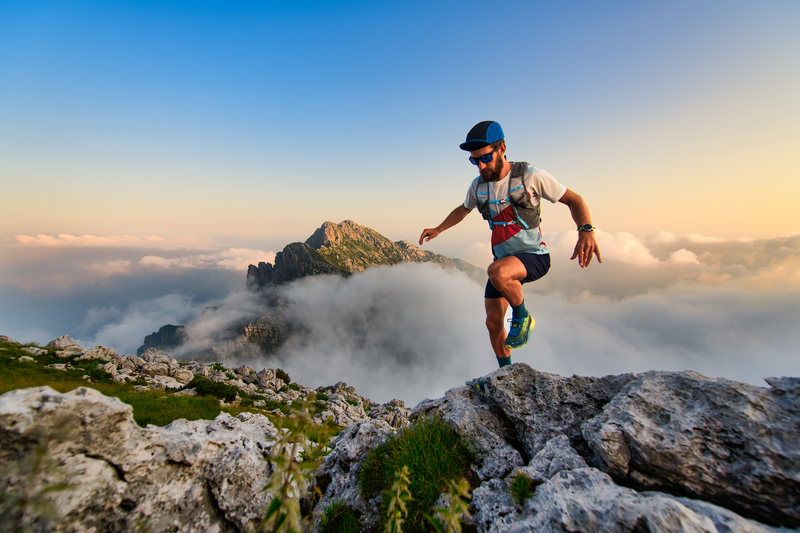
94% of researchers rate our articles as excellent or good
Learn more about the work of our research integrity team to safeguard the quality of each article we publish.
Find out more
BRIEF RESEARCH REPORT article
Front. Pediatr. , 15 February 2022
Sec. Pediatric Immunology
Volume 9 - 2021 | https://doi.org/10.3389/fped.2021.804912
Severe combined immunodeficiencies (SCIDs) correspond to the most severe form of primary immunodeficiency. Allogeneic hematopoietic stem cell transplantation (HSCT) and gene therapy are curative treatments, depending on the donor's availability and molecular diagnostics. A partially human leukocyte antigen (HLA)-compatible donor used has been developed for this specific HSCT indication in the absence of a matched donor. However, the CD34+ selected process induces prolonged post-transplant T-cell immunodeficiency. The aim here was to investigate a modeling approach to predict the time course and the extent of CD4+ T-cell immune reconstitution after CD34+ selected transplantation. We performed a Bayesian approach based on the age-related changes in thymic output and the cell proliferation/loss model. For that purpose, we defined specific individual covariates from the data collected from 10 years of clinical practice and then evaluated the model's predicted performances and accuracy. We have shown that this Bayesian modeling approach predicted the time course and extent of CD4+ T-cell immune reconstitution after SCID transplantation.
Severe combined immunodeficiencies (SCIDs) constitute a heterogeneous group of inherited disorders with a profound T-cell count reduction (1). Graft recipients with a matched sibling donor are curative and have the best clinical outcomes (2–4). However, in the absence of matched sibling donors, the optimal alternative donors and cell therapy strategies are subject to debate. Prolonged T-cell immunodeficiency observed after conventional T-cell graft depletion by CD34+ cell selection is a troublesome barrier to better clinical outcomes (5). Despite their immunodeficiency, SCID patients may manifest graft rejection or loss, and a small part of typical SCID will attain poor immune reconstitution (6). Recently published cohort studies suggest that a CD4+ T-cell count >500/mm3 in patients with SCID at 6 and 12 months after hematopoietic stem cell transplantation (HSCT) predicts long-term survival sustained immune reconstitution (7, 8). A challenging problem in this domain is giving value to interpreting the early time point of T-cell reconstitution. Therefore, a new approach is needed to make a faster and more robust analysis of post-transplant immune reconstitution. The aim here is to investigate a modeling approach to predict the time course and extent of CD4+ T-cell immune reconstitution after SCID transplantation. A mixed-effects framework and early post-HSCT data can usefully obtain the approximate Bayesian computation for individual children's long-term reconstitution trajectories. We will test the algorithm's performance to compute faster immune reconstitution analysis.
