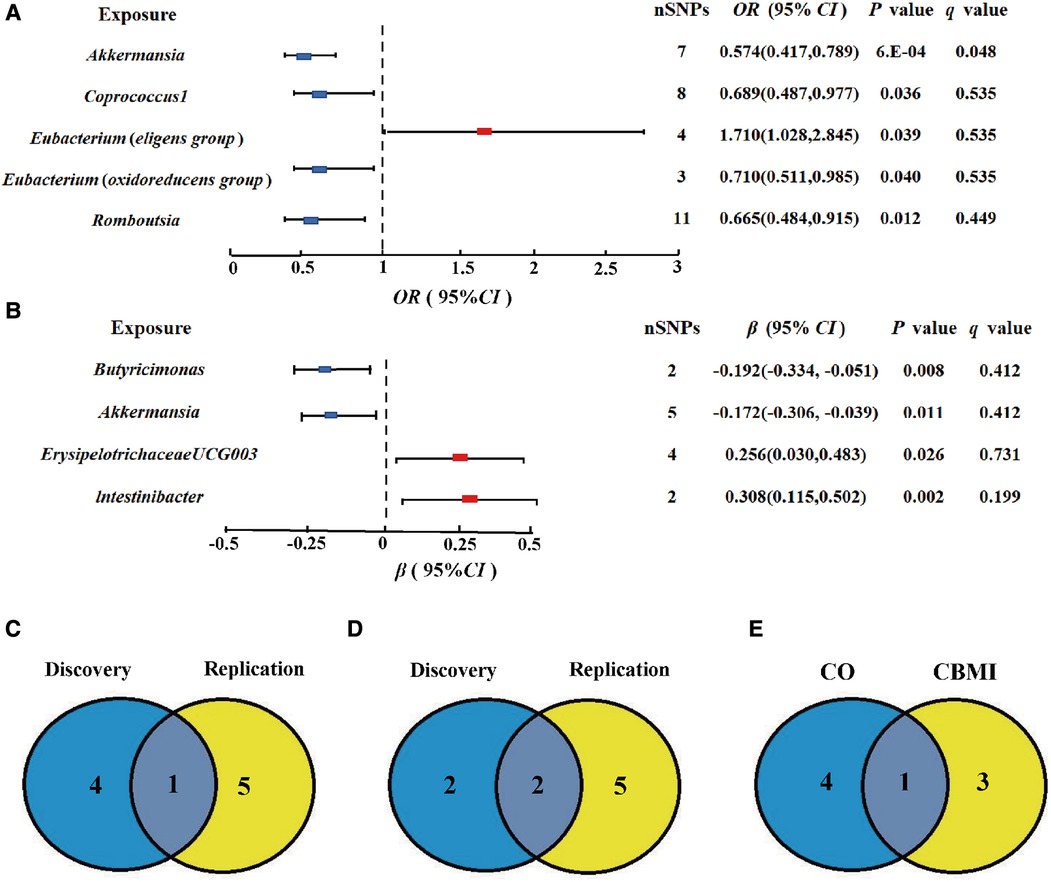
Figure 3. Gut microbiota (GM) which associated with childhood obesity (CO). (A,B) Associations of GM with the risk of CO and childhood body mass index (CBMI) using IVW method. (C) Venn diagram of the CO-related GM in discovery and replication dataset. (D) Venn diagram of the CBMI-related GM in discovery and replication dataset. (E) Venn diagram of the causal GM in CO and CBMI dataset SNP, single nucleotide polymorphisms; OR, odds ratio; Cl, confidence interval.
Cochran's Q statistics showed no significant heterogeneity in selected IVs (P > 0.05 in IVW and MR-Egger methods, Supplementary Table S6). Both the MR-Egger intercept and the MR-PRESSO global test confirmed there is no significant directional horizontal pleiotropy (P > 0.05, Supplementary Table S6). Additionally, the leave-one-out analysis revealed that there are no outlier IVs that would have a significant impact on the result if retained (Supplementary Figure S2).
