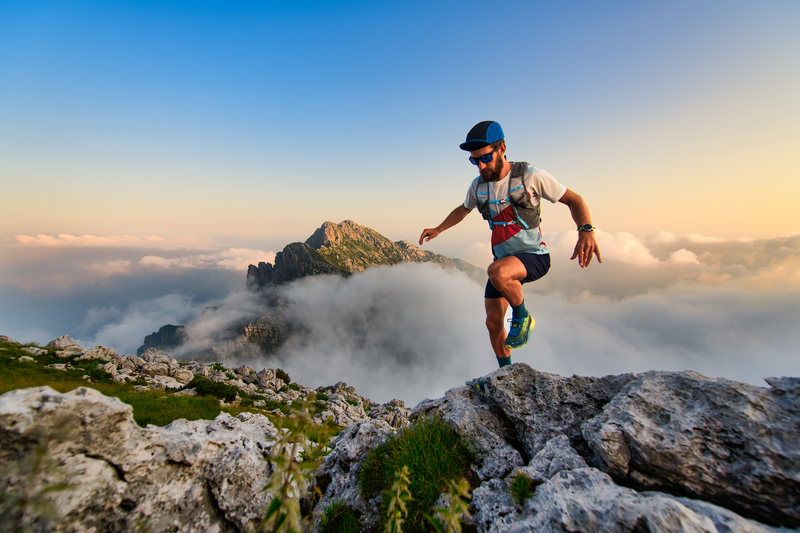
95% of researchers rate our articles as excellent or good
Learn more about the work of our research integrity team to safeguard the quality of each article we publish.
Find out more
PERSPECTIVE article
Front. Pediatr. , 10 May 2022
Sec. Pediatric Critical Care
Volume 10 - 2022 | https://doi.org/10.3389/fped.2022.864755
This article is part of the Research Topic Insights in Pediatric Critical Care: 2021 View all 11 articles
Pediatric intensivists are bombarded with more patient data than ever before. Integration and interpretation of data from patient monitors and the electronic health record (EHR) can be cognitively expensive in a manner that results in delayed or suboptimal medical decision making and patient harm. Machine learning (ML) can be used to facilitate insights from healthcare data and has been successfully applied to pediatric critical care data with that intent. However, many pediatric critical care medicine (PCCM) trainees and clinicians lack an understanding of foundational ML principles. This presents a major problem for the field. We outline the reasons why in this perspective and provide a roadmap for competency-based ML education for PCCM trainees and other stakeholders.
Pediatric intensivists are bombarded with more patient data than ever before. The density and complexity of data generated from patients, their monitoring devices, and electronic health records (EHR) pose significant cognitive challenges. Clinicians are required to integrate data from a variety of sources to inform medical decision-making, which is further challenged by high stakes, time-sensitivity, uncertainty, missing data, and organizational limitations (1, 2).
These constraints make critical care environments a compelling use case for artificial intelligence (AI) in medicine. AI is an umbrella term that contains multiple techniques and approaches. Modern advances in AI have largely been driven by machine learning (ML) methods such as supervised, unsupervised, deep, and reinforcement learning (3). ML has the potential to decrease cognitive load and enhance decision making at the point of care. Additionally, ML may be uniquely suited to analyzing the heterogeneous data generated during care and quantifying the complex determinants of the behavior of critically ill patients. Techniques, expectations, and infrastructures for developing and utilizing ML have matured, and there are many examples of ML algorithms published in the critically ill adult (4–8) and pediatric (9–16) literature that robustly predict morbidities and mortality.
However, these algorithms are at risk of being deployed in an environment where many intended end-users currently lack a basic understanding of how they work (17–20). We argue that the ML education gap in pediatric critical care medicine (PCCM) presents a major problem for the field because it may contribute to either distrust or blind trust of ML, both of which may harm patients.
Clinician distrust of ML is inversely associated with clinician engagement with the ML tool. Distrustful, disengaged clinicians are less likely to use even well-performing ML (21), limiting potential benefits to patients. Distrust may also manifest as missed opportunities to demystify the technology for trainees, recruit clinician champions for future ML projects, and realize the return on institutional and/or extramural investment. Distrust can therefore be enormously costly—in both non-monetary and monetary terms—and efforts to combat clinician distrust in ML through extensive pre-integration education have been successfully employed in both adult (22) and pediatric critical care (23).
