- 1Department of Epidemiology, Capital Institute of Pediatrics, Beijing, China
- 2Child Health Big Data Research Center, Capital Institute of Pediatrics, Beijing, China
- 3Department of Integrated Early Childhood Development, Capital Institute of Pediatrics, Beijing, China
Purpose: To assess the relationship between fat-free mass (FFM) and glucose metabolism in children 0–18 years of age.
Methods: We performed a systematic review of the literature on Medline/PubMed, SinoMed, Embase, and the Cochrane Library using the PRISMA 2020 guidelines to 12 October 2021; this encompassed observational studies in which the relationship between FFM and glucose metabolism was assessed. Correlation coefficient (r), regression coefficient (β), and odds ratio (OR) values in the studies were extracted and recorded as the primary data. “Agency for Healthcare Research and Quality” quality-assessment forms recommended for cross-sectional/prevalence studies were applied to evaluate the quality of the selected studies, and we executed R software to combine the pooled data.
Results: We included eight studies comprising 13,282 individuals, five of which involved the assessment of the relationship between FFM and blood glucose, and four on the relationship between FFM and insulin resistance (IR). Our results showed that FFM was significantly associated with fasting plasma insulin levels (r = 0.34, 95% CI: 0.30–0.39, P < 0.001). Due to high heterogeneity or insufficient quantity of data, the studies of the relationship between FFM and fasting plasma glucose, HOMA-IR, or HbA1c were not congruent, and were therefore not suitable for meta-analysis.
Conclusion: Our results indicated that FFM was significantly associated with fasting plasma insulin levels. As far as we have determined, this is the first-ever systematic review and meta-analysis of the associations between FFM and glucose metabolism in children and adolescents; and our results thus provide novel information to fill a gap in the literature in this area.
Systematic Review Registration: https://www.crd.york.ac.uk/prospero/display_record.php?ID=CRD42020150320, PROSPERO CRD42020150320.
Introduction
Type 2 diabetes (T2D) is one of the most common metabolic disorders. In 2014, 9% of adults exhibited the disease worldwide (1), and its prevalence continues to climb (2). Childhood obesity increases the risk of developing T2D (3), and the early prevention of childhood obesity may therefore be critical to T2D prevention (4).
It is well-recognized that skeletal muscle is an important component of body composition and a metabolic sink for glucose disposal (5). Skeletal muscle also plays an important role in the regulation of blood glucose levels and affects the development of insulin resistance (IR) and T2D (6). A previous study reported that decreased skeletal muscle mass is associated with deterioration of insulin sensitivity (7). A longitudinal study showed a positive association between abdominal muscle quantity and T2D (8), and a cross-sectional study revealed that trunk muscle quality was related to glucose tolerance (9). Recently, some studies have found similar results in the pediatric population (10, 11). Thus, targeting the mechanism(s) underlying skeletal muscle activity in glucose metabolism may prevent or delay IR and T2D.
There are several potential pathways that subserve the effects of skeletal muscle on glucose metabolism. (a) Skeletal muscle stores glucose as glycogen through the translocation of glucose transporter type-4 via insulin signals (12). (b) Testosterone may be critical to skeletal muscle glucose metabolism, as it has been reported that elevated muscular 5α-dihydrotestosterone may contribute to the improvement of hyperglycemia in T2D rats (13), and higher testosterone concentrations and muscle mass were associated with improved glucose metabolism in children (11). (c) Forkhead box class O transcription factors regulate glucose metabolism and markers of inflammation in skeletal muscle (14). Although skeletal muscle mass is negatively correlated with IR and T2D, a precise role for skeletal muscle mass in glucose metabolism has not yet been fully elucidated.
Because it is difficult to directly quantify the amount of muscle mass, body composition is usually divided into fat mass and fat-free mass (FFM) for evaluation. To the best of our knowledge, there is no extant meta-analysis on the relationship between FFM and glucose metabolism (blood glucose and IR) in children aged 0–18 years. Our study will therefore assist in clarifying the association between muscle mass and glucose metabolism in children and adolescents.
Methods
The protocol we used for the present systematic review was based on PRISMA 2020 (Preferred Reporting Items for Systematic reviews and Meta-Analyses) (15) and was registered in PROSPERO (the International Prospective Register of Systematic Reviews; http://www.crd.york.ac.uk/prospero) under the registration number CRD42020150320.
