- 1Graduate School, Beijing University of Chinese Medicine, Beijing, China
- 2Department of Pediatrics, China-Japan Friendship Hospital, Beijing, China
- 3International Medical Services, China-Japan Friendship Hospital, Beijing, China
- 4Institute of Clinical Medical Sciences, China-Japan Friendship Hospital, Beijing, China
Objectives: We, in a large survey of school students from Beijing, aimed to identify the minimal number of promising factors associated with central obesity and the optimal machine-learning algorithm.
Methods: Using a cluster sampling strategy, this cross-sectional survey was conducted in Beijing in early 2022 among students 6–14 years of age. Information was gleaned via online questionnaires and analyzed by the PyCharm and Python.
Results: Data from 11,308 children were abstracted for analysis, and 3,970 of children had central obesity. Light gradient boosting machine (LGBM) outperformed the other 10 models. The accuracy, precision, recall, F1 score, area under the receiver operating characteristic of LGBM were 0.769982, 0.688312, 0.612323, 0.648098, and 0.825352, respectively. After a comprehensive evaluation, the minimal set involving top 6 important variables that can predict central obesity with descent performance was ascertained, including father's body mass index (BMI), mother's BMI, picky for foods, outdoor activity, screen, and sex. Validation using the deep-learning model indicated that prediction performance between variables in the minimal set and in the whole set was comparable.
Conclusions: We have identified and validated a minimal set of six important factors that can decently predict the risk of central obesity when using the optimal LGBM model relative to the whole set.
Introduction
Childhood obesity is a global problem and it is increasing to epidemic proportions (1, 2). As reported by the Global Burden of Disease Study 2013, the prevalence of obesity in children and adolescents has substantially increased around the world, especially in developing countries, from 8.1% to 12.9% for boys and from 8.4% to 13.4% in girls in 2013 (3). In China, the prevalence of overweight or obesity was 5.3% in 1995, and this number was skyrocketed to 20.5% in 2010 (4). Given the facts that obesity in childhood frequently persists into adulthood and obesity is an established risk factor for many chronic diseases (5), a better understanding of the etiology of childhood obesity can facilitate the development of effective strategies for preventing this outcome and its resultant sequelae.
It is well known that obesity is a complex, multifactorial disease with a highly inheritable tendency. There is evidence that children who have parents/grandparents with obesity are at higher risk of becoming obese than others. Besides, lifestyle-related factors such as eating habits and sleep duration also play a contributory role in the development of childhood obesity. In the literature, the majority of studies have examined risk profiles of childhood obesity using body mass index, which is a reflection of general obesity. As compared with general obesity, central obesity is a strong risk factor for cardio-metabolic disorders in children and adolescents (6, 7) and their unfavorable prognoses (8–11), because the endocrine of abdominal fat is more vigorous (12). In an observational study, central obesity in children who were school-aged was found to be associated with the development of autoimmune diseases, but being overweight was not (13). To this point, it is important to determine the risk factors behind central obesity in children. In a large sample of children who were school-aged from Greece, frequent breakfast, snack consumption, and frequent participation in sedentary activities were the strongest lifestyle determinants of central obesity (14). Another study indicated that higher adherence to the Mediterranean dietary pattern and higher cardiorespiratory fitness were associated with lower waist circumference in preschool children (15). Considering the complex etiology of central obesity, delineation of potential nonlinear, collinear or synergistic contributions of individual risk factors is challenging and beyond the capability of traditional statistical methods, like Logistic regression analysis. Fortunately, advancements in machine-learning and deep-learning techniques can at least partly shed some light on this challenge (16), due to their versatility, power and scalability in solving large and highly complex tasks.
To produce more information, we decided to survey factors from both students and parents and employ machine-learning techniques, aiming to identify the minimal number of promising factors associated with central obesity and the optimal machine-learning algorithm with decent performance, which can be applied in practical settings to predict the risk of childhood central obesity.
Materials and methods
Study design and ethical approval
This survey is designed to cross-sectionally collect data from students and their parents. Students from 26 schools located in a suburban district (Ping Gu) of Beijing were surveyed during the first month of 2022. The implementation of this survey conformed to the principles in the Declaration of Helsinki, and was approved by the Ethics Committee of Beijing University of Chinese Medicine.
Study participants
Students aged 6–14 years from 8 primary schools and 18 junior schools in Ping Gu district formed the study participants. With the exception of severe endocrine disorders, including but not limiting to hyperthyroidism, hypothyroidism and diabetes mellitus, all students are deemed eligible for inclusion.