All methods in reverse MR analysis showed no causal relationship from CO to GM (P > 0.05, Table 2) except for the genus Roseburia. The sensitivity analyses including Cochran's Q statistics, MR-Egger intercept, MR-PRESSO global test, and the leave-one-out analysis demonstrated the robustness of the reverse MR results (Supplementary Table S6, Figure S3).
Childhood BMI
At the genus level, we found four suggestive causal effects of GM on CBMI (P < 0.05, q > 0.1) in the discovery dataset. Specifically, Butyricimonas (β = −0.192; 95% CI: −0.334, −0.051; P = 0.008) and Akkermansia (β = −0.172; 95% CI: −0.306, −0.039; P = 0.011) were negatively associated with CBMI, while ErysipelotrichaceaeUCG003 (β = 0.256; 95%CI: 0.030, 0.483; P = 0.026) and lntestinibacter (β = 0.308; 95% CI: 0.115, 0.502; P = 0.002) were positively associated with CBMI (Figure 3B). However, after multiple comparison corrected, the causal associations didn't exist but the replication dataset also supported the causal effect of Butyricimonas (β = −0.101; 95% CI: −0.181, 0.020; P = 0.015) and lntestinibacter (β = 0.191; 95% CI: 0.090, 0.332; P = 0.008) on CBMI (Figure 3D). Details of all the IVW results in both discovery and replication datasets were shown in Supplementary Table S7, S8. The F-statistics ranged from 22.53 to 27.75 among all the results above. The causal associations between GM and CBMI were found in more than three MR methods (Table 1, Supplementary Figure S4). Furthermore, in the comparison of GM that have a causal relationship with both CO and CBMI, we observed that the genus Akkermansia exhibited the protective effect on both outcomes (Figure 3E). However, at the order level, all the P values (ranging from 0.074 to 0.996) were above 0.05, indicating no significant associations between bacterial orders and CBMI (Supplementary Table S9).
All methods in reverse MR analysis showed no causal relationship from CBMI to GM (P > 0.05, Table 2). The sensitivity analyses demonstrated the robustness of both the forward and reverse MR results (Supplementary Table S10, Figures S5, S6).
Discussion
In this bidirectional two-sample MR study, we detected suggestive causal associations between eight particular bacterial genera and the risk of childhood obesity (five for CO and four for CBMI). We validated these findings in replication datasets and further identified three gut microbiota (GM) taxa, namely Akkermansia, Intestinibacter, and Butyricimonas, that were associated with childhood obesity. Specifically, our MR analysis revealed a protective effect of Akkermansia on childhood obesity (CO) in both the discovery and replication datasets. Importantly, this association remained significant even after correcting for multiple comparisons. Furthermore, this genus was negatively associated with CBMI. Akkermansia, a genus in the phylum Verrucomicrobia, can generate acetate and propionate in the human intestinal tract (33). Previous studies have demonstrated an inverse association between the abundance of Akkermansia and triglyceride levels as well as BMI in genetically obese mice and mice fed a high-fat diet (34, 35). Karlsson et al. conducted a case-control study involving forty preschool students and observed a reduced concentration of Akkermansia in fecal samples from overweight/obese children (36). These findings were consistent with two other cross-sectional studies, providing support for our result (37). Reduced levels of Akkermansia muciniphila may lead to excessive intestinal permeability, whereas increased levels of this genus aid in interleukin-36 protection against obesity (38). Akkermansia has the potential to prevent simple obesity and exhibit hepatoprotective effects through the inhibition of metabolic pathways involving tyrosine, phenylalanine, and tryptophan (39). Additionally, Akkermansia impairs acetyl-CoA oxidation and encourages ketogenesis (40). Taking into account evidence from animal experiments, epidemiological studies, and the potential mechanism by which Akkermansia contributes to body weight regulation, several RCTs were conducted to confirm its protective effect (41–43). In a pilot study involving thirty-three overweight/obese subjects, supplementation with Akkermansia muciniphila for three months led to reductions in several obesity-related indicators, such as fat mass, hip circumference, and plasma total cholesterol, compared to the baseline data (41). It indicated that the genus Akkermansia might be a promising prevention and treatment probiotic target for obesity as well as long-term obesity-related disorders, therefore, further studies could be focused on children to explore its possible effect on childhood obesity.