In a retrospective study between January 2008 and December 2017, we included 32 consecutive patients with SCID having received a primary HLA-haploidentical CD34+ selected graft from a family member. Patients with a diagnosis of adenosine deaminase deficiency and intrathymic deficiency were excluded. The dataset used for model building and covariate analysis was collected during routine clinical practice in the Immunohematology and Rheumatology Department at Necker Children's Hospital (Paris, France). The myeloablative conditioning regimen (CR, when used) was based on a combination of busulfan, fludarabine (160 mg/m2), and serotherapy [5 or 10 mg/kg anti-thymocyte globulin (ATG) or 1 mg/kg alemtuzumab] (9). Only four patients treated before 2010 underwent myeloablative CR with a CY-BU myeloablative conditioning protocol (10). In the Omenn syndrome, we initiated a 3-month therapeutic course with cyclosporine for most cases. All patients and donors gave their written informed consent to the collection and anonymous analysis of HSCT-related data.
The mechanistic inference model of the CD4+ T-cell count was based on naive CD4+ T-cell homeostasis, age-related changes in the thymic output, and the cell proliferation/loss model optimized from the Hoare et al. model (11, 12).
The complete model from Hoare et al. can be summarized as follows:
Λ represents the thymic output of T cells and was coded as λ(t, τ) = λ0λageΔHSCT(t); λ0 corresponds to the theoretical thymic output (cell/days); λage stands for the age-scaling function of the thymic output; ΔHSCT(t) stands for the sigmoidal function to recover the thymic output (including both the time to recovery of the thymic output and the rate of recovery in thymic output parameters). The parameter p represents the proliferation rate and was coded as , where p0 stands for the theoretical proliferation rate (/days); X(t) stands for CD4 T-cell concentration with time t after HSCT; N(τ) stands for the expected total CD4 for a healthy child of age τ days; and y(τ) is the proportion of CD4 cells expressing Ki67 as a function of age. The parameter d stands for the cell loss rate and was coded as , where d0 stands for the theoretical cell loss rate (/days). This mechanistic non-linear mixed-effect model was applied to our data, and model parameters were estimated using Monolix software (version 2019, Lixoft, Antony, France) (13). The Monolix code is provided in the Supplementary Data.
We chose eight specific pre-HSCT covariate parameters that putatively influence CD4+ T-cell reconstitution after CD34+ selected stem cell transplantation: age at transplantation, the type of molecular defect, Omen syndrome, pre-HSCT viral diseases, the ATG dose level (5 or 10 mg/kg), the presence or absence of a CR, and the CD34+ or CD3+ count of the stem cell graft. Model parameters were estimated using the stochastic approximation expectation–maximization and Markov chain Monte Carlo algorithm. The Fisher information matrix and the likelihood were computed using stochastic approximation and importance sampling. We also used the likelihood ratio test (including the log-likelihood), Akaike information criterion, and Bayesian information criterion to test different hypotheses regarding the covariate effects on parameters, the residual error model (additive, proportional, or combined), and the structure of the variance–covariance matrix between-subject variability parameters. A sensitivity analysis was also conducted by excluding patients with “other” diagnosis, and model parameters were re-estimated.
The model's goodness of fit was evaluated by a visual inspection of the observed–predicted confrontation (population and individual) scatter plots. Diagnostic graphics and other statistics (including the visual predictive check and normalized prediction distribution errors) were generated using R software (14).
We simulated different reconstitution profiles with one data point per month (1,000 Monte Carlo simulations from the final model) (15). The simulated CD4+ T-cell count at 12 months was predicted (using Bayesian forecasting) from 3- or 6-month truncated simulated trajectories. The probability of a correctly predicted CD4+ T-cell count <500/mm3 at 12 months defined the sensitivity. The method's specificity was defined as the probability of correctly predicting a CD4+ T-cell count >500/mm3 at 12 months. Our model's accuracy was determined by the overall probability of correctly classifying a patient with a CD4+ T cell > or <500/mm3 at 12 months.
A total of 231 data points for the T-cell phenotype of 32 consecutive patients were available for the 2 years following HSCT and were included in the immune reconstitution model (Figure 1). We obtained a median of seven samples per patient (range: 3–23 samples) over a median follow-up period of 20 months (range: 3–24). The patients' characteristics and outcomes are summarized in Table 1. Five of the patients (15.6%) died during the study period: four from infectious diseases and one from GvHD, followed by an infection. Two patients did not engraft, and the cumulative incidence of acute grade ≥II GvHD was 20.5%.