At first, this survey included 11,633 students whose parents or guardians were requested to complete the questionnaire on smartphone. Finally, 11,308 questionnaires were valid, with a return rate of 98%.
Data collection
Survey was deployed by means of self-designed questionnaire. This questionnaire is sent electronically to the parents or guardians of students who attended primary schools or junior schools in the form of QR code by their class teachers. The class teachers and school health physicians were trained online about how to understand and fill in the questionnaire.
This questionnaire was designed on a network platform named “Wenjuanxing” (available at https://www.wenjuan.com/). At the end of survey, data were downloaded as an Excel file from this platform and were checked by research scientists.
Quality control
Before circulating this questionnaire, reliability coefficient (alpha) was calculated a prior and it exceeded 0.85. As data from this survey were collected online, it is essential to ensure the quality. All data were double checked by trained staff. In case of missing data or data with extreme values, school class teachers were contacted by re-inviting the parents or guardians to provide or validate data.
Definition of central obesity
As students in this study are in growth periods, height-dependent central obesity is preferred for practical applications. To this point, the cut-off value of waist-to-height ratio (WHtR) is used to define central obesity, and this value is referenced based on age and sex. In the present study, the cut-off value of WHtR is set at 0.46 for girls and 0.48 for boys according to previous reports (17, 18).
Items in questionnaire
Items in the questionnaire were designed to cover information from both students and their parents. Information from students covered birth date, sex, gestational age (in weeks), pregnant and birth order, delivery mode (natural labor or cesarean section), twins (yes or no), birth weight (in grams), birth height (in centimeters), breastfeeding duration (in months), solid food introduction age (in months), weight (in kilograms), height (in centimeters), hip and waist circumference (in centimeters), chronic diseases, family history of diabetes and hypertension, lifestyle habits such as mean daily outdoor duration (in hours), mean daily sitting time (in hours), mean daily screen time (in hours), fall asleep time and sleep duration (in hours), eating habits (fussy eating or not, frequency of snacks and other food intakes), and stool customs (frequency and character). Height and weight were measured by school health physicians.
Information from parents included age, body height (self-reported in centimeters), weight (self-reported in kilograms), education, and family annual income (RMB).
Definition of items
Waist circumference was horizontally measured at about a centimeter above the navel, and hip circumference was at the most protruding point level of their hips. Medical history of students referred to chronic kidney disease, hypothyroidism, congenital heart disease, chronic respiratory diseases, and other chronic diseases diagnosed from second-class or above hospitals. Delivery modes included natural birth, c-section and forceps delivery. Pregnant order and delivery order were divided into 2 groups as <2 and ≥2. Fiber foods included fruits and vegetables in season and grains. Animal protein refers to meat and processed meat. Soy protein was to point to legume and bean product. Dietary supplements included tonics such as royal jelly. Fast food referred to foods that are high in energy and low in nutrition (such as hamburgers and French fries). Night meal was to point to eating within 2 h of bedtime. Sleep duration, duration of physical activity, and daily sitting time were separately calculated as the sum of both corresponding time on workdays × 5 and corresponding time on weekends × 2 divided by 7. Stool character was defined according to the Bristol Stool Scale (BSFS) (19), and it was divided into 4 categories: individual lumps like nuts; like sausages but lumpy; like sausages, but with cracks on the surface; like sausages and smooth and soft, fluffy, watery. Family history of diabetes or hypertension was expressed by the number of parents and grandparents who were clinically diagnosed with diabetes or hypertension. Education level of parents was divided into senior high school/technical secondary school and below, undergraduate/junior college, and graduate school or above. Family annual income was classified into <100,000, 100,000 to 300,000, and >300,000 RMB per year.
Statistical analyses
After quality control, data were imported into the R programming environment (Version 4.1.1) for cleaning. Multiple-choice items were encoded as numbers. Missing data were imputed for multiple times (N = 5) with the R MICE package if percentage of missing values for each item is less than 30%, and were removed otherwise.
Continuous data were checked for normality, and if satisfied, they are expressed as mean (standard deviation) and median (quartile range) otherwise. Categorical data are uniformly expressed as number (percentage). Depending on the presence or absence of central obesity, data were divided into two groups. The distribution of survey items on either continuous or categorical scale were compared between the central obesity group and non-central obesity group by using t-test, rank-rum test or χ2 test where appropriate.