Our study found that the bacterial genera Intestinibacter and Butyricimonas were negatively associated with CBMI both in discovery and replication datasets. In a meta-analysis examining GM markers associated with obesity, it was found that twenty-three genera, including Butyricimonas, were less abundant in the fecal samples of individuals with simple obesity (44). By inoculating the stools of obesity donors to mice, Rodriguez et al. demonstrated that the Butyricimonas contribute to the decrease in adiposity and hepatic steatosis (45). However, further confirmation of its protective effect against the risk of obesity in the pediatric population is necessary. Similar to our findings, Tian et al. reported that a dietary fiber intervention, known for its weight control benefits, significantly inhibits the growth of Intestinibacter (46). Another RCT conducted in metformin-treated weight loss people also reported the decreased relative abundance of Intestinibacter both in the short and long-time interventions (47). Limited previous studies have investigated the association between Intestinibacter and childhood obesity. However, both observational studies and animal models consistently emphasize the role of Intestinibacter in the production of butyrate, a metabolite generated through microbial fermentation (48, 49). Butyrate increases fatty acid oxidation in the muscle and decreases lipolysis via the orphan G protein–coupled receptor 43 pathway in white adipose tissue (50). In vitro studies demonstrated that butyrate is the primary ATP source for intestinal epithelial cells to absorb nutrition (51). Furthermore, butyrate is metabolized in the liver to produce fatty acids, cholesterol, and ketone bodies, which serve as essential building blocks for fat synthesis (52). In a cross-sectional study, it was observed that the fecal butyrate concentration progressively increases in children with severe obesity when compared to the leaner group (37). All the evidence above may suggest the mechanism that the genus Intestinibacter affects body weight by butyrate metabolism, however, further human-based studies and functional experiments are needed to support this association.
Our study has some strengths. Firstly, this is the first MR analysis to investigate the possible causal associations between GM and childhood obesity with the advantages of fewer confounding factors and rare reverse causations. Secondly, we employed two outcomes, namely obesity and BMI, to provide a comprehensive evaluation of childhood obesity. Additionally, we conducted analyses using both discovery and replication datasets to validate our findings. Thirdly, the data sources of exposure and outcome are the largest GWAS to date, along with bidirectional MR and several sensitivity analyses which ensure the robustness of our findings.
There are still several limitations. Firstly, the significance threshold of exposure IVs was set at 1 × 10−05 because of insufficient IVs under genome-wide significance. However, we tested the F-statistics to exclude the weak instrumental bias. Secondly, the population of the original GWAS is mainly European, which may restrict the generalizability of our findings to other ethnic populations. Thirdly, MR analyses were limited to the order and genus level rather than at a more specific species level due to the constrained resolution of 16S rRNA sequencing. Lastly, we were unable to investigate the relationship between specific measures of general gut microbiota composition and childhood obesity or childhood BMI due to the unavailability of the GWAS dataset on GM composition.
In conclusion, by performing bidirectional MR analyses on GWAS summary data, our study comprehensively explored the causal effects of gut microbiota on childhood obesity. Our findings have the potential to offer valuable insights into the prevention and treatment of childhood obesity, providing a useful strategy, as well as enhancing our understanding of the underlying mechanism from the perspective of gut microbiota. However, further validation of our findings is necessary through additional functional experiments and randomized controlled trials.
Data availability statement
The original contributions presented in the study are included in the article/Supplementary Material, further inquiries can be directed to the corresponding author.
Author contributions
QL conceived the study and performed the statistical analysis. JG composed the article. JL and DL prepared the figures and tables. XW conceived the study and reviewed the article. All authors contributed to the article and approved the submitted version.
Funding
This work was supported by the National Natural Science Foundation of China (82260264), the National Undergraduate Innovation Training Program Project (202110531018, S202310531011), the Natural Science Foundation of Hunan Province (2022JJ40343), the Scientific Research Project of Education Department of Hunan Province (21B0513), and the Scientific Research Project of Hunan Provincial Health Commission (202212053368).
Acknowledgments
We appreciate all the people who gave their help in this study.