Conversely, blind trust of ML is also problematic for PCCM. Humans are prone to automation bias whereby automated decisions are implicitly trusted, especially when end-users poorly understand the subject matter (24). Automation bias has been reported in the ML literature, especially among inexperienced end-users (25). In PCCM, blind trust of ML has the potential to harm patients. Clinicians may make flawed decisions when inappropriately using algorithms developed using biased training datasets (26). Furthermore, algorithms may degrade in performance over time (27) and across different care settings (28). These phenomena may be more prevalent in ML developed from relatively small training datasets (12), as in PCCM. Pediatric intensivists must be able to critically appraise ML literature and any ML-based tool. Identifying strengths and weaknesses of any potential ML intervention is vital to its proper application at the bedside, and critically ill pediatric patients deserve the same rigor applied to ML as other important topics in PCCM.
After acknowledging that a ML knowledge gap exists in PCCM, we must make concerted efforts to close it for the benefit of our patients. Understanding foundational principles of ML will be required to effectively interact with many applications of ML, including diagnostic and therapeutic decision support systems (e.g., disease risk prediction models, treatment recommender systems, etc.). Published ML curricula for medical students (29, 30) and medical (31, 32) and surgical (33) subspecialities converge on several key domains such as the “critical appraisal of AI systems” and “ethical and legal implications” (34). We propose below an ML curriculum for PCCM trainees and stakeholders based on similar domains, but with key adaptations for clinicians caring for critically ill children where appropriate.
We used Kern's six step approach for curriculum development (35) as a guiding framework for our ML in PCCM curriculum (Table 1). After identifying the PCCM education gap problem above (Kern's Step 1), our group of experts in PCCM, medical education, and ML identified high-priority curricular needs (Kern's Step 2) based on previous literature (30) and group consensus using modified Delphi methodology (36). We determined specific curriculum objectives (Kern's Step 3), which were operationalized into measurable, enabling competencies. Competencies were designed to be checked “yes, achieved” or “no, not achieved” at the competition of the curriculum and/or PCCM training. We suggest educational strategies (Kern's Step 4) to achieve specific competencies.
Step 5 of Kern's approach relates to implementation, which is the practical deployment of the education strategies listed above within the context of PCCM training resources and modalities. Many of the forums/methods necessary to institute the ML in PCCM curriculum already exist in many programs, thereby increasing the feasibility of delivery. We outline implementation resources common to many PCCM training programs, organized by curriculum objective, in Figure 1. Implementation of the curriculum may be more challenging in institutions that lack these resources. Shared access to materials that can be delivered virtually (e.g., freely accessible online modules, ML conferences/webinars, discussion with computer scientists via video conference, etc.) may increase the feasibility of curriculum implementation at less resourced centers. Curriculum champions at early adopting centers can also provide mentorship and promote faculty development at centers that have the desire to implement the curriculum but lack ML experience or expertise.
Figure 1. One potential roadmap for leveraging existing curricular implementation resources common to many PCCM training programs. Resources are divided by the ML in PCCM curriculum objectives.
The final step of Kern's approach relates to evaluation and assessment (35). We recommend a multifaceted approach. Traditional pre-post assessments using the Kirkpatrick outcomes hierarchy (37) can collect objective data such as knowledge of core ML concepts and subjective data such as trainee confidence applying those concepts. These assessments should be combined with open-ended discussions with key stakeholders (i.e., educational and institutional leaders, clinical faculty, trainees, interprofessional team/allied health members, course teachers, etc.) regarding key outcomes of interest. This multifaceted approach acknowledges the known limitations of pre-post assessments in richly understanding how a curriculum impacts learners. Evaluations should be repeated longitudinally to measure retention and identify new high-yield curricular objectives for PCCM that may arise in the fast-changing field of ML.