Machine-learning and deep-learning models are implemented using Integrated Development Environment (IDE) PyCharm Community Edition (2018.1 ×64) shipping the Python language (version 3.7.6). Models were trained on 60% of participating students (the training set) and tested on the remaining 40% (the validation set) as an internal validation of the central obesity-prediction model. In this study, 11 machine-learning models were respectively trained, including Logistic regression, random forest, support vector machine (SVM), decision tree, K-nearest neighbors (KNN), gradient boosting machine (GBM), light gradient boosting machine (LGBM), extreme gradient boosting machine (XGBoost), Gaussian naive Bayes (gNB), multinomial naive Bayes (mNB), and Bernoulli naive Bayes (bNB). Additionally, two decision-level fusion techniques, hard-voting and soft-voting classifiers, were applied based on above 11 machine-learning models. Model performance was assessed from five aspects, that is, accuracy (the prediction of correct outcomes as a percentage of the total sample), precision (the probability of the sample that was predicted to be positive being positive), recall score (the probability of being predicted to be a positive sample in a sample that is positive), F1 score (the harmonic mean of precision and recall), and AUROC (area under the receiver operating characteristic). The optimal model was selected after a comprehensive weighing up of the five aspects.
Generally, incorporation of more variables can improve model performance. For practical reasons, identification of a minimal set of variables that can capture much of model variation is critical. To achieve this goal, each variable was assigned an importance value generated by the χ2-based Scikit-learn feature selection method and the Shapley additive explanation (SHAP) tool, with a larger value corresponding to more importance in prediction for central obesity. Then, the importance of all variables under study was ranked in a descending order, and from the largest to the smallest, a panel of machine-learning models were generated by additional incorporation of one variable each time. The cumulative model performance was assessed by means of accuracy, precision, and AUROC, which were used to determine the minimal set of important variables.
Further, the prediction performance of variables in the minimal set as compared with the whole set was tested by the deep-learning sequential model, which was separately constructed with three different optimizers (adaptive moment estimation [Adam], root mean square prop [RMSprop], and stochastic gradient descent [SGD]). Model accuracy and model loss were computed for comparison in both training set and validation set.
Results
Baseline characteristics
Finally, data from 11,308 children were abstracted for analysis, and 3,970 of children (35.1%) had central obesity. Upon stratification by central obesity, the baseline characteristics of 11,308 students are shown in Table 1.
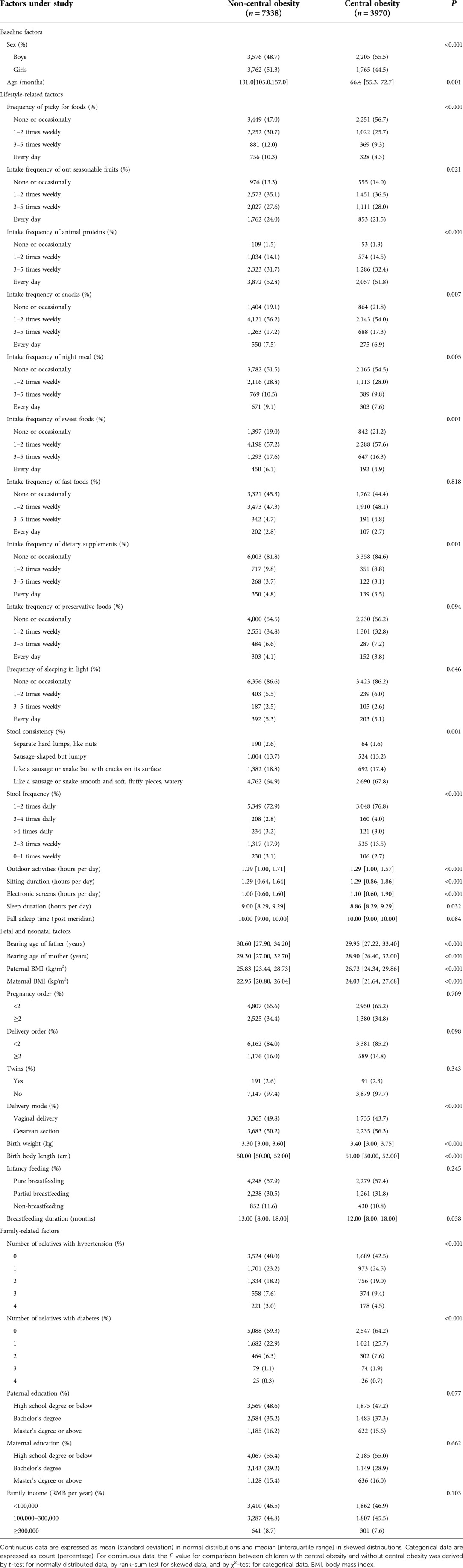
Table 1. The baseline characteristics of school students by the presence or absence of central obesity.