Conflict of interest
The authors declare that the research was conducted in the absence of any commercial or financial relationships that could be construed as a potential conflict of interest.
Publisher's note
All claims expressed in this article are solely those of the authors and do not necessarily represent those of their affiliated organizations, or those of the publisher, the editors and the reviewers. Any product that may be evaluated in this article, or claim that may be made by its manufacturer, is not guaranteed or endorsed by the publisher.
Supplementary material
The Supplementary Material for this article can be found online at: https://www.frontiersin.org/articles/10.3389/fped.2023.1229236/full#supplementary-material
References
1. Lobstein T, Jackson-Leach R, Moodie ML, Hall KD, Gortmaker SL, Swinburn BA, et al. Child and adolescent obesity: part of a bigger picture. Lancet. (2015) 385(9986):2510–20. doi: 10.1016/S0140-6736(14)61746-3
2. Collaborators GBDO, Afshin A, Forouzanfar MH, Reitsma MB, Sur P, Estep K, et al. Health effects of overweight and obesity in 195 countries over 25 years. N Engl J Med. (2017) 377(1):13–27. doi: 10.1056/NEJMoa1614362
3. Collaboration NCDRF. Worldwide trends in body-mass index, underweight, overweight, and obesity from 1975 to 2016: a pooled analysis of 2416 population-based measurement studies in 128.9 million children, adolescents, and adults. Lancet. (2017) 390(10113):2627–42. doi: 10.1016/S0140-6736(17)32129-3
4. Franks PW, Hanson RL, Knowler WC, Sievers ML, Bennett PH, Looker HC. Childhood obesity, other cardiovascular risk factors, and premature death. N Engl J Med. (2010) 362(6):485–93. doi: 10.1056/NEJMoa0904130
5. Nehus E, Mitsnefes M. Childhood obesity and the metabolic syndrome. Pediatr Clin North Am. (2019) 66(1):31–43. doi: 10.1016/j.pcl.2018.08.004
6. Tsoi MF, Li HL, Feng Q, Cheung CL, Cheung TT, Cheung BMY. Prevalence of childhood obesity in the United States in 1999–2018: a 20-year analysis. Obes Facts. (2022) 15(4):560–9. doi: 10.1159/000524261
7. Riva A, Borgo F, Lassandro C, Verduci E, Morace G, Borghi E, et al. Pediatric obesity is associated with an altered gut microbiota and discordant shifts in Firmicutes populations. Environ Microbiol. (2017) 19(1):95–105. doi: 10.1111/1462-2920.13463
8. Vijay-Kumar M, Aitken JD, Carvalho FA, Cullender TC, Mwangi S, Srinivasan S, et al. Metabolic syndrome and altered gut microbiota in mice lacking toll-like receptor 5. Science (New York, NY). (2010) 328(5975):228–31. doi: 10.1126/science.1179721
9. Ridaura VK, Faith JJ, Rey FE, Cheng J, Duncan AE, Kau AL, et al. Gut microbiota from twins discordant for obesity modulate metabolism in mice. Science (New York, NY). (2013) 341(6150):1241214. doi: 10.1126/science.1241214
10. Schwiertz A, Taras D, Schäfer K, Beijer S, Bos NA, Donus C, et al. Microbiota and Scfa in lean and overweight healthy subjects. Obesity (Silver Spring, Md). (2010) 18(1):190–5. doi: 10.1038/oby.2009.167
11. Andoh A, Nishida A, Takahashi K, Inatomi O, Imaeda H, Bamba S, et al. Comparison of the gut microbial community between obese and lean peoples using 16s gene sequencing in a Japanese population. J Clin Biochem Nutr. (2016) 59(1):65–70. doi: 10.3164/jcbn.15-152
12. Escobar JS, Klotz B, Valdes BE, Agudelo GM. The gut microbiota of colombians differs from that of Americans, Europeans and Asians. BMC Microbiol. (2014) 14:311. doi: 10.1186/s12866-014-0311-6
13. Smith GD, Ebrahim S. ‘Mendelian randomization’: can genetic epidemiology contribute to understanding environmental determinants of disease? Int J Epidemiol. (2003) 32(1):1–22. doi: 10.1093/ije/dyg070