The promise of ML to improve medical decision making and patient outcomes is tempered by an incomplete understanding of the technology in PCCM. This education gap presents a major problem for the field because trainees and key stakeholders are at risk for developing distrust or blind trust of ML, which may negatively impact patients. However, this problem also presents an opportunity to effectively close the education gap by instituting an ML in PCCM curriculum. Our multidisciplinary group is the first to present such a curriculum in this perspective, focusing on key high-yield objectives, measurable enabling competencies, and suggested educational strategies that can utilize existing resources common to many PCCM training programs. We hope to empower PCCM trainees and stakeholders with the skills necessary to rigorously evaluate ML and harness its potential to benefit patients.
The original contributions presented in the study are included in the article/supplementary material, further inquiries can be directed to the corresponding author/s.
DE, VH, and FM: literature search, background and rationale, writing all or part of the manuscript, critical revision of the manuscript, and editing of the manuscript. LR, AJ, and BM: critical revision of the manuscript and editing of the manuscript. MM: background and rationale, critical revision of the manuscript, and editing of the manuscript. All authors agree to be accountable for the content of the work. All authors contributed to the article and approved the submitted version.
VH was supported through an Ontario Graduate Scholarship, Canadian Institutes of Health Research Banting and Best Master's and Doctoral Awards, and Vector Institute Postgraduate Fellowship. LR was supported through a Canada Research Chair in Population Health Analytics (950-230702).
The authors declare that the research was conducted in the absence of any commercial or financial relationships that could be construed as a potential conflict of interest.
All claims expressed in this article are solely those of the authors and do not necessarily represent those of their affiliated organizations, or those of the publisher, the editors and the reviewers. Any product that may be evaluated in this article, or claim that may be made by its manufacturer, is not guaranteed or endorsed by the publisher.
1. Patel VL, Kaufman DR, Arocha JF. Emerging paradigms of cognition in medical decision-making. J Biomed Inform. (2002) 35:52–75. doi: 10.1016/S1532-0464(02)00009-6
2. Nemeth C, Blomberg J, Argenta C, Serio-Melvin ML, Salinas J, Pamplin J. Revealing ICU cognitive work through naturalistic decision-making methods. J Cogn Eng Decis Making. (2016) 10:350–68. doi: 10.1177/1555343416664845
3. Komorowski M. Artificial intelligence in intensive care: are we there yet? Intensive Care Med. (2019) 45:1298–300. doi: 10.1007/s00134-019-05662-6
4. Gutierrez G. Artificial intelligence in the intensive care unit. Crit Care. (2020) 24:101. doi: 10.1186/s13054-020-2785-y
5. Nassar AP Jr, Caruso P. ICU physicians are unable to accurately predict length of stay at admission: a prospective study. Int J Qual Health Care. (2016) 28:99–103. doi: 10.1093/intqhc/mzv112
6. Awad A, Bader-El-Den M, McNicholas J, Briggs J. Early hospital mortality prediction of intensive care unit patients using an ensemble learning approach. Int J Med Inform. (2017) 108:185–95. doi: 10.1016/j.ijmedinf.2017.10.002
7. Zeiberg D, Prahlad T, Nallamothu BK, Iwashyna TJ, Wiens J, Sjoding MW. Machine learning for patient risk stratification for acute respiratory distress syndrome. PLoS ONE. (2019) 14:e0214465. doi: 10.1371/journal.pone.0214465
8. Sottile PD, Albers D, Higgins C, Mckeehan J, Moss MM. The association between ventilator dyssynchrony, delivered tidal volume, and sedation using a novel automated ventilator dyssynchrony detection algorithm. Crit Care Med. (2018) 46:e151–e157. doi: 10.1097/CCM.0000000000002849