Selection of optimal machine-learning algorithm
Figure 1 presents the radar-based accuracy of 11 machine-learning models, along with hard-voting and soft-voting classifiers, and detailed assessment of model performance is displayed in Table 2.
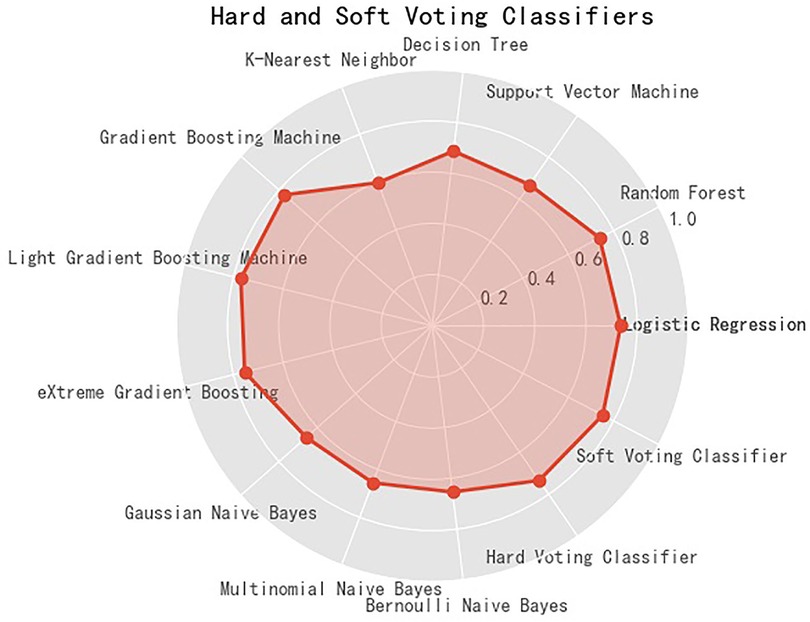
Figure 1. Accuracy of eleven machine learning models, along with hard-voting and soft-voting classifiers in predicting the risk of central obesity among school students. The solid red circle denotes the accuracy.
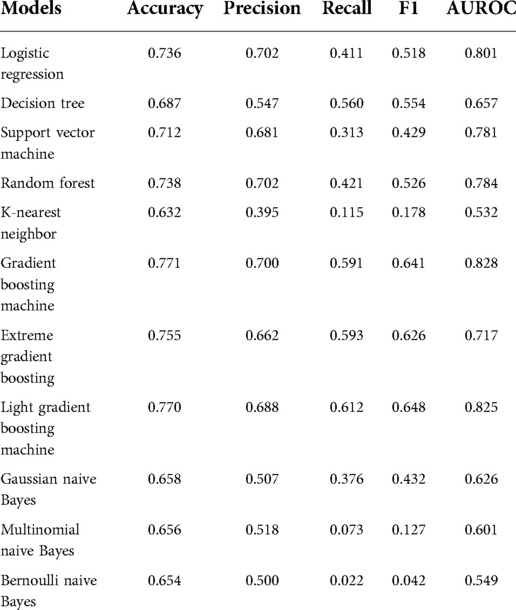
Table 2. Prediction performance of 11 machine-learning models for central obesity using accuracy, precision, recall, F1 score, and area under the receiver operating characteristic curve (AUROC).
After comparison, LGBM outperformed the other 10 models. The accuracy, precision, recall, F1 score, AUROC of LGBM were 0.769982, 0.688312, 0.612323, 0.648098, and 0.825352, respectively. Importantly, the accuracy of LGBM was comparable with that of hard-voting and soft-voting classifiers. Hence in this study, LGBM was identified as the overall best machine-learning model to predict central obesity in students aged 6 to 14 years.
Importance assessment and ascertainment of minimal set
Under the LGMB model, the importance of all studied variables was calculated and that of the top 20 variables is illustrated in Figure 2. The cumulative performance of top ten variables is shown in Table 3. After a comprehensive evaluation, the minimal set involving top 6 important variables that can predict central obesity with descent performance was ascertained, including father's BMI, mother's BMI, picky for foods, outdoor activity, screen, and sex.
