- Department of Pediatrics, The Second Affiliated Hospital of Harbin Medical University, Harbin, China
The role of N6-methyladenosine modification in immunity is increasingly being appreciated. However, the landscape of m6A regulators in juvenile idiopathic arthritis (JIA) is poorly understood. Thus, this study explored the impact of m6A modification and related lncRNAs in JIA immune microenvironment. Fourteen m6A regulators and eight lncRNAs were identified as potential diagnostic biomarkers for JIA. Two diagnostic models for JIA were also constructed. The putative molecular regulatory mechanism of FTO-mediated m6A modification in JIA was hypothesized. Three distinct m6A patterns mediated by 26 m6A regulators and three diverse lncRNA clusters mediated by 405 lncRNAs were thoroughly investigated. They exhibited dramatically diverse immune microenvironments and expression of HLA genes. The identification of two separate subtypes of enthesitis-related arthritis implies that our work may aid in the establishment of a more precise categorization system for JIA. m6A modification-related genes were obtained, and their underlying biological functions were explored. The m6Ascore system developed for individual JIA patients may be utilized to evaluate the immunological state or molecular pattern, thereby offering therapy recommendations. In short, through the investigation of the m6A regulators in JIA, the current work may contribute to our knowledge of the pathophysiology of JIA.
Introduction
Juvenile idiopathic arthritis (JIA) is defined as unexplained joint swelling and pain that lasts longer than 6 weeks in childhood (under 16 years of age) (1). JIA is the most frequent rheumatological chronic inflammatory illness in children, characterized by chronic synovitis and systemic multi-organ dysfunction. Neither the etiology nor the pathogenesis of JIA is clear. Furthermore, while JIA is a collective name for a set of disorders, their pathophysiology may differ. It is now believed that JIA is the consequence of environmental exposure in genetically susceptible individuals, and there is the involvement of infection, genetics, epigenetics, immunity, and other factors (2). Multiple hypotheses have been proposed to explain the pathophysiology of JIA. For instance, the specific components of various infectious microorganisms act as foreign antigens to genetically susceptible children, activating immune cells and inflicting damage and degeneration to self-tissues either directly or indirectly through the secretion of cytokines and autoantibodies that promote abnormal immune responses. Many studies have confirmed that JIA has a genetic basis, with human leukocyte antigens (HLA) being the most extensively examined. Individuals with the HLA-DR4, HLA-DR5, and HLA-DR8 alleles are predisposed to JIA (3). JIA was formerly known as juvenile rheumatoid arthritis (JRA) by the American College of Rheumatology (ACR) and juvenile chronic arthritis (JCA) by the European Alliance of Associations for Rheumatology (EULAR). Not only were the names dissimilar, but they were also classified in different ways. It was not until 2001 that the International League Against Rheumatism (ILAR) defined a common classification standard for JIA (1). Although the ILAR categorization system has made a substantial contribution to JIA research, the limitations of this classification have been more evident in recent years (4). A more sophisticated categorization system for JIA based on pathogenesis and molecular biology is urgently required. Modern diagnostic methods for JIA rely heavily on clinical signs and the exclusion of other similar diseases. At this time, there are also no laboratory tests that can be used to diagnose JIA. As modern medicine advances by leaps and bounds, the prognosis of JIA has improved dramatically. However, certain children are afflicted with severe complications such as loss of joint function, lifelong eye damage owing to uveitis, and macrophage activation syndrome (MAS), which may be fatal (5, 6). Therefore, effective early diagnostic biomarkers or models of JIA are highly sought after.
Ribonucleic acid modification (RNA) is regarded as the third layer of epigenetics, and its role in biology is becoming increasingly appreciated (7). It has been shown that RNA has more than 100 distinct forms of post-synthesis modifications, including N6-methyladenosine (m6A), 5-methylcytosine (m5C), N1-methyladenosine (m1A), 3-methylcytosine (m3C), and N1-methylguanosine (m1G) (8). m6A is the most common post-transcriptional modification in eukaryotic cells, where it regulates the transcription, processing, splicing, degradation, and translation of RNA (9). m6A modifies and regulates RNA through dynamic interactions between the three homologous components, including methyltransferases (writers), binding proteins (readers), and demethylases (erasers) (10). m6A RNA methylation modification has been confirmed to play a pivotal role in a wide range of diseases, including cancers, immune-related illnesses, and neurodegenerative diseases (11–13). It has been demonstrated that m6A modification and m6A regulator proteins play prominent roles in regulating both innate and adaptive immunity (14). A study indicates that hnRNPA2B1 detects herpesvirus DNA, thereby increasing m6A modification to elicit innate immune responses (15). In mouse T cells, the deficiency of METTL3 impairs the differentiation and homeostasis of T cells (16). CD4+ regulatory T cells (Tregs) without m6A RNA modification were also reported to lose their systematic suppressive effect against immune cells (17). Additionally, m6A is crucial for the activation of dendritic cells (DCs), which produce co-stimulatory molecules and promote the activation of adaptive immune responses through antigen cross-presentation (14). Non-coding RNA is thought to be the foundation of epigenetic regulation (18). Long non-coding ribonucleic acids (lncRNAs) are characterized as transcripts with a length exceeding 200 nucleotides that do not undergo protein translation (19). lncRNAs have been proven to be pivotal regulators of mRNA processing, post-transcriptional regulation, and protein activity (20). There is a substantial correlation between m6A and lncRNAs. On the one hand, m6A modifications can affect the structure of the RNA-DNA triple helix, thereby regulating the association of lncRNAs with specific DNA loci; on the other hand, m6A modifications can also create binding sites for binding proteins or modify the structure of local RNAs, which in turn induce the binding of RNA-binding proteins (RBPs) to influence the function of lncRNAs (21, 22). Additionally, lncRNAs contain more m6A modification sites than mRNAs do (23). 5-methylcytosine (m5C) is another frequent post-transcriptional modification of RNA (24). m5C performs similar functions to m6A, and the methylation of m5C also requires the involvement of writers, erasers, and readers. However, it’s not clear how these modifications work in juvenile idiopathic arthritis.
This research was based on gene expression profiles of peripheral blood mononuclear cells (PBMCs) from JIA children and healthy controls. We systematically analyzed the molecular patterns mediated by m6A regulators and m6A-related lncRNAs, respectively. We undertook detailed assessments of the differences in biological function and immunological microenvironment across these patterns, as well as putative molecular explanations. Fourteen m6A regulators and eight lncRNAs were identified to be crucial for JIA diagnosis. They may serve as potential biomarkers for JIA diagnosis. Two diagnostic models were developed utilizing m6A regulators and lncRNAs, respectively. Furthermore, two models were tested using the same external dataset and then compared against one another to identify the superior model. Additionally, genes influencing the formation of distinct m6A patterns were identified, and their potential biological functions were elucidated. Two distinct patterns in enthesitis-related arthritis (ERA) were observed in our study, indicating that there may be two separate subtypes with distinct pathogenesis and progression in ERA. This also suggests that m6A regulators and lncRNAs may assist in establishing the novel JIA classification system. Finally, taking into account the individual heterogeneity of m6A modifications, the m6Ascore system was designed to estimate the m6A modification status of individual JIA patients.
Materials and methods
Microarray data collection
The analysis of this study was mainly based on five datasets from the GEO database1. All five datasets (GSE11083, GSE13501, GSE20307, GSE21521, and GSE67596) were produced using the same microarray platform of GPL570 (Affymetrix Human Genome U133 Plus 2.0 Array). This guaranteed the reliability of the merged analysis. Following the removal of duplicates, 196 JIA samples and 89 healthy samples were identified. Raw CEL files were extracted from the GEO supplementary files and assembled into a single dataset. The background adjustment and normalization were implemented using the rma function in the Bioconductor package affy (25). Non-biological batch effects among five datasets were eliminated using the ComBat algorithm (26). Probes without annotation information were removed. As for more than one probe corresponding to the same gene symbol, the median value was taken as the gene expression value. As an additional validation, we collected another independent dataset (GSE15645) to test the two diagnostic models we developed in this study.
Different expression of m6A regulators between juvenile idiopathic arthritis and healthy controls
Pearson correlation analysis was executed in order to examine the co-expression patterns of 26 m6A RNA methylation regulators in all samples and just within JIA samples. Based on the Wilcox test, we systematically evaluated the different expressions of 26 m6A regulators between JIA samples and healthy control samples. All 26 m6A regulators were separately analyzed using univariate logistic regression to recognize JIA diagnosis-related m6A regulators (p < 0.05). The m6A regulators picked by univariate logistic regression were then submitted to LASSO (Least Absolute Shrinkage and Selection Operator) regression for dimensionality reduction analysis, and 10-fold cross-validation was applied. Following LASSO’s selection of m6A regulators, a diagnosis model for JIA was developed through multivariate logistic regression. Receiver operating characteristic (ROC) curves and the area under the curve (AUC) were used to estimate the prediction power of the JIA model.
Three molecular patterns mediated by 26 m6A regulators
To observe the different m6A patterns in JIA samples, unsupervised hierarchical clustering analysis was employed. On the basis of the gene expression of 26 m6A regulators, we executed unsupervised consensus clustering with 1,000 replications using the ConsensusClusterPlus R package (27). According to published literature and the R package reference manual, the optimal number of clusters was calculated using the K value with the lowest proportion of ambiguously clustered pairs (PAC) (28). Gene set variation analysis (GSVA) was conducted to evaluate biological function and progress variations among these m6A patterns (29). The KEGG gene set (c2.cp.kegg.v7.5.1.symbols.gmt) from MSigDB was downloaded for running GSVA. Statistical significance was determined only for pathways with an adjusted p-value less than 0.05. Single-sample gene set enrichment analysis (ssGSEA) was used to assess the infiltration status of 23 immune cell types and immune reactions among m6A patterns. The gene signatures of each immune cell type were obtained from prior research (30). Seventeen immune reactions related gene sets were downloaded from the ImmPort database2 (31). The relationship between HLA and JIA has been extensively studied. Here we also examined the differences in HLA expression among m6A patterns. All tests were Wilcox unless otherwise noted.
Identification of m6A modification-related genes
Genes that show significant differences in expression between different m6A patterns were identified using the limma R package (32). Specifically, genes with | Log2FC (fold-change)| > 1 and adjusted p-value < 0.05 were considered as DEGs. To gain a deeper insight into the biological functions of m6A modification-related genes, Gene Ontology (GO) and Kyoto Encyclopedia of Genes and Genomes (KEGG) enrichment analysis were conducted with the clusterProfiler R package (33). Using weighted gene correlation network analysis (WGCNA), we identified gene modules associated with different patterns to obtain the feature genes of each molecular pattern mediated by the m6A regulators (34).
m6A- and m5C-related long non-coding ribonucleic acids
Pearson correlation analysis was used to assess the expression correlation between lncRNAs and m6A regulators. We defined m6A-related lncRNAs as those whose expression value was correlated with at least one of the 26 m6A regulators (| correlation coefficients| > 0.4 and p < 0.001). The same process was used to find m5C-related lncRNAs. Next, we considered lncRNAs that overlapped in both m6A-related lncRNAs and m5C-related lncRNAs as m6A- and m5C-related lncRNAs. Unsupervised clustering analysis was performed to explore the potential biological functions of m6A- and m5C-related lncRNAs in JIA. Using the same analysis process as described above, the degree of immune cells infiltration and immune reactions, as well as the HLA expression relationship were measured. The LASSO regression algorithm was then employed to screen important lncRNAs that could accurately discriminate JIA samples from normal samples. Using multivariate logistic regression, an additional diagnosis model was constructed based on lncRNA signatures. We compared the diagnostic capabilities of both models in order to select the model with the best performance.
m6Ascore: Construction and application
Taking into consideration the individual phenotypic variation and intricacy of m6A modification, a scoring system was constructed to assess the m6A modification panorama of individual JIA patients based on the m6A modification-related genes. All samples were subjected to principal component analysis (PCA) of the m6A modification-related genes expression profiles. Summing the PC1 and PC2 scores of each sample yields the m6Ascore for the sample. m6Ascore was compared between the different patterns. JIA children were divided into two groups based on low or high m6Ascore. Further assessment was made of the differences in immune microenvironment between patients with low and high m6Ascore.