14. Lawlor DA, Harbord RM, Sterne JA, Timpson N, Davey Smith G. Mendelian randomization: using genes as instruments for making causal inferences in epidemiology. Stat Med. (2008) 27(8):1133–63. doi: 10.1002/sim.3034
15. Kurilshikov A, Medina-Gomez C, Bacigalupe R, Radjabzadeh D, Wang J, Demirkan A, et al. Large-scale association analyses identify host factors influencing human gut microbiome composition. Nat Genet. (2021) 53(2):156–65. doi: 10.1038/s41588-020-00763-1
16. Quast C, Pruesse E, Yilmaz P, Gerken J, Schweer T, Yarza P, et al. The silva ribosomal rna gene database project: improved data processing and web-based tools. Nucleic Acids Res. (2013) 41(Database issue):D590–6. doi: 10.1093/nar/gks1219
17. Flegal KM, Wei R, Ogden C. Weight-for-stature compared with body mass index-for-age growth charts for the United States from the centers for disease control and prevention. Am J Clin Nutr. (2002) 75(4):761–6. doi: 10.1093/ajcn/75.4.761
18. Bradfield JP, Vogelezang S, Felix JF, Chesi A, Helgeland Ø, Horikoshi M, et al. A trans-ancestral meta-analysis of genome-wide association studies reveals loci associated with childhood obesity. Hum Mol Genet. (2019) 28(19):3327–38. doi: 10.1093/hmg/ddz161
19. Bradfield JP, Taal HR, Timpson NJ, Scherag A, Lecoeur C, Warrington NM, et al. A genome-wide association meta-analysis identifies new childhood obesity loci. Nat Genet. (2012) 44(5):526–31. doi: 10.1038/ng.2247
20. Vogelezang S, Bradfield JP, Ahluwalia TS, Curtin JA, Lakka TA, Grarup N, et al. Novel loci for childhood body mass index and shared heritability with adult cardiometabolic traits. PLoS Genet. (2020) 16(10):e1008718. doi: 10.1371/journal.pgen.1008718
21. Felix JF, Bradfield JP, Monnereau C, van der Valk RJ, Stergiakouli E, Chesi A, et al. Genome-wide association analysis identifies three new susceptibility loci for childhood body mass index. Hum Mol Genet. (2016) 25(2):389–403. doi: 10.1093/hmg/ddv472
22. Sanna S, van Zuydam NR, Mahajan A, Kurilshikov A, Vich Vila A, Võsa U, et al. Causal relationships among the gut microbiome, short-chain fatty acids and metabolic diseases. Nat Genet. (2019) 51(4):600–5. doi: 10.1038/s41588-019-0350-x
23. Purcell S, Neale B, Todd-Brown K, Thomas L, Ferreira MA, Bender D, et al. Plink: a tool set for whole-genome association and population-based linkage analyses. Am J Hum Genet. (2007) 81(3):559–75. Epub 20070725. doi: 10.1086/519795
24. Johnson AD, Handsaker RE, Pulit SL, Nizzari MM, O'Donnell CJ, de Bakker PI. Snap: a web-based tool for identification and annotation of proxy snps using hapmap. Bioinformatics (Oxford, England). (2008) 24(24):2938–9. doi: 10.1093/bioinformatics/btn564
25. Burgess S, Thompson SG. Avoiding bias from weak instruments in Mendelian randomization studies. Int J Epidemiol. (2011) 40(3):755–64. doi: 10.1093/ije/dyr036
26. Burgess S, Butterworth A, Thompson SG. Mendelian randomization analysis with multiple genetic variants using summarized data. Genet Epidemiol. (2013) 37(7):658–65. doi: 10.1002/gepi.21758
27. Storey JD, Tibshirani R. Statistical significance for genomewide studies. Proc Natl Acad Sci U S A. (2003) 100(16):9440–5. doi: 10.1073/pnas.1530509100
28. Luque-Fernandez MA, Schomaker M, Rachet B, Schnitzer ME. Targeted maximum likelihood estimation for a binary treatment: a tutorial. Stat Med. (2018) 37(16):2530–46. doi: 10.1002/sim.7628
29. Bowden J, Davey Smith G, Haycock PC, Burgess S. Consistent estimation in Mendelian randomization with some invalid instruments using a weighted median estimator. Genet Epidemiol. (2016) 40(4):304–14. doi: 10.1002/gepi.21965
30. Zhao Q, Wang J, Hemani G, Bowden J, Small DS. Statistical inference in two-sample summary-data Mendelian randomization using robust adjusted profile score. The Annals of Statistics. (2018) 48(3).
