- 1Department of Anesthesiology, Pharmacology & Therapeutics, University of British Columbia, Vancouver, BC, Canada
- 2Centre for International Child Health, BC Children’s Hospital Research Institute, Vancouver, BC, Canada
- 3WALIMU, Kampala, Uganda
- 4Department of Pediatrics, Jinja Regional Referral Hospital, Jinja, Uganda
- 5Department of Pediatrics, University of British Columbia, Vancouver, BC, Canada
Introduction: Early and accurate recognition of children at risk of progressing to critical illness could contribute to improved patient outcomes and resource allocation. In resource limited settings digital triage tools can support decision making and improve healthcare delivery. We developed a model for rapid identification of critically ill children at triage.
Methods: This was a prospective cohort study of acutely ill children presenting at Jinja Regional Referral Hospital in Eastern Uganda. Variables collected in the emergency department informed the development of a logistic model based on hospital admission using bootstrap stepwise regression. Low and high-risk thresholds for 90% minimum sensitivity and specificity, respectively generated three risk level categories. Performance was assessed using receiver operating characteristic curve analysis on a held-out test set generated by an 80:20 split with 10-fold cross validation. A risk stratification table informed clinical interpretation.
Results: The model derivation cohort included 1,612 participants, with an admission rate of approximately 23%. The majority of admitted patients were under five years old and presenting with sepsis, malaria, or pneumonia. A 9-predictor triage model was derived: logit (p) = −32.888 + (0.252, square root of age) + (0.016, heart rate) + (0.819, temperature) + (−0.022, mid-upper arm circumference) + (0.048 transformed oxygen saturation) + (1.793, parent concern) + (1.012, difficulty breathing) + (1.814, oedema) + (1.506, pallor). The model afforded good discrimination, calibration, and risk stratification at the selected thresholds of 8% and 40%.
Conclusion: In a low income, pediatric population, we developed a nine variable triage model with high sensitivity and specificity to predict who should be admitted. The triage model can be integrated into any digital platform and used with minimal training to guide rapid identification of critically ill children at first contact. External validation and clinical implementation are in progress.
Introduction
Child mortality in low- and-middle income countries (LMICs) remains high (1). Most of these deaths are preventable or easy to treat and occur within 24 h of hospital admission (2). Rapid identification of critical illness is important because delay in treatment is associated with an escalated risk of death and disability (2). Triage, the prioritization of patients to identify the sickest for earliest intervention, can optimize resource allocation and markedly improve patient outcomes especially in resource limited settings (3–5).
The validity of current pediatric triage tools is uncertain (6, 7), particularly within resource limited settings. A lack of standardized data and insufficient follow-up on children not admitted to health facilities remain major limitations of research in this field. The current gold standards for triage are the World Health Organization's (WHO) Integrated Management of Childhood Illness (IMCI) (8) and Emergency Triage Assessment and Treatment Plus (ETAT+) (9) guidelines for diagnosing and managing sick patients. However, health workers in resource limited settings often lack the training and infrastructure to follow these clinical recommendations (10, 11).
Digital health interventions have the potential to support clinical decision making and improve the quality of care in resource limited settings (12). Digital platforms can facilitate quality improvement, and data-driven algorithms can be continuously updated and improved with new information or optimized to meet the specific needs of each setting (13). It has been demonstrated that digital triage tools can optimize resource allocations, reduce treatment wait times, and improve patient outcomes (14).
We describe the development of a simple triage model combining clinical signs, symptoms, and readily available vital signs that can be embedded into a digital platform. The model was designed for use with limited clinical training to guide rapid identification of critically ill children at the first point of contact. Model development was the first phase in the Smart Triage study. The model has since been implemented in a mobile triage platform and is being used at hospital sites in Kenya and Uganda with the goal of reducing time to treatment in children.
Methods
Model development and reporting followed TRIPOD (transparent reporting of a multivariable prediction model for individual prognosis or diagnosis) guidelines (Supplementary File S1) (15).
Trial registration
Clinical Trials.gov Identifier: NCT04304235, Registered 11 March 2020.
Study design and setting
This study was conducted at the pediatric emergency department (ED) of Jinja Regional Referral Hospital (JRRH), a public hospital funded by the Uganda Ministry of Health. JRRH is the largest referral hospital in Eastern Uganda and serves patients residing in Jinja and eight surrounding districts. In 2021, the pediatric ED evaluated 29,022 patients and had an admission rate of approximately 20%. There are 87 admission beds in the unit. Most children who present to the pediatric ED are under five years of age.