Results
The landscape of m6A regulators in juvenile idiopathic arthritis
In a review of published studies, 26 m6A regulators were identified, including nine writers, three erasers, and fourteen readers (Supplementary Table 1). The co-expression network between m6A regulators was first investigated (Figure 1A). There was a broad and notable correlation observed among the expression of m6A regulators. In all samples, there were two erasers (FTO and ALKBH5) that were the most relevant m6A regulators in expression, while in only JIA samples, they were two readers (YTHDC1 and RBMX). These results suggest they could function synergistically during the course of JIA. YTHDF1 was the m6A regulator with the biggest fold change and the most pronounced statistical significance in JIA samples compared to healthy samples, suggesting that it may play an indispensable role in JIA pathogenesis (Figure 1B). Each type of m6A regulator had a unique regulator that behaved differently from the others. Other writers exhibited a positive relationship with erasers and readers, as opposed to METTL16, which had a broad negative relationship. Similarly, ALKBH1 did not show a positive correlation with other m6A regulators as did other erasers, but rather a negative correlation. The same goes for ELAVL1. The results above indicated that m6A regulators mediate an intricate network of RNA methylation modifications in JIA. There were prominent differences in m6A regulators expression between JIA and healthy samples (except for METTL14 and ZC3H13), and JIA generally had higher grades of m6A regulators expression (Figures 1C, 2A). Significant differences were observed between JIA and healthy samples in the immune cell infiltration (Figure 2B). Notably, the ratio of CD4 T cell to CD8 T cell was dramatically higher in JIA patients than in healthy controls, indicating that JIA was an autoimmune disease. However, no statistically significant change in the regulatory T (Treg) cell was observed, suggesting that the Treg cell may not be implicated in the etiology of JIA. This finding has been highlighted in previous research and was further supported here (35). Notably, type 2 T helper cell (Th2) was active in JIA, and their release of numerous cytokines could directly affect the inflammatory process in JIA. We hypothesized that the high level of eosinophils in JIA released a large amount of interleukin 4 (IL-4), which could induce the differentiation of Th0 cells to Th2 cells.
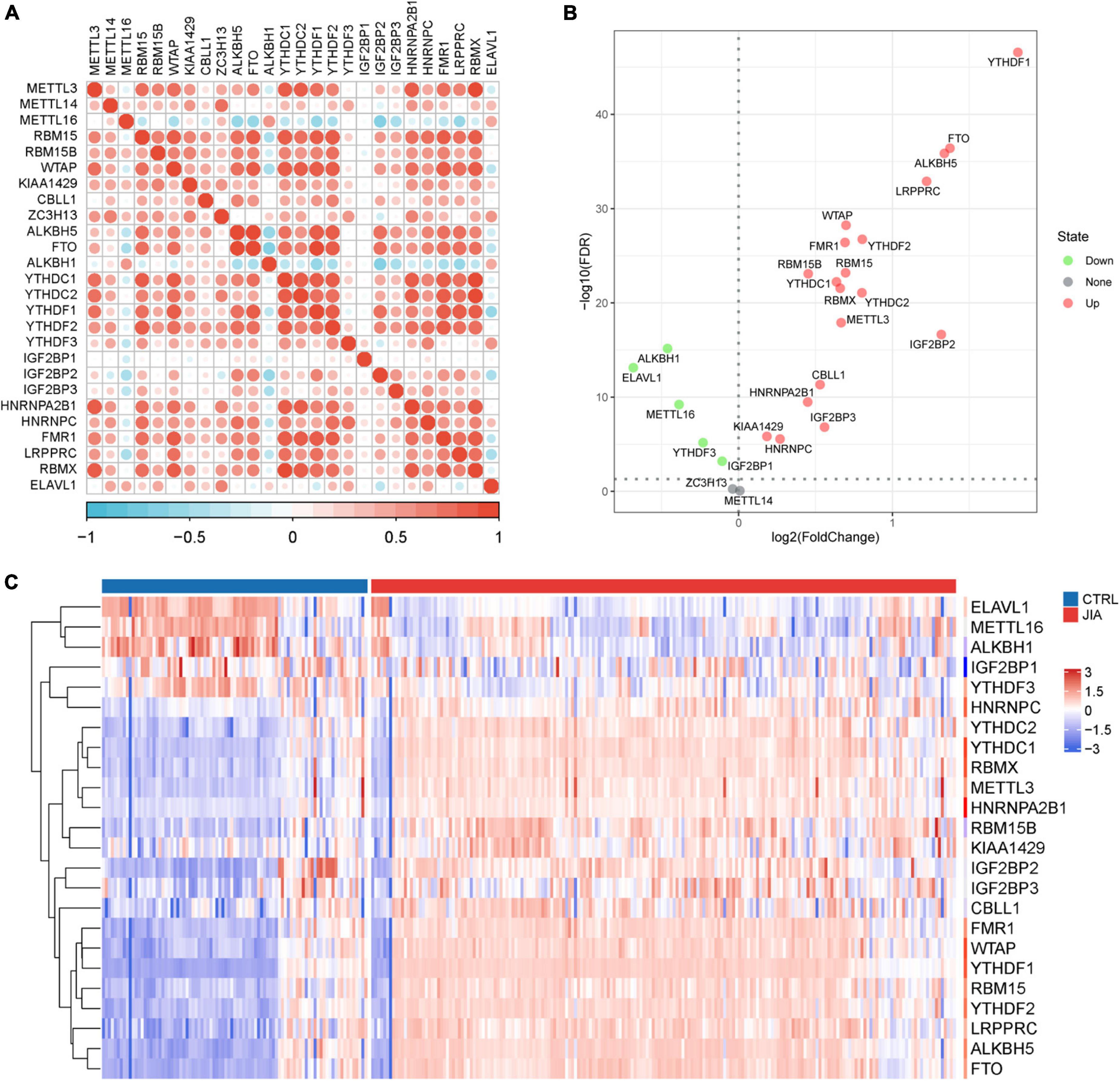
Figure 1. The landscape of m6A regulators in juvenile idiopathic arthritis (JIA). (A) The co-expression network of 26 m6A regulators. The upper triangle is the network in all samples, and the lower triangle is the network in just JIA samples. (B) The volcano plot shows the differential expression of m6A regulators between JIA and healthy controls samples. (C) The heatmap plot of 24 significantly differentially expressed m6A regulators.
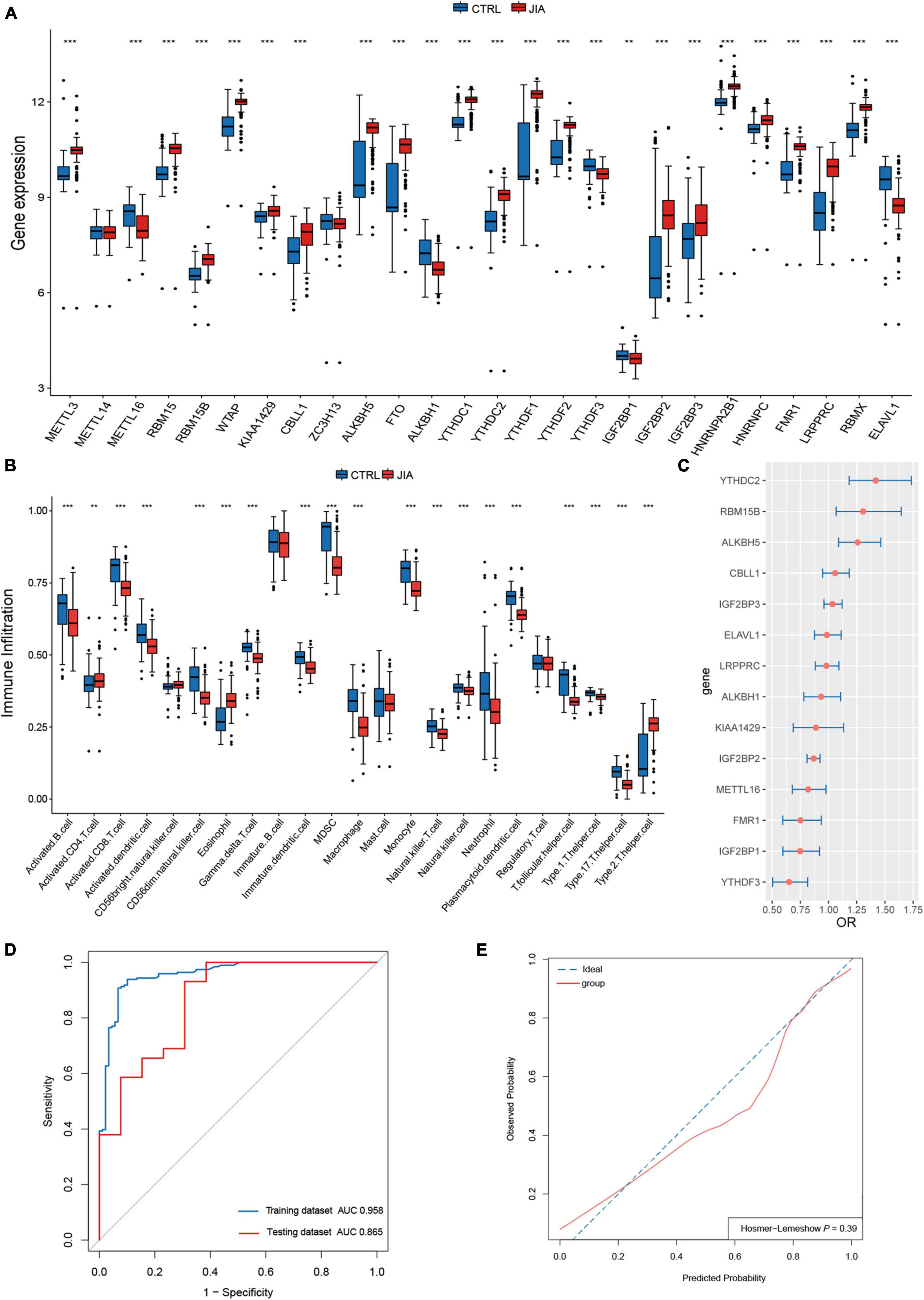
Figure 2. A diagnostic model was constructed with m6A regulators for juvenile idiopathic arthritis (JIA). (A) Comparison of the expression of 26 m6A regulators in JIA and normal control samples. (B) The discrepancies in the abundance of each infiltrating immunocyte between JIA and healthy samples. (C) Relative risks of 14 diagnostic m6A regulators for JIA were calculated. (D) The developed diagnostic model for JIA was evaluated by the ROC curve and AUC value. (E) Hosmer–Lemeshow (H–L) test was used to evaluate the fit of the developed model.