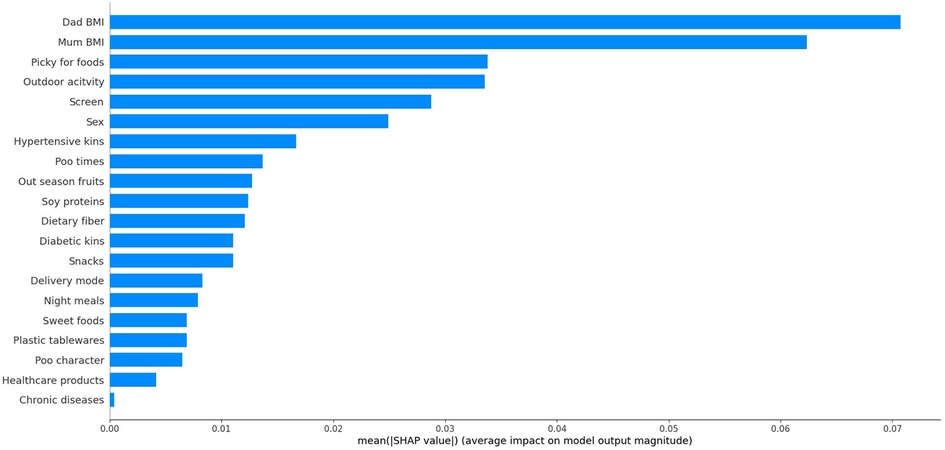
Figure 2. The ranking importance of top 20 factors associated with central obesity among school students.
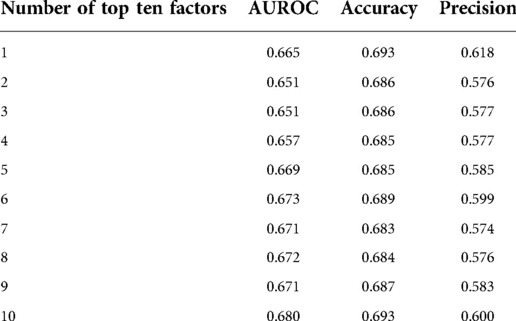
Table 3. The distributions of areas under the receiver operating curve (AUROC), accuracy, and precision with the cumulating number of top ten important factors associated with central obesity among school students.
Validation of minimal set
To validate whether variables in the minimal set can adequately predict central obesity relative to the whole set of variables involved, a deep-learning sequential model was adopted in both training set and validation set. As shown in Table 4, prediction performance between variables in the minimal set and in the whole set was comparable. For instance, using the optimizer Adam, model accuracy and model loss of the whole set were 67.07% and 20.28%, and that of the minimal set were 66.41% and 23.28% in the validation group.
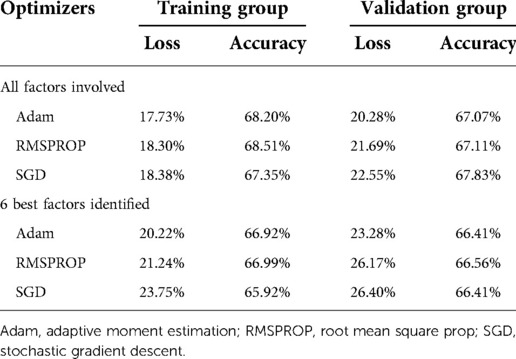
Table 4. Model loss and model accuracy estimates of deep learning sequential model in both training group and validation group.
Discussion
As an extension of our previous studies on general obesity and using traditional statistical models (20–23), we in this large survey, sought to identify factors in significant association with central obesity in students 6–14 years of age by use of artificial intelligence techniques. Importantly, we have identified and validated a minimal set of six important factors that can decently predict the risk of central obesity when using the optimal LGBM model relative to the whole set. The six factors are linked to central obesity of both parents, sex, and lifestyle behaviors of students. To our knowledge, this is the first study that has interrogated the risk profiles of central obesity in Chinese students in the literature.
As childhood obesity is established as a risk factor for a variety of adverse consequences in adulthood, it is of public health importance to propose an effective prediction tool for obesity and identify at-risk people at young age who might benefit from targeted interventions. Multiple prediction tools have been developed to predict obesity in children and adolescents; however, a lack of consistent reproducibility of these tools highlights the difficulties in identification of contributing factors and selection of proper models. Currently, the majority of prediction tools are developed by directly adopting linear (for continuous outcome) and Logistic (for categorical outcome) regression models, and these models cannot fully account for the collinearity and interaction of various factors. Bearing this in mind, we here adopted the advanced machine-learning and deep-learning techniques to solve these difficulties. These advanced techniques have been widely used in the medical field, especially for image recognition (24) and predicting/diagnosing diseases (25, 26).