Triage is conducted by one designated nurse with assistance from two to three student nurses.
This was a prospective cohort study conducted between April 2020 and March 2021. This study was approved by the institutional review boards at the University of British Columbia in Canada (ID: H19-02398; H20-00484), the Makerere University School of Public Health in Uganda and the Uganda National Council for Science and Technology. This is one modelling component of a multi-site clinical study for which the full protocol has been published (16).
Eligibility and sampling
Children under 19 years of age seeking assessment for an acute illness at the pediatric emergency department of JRRH between 8:00 am and 5:00 pm were enrolled. Children presenting for elective procedures, scheduled appointments or treatment of chronic illnesses were not eligible for enrollment. Participation was voluntary and written informed consent was provided by a parent or guardian prior to enrollment. Assent was required from children above eight years of age. A quasi-random sampling method based on time cut-offs was adopted (17).
Data collection and management
Study procedures were initiated in the triage waiting area of the pediatric OPD. Following enrollment, study nurses conducted an interview and clinical examination to capture the candidate predictor variables (Supplementary Table S2). Study procedures did not interfere with or introduce delays to care delivery. Data were collected using password secured Android tablets, and a custom-built mobile application with an encrypted database. The Masimo iSpO2® Pulse Oximeter with micro USB connector was connected directly to the tablet to collect pulse oximetry and heart rate and the SureTemp 692 thermometer was used to measure core temperature. At the end of each day, data was uploaded directly from the Android tablets to REDCap (Research Electronic Data Capture) (18) and sent to the central study server at the BC Children's Hospital Research Institute. After each upload, data on the tablets was automatically deleted. Standard operating protocols for data collection and management are available on the Pediatric Sepsis CoLab Dataverse (17).
Candidate predictor variables
Predictor variables were based on sepsis, the most common cause of preventable child deaths from infection (19). Candidate predictor variables were generated in accordance with the guidelines developed by the Pediatric Sepsis Predictor Standardization (PS2) working group (20, 21). The PS2 working group is a subgroup within the Pediatric Sepsis CoLab, a global network for collaboration and data sharing to improve the quality of care and decrease morbidity and mortality from sepsis (22). Guidelines for the standardized collection of predictor variables in studies for pediatric sepsis were derived using a systematic review and a modified Delphi approach to standardize definitions and assign a tier level to each variable using a three-point tiering system (Tier 1: essential, Tier 2: Important, Tier 3: Exploratory) (21, 23). Due to limitations in time and resources at the point of triage in resource limited settings, all Tier 1 variables and relevant Tier 2 variables (as determined by study investigators) applicable to children of all ages were selected for inclusion, for a total of 63 candidate predictor variables (Supplementary Table S2).
Modelling outcome
The primary outcome was hospital admission (as determined by the hospital clinicians) for more than 24 h. Currently, need for admission at JRRH is determined using ETAT + guidelines and clinician expertise. To capture any critically ill cases who may have been sent home on the day of enrollment, children readmitted within 48 h were also considered to have a positive admission outcome. The hospital registry was used to collect data on admission status. Caregivers were called 7 days post-discharge, or 7 days post-study enrollment (for non-admitted patients), to confirm admission status and determine readmission status. Therefore, readmissions to other healthcare centers were also counted in our primary outcome.
Statistical analysis
Sample size
The sample size estimation was computed based on the number of predictors expected in the final model (n), the outcome event rate (I), and calculated as N = (n × 10)/I by employing the typical minimum standard of 10 events per effective variable. Preliminary analysis at the study site suggested an estimated admission rate of 20%, and thus to allow for a model with 10 predictors we require a minimum sample of 500 participants. Based on the uncertainty commonly present in these smaller sample sizes and clinical feasibility (large case load), we targeted a minimum sample of 1,500 participants (24).
Model development, validation, and calibration
As missing data was expected to be minimal (<5%), median and mode imputation strategies were used for continuous and categorical predictors, respectively. Predictors with less than 10 events per variable were not considered for inclusion in the model to reduce potential of overfitting. Continuous variables were assessed graphically for linear associations with the outcome and transformed where appropriate. A physiological transformation of oxygen saturation was used to linearize the relationship between oxygen severity and impairment of gas exchange. Transformation was based on an altitude adaptive virtual shunt model [70.103 × log10(101.687 − SpO2) − 55.833], which has been demonstrated to improve the fit of logistic regression models (25, 26).