A diagnostic model constructed with m6A regulators for juvenile idiopathic arthritis
Given the noteworthy expression difference of m6A regulators between JIA and normal samples, we considered m6A regulators for JIA diagnosis. The construction of the diagnostic model also provided us with a better knowledge of the role of m6A regulators in JIA. The first step was to identify 24 m6A regulators associated with JIA diagnosis through univariate logistic regression analysis. It turns out that these 24 m6A regulators are the same as the differentially expressed m6A regulators from the previous step. LASSO regression analysis with 10-fold cross-validation was employed to filter out m6A regulators that were relatively insignificant to the diagnosis of JIA. Finally, fourteen m6A regulators (METTL16, RBM15B, KIAA1429, CBLL1, ALKBH5, ALKBH1, YTHDC2, YTHDF3, IGF2BP1, IGF2BP2, IGF2BP3, FMR1, LRPPRC, and ELAVL1) crucial to diagnosing JIA were obtained. Multivariate logistic regression analysis was performed to construct the diagnostic model of JIA with these 14 m6A regulators as variables. In addition, the odds ratio (OR) with a 95% confidence interval (CI) of each variable was calculated (Figure 2C). By tracing the ROC curve of the model, we could see that it was well suited to distinguish JIA from healthy samples (Figures 2D,E). The concordance index (C-index) of this model was 0.96 (95% CI 0.93–0.98), and the area under the curve (AUC) was 0.958 (95% CI 0.933–0.982). The diagnostic model was validated using the independent dataset GSE15645 to evaluate its reliability against an external independent dataset. The AUC value of the validation dataset was 0.865 (95% CI 0.739–0.991). The external validation result indicated that the derived model had a fair prediction capacity against an external dataset.
Identification of distinct m6A patterns in juvenile idiopathic arthritis
Unsupervised clustering analysis of JIA samples based on the 26 m6A regulators transcripts was used to examine the multiple m6A patterns in JIA. A total of three distinct m6A patterns were identified, with seven samples falling into pattern A, 109 samples falling into pattern B, and 80 samples falling into pattern C (Figure 3A). The three m6A patterns discovered were inconsistent with the current clinical categorization system that focused primarily on patient symptoms (Figure 3B). Enthesitis-related arthritis (ERA) samples were observed to be split into two m6A patterns: 7 cases in pattern A and 22 cases in pattern B, indicating that two different molecular environments may characterize ERA. Each of the 26 m6A regulators showed considerable variation across these three m6A patterns, demonstrating the presence of various molecular patterns in JIA (Figure 3C). Pattern A generally showed lower expression of m6A regulators than pattern B or C, with a handful of exceptions (ZC3H13, ALKBH1, YTHDF3, ELAVL1) showing strongly high-expressed.
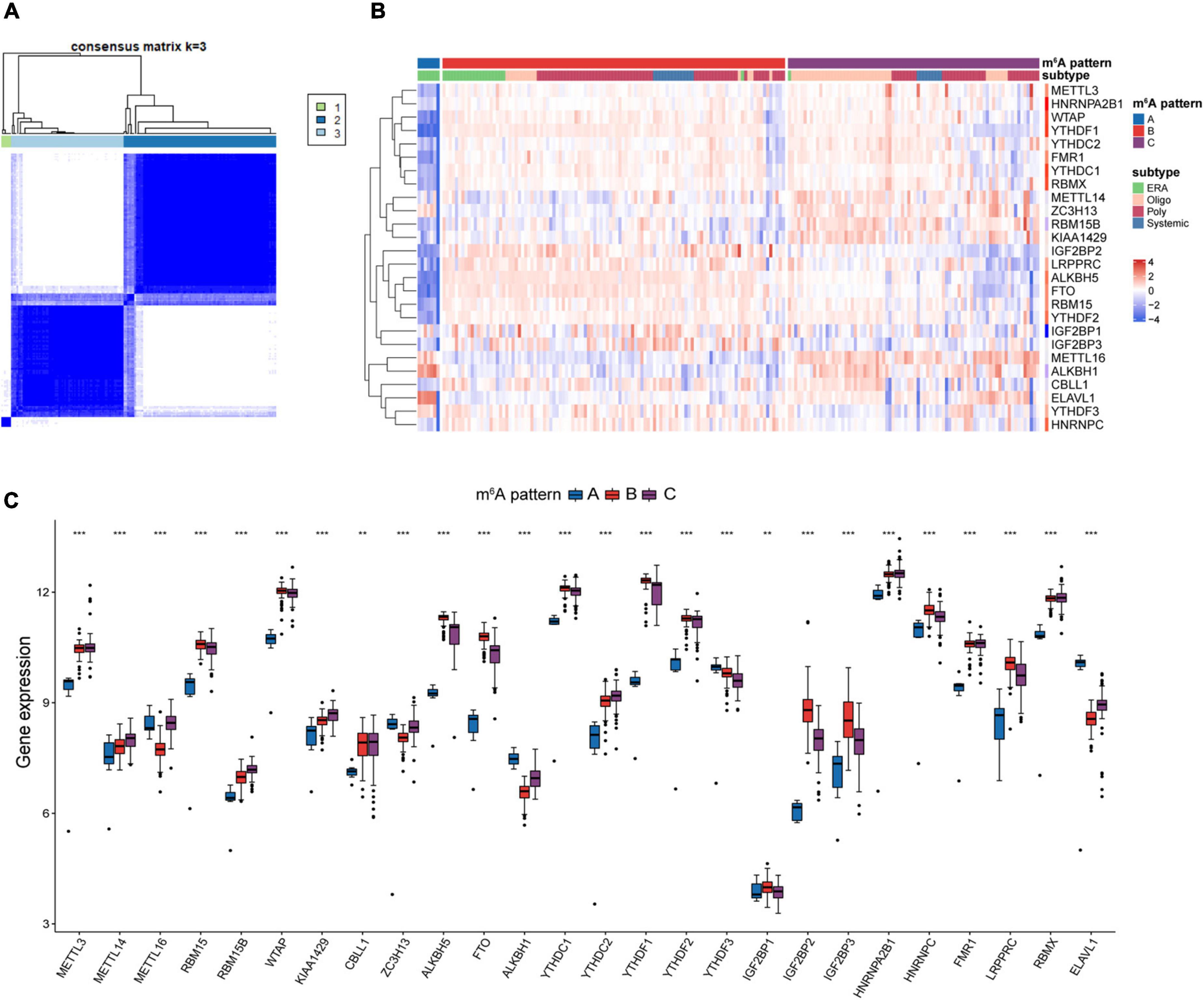
Figure 3. Unsupervised clustering analysis of 26 m6A regulators. (A) Consensus clustering classified juvenile idiopathic arthritis (JIA) into three m6A patterns. (B) Differences between the m6A patterns and extant classification system. (C) Different expressions of the m6A regulators in the three m6A patterns.
Divergent immune microenvironments in juvenile idiopathic arthritis
To investigate the biological differences among these three m6A patterns, we employed GSVA enrichment analysis to explore the KEGG pathways associated with each pattern. Compared with patterns B and C, pattern A was mainly enriched in the activation of immune related-pathways such as antigen processing and presentation, natural killer cell-mediated cytotoxicity, the intestinal immune network for IgA production, cytokine-cytokine receptor interaction, chemokine signaling pathway, and RIG I like receptor signaling pathway (Figures 4A,B). Additionally, matrix-related pathways such as ECM-receptor interaction and cell adhesion molecules (CAMs) could be discovered in pattern A. Pattern B revealed a slew of signaling pathways, including Notch signaling pathway, adipocytokine signaling pathway, Wnt signaling pathway, ErbB signaling pathway, p53 signaling pathway, Calcium signaling pathway, and MAPK signaling pathway (Figures 4A,C). It was also discovered that pattern A was linked to focal adhesion, and pattern B was connected with the regulation of actin cytoskeleton. Focal adhesion is the physical link between the cell actin cytoskeleton and extracellular matrix (ECM). Macrophage activation syndrome (MAS) is the most dangerous complication of JIA. Genome-Wide Association Studies (GWAS) investigations reveal that the aberrations in cellular cytotoxicity as the key disfunction in MAS may be caused by derangements in cellular assembly and cytoskeletal organization. Therefore, we speculated that additional research into the cytoskeleton would aid in our understanding of the molecular processes driving JIA development. In comparison to pattern B, pattern C also exhibited immune-related pathways enrichment. Ubiquitin-mediated proteolysis was more prevalent in pattern C than in pattern A, indicating that ubiquitination modifications may have a place in pattern C. A number of autoimmune disorders, malignancies, and neurodegenerative diseases were also identified in the enrichment analysis, suggesting that m6A modification may also participate in their pathogenesis. Many published studies have proved the association (36–38).
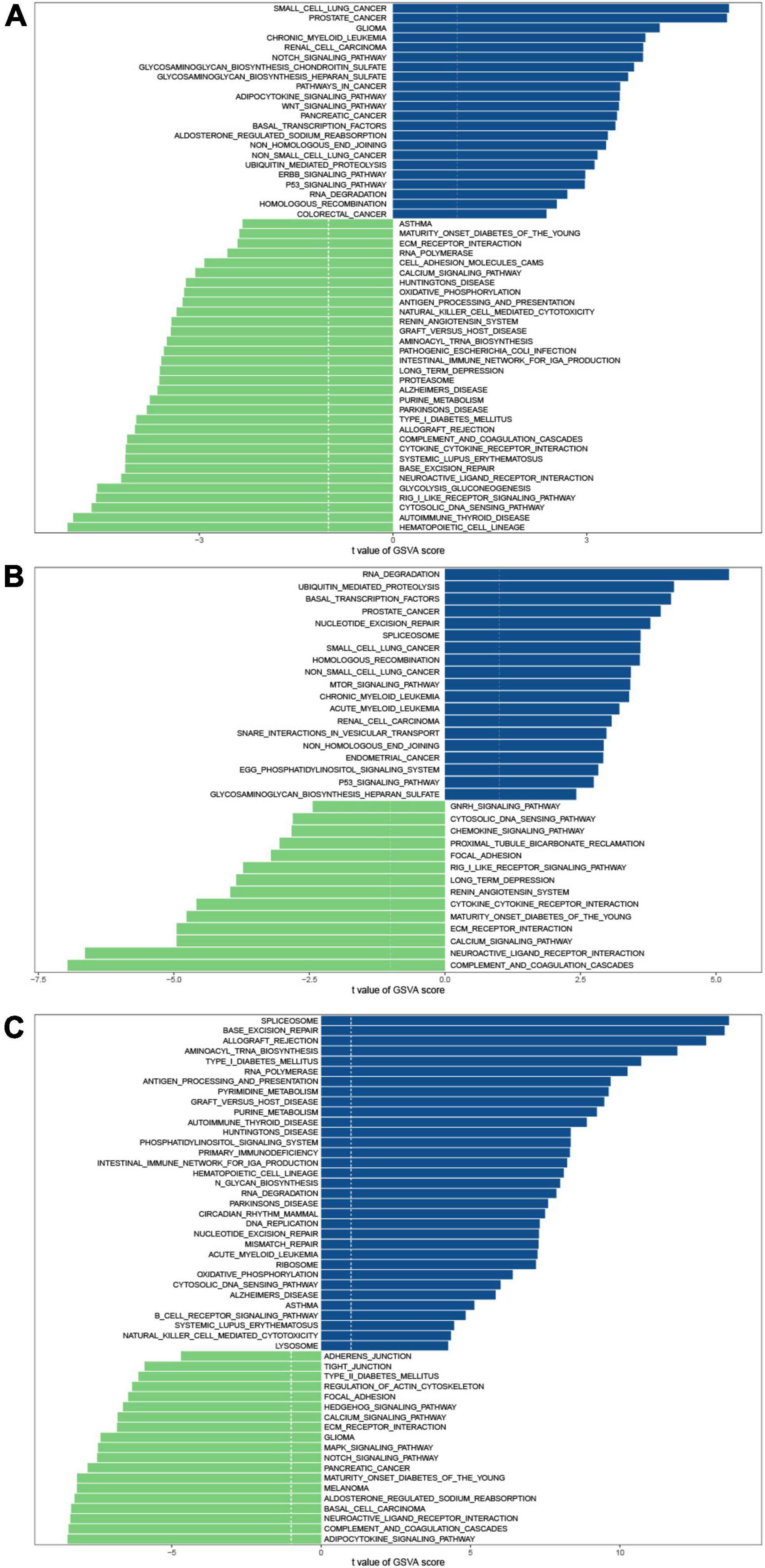
Figure 4. GSVA enrichment analysis shows the differences in the underlying biological processes between the three m6A patterns. (A) Pattern B vs. Pattern A. (B) Pattern C vs. Pattern A. (C) Pattern C vs. Pattern B.