We conducted our literature search using MEDLINE/PubMed, EMBASE, SinoMed, and the Cochrane Library; and restricted the search to (a) studies published in English or Chinese, and (b) studies on humans, with only samples from children and adolescents under 18 years of age.
Observational studies (of a cross-sectional or longitudinal design) that involved the relation between muscle mass/FFM and glucose metabolism in children and adolescents were then selected. To be included, studies were required to meet the following criteria: (a) muscle mass/FFM measured by a direct method, bioelectrical impedance analysis (BIA)/dual energy X-ray absorptiometry (DXA)/magnetic resonance imaging (MRI)/air-replaced method, or other method; and (b) assessment of at least one glucose metabolism indicator (fasting glucose, IR, homeostasis model assessment [HOMA-IR], or glycosylated hemoglobin A1c [HbA1c]).
Studies were not included if: (a) the study outcomes only focused on surrogate indicators of body composition or physical fitness rather than directly measured indicators; (b) they assessed the relationship in adults or non-human species; (c) they involved children with severe physical limitations; (d) they referred to children with disorders that could limit generalizability (e.g., those manifesting mitochondrial dysfunction; Prader-Willi syndrome; non-alcoholic fatty-liver disease; polycystic ovarian syndrome; or mental disorders that included attention-deficit/hyperactivity disorder [ADHD]; conduct or neuropsychiatric disorders that included schizophrenia; or any detected delay in communication, adaptive cognition, or socio-emotional domains); and (e) if they referred to a particular population group such as an aboriginal group, immigrant group; or to a specific economic status or to an unhealthy child.
Data Extraction (Selection and Coding)
Risk of Bias (Quality) Assessment
We used “Agency for Healthcare Research and Quality (AHRQ, USA)” quality-assessment forms containing 11-item checklists that are recommended for cross-sectional/prevalence studies to assess the methodological quality of the selected studies, and used two independent researchers (Fangfang Chen and Junting Liu). Any disagreements over the assessment of bias risk were discussed, and a consensus was reached, with a third researcher (Dongqing Hou) consulted if consensus could not be attained.
An item was scored “0” if it was answered “NO” or “UNCLEAR,” and “1” if it was answered “YES.” Article quality was assessed as follows: low quality, 0–3; moderate quality, 4–7; and high quality, 8–11.
Strategy for Data Synthesis
The amount of observed variance that reflected actual differences in effect size across the included trials was graded using the I2 statistic, with values representing mild, moderate, and severe heterogeneity (<25, 25–75, and > 75%, respectively) (16). We clustered subgroup analysis by sex and processed these data according to the results of the heterogeneity test and study quality. The correlation coefficients (r) and regression coefficients (β) for FFM and glucose metabolism (fasting plasma glucose [FPG], fasting plasma insulin [INS] and HOMA-IR [= FPG*FPI/22.5]) were then synthesized, and Tables were created to summarize the characteristics of the selected studies. The feasibility of conducting a meta-analysis was thus determined after the data were extracted.
R software was used to combine the pooled data. A fixed-effect model was exploited when there was no evidence of heterogeneity; otherwise, a random-effect model was applied.
Analysis of Subgroups or Subsets
Subgroup and meta-regression analyses were carried out on the main factors causing heterogeneity, including the sex of the study participants. Furthermore, the design and methodological quality of the studies were considered for additional subgroup analyses.
Results
Identification and Selection of Studies
The original search identified 3,450 studies, and after removal of duplicates and the elimination of papers based upon eligibility criteria, eight studies remained (Figure 1). Of the eight studies, the relationship between FFM and FPG was assessed in five, four involved an analysis between FFM and INS, four studies focused on the relationship between FFM and HOMA-IR, and in one study a linear analysis (β) was performed between FFM and HbA1c.
Study Characteristics
The characteristics of all eight studies are shown in Table 1. Three papers based on two studies (baseline and follow-up data were used separately) were conducted in the UK (study ID: 3, 4, 6), two in the U.S. (study ID: 2, 7), two in China (study ID: 1, 5), and one study in Brazil (study ID: 8). Table 2 depicts the quality evaluation of the methodology according to AHRQ quality-assessment forms (US 2004). In the case of AHRQ scoring, there were 2 studies with 8 points, 1 study with 7 points, 2 studies with 6 points, and 3 studies with 5 points. There were 2 high quality trials with 8 points and 6 moderate quality trials with an average score of 5.7 points.