Bootstrapped stepwise regression was used to select predictors to comprise the logistic regression model. A stepwise selection procedure, which repetitively added or dropped predictors to minimize Akaike information criterion (AIC), was repeated on 2,000 bootstrap replicates (27). A final consensus model was derived from the predictors present in at least 80% of the bootstrap sample models. To quantify the predictive accuracy of the consensus predictors, data was split into training (80%) and validation (20%) sets and a 10-fold cross validation procedure was applied to compute a pooled estimate of the area under the receiver operating characteristic (AUROC) curve, sensitivity, specificity, predictive values, and likelihood ratios. Standard errors of these characteristics were derived from 2,000 bootstrap replicates. Calibration was evaluated graphically (28). The final model was applied and evaluated using the methods above on the subpopulation of children under five years of age to assess robustness across age groups. The model was performance was also evaluated on only children under 5 years and alternatively using all admissions (regardless of length of stay) as positive outcome to assess robustness.
Risk stratification and clinical interpretation
Two risk thresholds were selected to divide participants into three triage categories (emergency, priority, and non-urgent). Low and high-risk thresholds were selected to correspond with a sensitivity and specificity of at least 90%, respectively. These markers were selected to develop a triage model highly sensitive in detecting high risk cases (avoiding false negatives) but specific in identifying emergency cases (avoiding false positives) to optimize resource allocation while seeing that critically ill children do not experience delays. A risk stratification table was used to evaluate model classification accuracy, defined as the ability of the model to separate participants into triage categories, such that cases with and without outcomes are more likely to be in the higher and lower risk strata, respectively.
Patient and public involvement
Patients were not involved in the conceptualisation, design or conduct of this study. The results of the study will not be disseminated directly to participants.
Results
Participants
During April 27th, 2020 and March 31st, 2021, 2,030 patients were screened for eligibility, of which 418 (20.6%) were excluded (Figure 1). In total, 1,612 participants were enrolled in the study. The prevalence of males and females was roughly equal and approximately 90% of the cohort was comprised of children under 5 years of age (Table 1). According to the anthropometric characteristics, 147 (12.3%) participants were underweight (weight-for-age z-score <−2), 325 (20.2%) were stunted (height-for-age z-score <−2), and 169 (10.5%) were wasted (weight-for-height z-score <−2) (Table 1).
A total of 375 (23.3%) participants were admitted to the hospital, of which 343 (21.3%) were admitted for a minimum duration of 24 h and 4 (0.1%) were readmitted within 48 h of discharge (Table 1). Additionally, 19 (1.9%) participants initially sent home returned and were admitted to a hospital or health facility within 48 h. This resulted in 364 (22.6%) positive admission outcomes (Table 1). The most common reasons for admission were malaria (45.3% of admitted participants), sepsis (22.1%), and pneumonia (13.3%) (Table 1). Five variables did not meet the minimum criteria of 10 events for inclusion in the model derivation process (Supplementary Table S3). Univariate analysis revealed many variables to be significantly associated with the admission outcome and missing data was minimal (Supplementary Table S3).
Triage model
The equation for the derived nine-predictor logistic triage model was: logit (p) = −32.888 + (0.252, square root of age) + (0.016, heart rate) + (0.819, temperature) + (−0.022, mid-upper arm circumference) + (0.048 transformed oxygen saturation) + (1.793, parent concern) + (1.012, difficulty breathing) + (1.814, oedema) + (1.506, pallor (Table 2). The model achieved good predictive accuracy quantified by a cross validated AUROC curve of 86% (Figure 2A) and was well calibrated (Figure 2B).