Due to the substantial association between the three patterns and immunity, we analyzed their variations in immune cells infiltrating, immune response processes, and HLA genes expression to recognize the immune microenvironment landscapes of three m6A patterns. In comparison to patterns B and C, pattern A exhibited a higher overall infiltration of immune cells (Figure 5A). Autoantibodies are often detected in JIA patients, and many studies have sought to determine the role of autoantibodies in JIA. Despite the fact that autoantibodies for JIA have not been found to be diagnostic, they have been proven to assist in assessing the severity of the condition. The Childhood Arthritis and Rheumatology Research Alliance (CARRA) has identified rheumatoid factor (RF) as a risk factor for poor prognosis in individuals with polyarticular JIA (39). Given the greater infiltration of activated B cells in pattern A compared to the other two patterns, it is hypothesized that autoantibodies were more likely to be detected in pattern A. Subsequent immune response analysis demonstrated pattern A exhibited more robust immunological responses, particularly in the humoral immunity (Figure 5B). Pattern A exhibited more activity in antigen processing and presentation, BCR signaling pathway, chemokines, chemokines receptors, cytokines, cytokine receptors, and natural killer cell cytotoxicity. Pattern B only exceeded the other patterns at the level of eosinophils and Th2 cells infiltration. On the other hand, Pattern A had the lowest amounts of eosinophils and Th2 cells infiltration. The co-expression of eosinophils and Th2 may validate our previous hypothesis that eosinophils regulated Th2 expression through releasing IL4. The infiltration degree of each immune cell in pattern C was mostly moderate. As for the immune reaction, pattern C also exhibited a moderate degree, whereas pattern B exhibited the least. There was no significant difference in activated CD4 T cell infiltration across three patterns, indicating that activated CD4 T cell was irreplaceable in all three m6A patterns. Similar trends can be detected in the expression of the HLA genes. The expression of HLA was generally higher in pattern A than in the other two patterns (Figure 5C). HLA gene expression varied significantly between the three m6A patterns, hinting this characteristic could serve as a criterion for the new categorization of JIA.
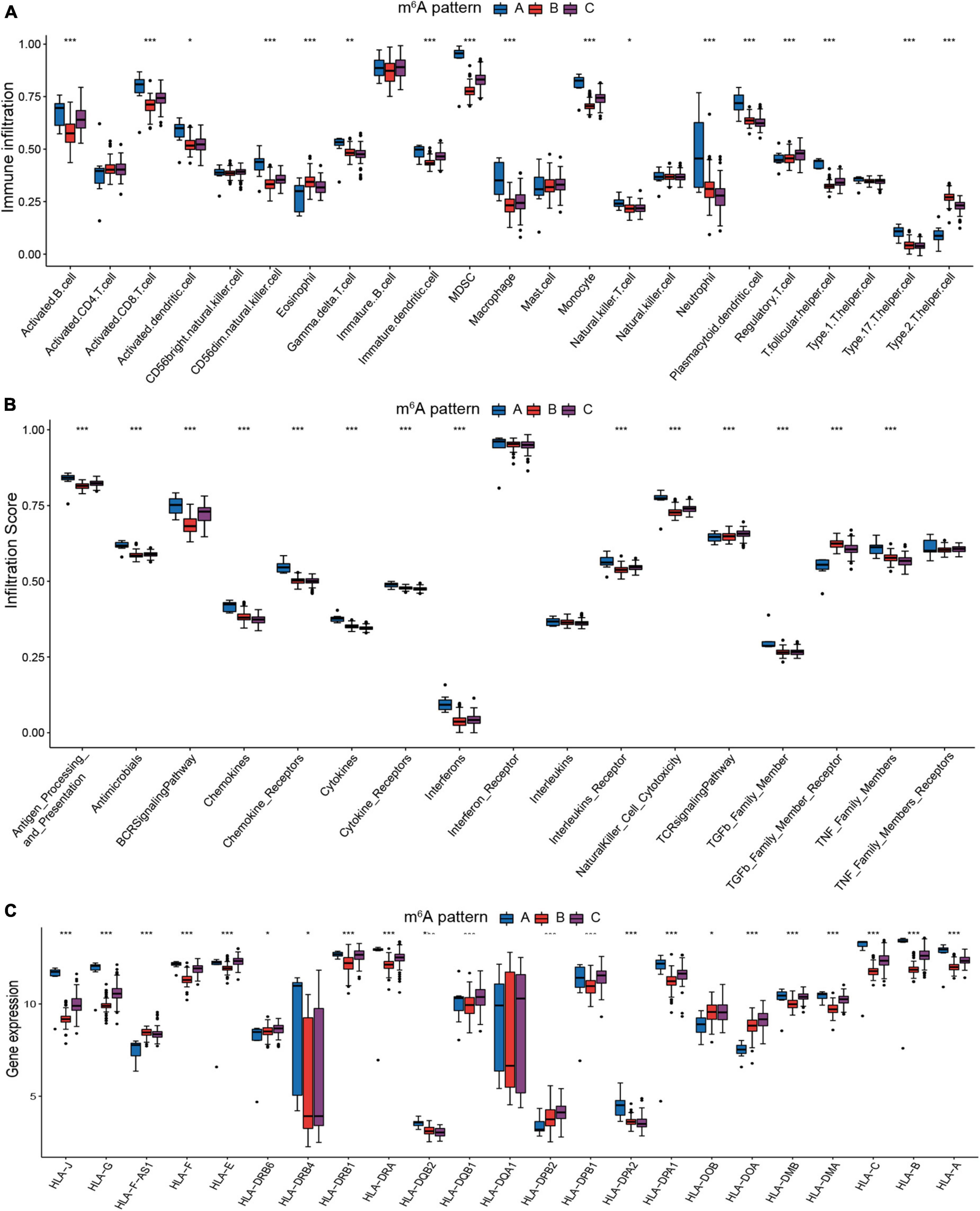
Figure 5. The immunological microenvironment varies significantly across the three m6A patterns. (A) The discrepancies in the abundance of each infiltrating immunocyte in the three m6A patterns. (B) The three patterns exhibit varying degrees of immunological response. (C) Human leukocyte antigens (HLA) gene expression varied significantly across the three m6A patterns.
FTO-mediated regulations of immune microenvironment in juvenile idiopathic arthritis
In addition, we checked for the correlation between each immune cell type and each m6A regulator using Spearman correlation analysis (Figure 6A). Here, we concentrated on FTO, which showed a considerable negative connection with various immune cells. FTO was the first m6A demethylase to be identified (40). The identification of FTO demonstrated that m6A modification is dynamic and reversible. To assess immunological disparities between JIA patients with high and low expression of FTO, JIA patients were split into two groups based on the expression level of FTO, using the mean value as a threshold. GSVA enrichment pathways demonstrated that patients with reduced FTO expression were associated with immunological activation and m6A-related regulatory mechanisms, such as antigen processing and presentation, natural killer cell mediated cytotoxicity, IgA production, DNA replication, mismatch repair, spliceosome, and aminoacyl-tRNA biosynthesis (Figure 6B). Further comparative analysis of immune cell infiltration in two groups revealed a significant increase in patients with low expression of FTO (Figure 6C). Notably, highly expressed activated dendritic cells were observed in patients with low expression of FTO. Activation of dendritic cells (DCs) requires the involvement of major histocompatibility complex (MHC), co-stimulatory molecules, cytokines, and chemokine receptors (41). Then we investigated the interaction between FTO and these molecules to learn how the expression of FTO affected DCs activation. The result showed that patients with low-expressed FTO exhibited significantly higher expression of co-stimulatory molecules, adhesion molecules, and MHC (Figure 6D). From the above results, we hypothesized that FTO-mediated m6A methylation modification might stimulate the production of MHC and co-stimulatory molecules and consequently activate conventional dendritic cells, enhancing the adaptive immune reaction in JIA patients.
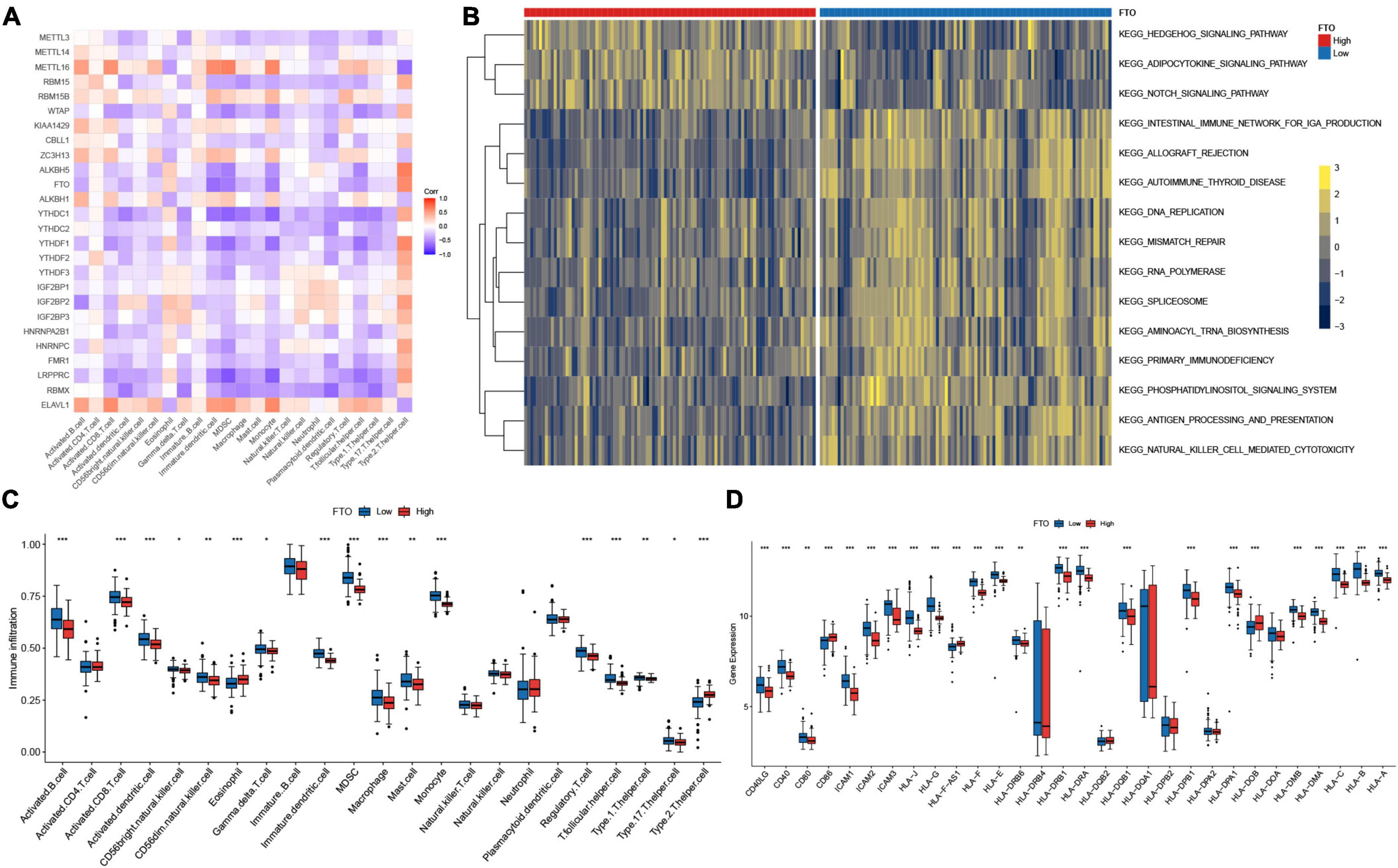
Figure 6. FTO-mediated regulations of the immune microenvironment in juvenile idiopathic arthritis (JIA). (A) Correlations between m6A regulators and the immunocytes. (B) GSVA enrichment analysis shows the differences in the underlying biological processes between the JIA patients with high and low expression of FTO. (C) The discrepancies in the abundance of each infiltrating immunocyte between the JIA patients with high and low expression of FTO. (D) Patients with low-expressed FTO exhibited significantly higher expression of co-stimulatory molecules, adhesion molecules, and MHC.
m6A modification-related genes and weighted gene correlation network analysis
To gain further insight into the mechanism underlying the formation of different molecular patterns, differentially expressed genes among the three m6A patterns were identified. The union of three DEGs sets was defined as the m6A modification-related genes. A total of 6919 m6A modification-related genes were obtained. The biological processes of GO enrichment analysis demonstrated that the genes were remarkably enriched in m6A-related regulatory mechanisms and immunity (Figure 7A). Similar results were noted in the KEGG pathway analysis (Figure 7B). Gene modules linked with diverse molecular patterns were identified using WGCNA. In total, nine gene modules were obtained (Figure 7C). For each m6A pattern, there were several modules substantially associated with it (Figure 7D). These gene modules may shed light on the m6A modification patterns from a more refined genetic perspective.