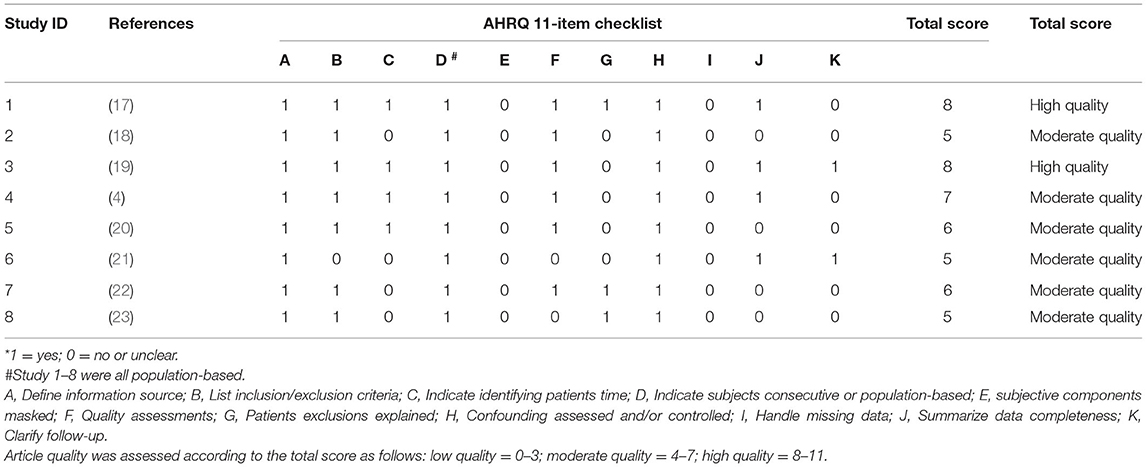
Table 2. The quality evaluation of the methodology according to Agency for Healthcare Research and Quality (AHRQ, US 2004) assessment forms*.
Relationship Between FFM and FPG
Regarding the relationship between FFM and FPG, three studies reported a correlation coefficient (r) (study ID: 1, 2, 3) (Figure 2): there was no statistically significant association found between FFM and FPG in two of these studies (study ID: 2, 3), and Chen et al. (17) (study ID: 1) reported inconsistent results. Three studies also furnished a regression coefficient (β) through linear analysis (study ID: 1, 4, 5) (Figure 3). While Lin et al. (20) (study ID: 5) reported that FFM was negatively associated with FPG, studies by Chen et al. (17) (study ID: 1) and Nightingale et al. (4) (study ID: 4) revealed that FFM was positively associated with FPG. Because of high heterogeneity (I2 = 71 and 96.1%, respectively), we deemed these studies as not suitable for meta-analysis.
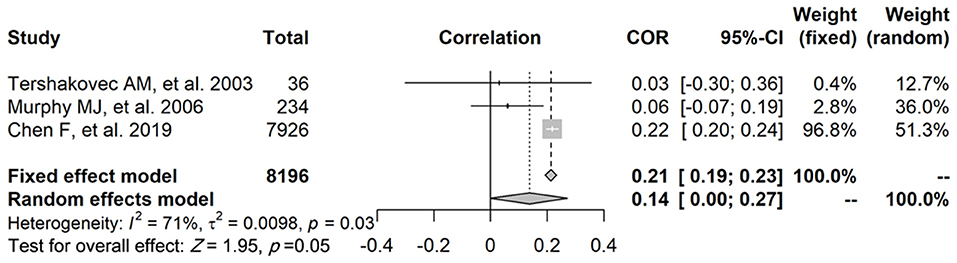
Figure 2. The relationship between FFM and FPG, and three studies provided the correlation coefficient (r).
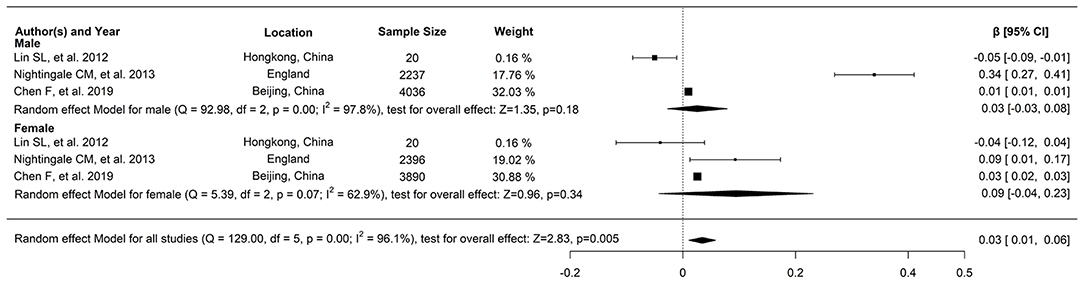
Figure 3. The relationship between FFM and FPG, and three studies provided the regression coefficient (β) through linear analysis.