9. Lonsdale H, Jalali A, Ahumada L, Matava C. Machine learning and artificial intelligence in pediatric research: current state, future prospects, and examples in perioperative and critical care. J Pediatr. (2020) 221S:S3–S10. doi: 10.1016/j.jpeds.2020.02.039
10. Kennedy CE, Aoki N, Mariscalco M, Turley JP. Using time series analysis to predict cardiac arrest in a PICU. Pediatr Crit Care Med. (2015) 16:e332–9. doi: 10.1097/PCC.0000000000000560
11. Zhai H, Brady P, Li Q, Lingren T, Ni Y, Wheeler DS, et al. Developing and evaluating a machine learning based algorithm to predict the need of pediatric intensive care unit transfer for newly hospitalized children. Resuscitation. (2014) 85:1065–71. doi: 10.1016/j.resuscitation.2014.04.009
12. Williams JB, Ghosh D, Wetzel RC. Applying machine learning to pediatric critical care data*. Pediatr Crit Care Med. (2018) 19:599–608. doi: 10.1097/PCC.0000000000001567
13. Sánchez Fernández I, Sansevere AJ, Gaínza-Lein M, Kapur K, Loddenkemper T. Machine learning for outcome prediction in Electroencephalograph (EEG)-Monitored children in the intensive care unit. J Child Neurol. (2018) 33:546–53. doi: 10.1177/0883073818773230
14. Lee B, Kim K, Hwang H, Kim YS, Chung EH, Yoon J-S, et al. Development of a machine learning model for predicting pediatric mortality in the early stages of intensive care unit admission. Sci Rep. (2021) 11:1263. doi: 10.1038/s41598-020-80474-z
15. Bose SN, Greenstein JL, Fackler JC, Sarma SV, Winslow RL, Bembea MM. Early prediction of multiple organ dysfunction in the pediatric intensive care unit. Front Pediatr. (2021) 9:711104. doi: 10.3389/fped.2021.711104
16. Aczon MD, Ledbetter DR, Laksana E, Ho LV, Wetzel RC. Continuous prediction of mortality in the PICU: a recurrent neural network model in a Single-Center Dataset*. Pediatr Crit Care Med. (2021) 22:519–29. doi: 10.1097/PCC.0000000000002682
17. Wood EA, Ange BL, Miller DD. Are we ready to integrate artificial intelligence literacy into medical school curriculum: students and faculty survey. J Med Educ Curric Dev. (2021) 8:23821205211024078. doi: 10.1177/23821205211024078
18. Pinto Dos Santos D, Giese D, Brodehl S, Chon SH, Staab W, Kleinert R, et al. Medical students' attitude towards artificial intelligence: a multicentre survey. Eur Radiol. (2019) 29:1640–6. doi: 10.1007/s00330-018-5601-1
19. Oh S, Kim JH, Choi S-W, Lee HJ, Hong J, Kwon SH. Physician confidence in artificial intelligence: an online mobile survey. J Med Internet Res. (2019) 21:e12422. doi: 10.2196/12422
20. Mamdani M, Slutsky AS. Artificial intelligence in intensive care medicine. Intensive Care Med. (2021) 47:147–9. doi: 10.1007/s00134-020-06203-2
21. Verma AA, Murray J, Greiner R, Cohen JP, Shojania KG, Ghassemi M, et al. Implementing machine learning in medicine. CMAJ. (2021) 193:E1351–E1357. doi: 10.1503/cmaj.202434
22. Wijnberge M, Geerts BF, Hol L, Lemmers N, Mulder MP, Berge P, et al. Effect of a machine learning-derived early warning system for intraoperative hypotension vs standard care on depth and duration of intraoperative hypotension during elective noncardiac surgery: the HYPE randomized clinical trial. JAMA. (2020) 323:1052–60. doi: 10.1001/jama.2020.0592
23. Sendak MP, Ratliff W, Sarro D, Alderton E, Futoma J, Gao M, et al. Real-world integration of a sepsis deep learning technology into routine clinical care: implementation study. JMIR Med Inform. (2020) 8:e15182. doi: 10.2196/15182
24. Bond RR, Novotny T, Andrsova I, Koc L, Sisakova M, Finlay D, et al. Automation bias in medicine: the influence of automated diagnoses on interpreter accuracy and uncertainty when reading electrocardiograms. J Electrocardiol. (2018) 51:S6–S11. doi: 10.1016/j.jelectrocard.2018.08.007
25. Gaube S, Suresh H, Raue M, Merritt A, Berkowitz SJ, Lermer E, et al. Do as AI say: susceptibility in deployment of clinical decision-aids. NPJ Digit Med. (2021) 4:31. doi: 10.1038/s41746-021-00385-9