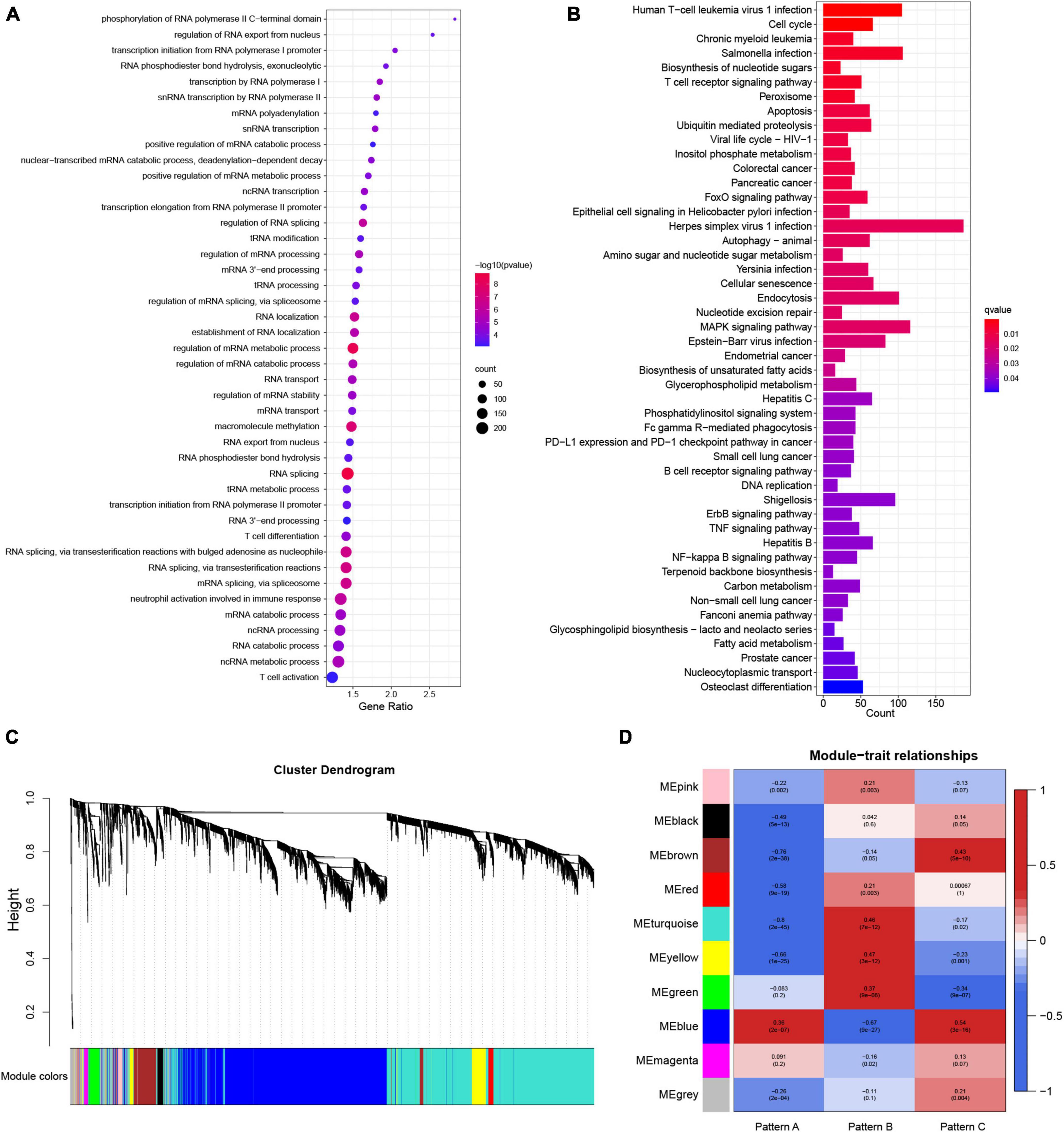
Figure 7. The underlying biological processes of m6A modification-related genes and weighted gene correlation network analysis (WGCNA) of the top 25% variation genes. (A) Partial results of Gene Ontology (GO) enrichment analysis for m6A modification-related genes in terms of biological processes (BP). (B) The Kyoto Encyclopedia of Genes and Genomes (KEGG) pathway analysis of m6A modification-related genes. (C) Identified modules by WGCNA. (D) Relationships between modules and traits.
m6A- and m5C-related long non-coding ribonucleic acids
Our attention has been drawn to the fact that lncRNAs have more m6A modification sites than mRNAs (23). m5C modification is another frequently occurring RNA methylation modification in humans. To get a better understanding of how m6A regulators regulate the immunological microenvironment in JIA and the intricate lncRNAs regulatory network generated by m6A and m5C regulators, the m6A- and m5C-related lncRNAs were identified in this study. On the basis of published data, 14 m5C regulators were identified, including ten writers, three erasers, and one reader (Supplementary Table 2). The Pearson correlation analysis revealed 500 m6A-related lncRNAs and 442 m5C-related lncRNAs. Eventually, a total of 405 m6A- and m5C-related lncRNAs were identified. Given the excellent performance of the diagnostic model for JIA conducted using the above 14 m6A regulators, we investigated if m6A- and m5C-related lncRNAs were also capable of diagnosing JIA. LASSO regression analysis with 10-fold cross-validation was performed to identify lncRNAs essential for JIA diagnosis. Finally, we collected eight lncRNAs (ZNF582-AS1, ZNF503-AS2, UBL7-AS1, TTTY5, PRKAG2-AS1, LINC01007, LINC00424, and ADARB2-AS1) for use in the development of the diagnostic model. The diagnostic model for JIA, which was based on the expression of eight lncRNAs, was developed using the same multivariate logistic regression methodology as previously described. The calculated AUC was 0.952 (95% CI 0.925–0.978). The model was further tested against the independent dataset GSE15645, and the AUC was 0.737 (95% CI 0.572–0.903) (Figure 8A). The comparison of AUC values indicated that the diagnostic model developed using m6A regulators was superior to the model constructed using lncRNAs.
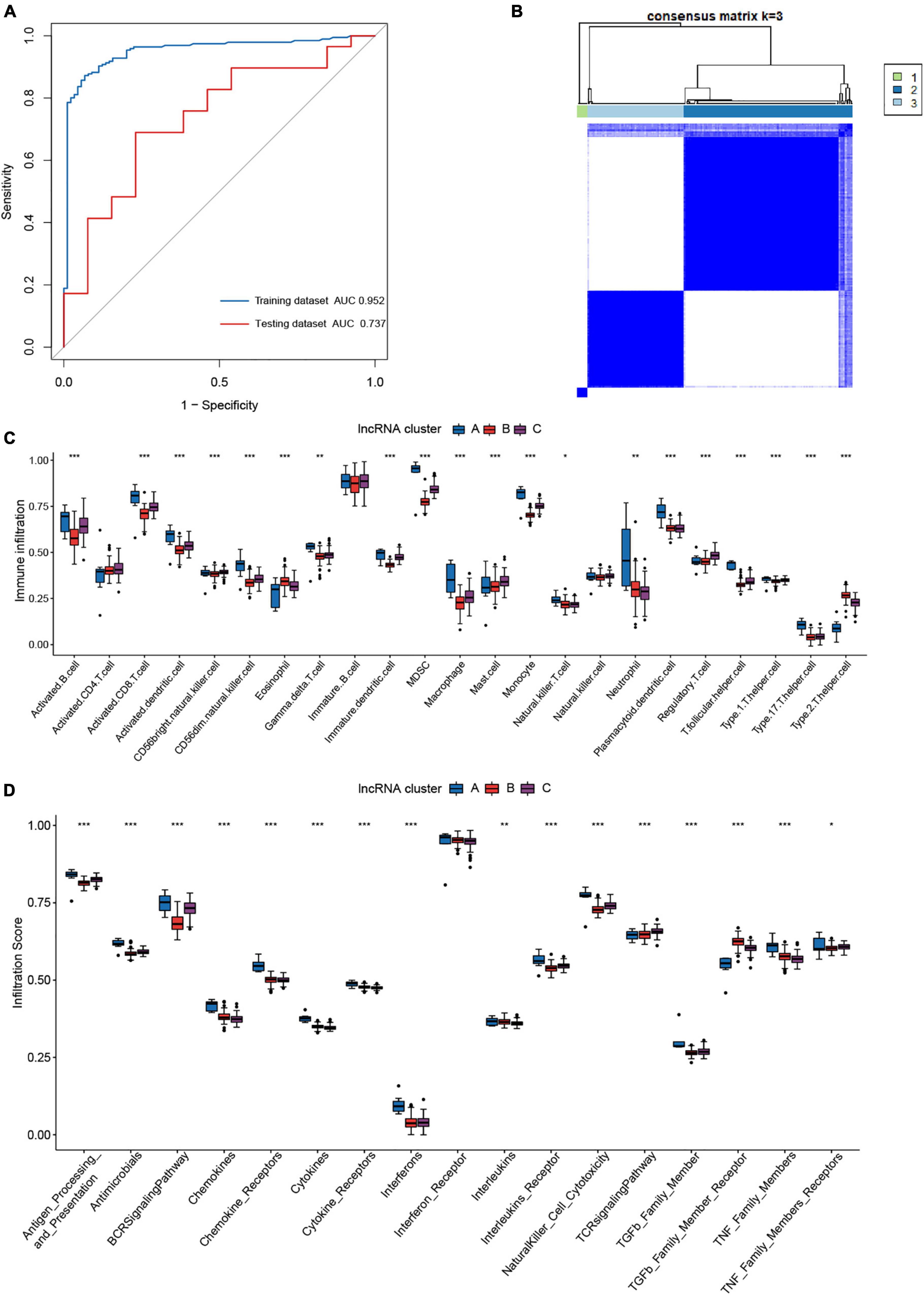
Figure 8. The role of m6A- and m5C-related long non-coding ribonucleic acids (lncRNAs) in juvenile idiopathic arthritis (JIA). (A) The ROC curve of the second diagnostic model constructed with lncRNAs for JIA. (B) Consensus clustering classified JIA into three lncRNA clusters. (C) The discrepancies in the abundance of each infiltrating immunocyte in the three lncRNA clusters. (D) The three clusters exhibit varying degrees of immunological response.