Figure 1. Immune reconstitution model. The prediction distribution from the final model. The blue area corresponds to the 95% prediction interval (PI). Blue points correspond to data for CD4+ T-cell reconstitution after HLA-haploidentical, CD34+ selected HSCT.
The one-compartment turnover model appeared to be suitable for comparing and assessing the time course of CD4+ T-cell reconstitution after HSCT. The thymic output increased from 2.7 months after HSCT [95%CI: (2.3–3.2)]. After transplantation, CD4+ T-cell counts had a lower distribution than age-appropriate reference. Interestingly, the 95th percentile of the observed values appeared within the normal range.
Observed vs. predicted CD4+ T-cell counts were close with the median (range) values, respectively, of 35 (0–576) vs. 35 (0–389) at 3 months, 414 (99–1,056) vs. 566 (108–1,059) at 6 months, and 564 (52–4,444) vs. 577 (67–2,661) at 12 months. The model parameters and diagnostic plots of the model's performance are provided in Figure 2.
Figure 2. Diagnostic plots from the final mode. (A) Scatter plot of the observed vs. predicted population CD4 T-cell counts. (B) Observed vs. predicted individual CD4 T-cell counts. (C) Normalized prediction distribution errors vs. time since transplantation. (D) Prediction-corrected visual predictive check plots. The green lines correspond to the observed data's 5th, 50th, and 95th percentiles. The shaded areas represent the 95% confidence interval around the simulated percentiles.
Genetic diagnosis and CR were the two pre-transplant covariates significantly associated with the time-course of T-cell reconstitution (p = 0.0047 and 0.01, respectively). Relative to patients with an IL2RG/JAK3 defect, patients with a RAG1 or ARTEMIS deficiency or another diagnosis had a 36.8% [95%CI: (18.3–73.6)] decrement in their thymic output function. Otherwise, CR significantly decreased the cell loss rate function [43% (22.5–82.1)]. The parameter estimates for the T-cell concentration at the time of HSCT were not influenced by ATG dosing, immediate pre-HSCT morbidities, or the stem cell graft's composition (Table 2). The sensitivity analysis excluding patients with “other” diagnosis showed that all structural parameters were very close to the primary analysis, suggesting the model's robustness. Furthermore, this sensitivity analysis showed that genetic diagnosis and CR were still significantly associated with the time course of T-cell reconstitution (p = 0.0396 and 0.015, respectively), supporting the primary analysis. The model parameters estimated from this sensitivity analysis are provided in the Supplementary Data.
Regarding covariate, our model provided four theoretical T-CD4+ immune reconstitution curves for SCID following HSCT with CD34+ selection: SCID T-B+ NK- or SCID T-B-NK+ with or without a CR (Figure 3). In addition, simulated change over time in the CD4+ T-cell count crossed into the normal reference range depending on the subgroup, especially for T-B+ SCID patients who received conditioning.
Figure 3. Immune reconstitution profiles. The PI for the simulated change over time in the CD4+ T-cell count time course for a child aged 6 months at the time of HSCT, as a function of significant covariate effects in the model (i.e., the presence or absence of a molecular defect and the presence or absence of conditioning). The continuous, dashed, and dotted black lines correspond to the median, 50%PI, and 95%PI, respectively. The green line and green area correspond to the age-appropriate reference value and the 95% lower and upper bound.
The population parameter means and variances found from the initial model fitting were used as the final Bayesian model's priors. For 1,000 simulated individuals, the overall accuracy in predicting the CD4+ reconstitution at 12 months with trajectories truncated at 3 and 6 months was 79 and 87%, respectively. The course truncated at 3 months had a low estimated sensitivity (41%) but very high specificity (88%). In contrast, the simulated trajectory truncated at 6 months was substantially more sensitive (74%) and still highly specific (90%).