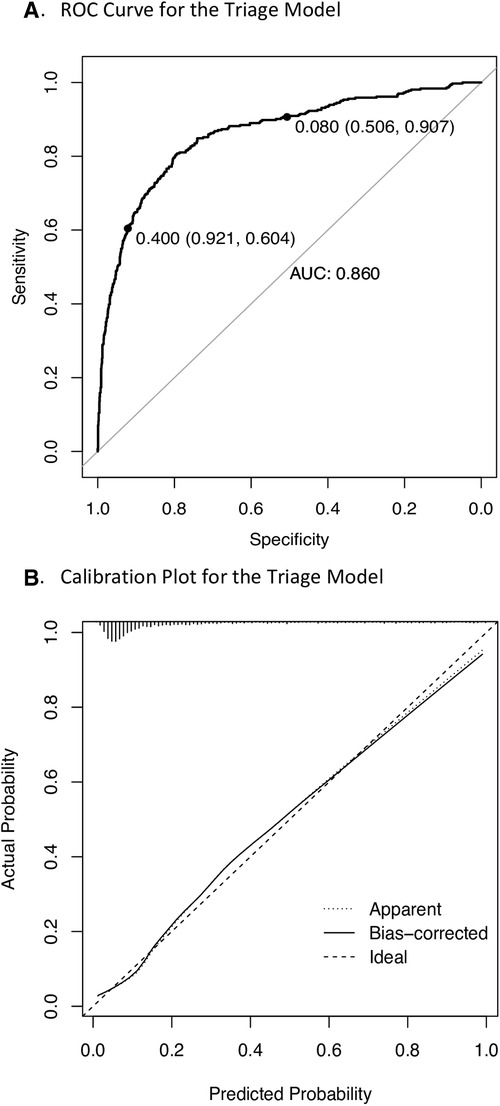
Figure 2. Performance of the triage model in the study cohort. ROC, receiver operating characteristics; AUC, area under the curve. (A) 10-fold cross validated ROC curve where labelled points represent low risk (8%) and high risk (40%) thresholds. (B) Calibration plot where 45-degree straight line corresponds to the line of perfect calibration on which model predicted risks coincide with the observed frequency and the bias corrected curve represents pooled results from 1,000 bootstrap samples.
Risk stratification
The model achieved 91% (95% CI 88%, 94%) sensitivity at an 8% low-risk threshold and 92% (95% CI 90%, 94%) specificity at a 40% high-risk threshold (Figure 2A) (Table 3). The non-urgent and priority strata each comprised of approximately 40% of participants, while the remaining 20% were stratified as emergency (Table 3). The model achieved similar AUROC performance characteristics when applied to children under five years of age and when admission of any duration was used as the modelling outcome (Supplementary Figures S4). This demonstrates robustness across the range of ages and to adjustments of the admission outcome.
Discussion
Summary
The logistic triage model comprised of five objectively measurable (age, heart rate, temperature, oxygen saturation, MUAC) and four easily assessable (difficulty breathing, oedema, pallor, parent concern) predictor variables was developed using hospital admission as an indicator of acuity. Most predictors retained in this model are consistent with previous pediatric triage models (29, 30); and international guidelines (8). Parent concern is not currently included in ETAT + or used as a formal criterion to determine admission status. Nevertheless, there is growing evidence to support the role of parent concern in early recognition of critical illness in children (31). This model, when used with good clinical judgement and adequate quality control can help and guide clinical decision making in terms of patient prioritization. This model represents the first iteration of a triage tool that will be continuously updated to reflect the latest available data and adapted based on illness profiles and population dynamics.
The WHO has acknowledged that digital health interventions and systems that use patient data to drive health practices can lead to improved quality of care (32). An ETAT + mobile health intervention and an electronic IMCI algorithm implemented at primary health centres in Malawi and Burkina Faso, respectively, improved both triage and resource allocation (33, 34). Yet data-driven triage algorithms provide unique benefits over digitally implemented clinical guidelines. They enable quality improvement, are easily updated, and can be adjusted to meet setting-specific needs. Implementation of a standardized platform for data collection (21), and detailed standard operating protocols including a robust mechanism to capture follow up of discharged participants (20) will enable reproducibility required for model validation that is lacking in the current literature.
It should be noted that this triage model cannot and does not function to replace ETAT + but rather to serve as an adjunct to the patient prioritization process. ETAT + has been developed as a package of guidelines, training, and quality improvement in East Africa, and has become the national framework for facility-based pediatric care in this country. This triage algorithm helps guide patient prioritization at first contact for children who would otherwise be waiting in line for hours to be formally triaged and treated by health workers.
Clinical interpretation
More than 90% of admitted participants were diagnosed with sepsis or infectious diseases thus validating the use of sepsis predictors as candidate predictors of critical illness in model development (Table 1). The high negative predictive performance of the model at the low-risk threshold supports its ability to exclude low-risk patients while the negative likelihood ratio indicates a 5.5-fold decrease in the odds of needing hospital admission for a patient classified as non-urgent (Table 3). A positive predictive value of 35% (95% CI 34%, 38%) was achieved at the high-risk threshold which, given the relatively low prevalence of positive admission outcomes, demonstrates capability of the model to correctly classify emergency patients. The positive likelihood ratio indicates a 7.5-fold increase in the odds of needing hospital admission for a patient classified as emergency (Table 3). Furthermore, 91.5% of participants admitted for at least 24 h, 78.3% of participants readmitted within 48 h, and 100% of deaths were contained in either the priority or emergency category (Table 3).