The ConsensusClusterPlus R package was used again to classify JIA patients into three unique molecular patterns based on the expression of m6A- and m5C-related lncRNAs, including 7 cases in pattern A, 120 cases in pattern B, and 69 cases in pattern C (Figure 8B). To differentiate them, we referred to these three patterns as lncRNA cluster A, lncRNA cluster B, and lncRNA cluster C. To our astonishment, samples classified as lncRNA cluster A were identical to those classified as pattern A for m6A typing. A synergistic relationship between m6A regulators and lncRNAs may be shown here. The same immune infiltration analysis was performed for the three lncRNA clusters, and the results revealed a more subtle regulatory network. The three lncRNA clusters demonstrated substantial differences in the activated dendritic cell, CD56 bright natural killer cell, mast cell, and Type 1 T helper cell that were not found in the m6A patterns (Figure 8C). Moreover, in other immune cell types, both the lncRNA clusters and m6A patterns produced almost identical outcomes. The distinctions in immune reactions between the three lncRNA clusters were subsequently investigated. As expected, the lncRNA clusters were likewise more distinct than the m6A patterns (Figure 8D). Not only were the differential immune responses between m6A patterns present in lncRNA clusters, but also there were apparent discrepancies of interleukins and TNF family members receptors between lncRNA clusters. The analysis of HLA gene expression in lncRNA clusters revealed similar results to m6A patterns (Figure 9A). The above results demonstrated the inextricable link between m6A-mediated methylation modification and lncRNAs, and the collaborative participation of m6A and lncRNAs in the epigenetic modification of JIA. Since we addressed pattern A in detail previously, here we concentrate on the variations of biological pathways between lncRNA cluster B and C. LncRNA cluster C demonstrated greater immunological activity than lncRNA cluster B (Figure 9B).
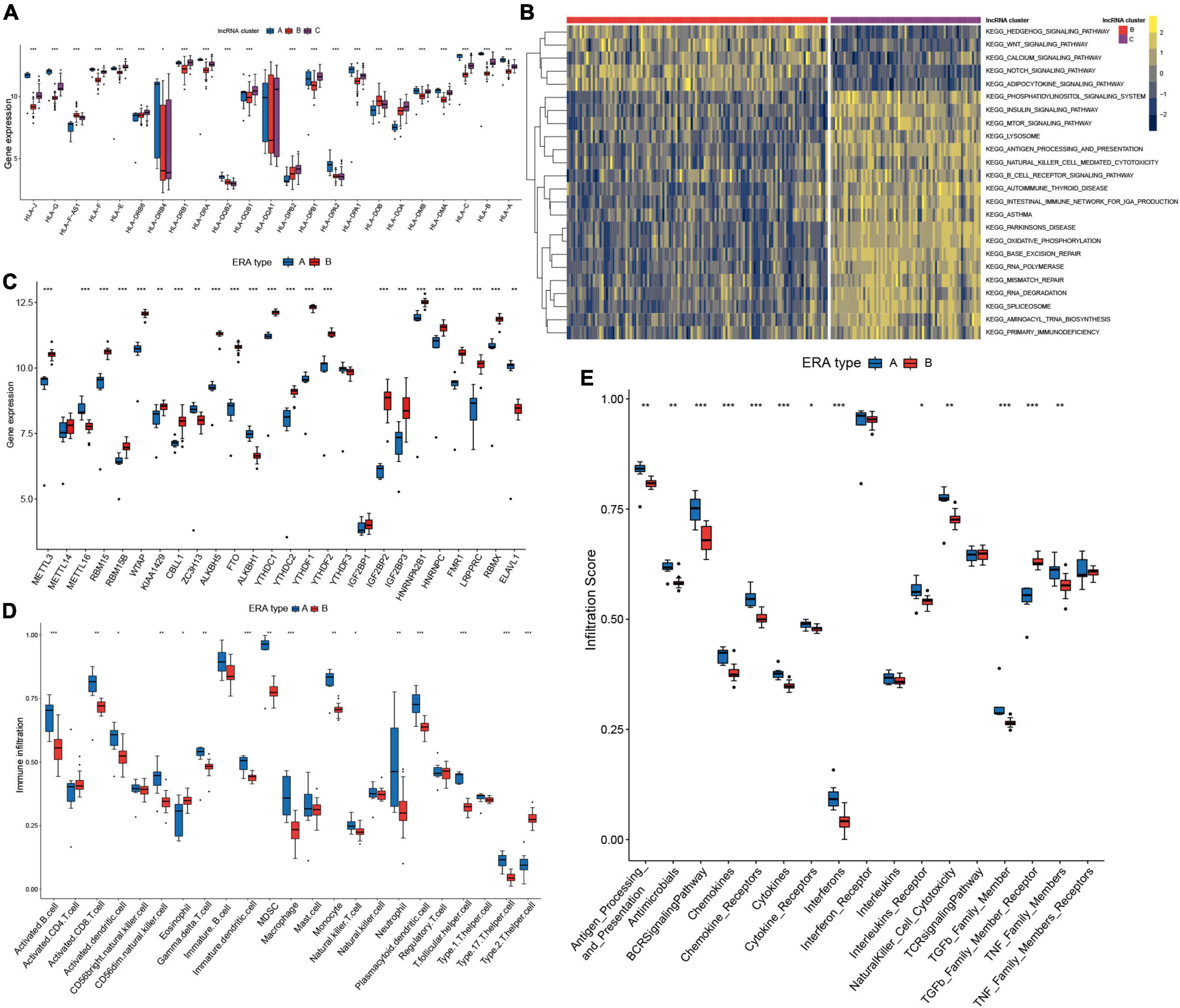
Figure 9. Two distinct subclusters of enthesitis-related arthritis (ERA) were identified. (A) Human leukocyte antigens (HLA) gene expression varied significantly across the three long non-coding ribonucleic acid (lncRNA) clusters. (B) GSVA enrichment analysis shows the differences in the underlying biological processes between the three lncRNA clusters. (C) Different expressions of the m6A regulators in the two subclusters of ERA. (D) The discrepancies in the abundance of each infiltrating immunocyte in the two subclusters of ERA. (E) The two subclusters of ERA exhibit varying degrees of immunological response.
Two distinct subclusters of enthesitis-related arthritis
Pattern A in m6A typing and lncRNA cluster A in lncRNA typing were determined to be the same and match the ERA subtype of JIA, whereas the remaining ERA samples were classified into pattern B. ERA piqued our interest because of the two unique types presented. We defined them as type A and type B. The expression of 26 m6A regulators in the two types was investigated and compared (Figure 9C). The majority of m6A regulators were discovered to express at substantially lower levels in type A than in type B, including the FTO previously addressed in detail. Immune cells infiltrated at much higher quantities in type A (Figure 9D). Both humoral and cellular immunity were greater in type A than in type B. Further immune response analyses revealed that type A elicited a more robust immune response (Figure 9E). The two distinct ERA types indicated that the existing classification method of JIA was unable to differentiate individuals with different molecular subtypes, suggesting that m6A typing may aid in the discovery of novel classification approaches for JIA.
Correlation between m6Ascore and immune microenvironment
The preceding research conducted on patient groups was insufficient to predict the m6A methylation modification of individual JIA patients correctly. A scoring system based on the m6A modification gene signatures was developed to quantify the m6A modification in individual JIA patients. The Sankey diagram illustrated the changes of the JIA subtypes, m6A patterns, lncRNA clusters, and m6Ascore (Figure 10A). Wilcox test revealed statistical differences in m6Ascore between three m6A patterns (Figure 10B). Pattern A had the lowest m6Ascore, which means that lower m6Ascore may be linked to better immune reactions. Significantly different m6Ascores were also observed in the three lncRNA clusters and two ERA types (Figures 10C,D). The analysis of the overall immune cell infiltration landscape revealed that the low m6Ascore group tended to have an upregulated immune infiltration level (Figure 10E). It was also discovered that patients with low m6Ascore had higher levels of immune response-related biological processes (Figure 10F). These results suggest that the m6Ascore may be utilized to evaluate the immunological state of JIA patients, thereby offering therapy recommendations.
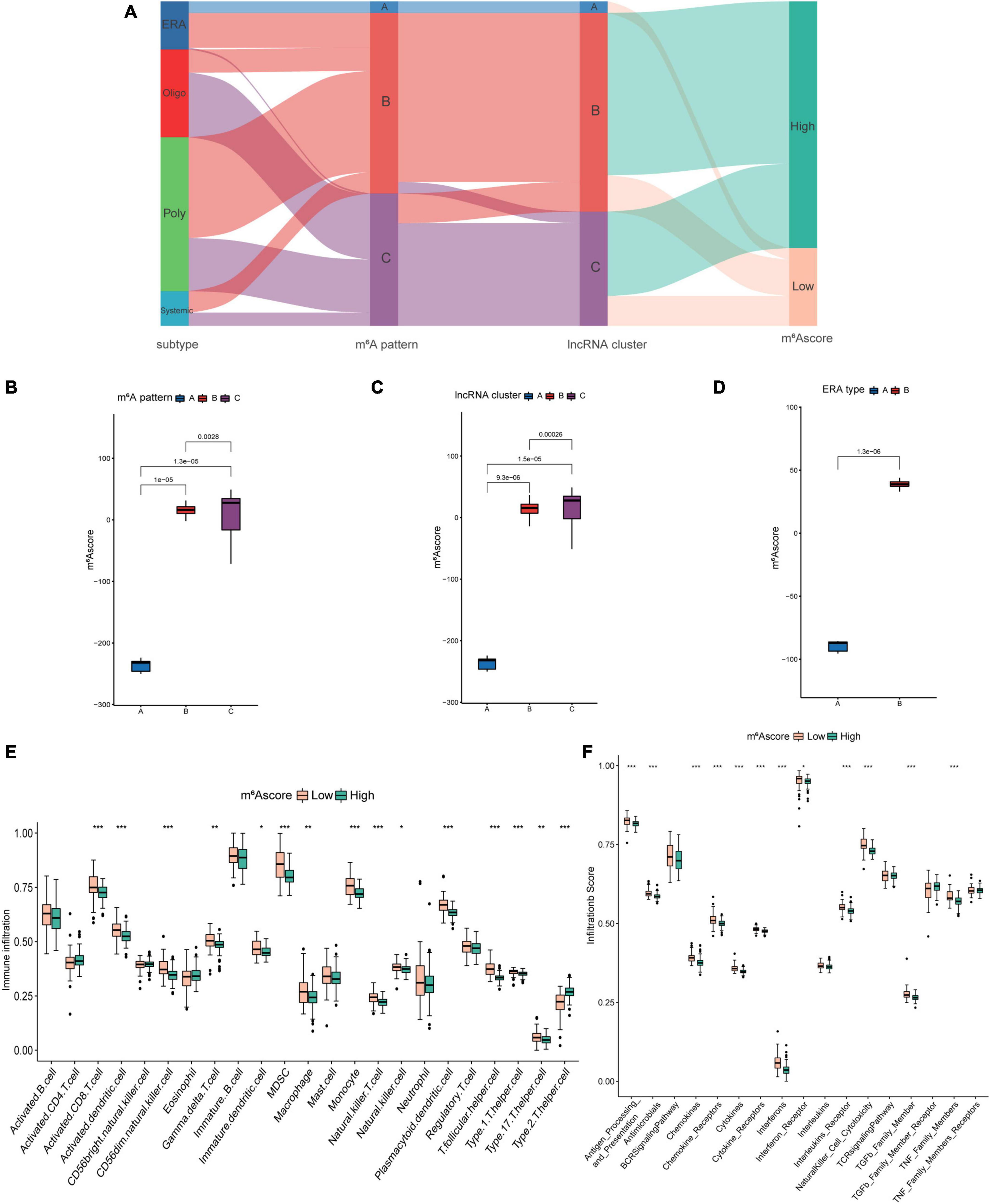
Figure 10. The construction and application of m6Ascore. (A) Alluvial diagram showing the changes of juvenile idiopathic arthritis (JIA) subtypes, m6A patterns, long non-coding ribonucleic acids (lncRNA) clusters, and m6Ascore. (B) Differences in m6Ascore between the three m6A patterns. (C) Differences in m6Ascore between the three lncRNA clusters. (D) Differences in m6Ascore between the two subclusters of enthesitis-related arthritis (ERA). (E) The discrepancies in the abundance of each infiltrating immunocyte between the high and low m6Ascore groups. (F) The high and low m6Ascore groups exhibit varying degrees of immunological response.