It is widely recognized that obesity is a multifactorial disease, to which inherited and non-inherited factors contributed interactively. With the rapid economic growth and the global threat of COVID-19 pandemic, lifestyle-related behaviors have dramatically changed. For example, online education is not uncommon in modern life, and screen time in school children is related to obesity, physical activity, dry eyes, and learning ability (27). To this point, we have taken both conventional and modern lifestyle-related behaviors into consideration to identify factors associated with the risk of central obesity, a more pertinent marker than general obesity.
In this survey, the prevalence of central obesity in students from primary and junior schools was 35.1%, consistent with that of previous studies (14, 28). After a wide coverage of potential factors and the adoption of multiple machine-learning models, six important factors, including father's BMI, mother's BMI, picky for foods, outdoor activity, screen, and sex, under the LGBM model can soundly predict the risk of central obesity in school students, with performance parallel to the modeling of all factors involved. The contribution of individual factor identified to the development of central obesity is easily understandable. Taking obesity of both parents as an example, it is generally believed that obesity is “contagious”, as there is evidence that a child with one parent who is obesity is three-time more likely to become obese as an adult, while when a child's parents are both affected by obese, this child has a 10-fold risk of future obesity (29). On the other hand, family-based lifestyles in terms of dietary habits or outdoor activities, can also support the relation between obesity in parents and in offsprings (30). The contribution of individual factors to central obesity is easy to understand; however, how they act in the optimal LGBM model is elusive. Most machine-learning models (such as LGBM) are less transparent than others (such as decision tree), and their results are harder to interpret. Therefore, a high standard of transparency is required to allow parents and healthcare professionals to make informed decisions.
Some limitations should be acknowledged for this study. First, this survey is cross-sectional in nature, and so the cause-and-effect relationship between identified factors and central obesity cannot be addressed. Second, only students from a suburban district of Beijing were surveyed, and whether our findings can be extrapolated to other regions is an open question. Third, data were collected via online questionnaires, and recall bias cannot be totally ruled out, albeit strict quality control was made. Fourth, the findings of this study were only internally validated, and external validation in other independent groups is warranted.
Taken together, we have identified and validated a minimal set of six important factors that can decently predict the risk of central obesity when using the optimal LGBM model relative to the whole set. For the sake of clinical application, we expect that this study will not be just an end of research, but tread a path to the adoption of advanced artificial intelligence techniques in more clinical and epidemiological settings in the future.
Data availability statement
The raw data supporting the conclusions of this article will be made available by the authors, without undue reservation.
Ethics statement
The studies involving human participants were reviewed and approved by Ethics Committee of Beijing University of Chinese Medicine. Written informed consent to participate in this study was provided by the participants’ legal guardian/next of kin.
Author contributions
ZZ planned and designed the study and directed its implementation. ZZ and WN drafted the protocol. YZ, QW, MX, BP, and MY obtained statutory and ethics approvals. YZ and QW contributed to data acquisition. YZ and WN conducted statistical analyses. YZ, QW, MX, BP, MY, and XD did the data preparation and quality control. YZ and WN wrote the manuscript. All authors contributed to the article and approved the submitted version.
Acknowledgments
We thank all the students and their guardians who participated in this study for their cooperation and the generous support of the schools.
Conflict of interest
The authors declare that the research was conducted in the absence of any commercial or financial relationships that could be construed as a potential conflict of interest.
Publisher's note
All claims expressed in this article are solely those of the authors and do not necessarily represent those of their affiliated organizations, or those of the publisher, the editors and the reviewers. Any product that may be evaluated in this article, or claim that may be made by its manufacturer, is not guaranteed or endorsed by the publisher.