31. Verbanck M, Chen CY, Neale B, Do R. Detection of widespread horizontal pleiotropy in causal relationships inferred from Mendelian randomization between complex traits and diseases. Nat Genet. (2018) 50(5):693–8. doi: 10.1038/s41588-018-0099-7
32. Rees JMB, Wood AM, Burgess S. Extending the Mr-Egger method for multivariable Mendelian randomization to correct for both measured and unmeasured pleiotropy. Stat Med. (2017) 36(29):4705–18. doi: 10.1002/sim.7492
33. Becken B, Davey L, Middleton DR, Mueller KD, Sharma A, Holmes ZC, et al. Genotypic and phenotypic diversity among human isolates of Akkermansia Muciniphila. mBio. (2021) 12(3). doi: 10.1128/mBio.00478-21
34. Parks BW, Nam E, Org E, Kostem E, Norheim F, Hui ST, et al. Genetic control of obesity and gut Microbiota composition in response to high-fat, high-sucrose diet in mice. Cell Metab. (2013) 17(1):141–52. doi: 10.1016/j.cmet.2012.12.007
35. Suriano F, Vieira-Silva S, Falony G, Roumain M, Paquot A, Pelicaen R, et al. Novel insights into the genetically obese (Ob/Ob) and diabetic (Db/Db) mice: two sides of the same coin. Microbiome. (2021) 9(1):147. doi: 10.1186/s40168-021-01097-8
36. Karlsson CL, Onnerfält J, Xu J, Molin G, Ahrné S, Thorngren-Jerneck K. The microbiota of the gut in preschool children with normal and excessive body weight. Obesity (Silver Spring, Md). (2012) 20(11):2257–61. doi: 10.1038/oby.2012.110
37. Gyarmati P, Song Y, Dotimas J, Yoshiba G, Christison A. Cross-sectional comparisons of gut microbiome and short-chain fatty acid levels among children with varied weight classifications. Pediatr Obes. (2021) 16(6):e12750. doi: 10.1111/ijpo.12750
38. Miura K, Ohnishi H. Role of gut microbiota and toll-like receptors in nonalcoholic fatty liver disease. World J Gastroenterol. (2014) 20(23):7381–91. doi: 10.3748/wjg.v20.i23.7381
39. Giannoudaki E, Hernandez-Santana YE, Mulfaul K, Doyle SL, Hams E, Fallon PG, et al. Interleukin-36 cytokines alter the intestinal microbiome and can protect against obesity and metabolic dysfunction. Nat Commun. (2019) 10(1):4003. doi: 10.1038/s41467-019-11944-w
40. Depommier C, Everard A, Druart C, Maiter D, Thissen JP, Loumaye A, et al. Serum metabolite profiling yields insights into health promoting effect of A. Muciniphila in human volunteers with a metabolic syndrome. Gut Microbes. (2021) 13(1):1994270. doi: 10.1080/19490976.2021.1994270
41. Depommier C, Everard A, Druart C, Plovier H, Van Hul M, Vieira-Silva S, et al. Supplementation with Akkermansia Muciniphila in overweight and obese human volunteers: a proof-of-concept exploratory study. Nat Med. (2019) 25(7):1096–103. doi: 10.1038/s41591-019-0495-2
42. Depommier C, Vitale RM, Iannotti FA, Silvestri C, Flamand N, Druart C, et al. Beneficial effects of Akkermansia Muciniphila are not associated with Major changes in the circulating endocannabinoidome but linked to higher mono-palmitoyl-glycerol levels as new pparα agonists. Cells. (2021) 10(1). doi: 10.3390/cells10010185
43. Perraudeau F, McMurdie P, Bullard J, Cheng A, Cutcliffe C, Deo A, et al. Improvements to postprandial glucose control in subjects with type 2 diabetes: a multicenter, double blind, randomized placebo-controlled trial of a novel probiotic formulation. BMJ Open Diabetes Res Care. (2020) 8(1). doi: 10.1136/bmjdrc-2020-001319