Discussion
The limitations of the present JIA categorization system are becoming more obvious, necessitating the development of a novel classification system for JIA (4). Regardless of the distinctions across JIA subtypes, their systematic study allows the identification of pathogenic pathways by focusing on the functional networks rather than individuals. JIA is a rheumatological chronic inflammatory disease, so the importance of immunity for JIA is hard to overstate. m6A modification and lncRNA have been proven to have critical regulatory roles in immunity (14, 42, 43). The investigation was carried out utilizing the gene expression profiles of PBMCs from 196 JIA and 89 healthy control samples. First, we compared the gene expression of m6A regulators in JIA with healthy control samples. Of the 26 m6A regulators investigated, 24 exhibited statistically significant changes. It suggested that the m6A modification may play a vital role in the pathogenesis of JIA. Most of the m6A regulators showed higher levels of expression among the JIA individuals. This phenomenon has also been observed in specific tumor types, including lung cancer, gastric cancer, and breast cancer (10). However, the precise molecular mechanisms of this regulation remain unknown. This also suggests the need for more precise and direct methods to assess the m6A modification landscape in JIA, thus revealing the complex crosstalk of m6A regulators. The LASSO regression analysis screened out 14 m6A regulators critical for JIA diagnosis, and they served as the foundation for the development of the first logistic diagnostic model. After 405 m6A- and m5C-related lncRNAs were obtained, the LASSO regression analysis algorithm was reapplied to determine eight diagnostically significant lncRNAs (ZNF582-AS1, ZNF503-AS2, UBL7-AS1, TTTY5, PRKAG2-AS1, LINC01007, LINC00424, and ADARB2-AS1). Of these eight lncRNAs, ZNF582-AS1 has been proved to regulate the m6A modification of MT-RNR1, hence facilitating the development and metastasis of renal clear cell carcinoma (44). One study discovered that UBL7-AS1 promotes the proliferation of glioblastoma cells (45). And PRKAG2-AS1 has been identified as a prognostic gene signature of esophageal squamous cell cancer (46). These eight lncRNAs may also play critical roles in JIA. The second diagnostic model for JIA was created in the same manner using these lncRNAs. ROC curves demonstrated that both models had a high diagnostic capacity. They were validated on an independent external dataset to compare their performance. It was discovered that the model developed by m6A regulators outperformed the other. These fourteen m6A regulators and eight lncRNAs may be potential biological diagnostic indicators or therapeutic targets of JIA. They may give guidance for future studies on JIA in general.
FTO and ALKBH5 were the most significantly co-expressed m6A regulators in all samples, and their expression levels were inversely linked with the quantity of immune infiltrating cells. The role of FTO in JIA was thoroughly investigated, and potential molecular mechanisms of FTO were explored. FTO is also known as the adiposity-related gene (47). FTO was found as the first m6A demethylase, demonstrating that m6A modification is dynamic and reversible (40). FTO has been reported to modulate targets genes expression by decreasing the level of m6A in transcripts, thereby promoting hematopoietic cell transformation and leukemogenesis (48). It also has been shown that over-expression of FTO promotes the development of esophageal cancer through modulating lncRNA (49). In this study, we found that the expression of co-stimulatory molecules, adhesion molecules, and MHC were enhanced across the board in patients with decreased FTO expression. Additionally, patients with low-expressed FTO also had increased infiltration of conventional DCs and a more robust immunological response. Therefore, we postulated that FTO-mediated m6A modification may increase MHC and co-stimulatory molecules production, thereby activating conventional dendritic cells and enhancing immunological response in JIA patients. However, this theory must be validated by further trials.
Unsupervised clustering of JIA samples revealed three distinct m6A patterns. Further analysis demonstrated that pattern A had a much higher level of immune cells infiltration and a more vigorous immunological response than the other two patterns. m6A modification-related genes were obtained, and their putative biological functions were explored. m6A modification-related regulatory mechanisms and activation of immunity were significantly over-represented among the genes. Thus, they helped us comprehend the pathophysiology of JIA from the viewpoint of m6A modification. WGCNA analysis revealed gene modules associated with diverse m6A patterns, assisting in the comprehension of the m6A regulator-mediated molecular functional network from a genetic perspective. Similarly, another unsupervised clustering analysis on JIA samples was performed based on 405 m6A- and m5C-related lncRNAs, and three distinct clusters were identified, with cluster A exhibiting the highest level of immunity. Additionally, we discovered that molecular subtypes mediated by lncRNAs were more sophisticated than those mediated by m6A regulators since there were more significant variations in immune cells infiltration, immunological response, and HLA genes expression amongst lncRNA clusters than m6A patterns. Our investigation of GSVA enrichment analysis among different modification patterns revealed a large number of diseases such as asthma, leukemia, Huntington’s disease, Alzheimer’s disease, Parkinson’s disease, autoimmune thyroid disease, and a variety of cancers. Research on the link between m6A modification and cancer has been exhaustive (10). Our findings may imply that m6A modification also plays a crucial role in these diseases, pointing the way forward for further research into these disorders. It has been established that m6A is associated with several disorders as mentioned above (38, 50). JIA has been shown to have substantial and well-documented relationships with HLA alleles (51). Significant changes in HLA genes expression across distinct m6A patterns were observed in this study, suggesting that HLA genes may contribute to JIA categorization and aid in establishing a novel JIA classification system. Additionally, the two unique patterns seen in ERA subtypes indicated that the existing JIA categorization scheme was insufficiently detailed. These findings may help us comprehend the varied immunological microenvironments in JIA, as well as the diverse molecular biological processes that may exist in JIA, and may lead to the development of more effective and tailored treatment options for JIA patients.
The discrepancies in the gene expression of diverse m6A patterns were evidenced to be strongly connected with m6A modification and immune-related molecular mechanisms in this research. A scoring system to assess the m6A modifications in individual JIA patients was developed due to the heterogeneity of JIA. Both m6A regulators typing and lncRNAs typing revealed considerable differences in m6Ascore among subtypes. And m6Ascore displayed a substantial and inverse association with the immunological response. The management of patients may be greatly aided by the identification of certain molecular subtype or immunological status. The establishment of m6Ascore enables the development of personalized treatment strategies for JIA patients.
Our study is the first to conduct a comprehensive analysis of the m6A regulators mediated molecular patterns in juvenile idiopathic arthritis. We have shed light on the potential molecular pathogenesis of JIA from an immunological perspective. We did not limit our investigation to m6A modification of mRNA, and we also explored the impact of m6A- and m5C-related lncRNAs in JIA. This study demonstrated that m6A modification is involved in the regulation of the immunological microenvironment of JIA and plays a crucial role in its progression. However, we must acknowledge that this research has certain flaws. Due to the absence of clinical data, the relationship between m6A patterns and clinical features was not extensively investigated in this research. Currently, clinical diagnosis of JIA for physicians primarily relies on differential diagnosis to rule out other similar disorders. But it is unknown if the models we developed can distinguish JIA from other similar diseases. Besides, this study relies on bioinformatics analysis. Although the conclusions are theoretically valid, they have not been empirically tested and must be confirmed by further research. Nevertheless, we hope this research could shed fresh light on establishing the new JIA classification criteria and the future direction of JIA research.
Conclusion
In conclusion, our study elucidates putative regulatory mechanisms behind the m6A modification in the immunological microenvironment of juvenile idiopathic arthritis. The analysis of m6A patterns and m6A- and m5C-related lncRNAs enables us to better understand the epigenetic landscape in JIA. The comprehensive assessment of m6A regulators in JIA will aid in the development of more effective and tailored immunotherapy for JIA patients.
Data availability statement
The datasets analyzed for this study can be found in the Gene Expression Omnibus (https://www.ncbi.nlm.nih.gov/geo/query/acc.cgi?acc=GSE11083/GSE13501/GSE20307/GSE21521/GSE67596/GSE15645).
Author contributions
ZT conceived and supervised the study. ZT and SZ designed the work. SZ, JQ, and YZ contributed to the data analysis. SZ, JQ, and JW wrote the manuscript. All authors contributed to the discussion and revision of the final manuscript.
Acknowledgments
We gratefully appreciate the GEO project and its contributors for the availability of the raw data. We also sincerely thank the R Core Team and all R package developers.
Conflict of interest
The authors declare that the research was conducted in the absence of any commercial or financial relationships that could be construed as a potential conflict of interest.
Publisher’s note
All claims expressed in this article are solely those of the authors and do not necessarily represent those of their affiliated organizations, or those of the publisher, the editors and the reviewers. Any product that may be evaluated in this article, or claim that may be made by its manufacturer, is not guaranteed or endorsed by the publisher.
Supplementary material
The Supplementary Material for this article can be found online at: https://www.frontiersin.org/articles/10.3389/fped.2022.930119/full#supplementary-material
Footnotes
References
1. Petty RE, Southwood TR, Manners P, Baum J, Glass DN, Goldenberg J, et al. International league of associations for rheumatology classification of juvenile idiopathic arthritis: second revision, Edmonton, 2001. J Rheumatol. (2004) 31:390–2.