References
1. Koletzko B, Fishbein M, Lee WS, Moreno L, Mouane N, Mouzaki M, et al. Prevention of childhood obesity: a position paper of the global federation of international societies of paediatric gastroenterology, hepatology and nutrition (FISPGHAN). J Pediatr Gastroenterol Nutr. (2020) 70(5):702–10. doi: 10.1097/MPG.0000000000002708
2. Spinelli A, Buoncristiano M, Nardone P, Starc G, Hejgaard T, Júlíusson PB, et al. Thinness, overweight, and obesity in 6- to 9-year-old children from 36 countries: the world health organization European childhood obesity surveillance initiative-COSI 2015–2017. Obes Rev. (2021) 22(Suppl 6):e13214. doi: 10.1111/obr.13214
3. Ng M, Fleming T, Robinson M, Thomson B, Graetz N, Margono C, et al. Global, regional, and national prevalence of overweight and obesity in children and adults during 1980–2013: a systematic analysis for the Global Burden of Disease Study 2013. Lancet. (2014) 384(9945):766–81. doi: 10.1016/S0140-6736(14)60460-8
4. Dong Y, Jan C, Ma Y, Dong B, Zou Z, Yang Y, et al. Economic development and the nutritional status of Chinese school-aged children and adolescents from 1995 to 2014: an analysis of five successive national surveys. Lancet Diabetes Endocrinol. (2019) 7(4):288–99. doi: 10.1016/s2213-8587(19)30075-0
5. Juonala M, Magnussen CG, Berenson GS, Venn A, Burns TL, Sabin MA, et al. Childhood adiposity, adult adiposity, and cardiovascular risk factors. N Engl J Med. (2011) 365(20):1876–85. doi: 10.1056/NEJMoa1010112
6. Gautier A, Roussel R, Ducluzeau PH, Lange C, Vol S, Balkau B, et al. Increases in waist circumference and weight as predictors of type 2 diabetes in individuals with impaired fasting glucose: influence of baseline BMI: data from the DESIR study. Diabetes Care. (2010) 33(8):1850–2. doi: 10.2337/dc10-0368
7. Ashwell M, Gunn P, Gibson S. Waist-to-height ratio is a better screening tool than waist circumference and BMI for adult cardiometabolic risk factors: systematic review and meta-analysis. Obes Rev. (2012) 13(3):275–86. doi: 10.1111/j.1467-789X.2011.00952.x
8. Sahakyan KR, Somers VK, Rodriguez-Escudero JP, Hodge DO, Carter RE, Sochor O, et al. Normal-weight central obesity: implications for total and cardiovascular mortality. Ann Intern Med. (2015) 163(11):827–35. doi: 10.7326/m14-2525
9. Parente EB, Harjutsalo V, Forsblom C, Groop PH. The impact of central obesity on the risk of hospitalization or death due to heart failure in type 1 diabetes: a 16-year cohort study. Cardiovasc Diabetol. (2021) 20(1):153. doi: 10.1186/s12933-021-01340-4
10. Katzmarzyk PT, Hu G, Cefalu WT, Mire E, Bouchard C. The importance of waist circumference and BMI for mortality risk in diabetic adults. Diabetes Care. (2013) 36(10):3128–30. doi: 10.2337/dc13-0219
11. Mohammadi H, Ohm J, Discacciati A, Sundstrom J, Hambraeus K, Jernberg T, et al. Abdominal obesity and the risk of recurrent atherosclerotic cardiovascular disease after myocardial infarction. Eur J Prev Cardiol. (2020) 27(18):1944–52. doi: 10.1177/2047487319898019
12. Chait A, den Hartigh LJ. Adipose tissue distribution, inflammation and its metabolic consequences, including diabetes and cardiovascular disease. Front Cardiovasc Med. (2020) 7:22. doi: 10.3389/fcvm.2020.00022
13. Raisanen L, Lommi S, Engberg E, Kolho KL, Viljakainen H. Central obesity in school-aged children increases the likelihood of developing paediatric autoimmune diseases. Pediatr Obes. (2022) 17(3):e12857. doi: 10.1111/ijpo.12857
14. Grigorakis DA, Georgoulis M, Psarra G, Tambalis KD, Panagiotakos DB, Sidossis LS. Prevalence and lifestyle determinants of central obesity in children. Eur J Nutr. (2016) 55(5):1923–31. doi: 10.1007/s00394-015-1008-9
15. Labayen Goni I, Arenaza L, Medrano M, Garcia N, Cadenas-Sanchez C, Ortega FB. Associations between the adherence to the Mediterranean diet and cardiorespiratory fitness with total and central obesity in preschool children: the PREFIT project. Eur J Nutr. (2018) 57(8):2975–83. doi: 10.1007/s00394-017-1571-3
16. Geron A. Hands-on machine learning with scikit-learn & tensorflow: O’Reilly Media, inc. 1005. Sebastopol, CA: Gravenstein Highway North (2017).