Relationship Between FFM and INS
The data of three studies (n = 1,453) were pooled, and the results showed that, after data pooling using a fixed-effect model, FFM was significantly associated with fasting plasma insulin levels (Figure 4) (r = 0.34, 95% confidence interval [CI]: 0.30–0.39, P < 0.001).
In addition, Tershakovec et al. (18) (study ID: 2) reported that FFM was significantly associated with HOMA-IR (r = 0.32, P = 0.08), while, in contrast, Murphy et al. (19) (study ID: 3) reported the negative results (r = −0.15, P > 0.05, in males; r = 0.02, P > 0.05, in females). Santos et al. (23) (study ID: 8) showed that the final linear model regression coefficients for FFM were associated with IR among post-pubertal obese adolescents (β = 37.01, P = 0.007), and Nightingale et al. (4) (study ID: 4) showed the associations between FFM and HOMA-IR (β = 35.6, P < 0.001, in males; β = 28.6, P < 0.001, in females), and the associations between FFM and HbA1c (β = 3.68, P < 0.0001, in males; β = −0.51, P < 0.0001, in females). Because of insufficient quantity, these studies are not suitable for ourmeta-analysis.
Discussion
Considering that skeletal muscle plays a major role in glucose metabolism, most previous research concerns the negative relationship of muscle mass with T2D (24–26). However, a recent study has shown that sarcopenia is a new complication of T2D (27), and there is a reverse causality between muscle mass and T2D in middle-aged and elderly individuals. In order to exclude the influence of confounding factors such as age and degenerative disease, the study of the pediatric population could be better to clarify the relationship between muscle mass and glucose metabolism. Our research is the first meta-analysis of the relationship between muscle mass and glucose metabolism in children and adolescents. The results indicated that FFM was positively associated with fasting plasma insulin levels in children and adolescents.
According to reference 17 (study ID: 1) and after stratifying for overweight and obesity, we evaluated the relationship between FFM and INS and found that FFM exerted a protective effect on INS in children with normal weight, but that among children who are overweight or obese, body composition was related to elevated blood pressure and dyslipidemia but not to INS. This suggests that the effect of FFM on INS is inconsistent among children of different weight statuses, and it is necessary to further verify and explore the mechanisms.
Childhood obesity increases the risk of abnormal glucose metabolism, and FFM plays an important role in glucose metabolism. Anthropometric measures such as body mass index (BMI) are widely used to evaluate adiposity owing to their feasibility and low cost, but its use is limited because it does not discriminate for FFM and fat mass (28). A higher BMI may arise not only from greater body fat, but also from higher lean mass and/or bone mass, making it an imperfect measure of adiposity (29, 30). Therefore, it is crucial to study the relationships between body composition and glucose metabolism in children.
Recently, a systematic review and meta-analysis on the association of FFM with IR or metabolic syndrome in children has been conducted (31). The study identified lower values of FFM/lean body mass (%) in children and adolescents with IR/glucose tolerance/metabolic syndrome and higher values of FFM/lean body mass when these are expressed in kg. Another recent study on the relationship between pediatric obesity and its cardiometabolic consequences has been explored (32). The study showed that the rates of impaired glucose tolerance and low insulin sensitivity increased progressively across quintiles of uric acid in youth with overweight/obesity, and impaired fasting glucose and IR were associated only with the highest quintile of uric acid. Takase et al. reported that FFM was related to HbA1c levels when the study excluded participants who had been identified as having have diabetes (33). Ghachem et al. showed that independently of age, FFM was an independent predictor of IR (34). However, more research on the association of FFM with glucose metabolism in children is still needed.