2. Martini A, Lovell DJ, Albani S, Brunner HI, Hyrich KL, Thompson SD, et al. Juvenile idiopathic arthritis. Nat Rev Dis Primers. (2022) 8:5. doi: 10.1038/s41572-021-00332-8
3. Ravelli A, Martini A. Juvenile idiopathic arthritis. Lancet. (2007) 369:767–78. doi: 10.1016/S0140-6736(07)60363-8
4. Nigrovic PA, Colbert RA, Holers VM, Ozen S, Ruperto N, Thompson SD, et al. Biological classification of childhood arthritis: roadmap to a molecular nomenclature. Nat Rev Rheumatol. (2021) 17:257–69. doi: 10.1038/s41584-021-00590-6
5. Minoia F, Davì S, Horne A, Demirkaya E, Bovis F, Li C, et al. Clinical features, treatment, and outcome of macrophage activation syndrome complicating systemic juvenile idiopathic arthritis: a multinational, multicenter study of 362 patients. Arthritis Rheumatol. (2014) 66:3160–9. doi: 10.1002/art.38802
6. Marelli L, Romano M, Pontikaki I, Gattinara MV, Nucci P, Cimaz R, et al. Long term experience in patients with JIA-associated uveitis in a large referral center. Front Pediatr. (2021) 9:682327. doi: 10.3389/fped.2021.682327
7. Roundtree IA, Evans ME, Pan T, He C. Dynamic RNA modifications in gene expression regulation. Cell. (2017) 169:1187–200. doi: 10.1016/j.cell.2017.05.045
8. Barbieri I, Kouzarides T. Role of RNA modifications in cancer. Nat Rev Cancer. (2020) 20:303–22. doi: 10.1038/s41568-020-0253-2
9. Li S, Mason CE. The pivotal regulatory landscape of RNA modifications. Annu Rev Genomics Hum Genet. (2014) 15:127–50. doi: 10.1146/annurev-genom-090413-025405
10. Huang H, Weng H, Chen J. m6A modification in coding and non-coding RNAs: roles and therapeutic implications in cancer. Cancer Cell. (2020) 37:270–88. doi: 10.1016/j.ccell.2020.02.004
11. Su R, Dong L, Li Y, Gao M, He PC, Liu W, et al. METTL16 exerts an m6A-independent function to facilitate translation and tumorigenesis. Nat Cell Biol. (2022) 24:205–16. doi: 10.1038/s41556-021-00835-2
12. Zhou J, Zhang X, Hu J, Qu R, Yu Z, Xu H, et al. m6A demethylase ALKBH5 controls CD4+ T cell pathogenicity and promotes autoimmunity. Sci Adv. (2021) 7:eabg0470. doi: 10.1126/sciadv.abg0470
13. Livneh I, Moshitch-Moshkovitz S, Amariglio N, Rechavi G, Dominissini D. The m6A epitranscriptome: transcriptome plasticity in brain development and function. Nat Rev Neurosci. (2020) 21:36–51. doi: 10.1038/s41583-019-0244-z
14. Shulman Z, Stern-Ginossar N. The RNA modification N6-methyladenosine as a novel regulator of the immune system. Nat Immunol. (2020) 21:501–12. doi: 10.1038/s41590-020-0650-4
15. Wang L, Wen M, Cao X. Nuclear hnRNPA2B1 initiates and amplifies the innate immune response to DNA viruses. Science. (2019) 365:eaav0758. doi: 10.1126/science.aav0758
16. Li H-B, Tong J, Zhu S, Batista PJ, Duffy EE, Zhao J, et al. m6A mRNA methylation controls T cell homeostasis by targeting the IL-7/STAT5/SOCS pathways. Nature. (2017) 548:338–42. doi: 10.1038/nature23450
17. Tong J, Cao G, Zhang T, Sefik E, Amezcua Vesely MC, Broughton JP, et al. m6A mRNA methylation sustains Treg suppressive functions. Cell Res. (2018) 28:253–6. doi: 10.1038/cr.2018.7
18. Amaral PP, Dinger ME, Mercer TR, Mattick JS. The eukaryotic genome as an RNA machine. Science. (2008) 319:1787–9. doi: 10.1126/science.1155472
19. Kopp F, Mendell JT. Functional classification and experimental dissection of long noncoding RNAs. Cell. (2018) 172:393–407. doi: 10.1016/j.cell.2018.01.011
20. Geisler S, Coller J. RNA in unexpected places: long non-coding RNA functions in diverse cellular contexts. Nat Rev Mol Cell Biol. (2013) 14:699–712. doi: 10.1038/nrm3679
21. Li Y, Syed J, Sugiyama H. RNA-DNA triplex formation by long noncoding RNAs. Cell Chem Biol. (2016) 23:1325–33. doi: 10.1016/j.chembiol.2016.09.011
22. Engreitz JM, Ollikainen N, Guttman M. Long non-coding RNAs: spatial amplifiers that control nuclear structure and gene expression. Nat Rev Mol Cell Biol. (2016) 17:756–70. doi: 10.1038/nrm.2016.126
23. Patil DP, Chen C-K, Pickering BF, Chow A, Jackson C, Guttman M, et al. m(6)A RNA methylation promotes XIST-mediated transcriptional repression. Nature. (2016) 537:369–73. doi: 10.1038/nature19342
24. Zhao BS, Roundtree IA, He C. Post-transcriptional gene regulation by mRNA modifications. Nat Rev Mol Cell Biol. (2017) 18:31–42. doi: 10.1038/nrm.2016.132
25. Gautier L, Cope L, Bolstad BM, Irizarry RA. affy–analysis of Affymetrix GeneChip data at the probe level. Bioinformatics. (2004) 20:307–15. doi: 10.1093/bioinformatics/btg405
26. Leek JT, Johnson WE, Parker HS, Jaffe AE, Storey JD. The sva package for removing batch effects and other unwanted variation in high-throughput experiments. Bioinformatics. (2012) 28:882–3. doi: 10.1093/bioinformatics/bts034
27. Wilkerson MD, Hayes DN. ConsensusClusterPlus: a class discovery tool with confidence assessments and item tracking. Bioinformatics. (2010) 26:1572–3. doi: 10.1093/bioinformatics/btq170
28. Şenbabaoğlu Y, Michailidis G, Li JZ. Critical limitations of consensus clustering in class discovery. Sci Rep. (2014) 4:6207. doi: 10.1038/srep06207
29. Hänzelmann S, Castelo R, Guinney J. GSVA: gene set variation analysis for microarray and RNA-seq data. BMC Bioinformatics. (2013) 14:7. doi: 10.1186/1471-2105-14-7
30. Charoentong P, Finotello F, Angelova M, Mayer C, Efremova M, Rieder D, et al. Pan-cancer immunogenomic analyses reveal genotype-immunophenotype relationships and predictors of response to checkpoint blockade. Cell Rep. (2017) 18:248–62. doi: 10.1016/j.celrep.2016.12.019
31. Bhattacharya S, Dunn P, Thomas CG, Smith B, Schaefer H, Chen J, et al. ImmPort, toward repurposing of open access immunological assay data for translational and clinical research. Sci Data. (2018) 5:180015. doi: 10.1038/sdata.2018.15
32. Ritchie ME, Phipson B, Wu D, Hu Y, Law CW, Shi W, et al. limma powers differential expression analyses for RNA-sequencing and microarray studies. Nucleic Acids Res. (2015) 43:e47. doi: 10.1093/nar/gkv007
33. Yu G, Wang L-G, Han Y, He Q-Y. clusterProfiler: an R package for comparing biological themes among gene clusters. OMICS. (2012) 16:284–7. doi: 10.1089/omi.2011.0118
34. Langfelder P, Horvath S. WGCNA: an R package for weighted correlation network analysis. BMC Bioinformatics. (2008) 9:559. doi: 10.1186/1471-2105-9-559
35. Petrelli A, Mijnheer G, Hoytema van Konijnenburg DP, van der Wal MM, Giovannone B, Mocholi E, et al. PD-1+CD8+ T cells are clonally expanding effectors in human chronic inflammation. J Clin Invest. (2018) 128:4669–81. doi: 10.1172/JCI96107
36. Paramasivam A, Priyadharsini JV, Raghunandhakumar S. Implications of m6A modification in autoimmune disorders. Cell Mol Immunol. (2020) 17:550–1. doi: 10.1038/s41423-019-0307-0
37. Ma S, Chen C, Ji X, Liu J, Zhou Q, Wang G, et al. The interplay between m6A RNA methylation and noncoding RNA in cancer. J Hematol Oncol. (2019) 12:121. doi: 10.1186/s13045-019-0805-7
38. Shafik AM, Zhang F, Guo Z, Dai Q, Pajdzik K, Li Y, et al. N6-methyladenosine dynamics in neurodevelopment and aging, and its potential role in Alzheimer’s disease. Genome Biol. (2021) 22:17. doi: 10.1186/s13059-020-02249-z
39. Ringold S, Weiss PF, Colbert RA, DeWitt EM, Lee T, Onel K, et al. Childhood arthritis and rheumatology research alliance consensus treatment plans for new-onset polyarticular juvenile idiopathic arthritis. Arthritis Care Res (Hoboken). (2014) 66:1063–72. doi: 10.1002/acr.22259
40. Jia G, Fu Y, Zhao X, Dai Q, Zheng G, Yang Y, et al. N6-methyladenosine in nuclear RNA is a major substrate of the obesity-associated FTO. Nat Chem Biol. (2011) 7:885–7. doi: 10.1038/nchembio.687
41. Morante-Palacios O, Fondelli F, Ballestar E, Martínez-Cáceres EM. Tolerogenic dendritic cells in autoimmunity and inflammatory diseases. Trends Immunol. (2021) 42:59–75. doi: 10.1016/j.it.2020.11.001
42. Chen YG, Satpathy AT, Chang HY. Gene regulation in the immune system by long noncoding RNAs. Nat Immunol. (2017) 18:962–72. doi: 10.1038/ni.3771
43. Tang Y, Zhou T, Yu X, Xue Z, Shen N. The role of long non-coding RNAs in rheumatic diseases. Nat Rev Rheumatol. (2017) 13:657–69. doi: 10.1038/nrrheum.2017.162
44. Yang W, Zhang K, Li L, Xu Y, Ma K, Xie H, et al. Downregulation of lncRNA ZNF582-AS1 due to DNA hypermethylation promotes clear cell renal cell carcinoma growth and metastasis by regulating the N(6)-methyladenosine modification of MT-RNR1. J Exp Clin Cancer Res. (2021) 40:92. doi: 10.1186/s13046-021-01889-8
45. Cao M, Ma R, Li H, Cui J, Zhang C, Zhao J. Therapy-resistant and -sensitive lncRNAs, SNHG1 and UBL7-AS1 promote glioblastoma cell proliferation. Oxid Med Cell Longev. (2022) 2022:2623599. doi: 10.1155/2022/2623599
46. Zhang C, Zhang Z, Zhang G, Xue L, Yang H, Luo Y, et al. A three-lncRNA signature of pretreatment biopsies predicts pathological response and outcome in esophageal squamous cell carcinoma with neoadjuvant chemoradiotherapy. Clin Transl Med. (2020) 10:e156. doi: 10.1002/ctm2.156
47. Frayling TM, Timpson NJ, Weedon MN, Zeggini E, Freathy RM, Lindgren CM, et al. A common variant in the FTO gene is associated with body mass index and predisposes to childhood and adult obesity. Science. (2007) 316:889–94. doi: 10.1126/science.1141634
48. Li Z, Weng H, Su R, Weng X, Zuo Z, Li C, et al. FTO plays an oncogenic role in acute myeloid leukemia as a N6-methyladenosine RNA demethylase. Cancer Cell. (2017) 31:127–41. doi: 10.1016/j.ccell.2016.11.017
49. Cui Y, Zhang C, Ma S, Li Z, Wang W, Li Y, et al. RNA m6A demethylase FTO-mediated epigenetic up-regulation of LINC00022 promotes tumorigenesis in esophageal squamous cell carcinoma. J Exp Clin Cancer Res. (2021) 40:294. doi: 10.1186/s13046-021-02096-1
50. Vu LP, Pickering BF, Cheng Y, Zaccara S, Nguyen D, Minuesa G, et al. The N6-methyladenosine (m6A)-forming enzyme METTL3 controls myeloid differentiation of normal hematopoietic and leukemia cells. Nat Med. (2017) 23:1369–76. doi: 10.1038/nm.4416
Keywords: m6A, juvenile idiopathic arthritis, immune microenvironment, long non-coding RNA, m5C, diagnostic model
Citation: Zhang S, Qin J, Zhao Y, Wang J and Tian Z (2022) Identification of molecular patterns and diagnostic biomarkers in juvenile idiopathic arthritis based on the gene expression of m6A regulators. Front. Pediatr. 10:930119. doi: 10.3389/fped.2022.930119
Received: 27 April 2022; Accepted: 04 August 2022;
Published: 08 September 2022.
Edited by:
Klaus Tenbrock, RWTH Aachen University, GermanyReviewed by:
Jorg Van Loosdregt, University Medical Center Utrecht, NetherlandsBor-Luen Chiang, National Taiwan University, Taiwan
Copyright © 2022 Zhang, Qin, Zhao, Wang and Tian. This is an open-access article distributed under the terms of the Creative Commons Attribution License (CC BY). The use, distribution or reproduction in other forums is permitted, provided the original author(s) and the copyright owner(s) are credited and that the original publication in this journal is cited, in accordance with accepted academic practice. No use, distribution or reproduction is permitted which does not comply with these terms.
*Correspondence: Zhiliang Tian, dGlhbnpoaWxpYW5nOTk5QDE2My5jb20=