44. Gong J, Shen Y, Zhang H, Cao M, Guo M, He J, et al. Gut microbiota characteristics of people with obesity by meta-analysis of existing datasets. Nutrients. (2022) 14(14). doi: 10.3390/nu14142993
45. Rodriguez J, Hiel S, Neyrinck AM, Le Roy T, Pötgens SA, Leyrolle Q, et al. Discovery of the gut microbial signature driving the efficacy of prebiotic intervention in obese patients. Gut. (2020) 69(11):1975–87. doi: 10.1136/gutjnl-2019-319726
46. Tian T, Zhang X, Luo T, Wang D, Sun Y, Dai J. Effects of short-term dietary fiber intervention on gut microbiota in young healthy people. Diabetes, Metab Syndr Obes: Targets Ther. (2021) 14:3507–16. doi: 10.2147/dmso.S313385
47. Mueller NT, Differding MK, Zhang M, Maruthur NM, Juraschek SP, Miller ER III, et al. Metformin affects gut microbiome composition and function and circulating short-chain fatty acids: a randomized trial. Diabetes Care. (2021) 44(7):1462–71. doi: 10.2337/dc20-2257
48. Zhang L, Wang Z, Zhang X, Zhao L, Chu J, Li H, et al. Alterations of the gut microbiota in patients with diabetic nephropathy. Microbiol Spectr. (2022) 10(4):e0032422. doi: 10.1128/spectrum.00324-22
49. Uddin MK, Mahmud MR, Hasan S, Peltoniemi O, Oliviero C. Dietary micro-fibrillated cellulose improves growth, reduces diarrhea, modulates gut microbiota, and increases butyrate production in post-weaning piglets. Sci Rep. (2023) 13(1):6194. doi: 10.1038/s41598-023-33291-z
50. Terova G, Díaz N, Rimoldi S, Ceccotti C, Gliozheni E, Piferrer F. Effects of sodium butyrate treatment on histone modifications and the expression of genes related to epigenetic regulatory mechanisms and immune response in European sea bass (dicentrarchus labrax) fed a plant-based diet. PloS one. (2016) 11(7):e0160332. doi: 10.1371/journal.pone.0160332
51. Macfarlane GT, Macfarlane S. Fermentation in the human large intestine: its physiologic consequences and the potential contribution of prebiotics. J Clin Gastroenterol. (2011) 45(Suppl):S120–7. doi: 10.1097/MCG.0b013e31822fecfe
Keywords: childhood obesity, childhood BMI, gut microbiota, Mendelian randomization, causal relationship
Citation: Li Q, Gao J, Luo J, Lin D and Wu X (2023) Mendelian randomization analyses support causal relationship between gut microbiota and childhood obesity. Front. Pediatr. 11:1229236. doi: 10.3389/fped.2023.1229236
Received: 26 May 2023; Accepted: 13 July 2023;
Published: 1 August 2023.
Edited by:
Anna Di Sessa, University of Campania Luigi Vanvitelli, ItalyReviewed by:
Luis Rodrigo Macias Kauffer, Universität zu Lübeck, GermanyLenycia De Cassya Lopes Neri, University of São Paulo, Brazil
© 2023 Li, Gao, Luo, Lin and Wu. This is an open-access article distributed under the terms of the Creative Commons Attribution License (CC BY). The use, distribution or reproduction in other forums is permitted, provided the original author(s) and the copyright owner(s) are credited and that the original publication in this journal is cited, in accordance with accepted academic practice. No use, distribution or reproduction is permitted which does not comply with these terms.
*Correspondence: Xinrui Wu d3V4aW5ydWk5OUBxcS5jb20=
†These authors have contributed equally to this work and share first authorship