Limitations
This model may not be generalizable to other contexts as it was developed from a very young cohort population, with high rates of malaria and malnutrition. While this type of cohort is typical of pediatric populations in low-income countries, model performance may be affected by varying age distributions and in settings without high rates of malaria and malnutrition.
A major limitation of the study was the use of hospital admission as a surrogate for severity of illness. The study cohort consisted only of children presenting with acute illnesses. To exclude admitted cases lacking severe illness, a duration of stay of at least 24 h was required to count as a positive outcome. A 7-day follow up call was conducted to capture children inadvisably sent home. Children who returned to a health facility and were readmitted within 48 h were also counted as positive outcomes. Mortality was also ascertained from the follow up call. Deaths in this 7-day period were minimal for both admitted (n = 9) and non-admitted (n = 2) participants and meaningful analysis could not be conducted. Both non-admitted cases that died at home were classified as emergency by the triage model and perhaps should have been considered positive outcomes. This however was unlikely to have impacted the results due to the low mortality rate of children sent home (0.6%).
Another limitation is the lack of external validation, which is needed to assess the model's clinical utility. The model is currently being evaluated in a multisite study that will include clinical implementation in low-resource hospitals across sub-Saharan Africa to assess performance in varied geographical locations, seasons, and with different disease prevalence and severity (16).
Finally, there are concerns regarding implementation of digital health systems in LMICs. These include limited availability of smartphones, computer infrastructure, and a continuous supply of electricity, in addition to staffing attitude and training challenges. In response, to complement the digital triage interventional study, we are conducting a cost-effectiveness analysis to maximize the benefit of implementing such technology in low resource hospitals (35). The WHO views digital health as an accessible and affordable solution for global health delivery in LMICs and an important tool in achieving sustainable development goals (36). Safe and appropriate scale up has been prioritized within national and global digital health strategies (37–39).
Data availability statement
The data studied, data dictionary, and code are available from the Pediatric Sepsis CoLab Dataverse, subject to an application meeting the ethical and governance requirements of the CoLab. Requests to access these datasets should be directed to jessica.trawin@cw.bc.ca (40).
Ethics statement
The studies involving human participants were reviewed and approved by the institutional review boards at the University of British Columbia in Canada (ID: H19-02398; H20-00484), the Makerere University School of Public Health in Uganda and the Uganda National Council for Science and Technology. Written informed consent to participate in this study was provided by the participants’ legal guardian/next of kin.
Author contributions
MA, MOW, NK, TAV, NKM conceived of and designed the study. CK, AM, EL and TAV were responsible for data collection and study monitoring. DD was responsible for software development, data collection systems, and data management. AM conducted the statistical analysis. AM wrote the manuscript, which was critically reviewed and revised by SKN. All authors contributed to the article and approved the submitted version.
Funding
This research was supported by the Wellcome Trust Innovator Award (ID: 215695/B/19/Z), University of Oxford, Engineering and Physical Sciences Research Council, National Institute for Health Research Oxford Biomedical Research Centre. The funders of the study had no role in study design, data collection, data analysis, data interpretation, or writing of the manuscript. All authors had full access to all the data in the study and accept responsibility to submit for publication.
Acknowledgments
We would like to thank the administration and staff of Jinja Regional Referral Hospital, participants and caregivers for their time and enthusiasm. We would also like to thank the research nurses and timekeepers for their hard work and dedication to Smart Triage.
Conflict of interest
NK serves as a Specialty Chief Editor for Frontiers in Pediatrics. The peer-review process was guided by an independent editor, and the authors have no other competing interests to declare.
Publisher's note
All claims expressed in this article are solely those of the authors and do not necessarily represent those of their affiliated organizations, or those of the publisher, the editors and the reviewers. Any product that may be evaluated in this article, or claim that may be made by its manufacturer, is not guaranteed or endorsed by the publisher.
Supplementary material
The Supplementary Material for this article can be found online at: https://www.frontiersin.org/articles/10.3389/fped.2022.976870/full#supplementary-material.