Several limitations need to be addressed. First, due to limited conditions, we only searched MEDLINE/PubMed, EMBASE, SinoMed, and the Cochrane Library, and only those studies published in English or Chinese were involved. There was a paucity of studies on FFM and glucose metabolism in children and adolescents, and there might have therefore been some omissions. Second, heterogeneity among studies was significant, which may be partially explained by the variety in assays used for the measurement of FFM in the studies. Third, there was a possibility of publication bias among the included trials. Fourth, because of the relatively small number of studies, we could not execute a stratified analysis of the relationship between FFM and glucose metabolism in children and adolescents. We noted a transient elevation in plasma glucose in adolescence, and studies have revealed that in normal healthy children FFM increased with age in boys, particularly during puberty. Adolescence—particularly in the early and middle periods—was also reported to be associated with insulin resistance directed toward carbohydrate metabolism, and with higher insulin levels than those found in younger children. This may be due to higher levels of growth hormone or other hormones that include pubertal hormones, or it may relate to an aspect of body mass. Unfortunately, puberty was not a weighted factor in the present analysis because of our limited access to raw data. Fifth, because FFM with age differs between men and women, it is necessary to consider the relationship between FFM and glucose metabolism in different age groups. Sixth, although BIA possesses several limitations, we included BIA-based studies in our meta-analysis because of the limiting conditions. This is a potential limitation of the study.
In the future, prospective cohorts with standardized assay measurements are needed to ascertain the causality between FFM and glucose metabolism in children and adolescents.
Conclusion
From this systematic review and meta-analysis, we found that there is a positive relationship between FFM and fasting plasma insulin levels. This is the first known systematic review and meta-analysis to determine the associations of FFM with glucose metabolism in children and adolescents. Our results provided novel information with which to fill a gap in the literature in this area. Further examination of these relationships requires the execution of additional cohort studies.
Data Availability Statement
The original contributions presented in the study are included in the article/supplementary material, further inquiries can be directed to the corresponding author/s.
Author Contributions
FC conceived and designed the study. FC, JL, and DH conducted the systematic search, screened articles, and selected eligible articles. LW and FC wrote the initial draft of the manuscript, with revisions by all authors. FC, TL, YC, and ZL reviewed the manuscript and performed the analyses. All authors contributed to the article and approved the submitted version.
Funding
This work was supported by the National Key Research and Development Program of China (2016YFC1300100), Public service development and reform pilot project of Beijing Medical Research Institute (BMR2019-11), and Beijing Municipal Administration of Hospitals Incubating Program (Px2022052).
Conflict of Interest
The authors declare that the research was conducted in the absence of any commercial or financial relationships that could be construed as a potential conflict of interest.
Publisher's Note
All claims expressed in this article are solely those of the authors and do not necessarily represent those of their affiliated organizations, or those of the publisher, the editors and the reviewers. Any product that may be evaluated in this article, or claim that may be made by its manufacturer, is not guaranteed or endorsed by the publisher.
References
1. World Health Organization. Global Status Report on Noncommunicable Diseases 2014. World Health Organization (2014). Available online at: https://www.who.int/publications/i/item/9789241564854 (accessed October 26, 2014)