17. Dai Y, Fu J, Liang L, Gong C, Xiong F, Liu G, et al. A proposal for the cutoff point of waist-to-height for the diagnosis of metabolic syndrome in children and adolescents in six areas of China. Chin J Epidemiol. (2014) 35(08):882–5. doi: 10.3760/cma.j.issn.0254-6450.2014.08.002
18. Liu B, Jiang R, Li P, Liu C, Li L. Cutoff waist-to-height and waist-to-hip ratios for metabolic syndrome in Chinese children and adolescents. J China Med Univ. (2017) 46(5):434–43. doi: 10.12007/j.issn.0258-4646.2017.05.013
19. Lewis SJ, Heaton KW. Stool form scale as a useful guide to intestinal transit time. Scand J Gastroenterol. (1997) 32(9):920–4. doi: 10.3109/00365529709011203
20. Wang Q, Yang M, Deng X, Wang S, Zhou B, Li X, et al. Explorations on risk profiles for overweight and obesity in 9501 preschool-aged children. Obes Res Clin Pract. (2022) 16(2):106–14. doi: 10.1016/j.orcp.2022.02.007
21. Zhou B, Yuan Y, Wang K, Niu W, Zhang Z. Interaction effects of significant risk factors on overweight or obesity among 7222 preschool-aged children from Beijing. Aging (Albany NY). (2020) 12(15):15462–77. doi: 10.18632/aging.103701
22. Liu S, Lei J, Ma J, Ma Y, Wang S, Yuan Y, et al. Interaction between delivery mode and maternal age in predicting overweight and obesity in 1,123 Chinese preschool children. Ann Transl Med. (2020) 8(7):474. doi: 10.21037/atm.2020.03.128
23. Liu S, Zhang J, Ma J, Shang Y, Ma Y, Zhang X, et al. Synergistic interaction between bedtime and eating speed in predicting overweight and obesity in Chinese preschool-aged children. Aging (Albany NY). (2019) 11(7):2127–37. doi: 10.18632/aging.101906
24. Visvikis D, Cheze Le Rest C, Jaouen V, Hatt M. Artificial intelligence, machine (deep) learning and radio(geno)mics: definitions and nuclear medicine imaging applications. Eur J Nucl Med Mol Imaging. (2019) 46(13):2630–7. doi: 10.1007/s00259-019-04373-w
25. Lian C, Liu M, Wang L, Shen D. Multi-Task weakly-supervised attention network for dementia Status estimation with structural MRI. IEEE Trans Neural Netw Learn Syst. (2021) 33(8):4056–68. doi: 10.1109/tnnls.2021.3055772
26. Lin A, Manral N, McElhinney P, Killekar A, Matsumoto H, Kwiecinski J, et al. Deep learning-enabled coronary CT angiography for plaque and stenosis quantification and cardiac risk prediction: an international multicentre study. Lancet Digit Health. (2022) 4(4):e256–65. doi: 10.1016/s2589-7500(22)00022-x
27. Mineshita Y, Kim HK, Chijiki H, Nanba T, Shinto T, Furuhashi S, et al. Screen time duration and timing: effects on obesity, physical activity, dry eyes, and learning ability in elementary school children. BMC Public Health. (2021) 21(1):422. doi: 10.1186/s12889-021-10484-7
28. Wang Y, Beydoun MA, Min J, Xue H, Kaminsky LA, Cheskin LJ. Has the prevalence of overweight, obesity and central obesity levelled off in the United States? Trends, patterns, disparities, and future projections for the obesity epidemic. Int J Epidemiol. (2020) 49(3):810–23. doi: 10.1093/ije/dyz273
29. Corica D, Aversa T, Valenzise M, Messina MF, Alibrandi A, De Luca F, et al. Does family history of obesity, cardiovascular, and metabolic diseases influence onset and severity of childhood obesity? Front Endocrinol (Lausanne). (2018) 9:187. doi: 10.3389/fendo.2018.00187
Keywords: central obesity, school students, artificial intelligence, risk factors, prediction
Citation: Zhang Y, Wang Q, Xue M, Pang B, Yang M, Zhang Z and Niu W (2022) Identifying factors associated with central obesity in school students using artificial intelligence techniques. Front. Pediatr. 10:1060270. doi: 10.3389/fped.2022.1060270
Received: 3 October 2022; Accepted: 14 November 2022;
Published: 30 November 2022.
Edited by:
Artur Mazur, University of Rzeszow, PolandReviewed by:
Rahim Alhamzawi, University of Al-Qadisiyah, IraqDavid Aebisher, University of Rzeszow, Poland
© 2022 Zhang, Wang, Xue, Pang, Yang, Zhang and Niu. This is an open-access article distributed under the terms of the Creative Commons Attribution License (CC BY). The use, distribution or reproduction in other forums is permitted, provided the original author(s) and the copyright owner(s) are credited and that the original publication in this journal is cited, in accordance with accepted academic practice. No use, distribution or reproduction is permitted which does not comply with these terms.
*Correspondence: Wenquan Niu niuwenquan_shcn@163.com Zhixin Zhang zhangzhixin032@163.com
†These authors share first authorship
Specialty Section: This article was submitted to Pediatric Endocrinology, a section of the journal Frontiers in Pediatrics