References
1. World Health Organization. Children: improving survival and well-being. (2020). Available at: https://www.who.int/news-room/fact-sheets/detail/children-reducing-mortality (cited April 1, 2021).
2. Jofiro G, Jemal K, Beza L, Bacha Heye T. Prevalence and associated factors of pediatric emergency mortality at Tikur Anbessa specialized tertiary hospital: a 5 year retrospective case review study. BMC Pediatr. (2018) 18(1):316. doi: 10.1186/s12887-018-1287-4
3. Dekker-Boersema J, Hector J, Jefferys LF, Binamo C, Camilo D, Muganga G, et al. Triage conducted by lay-staff and emergency training reduces paediatric mortality in the emergency department of a rural hospital in northern Mozambique. Afr J Emerg Med. (2019) 9(4):172–6. doi: 10.1016/j.afjem.2019.05.005
4. Molyneux E, Ahmad S, Robertson A. Improved triage and emergency care for children reduces inpatient mortality in a resource-constrained setting. Bull World Health Organ. (2006) 8(4):314–9. doi: 10.2471/BLT.04.019505
5. Turner EL, Nielsen KR, Jamal SM, von Saint André-von Arnim A, Musa NL. A review of pediatric critical care in resource-limited settings: a look at past, present, and future directions. Front Pediatr. (2016) 4:5. doi: 10.3389/fped.2016.00005
6. Hansoti B, Jenson A, Keefe D, De Ramirez SS, Anest T, Twomey M, et al. Reliability and validity of pediatric triage tools evaluated in low resource settings: a systematic review. BMC Pediatr. (2017) 17(1):37. doi: 10.1186/s12887-017-0796-x
7. Jenson A, Hansoti B, Rothman R, de Ramirez SS, Lobner K, Wallis L. Reliability and validity of emergency department triage tools in low- and middle-income countries: a systematic review. Eur J Emerg Med. (2018) 25(3):154–60. doi: 10.1097/MEJ.0000000000000445
8. World Health Organization. IMCI chart booklet. Geneva: World Health Organization. (2014). Available at: http://www.who.int/maternal_child_adolescent/documents/IMCI_chartbooklet/en/ (cited April 1, 2021).
9. Emergency Triage Assessment and Treatment (ETAT) course. Geneva: World Health Organization. (2005). Available at: http://www.who.int/maternal_child_adolescent/documents/9241546875/en/ (cited April 1, 2021).
10. Hategeka C, Mwai L, Tuyisenge L. Implementing the emergency triage, assessment and treatment plus admission care (ETAT+) clinical practice guidelines to improve quality of hospital care in Rwandan district hospitals: healthcare workers’ perspectives on relevance and challenges. BMC Health Serv Res. (2017) 17(1):256. doi: 10.1186/s12913-017-2193-4
11. Sigalet EL, Lufesi N, Dubrowski A, Haji F, Khan R, Grant D, et al. Simulation and active learning decreases training time of an emergency triage assessment and treatment course in pilot study in Malawi: implications for increasing efficiency and workforce capacity in low-resource settings. Pediatr Emerg Care. (2020) 12(37):e1259–64. doi: 10.1097/PEC.0000000000001996
12. Pellé KG, Rambaud-Althaus C, D'Acremont V, Moran G, Sampath R, Katz Z, et al. Electronic clinical decision support algorithms incorporating point-of-care diagnostic tests in low-resource settings: a target product profile. BMJ Global Health. (2020) 5(2):e002067. doi: 10.1136/bmjgh-2019-002067
13. Mehl G, Tunçalp Ö, Ratanaprayul N, Tamrat T, Barreix M, Lowrance D, et al. WHO SMART guidelines: optimising country-level use of guideline recommendations in the digital age. Lancet Digital Health. (2021) 3(4):e213–6. doi: 10.1016/S2589-7500(21)00038-8
14. Lee V, Dunsmuir D, Businge S, Tumusiime R, Karugaba J, Wiens MO, et al. Evaluation of a digital triage platform in Uganda: a quality improvement initiative to reduce the time to antibiotic administration. PloS one. (2020) 15(10):e0240092. doi: 10.1371/journal.pone.0240092
15. Collins GS, Reitsma JB, Altman DG, Moons KGM. Transparent reporting of a multivariable prediction model for individual prognosis or diagnosis (TRIPOD): the TRIPOD statement. BMC Med. (2015) 13(1):1. doi: 10.1186/s12916-014-0241-z
16. Mawji A, Li E, Komugisha C, Akech S, Dunsmuir D, Wiens MO, et al. Smart triage: triage and management of sepsis in children using the point-of-care pediatric rapid sepsis trigger (PRST) tool. BMC Health Serv Res. (2020) 20(1):493. doi: 10.1186/s12913-020-05344-w
17. Mawji A. Smart Triage Jinja Standard Operating Protocols, V1 [dataset]. (2021). Scholars Portal Dataverse. Available at: https://borealisdata.ca/dataset.xhtml?persistentId=doi: 10.5683/SP2/WLU0DJ (cited April 20, 2021).