2. Guariguata L, Whiting DR, Hambleton I, Beagley J, Linnen¬kamp U, Shaw JE. Global estimates of diabetes prevalence for 2013 and projections for 2035. Diabetes Res Clin Pract. (2014) 103:137–49. doi: 10.1016/j.diabres.2013.11.002
3. Deckelbaum RJ, Williams CL. Childhood obesity: the health issue. Obes Res. (2009) Suppl 4:239S−243S. doi: 10.1038/oby.2001.125
4. Nightingale CM, Rudnicka AR, Owen CG, Wells JCK, Sattar N, Cook DG, et al. Influence of adiposity on insulin resistance and glycemia markers among U.K. Children of South Asian, black African-Caribbean, and white European origin: child heart and health study in England. Diabetes Care. (2013) 36:1712–9. doi: 10.2337/dc12-1726
5. Smith AG, Muscat GE. Skeletal muscle and nuclear hormone receptors: implications for cardiovascular and metabolic disease. Int J Biochem Cell Biol. (2005) 37:2047–63. doi: 10.1016/j.biocel.2005.03.002
6. DeFronzo RA, Tripathy D. Skeletal muscle insulin resistance is the primary defect in type 2 diabetes. Diabetes Care. (2009) 32 (Suppl 2):S157–63. doi: 10.2337/dc09-S302
7. Srikanthan P, Karlamangla AS. Relative muscle mass is inversely associated with insulin resistance and prediabetes. findings from the third national health and nutrition examination survey. J Clin Endocrinol Metab. (2011) 96:2898–903. doi: 10.1210/jc.2011-0435
8. Granados A, Gebremariam A, Gidding SS, Terry JG, Carr JJ, Steffen LM, et al. Association of abdominal muscle composition with prediabetes and diabetes: the CARDIA study. Diabetes Obes Metab. (2019) 21:267–75. doi: 10.1111/dom.13513
9. Maltais A, Alméras N, Lemieux I, Tremblay A, Bergeron J, Poirier P, et al. Trunk muscle quality assessed by computed tomography: association with adiposity indices and glucose tolerance in men. Metabolism. (2018) 85:205–12. doi: 10.1016/j.metabol.2018.04.003
10. Weber DR, Leonard MB, Shults J, Zemel BS. A comparison of fat and lean body mass index to BMI for the identification of metabolic syndrome in children and adolescents. J Clin Endocrinol Metab. (2014) 99:3208–16. doi: 10.1210/jc.2014-1684
11. Hou WW, Tse MA, Lam TH, Leung GM, Schooling CM. Adolescent testosterone, muscle mass and glucose metabolism: evidence from the 'Children of 1997' birth cohort in Hong Kong. Diabet Med. (2015) 32:505–12. doi: 10.1111/dme.12602
12. Huang S, Czech MP. The GLUT4 glucose transporter. Cell Metab. (2007) 5:237–52. doi: 10.1016/j.cmet.2007.03.006
13. Horii N, Sato K, Mesaki N, Iemitsu M. Increased muscular 5α-dihydrotestosterone in response to resistance training relates to skeletal muscle mass and glucose metabolism in type 2 diabetic Rats. PLoS One. (2016) 11:e0165689. doi: 10.1371/journal.pone.0165689
14. Lundell LS, Massart J, Altintaş A, Krook A, Zierath JR. Regulation of glucose uptake and inflammation markers by FOXO1 and FOXO3 in skeletal muscle. Mol Metab. (2019) 20:79–88. doi: 10.1016/j.molmet.2018.09.011
15. Page MJ, McKenzie JE, Bossuyt PM, Boutron I, Hoffmann TC, Mulrow CD, et al. The PRISMA 2020 statement: an updated guideline for reporting systematic reviews. J Clin Epidemiol. (2021) 134:178–89. doi: 10.1016/j.jclinepi.2021.02.003
16. Higgins JP, Thompson SG, Deeks JJ, Altman DG. Measuring inconsistency in meta-analyses. BMJ. (2003) 327:557–60. doi: 10.1136/bmj.327.7414.557
17. Chen F Liu J Yan Y Mi J on on behalf of the China Child and Adolescent Cardiovascular Health (CCACH) Study Group. Abnormal metabolic phenotypes among urban Chinese children: epidemiology and the impact of DXA-measured body composition. Obesity. (2019) 27:837–44. doi: 10.1002/oby.22426
18. Tershakovec AM, Kuppler KM, Zemel BS, Katz L, Weinzimer S, Harty MP, et al. Body composition and metabolic factors in obese children and adolescents. Int J Obes Relat Metab Disord. (2003) 27:19–24. doi: 10.1038/sj.ijo.0802185
19. Murphy MJ, Metcalf BS, Jeffery AN, Voss LD, Wilkin TJ. Does lean rather than fat mass provide the link between birth weight, BMI, and metabolic risk? EarlyBird 23. Pediatr Diabetes. (2006) 7:211–4. doi: 10.1111/j.1399-5448.2006.00180.x
20. Lin SL, Lee SL, Hui LL, Yeung SLA, Tse MA, Leung GM, et al. Pubertal muscle mass and diabetes markers in Chinese adolescents. Am J Hum Biol. (2012) 24:183–5. doi: 10.1002/ajhb.22210