26. Obermeyer Z, Powers B, Vogeli C, Mullainathan S. Dissecting racial bias in an algorithm used to manage the health of populations. Science. (2019) 366:447–53. doi: 10.1126/science.aax2342
27. Subbaswamy A, Saria S. From development to deployment: dataset shift, causality, and shift-stable models in health AI. Biostatistics. (2020) 21:345–52. doi: 10.1093/biostatistics/kxz041
28. Wong A, Otles E, Donnelly JP, Krumm A, McCullough J, DeTroyer-Cooley O, et al. External validation of a widely implemented proprietary sepsis prediction model in hospitalized patients. JAMA Intern Med. (2021) 181:1065–70. doi: 10.1001/jamainternmed.2021.2626
29. McCoy LG, Nagaraj S, Morgado F, Harish V, Das S, Celi LA. What do medical students actually need to know about artificial intelligence? NPJ Digit Med. (2020) 3:86. doi: 10.1038/s41746-020-0294-7
30. Lee J, Wu AS, Li D, Kulasegaram KM. Artificial intelligence in undergraduate medical education: a scoping review. Acad Med. (2021) 96:S62–S70. doi: 10.1097/ACM.0000000000004291
31. Schuur F, Rezazade Mehrizi MH, Ranschaert E. Training opportunities of artificial intelligence (AI) in radiology: a systematic review. Eur Radiol. (2021) 31:6021–9. doi: 10.1007/s00330-020-07621-y
32. Valikodath NG, Cole E, Ting DSW, Campbell JP, Pasquale LR, Chiang MF, et al. Impact of artificial intelligence on medical education in ophthalmology. Transl Vis Sci Technol. (2021) 10:14. doi: 10.1167/tvst.10.7.14
33. Bilgic E, Gorgy A, Yang A, Cwintal M, Ranjbar H, Kahla K, et al. Exploring the roles of artificial intelligence in surgical education: a scoping review. Am J Surg. (2021). doi: 10.1016/j.amjsurg.2021.11.023
34. Sapci AH, Sapci HA. Artificial intelligence education and tools for medical and health informatics students: systematic review. JMIR Med Educ. (2020) 6:e19285. doi: 10.2196/19285
35. Singh MK, Gullett HL, Thomas PA. Using Kern's 6-step approach to integrate health systems science curricula into medical education. Acad Med. (2021) 96:1282. doi: 10.1097/ACM.0000000000004141
36. Humphrey-Murto S, Varpio L, Wood TJ, Gonsalves C, Ufholz L-A, Mascioli K, et al. The use of the Delphi and other consensus group methods in medical education research. Acad Med. (2017) 92:1491–8. doi: 10.1097/ACM.0000000000001812
Keywords: artificial intelligence, machine learning, pediatric critical care medicine, medical education, learning curricula
Citation: Ehrmann D, Harish V, Morgado F, Rosella L, Johnson A, Mema B and Mazwi M (2022) Ignorance Isn't Bliss: We Must Close the Machine Learning Knowledge Gap in Pediatric Critical Care. Front. Pediatr. 10:864755. doi: 10.3389/fped.2022.864755
Received: 28 January 2022; Accepted: 18 April 2022;
Published: 10 May 2022.
Edited by:
Jhuma Sankar, All India Institute of Medical Sciences, IndiaReviewed by:
Yves Ouellette, Mayo Clinic, United StatesCopyright © 2022 Ehrmann, Harish, Morgado, Rosella, Johnson, Mema and Mazwi. This is an open-access article distributed under the terms of the Creative Commons Attribution License (CC BY). The use, distribution or reproduction in other forums is permitted, provided the original author(s) and the copyright owner(s) are credited and that the original publication in this journal is cited, in accordance with accepted academic practice. No use, distribution or reproduction is permitted which does not comply with these terms.
*Correspondence: Daniel Ehrmann, ZGVocm1hbm5AdW1pY2guZWR1
†These authors share first authorship
Disclaimer: All claims expressed in this article are solely those of the authors and do not necessarily represent those of their affiliated organizations, or those of the publisher, the editors and the reviewers. Any product that may be evaluated in this article or claim that may be made by its manufacturer is not guaranteed or endorsed by the publisher.
Research integrity at Frontiers
Learn more about the work of our research integrity team to safeguard the quality of each article we publish.