18. Harris PA, Taylor R, Thielke R, Payne J, Gonzalez N, Conde JG. Research electronic data capture (REDCap) – A metadata-driven methodology and workflow process for providing translational research informatics support. J Biomed Inform. (2009) 42(2):377–81. doi: 10.1016/j.jbi.2008.08.010
19. Fleischmann-Struzek C, Goldfarb DM, Schlattmann P, et al. The global burden of paediatric and neonatal sepsis: a systematic review. Lancet Respir Med. (2018) 6:223–30. doi: 10.1016/S2213-2600(18)30063-8
20. Li E, Mawji A, Akech S, Chandna A, Kissoon N, Kortz T, Schlapbach LJ, Reinhart K, Kissoon N. Guidelines for the standardized collection of predictor variables in studies for pediatric sepsis (guidelines)∼Pediatric Sepsis Predictors Standardization (PS2) Working Group [dataset]. (2020). Scholars Portal Dataverse. Available at: https://dataverse.scholarsportal.info/dataset.xhtml?persistentId=doi: 10.5683/SP2/02LVVT. Referenced in doi: 10.5683/SP2/02LVVT (cited April 1, 2021).
21. Mawji A, Li E, Chandna A, Kortz T, Akech S, Wiens MO, et al. Common data elements for predictors of pediatric sepsis: a framework to standardize data collection. PLoS One. (2021) 16(6):e0253051. doi: 10.1371/journal.pone.0253051
22. BC Children’s Hospital Research Institute. Pediatric sepsis data colab. (2021). Available at: https://www.bcchr.ca/pediatric-sepsis-data-colab#:∼:text=The%20Pediatric%20Sepsis%20Data%20CoLaboratory,%2D%20and%20middle%2Dincome%20countries (cited April 1, 2021).
23. Fung JST, Akech S, Kissoon N, Wiens MO, English M, Ansermino JM. Determining predictors of sepsis at triage among children under 5 years of age in resource-limited settings: a modified delphi process. PloS One. (2019) 14(1):e0211274. doi: 10.1371/journal.pone.0211274
24. van Smeden M, Moons KGM, de Groot JAH, Collins GS, Altman DG, Eijkemans MJC, et al. Sample size for binary logistic prediction models: beyond events per variable criteria. Stat Methods Med Res. (2019) 28(8):2455–74. doi: 10.1177/0962280218784726
25. Tüshaus L, Moreo M, Zhang J, Hartinger SM, Mäusezahl D, Karlen W. Physiologically driven, altitude-adaptive model for the interpretation of pediatric oxygen saturation at altitudes above 2,000 m a.s.l. J Appl Physiol. (2019) 127(3):847–57. doi: 10.1152/japplphysiol.00478.2018
26. Zhou G, Karlen W, Brant R, Wiens M, Kissoon N, Ansermino JM. A transformation of oxygen saturation (the saturation virtual shunt) to improve clinical prediction model calibration and interpretation. Pediatr Res. (2019) 86(6):732–7. doi: 10.1038/s41390-019-0525-2
27. Pijyan A, Zheng Q, Hong HG, Li Y. Consistent estimation of generalized linear models with high dimensional predictors via stepwise regression. Entropy. (2020) 22(9):965. doi: 10.3390/e22090965
28. Steyerberg EW. Springerlink (online service). In SpringerLINK ebooks - mathematics and statistics. Clinical prediction models: A practical approach to development, validation, and updating, 2nd ed. Cham: Springer International Publishing (2019) p. 255–311.