21. Wells JCK, Cole TJ. Height, adiposity and hormonal cardiovascular risk markers in childhood: how to partition the associations? Int J Obes. (2014) 38:930–5. doi: 10.1038/ijo.2014.24
22. Glaser Pediatric Research Network Obesity Study Group. Intraperitoneal fat and insulin resistance in obese adolescents. Obesity. (2010) 18:402–9. doi: 10.1038/oby.2009.261
23. Santos LC, Cintra Ide P, Fisberg M, Martini LA. Body trunk fat and insulin resistance in post-pubertal obese adolescents. Sáo Paulo Med J. (2008) 126:82–6. doi: 10.1590/S1516-31802008000200004
24. Tanaka M, Okada H, Hashimoto Y, Kumagai M, Nishimura H, Fukui M. Low-attenuation muscle is a predictor of diabetes mellitus: a population-based cohort study. Nutrition. (2020) 74:110752. doi: 10.1016/j.nut.2020.110752
25. Han SJ, Boyko EJ, Kim SK, Fujimoto WY, Kahn SE, Leonetti DL. Association of thigh muscle mass with insulin resistance and incident type 2 diabetes mellitus in Japanese Americans. Diabetes Metab J. (2018) 42:488–95. doi: 10.4093/dmj.2018.0022
26. Camporez JPG, Petersen MC, Abudukadier A, Moreira GV, Jurczak MJ, Friedman G, et al. Anti-myostatin antibody increases muscle mass and strength and improves insulin sensitivity in old mice. Proc Natl Acad Sci USA. (2016) 113:2212–7. doi: 10.1073/pnas.1525795113
27. He Q, Wang X, Yang C, Zhuang X, Yue Y, Jing H, et al. Metabolic and nutritional characteristics in middle-aged and elderly sarcopenia patients with type 2 diabetes. J Diabetes Res. (2020) 2020:6973469. doi: 10.1155/2020/6973469
28. Yajnik CS, Yudkin JS. The Y-Y paradox. Lancet. (2004) 363:163. doi: 10.1016/S0140-6736(03)15269-5
29. Wells JCK. Toward body composition reference data for infants, children, and adolescents. Adv Nutr. (2014) 5:320S−9S. doi: 10.3945/an.113.005371
30. Javed A, Jumean M, Murad MH, Okorodudu D, Kumar S, Somers VK, et al. Diagnostic performance of body mass index to identify obesity as defined by body adiposity in children and adolescents: a systematic review and meta-analysis. Pediatr Obes. (2015) 10:234–44. doi: 10.1111/ijpo.242
31. Córdoba-Rodríguez DP, Iglesia I, Gomez-Bruton A, Rodríguez G, Casajús JA, Morales-Devia H, et al. Fat-free/lean body mass in children with insulin resistance or metabolic syndrome: a systematic review and meta-analysis. BMC Pediatr. (2022) 22:58. doi: 10.1186/s12887-021-03041-z
32. Di Bonito P, Valerio G, Licenziati MR, Campana G, Del Giudice EM, Di Sessa A, et al. Uric acid, impaired fasting glucose and impaired glucose tolerance in youth with overweight and obesity. Nutr Metab Cardiovasc Dis. (2021) 31:675–80. doi: 10.1016/j.numecd.2020.10.007
33. Takase M, Nakamura T, Hirata T, Tsuchiya N, Kogure M, Itabashi F, et al. Association between fat mass index, fat-free mass index and hemoglobin A1c in a Japanese population: the Tohoku Medical Megabank Community-based Cohort Study. J Diabetes Investig. (2021) doi: 10.1111/jdi.13729. [Epub ahead of print].
Keywords: fat-free mass, children, glucose metabolism, systematic review, meta-analysis
Citation: Wu L, Chen F, Liu J, Hou D, Li T, Chen Y and Liao Z (2022) The Relationship Between Fat-Free Mass and Glucose Metabolism in Children and Adolescents: A Systematic Review and Meta-Analysis. Front. Pediatr. 10:864904. doi: 10.3389/fped.2022.864904
Received: 29 January 2022; Accepted: 31 March 2022;
Published: 26 April 2022.
Edited by:
Ronald Cohen, The University of Chicago, United StatesReviewed by:
Anna Di Sessa, University of Campania Luigi Vanvitelli, ItalyPaulina Correa, University of Chile, Chile
Copyright © 2022 Wu, Chen, Liu, Hou, Li, Chen and Liao. This is an open-access article distributed under the terms of the Creative Commons Attribution License (CC BY). The use, distribution or reproduction in other forums is permitted, provided the original author(s) and the copyright owner(s) are credited and that the original publication in this journal is cited, in accordance with accepted academic practice. No use, distribution or reproduction is permitted which does not comply with these terms.
*Correspondence: Fangfang Chen, YWlyZWNoZW5AMTI2LmNvbQ==