29. Mawji A, Akech S, Mwaniki P, Dunsmuir D, Bone J, Wiens MO, et al. Derivation and internal validation of a data-driven prediction model to guide frontline health workers in triaging children under-five in Nairobi, Kenya. Wellcome Open Res. (2019) 4:121. doi: 10.12688/wellcomeopenres.15387.1
30. Raihana S, Dunsmuir D, Huda T, Zhou G, Rahman QS, Garde A, et al. Correction: development and internal validation of a predictive model including pulse oximetry for hospitalization of under-five children in Bangladesh. PloS One. (2016) 11(1):e0147560. doi: 10.1371/journal.pone.0147560
31. Harley A, Latour JM, Schlapbach LJ. The role of parental concerns in the recognition of sepsis in children: a literature review. Front Pediatr. (2019) 7:161. doi: 10.3389/fped.2019.00161
32. World Health Organization. Global strategy on digital health 2020–2025. Geneva: World Health Organization. (2020). Available at: https://www.who.int/docs/default-source/documents/gs4dhdaa2a9f352b0445bafbc79ca799dce4d.pdf (cited April 1, 2021).
33. Gondwe MJ, Henrion MYR, O'Byrne T, Masesa C, Lufesi N, Dube Q, et al. Clinical diagnosis in paediatric patients at urban primary health care facilities in southern Malawi: a longitudinal observational study. BMC Health Serv Res. (2021) 21(1):150. doi: 10.1186/s12913-021-06151-7
34. Bessat C, Zonon NA, D'Acremont V. Large-scale implementation of electronic integrated management of childhood illness (eIMCI) at the primary care level in Burkina Faso: a qualitative study on health worker perception of its medical content, usability and impact on antibiotic prescription and resistance. BMC Public Health. (2019) 19(1):449. doi: 10.1186/s12889-019-6692-6
35. Li ECK, Grays S, Tagoola A, Komugisha C, Nabweteme AM, Ansermino JM, et al. Cost-effectiveness analysis protocol of the smart triage program: a point-of-care digital triage platform for pediatric sepsis in eastern Uganda. PLoS One. (2021) 16(11):e0260044. doi: 10.1371/journal.pone.0260044
36. World Health Organization. Seventy-first world health assembly: digital health. Geneva: WHA (2018). Vol. 7, 71 p.
37. Ministry of Health, Republic of Uganda. Uganda National eHealth policy. Kampala (UG): Uganda Ministry of Health (2016). Available at: https://health.go.ug/sites/default/files/National%20eHealth%20Policy%202016_1.pdf (cited December 23, 2021).
38. World Health Organization. Global strategy on digital health 2020–2025. Geneva (CH): World Health Organization (2021). Available at: https://www.who.int/docs/default-source/documents/gs4dhdaa2a9f352b0445bafbc79ca799dce4d.pdf (cited Mar 24, 2022).
39. USAID's Digital Strategy Overview. U.S. Agency for International Development. (2021). Available at: https://www.usaid.gov/usaid-digital-strategy (cited June 10, 2022).
40. Pediatric Sepsis Data CoLaboratory. Pediatric sepsis data colaboratory membership application. (2021). Available at: https://rc.bcchr.ca/redcap/surveys/?s=EDCYL7AC79 (cited April 1, 2021).
Keywords: sepsis, triage, low-and-middle income countries, prediction, model, children, logistic regression
Citation: Mawji A, Li E, Dunsmuir D, Komugisha C, Novakowski SK, Wiens M, Vesuvius TA, Kissoon N and Ansermino JM (2022) Smart triage: Development of a rapid pediatric triage algorithm for use in low-and-middle income countries. Front. Pediatr. 10:976870. doi: 10.3389/fped.2022.976870
Received: 23 June 2022; Accepted: 1 November 2022;
Published: 22 November 2022.
Edited by:
Claudio Flauzino De Oliveira, Latin American Sepsis Institute, BrazilReviewed by:
Qalab Abbas, Aga Khan University, PakistanNesrin Alharthy, King Saud bin Abdulaziz University for Health Sciences, Saudi Arabia
© 2022 Mawji, Li, Dunsmuir, Komugisha, Novakowski, Wiens, Vesuvius, Kissoon and Ansermino. This is an open-access article distributed under the terms of the Creative Commons Attribution License (CC BY). The use, distribution or reproduction in other forums is permitted, provided the original author(s) and the copyright owner(s) are credited and that the original publication in this journal is cited, in accordance with accepted academic practice. No use, distribution or reproduction is permitted which does not comply with these terms.
*Correspondence: Alishah Mawji alishah5@student.ubc.ca
Specialty Section: This article was submitted to Pediatric Critical Care, a section of the journal Frontiers in Pediatrics