Bayesian methods are becoming increasingly important in the biological sciences for inferring cellular networks, modeling biology systems, and integrating medical data (16, 17). Bayesian computation may provide significant benefits in terms of early post-transplant immune reconstitution investigation. For example, after stem cell transplantation for SCID, competent and fast immune reconstitution is the primary prognostic criterion, especially when considering severe pre-transplant infectious diseases.
The model fitted well with our retrospective data. Unfortunately, cross-validation was hard to provide in the case of rare diseases. Nevertheless, our modeling results were interestingly in line with the data from previous cohort studies (18). Computation reflected, in part, the physiopathology of post-HSCT CD4+ T-cell reconstitution for SCID. RAG1 and Artemis deficiencies are characterized by a defect of V(D)J recombination activity and altered thymic stroma, and T-B-NK+ SCID was associated in the model with a lower thymic output (19). On the other hand, CR decreased the cell loss rate function, suggesting better engraftment of a T-cell precursor.
As a prediction model, we decide to use only pretransplant covariates. Therefore, moderate-to-severe GvHD or post-transplant immune-suppressive treatment was not considered. Otherwise, the myeloid engraftment is associated with improved immune reconstitution, including long-term T-cell reconstitution. In recent years, there has been significant interest in exploring busulfan exposures in the sub-myeloablative range as a compromise between efficacy and safety and a guide for making treatment decisions. Unfortunately, we could not recover the data for BU exposure for all patients to implement our model. In addition, ATG pharmacokinetic studies were not performed in routine during this time of the study.
Other modeling strategies, based on multivariate immune reconstitution patterns, identified variables and described the correlation between immune system dysfunction and the occurrence of complications. Principal component analysis is a performant tool and is valuable for the simultaneous interpretation of different parameters (20, 21). However, those models need an exhaustive post-transplant multiparameter database, which is not adapted to rare diseases. Furthermore, they do not use single factors, and neither elaborate prediction adjusts to clinical practice.
A low number of patients, large dataset, and multiparameter studies do not also fit with conventional statistical methods, in which problems with mass significance can quickly occur. In contrast to a comparative statistical test at a single time point, the model provides a dynamic assessment of immune reconstitution while increasing substantially (by up to 10-fold) the ability to detect covariate effects. Our model also counts the variability in the number of data points per patient. In addition, the representation and use of the probability theory make Bayesian computation suitable for combining domain knowledge and data, avoiding to overfit a model to training data, and learning from incomplete datasets. The establishment of the model and parameter allowed us to predict a probability of distribution of T-CD4+ for each subpopulation. For example, in the T-B- group, nearly everyone received conditioning. However, only 1/11 patients were unconditioned due to pre-transplant severe comorbidities and a high risk of toxicity. Therefore, the Bayesian method did not consider only one patient but interpreted all data points with model and covariate adjustments to compute the curve of IR for the T-B- group.
However, a larger dataset may have also allowed us to point out other covariates. It could explain the remaining between individual variability and substantially. A larger data set may also increase the model's ability to predict personal CD4+ reconstitution very soon after transplantation. Post-transplant clinical events had a low incidence in our cohort. Contrary to other studies, we were not able to report the different immune reconstitution of T-CD4+ before the onset of GvHD, post-transplant viral infectious disease, or BCGitis (22).
The Bayesian inference is a data-driven technique; the prior probabilities of an event are updated when the new data are gathered. It was able to identify a relatively high proportion of satisfactorily simulated trajectories very soon after transplantation (i.e., within 3 months). A repetitive time point of phenotyping T cell until 6 months should be required to increase sensitivity and specificity substantially. CD4+ T-cell immune reconstitution is widely used and is a strong predictor of event-free survival (EFS), overall survival (OS), viral diseases, and reactivation or non-relapse mortality (NRM), depending on stem cell transplant indications (23–25). Only a few models attempt to predict T-CD4+ cell reconstitution (12, 26). In the context of new cell therapy approaches for T-cell depletion or in vitro thymic maturation, the mechanistic model presented here could be used to design clinical trials and provide early assessments of their results. Furthermore, it should be an exciting model to compare immune reconstitution from a different group of patients statistically. The Bayesian modeling approach predicted the time course and extent of CD4+ T-cell immune reconstitution after SCID transplantation.
The raw data supporting the conclusions of this article will be made available by the authors, without undue reservation.
J-SD and NB conceptualized, designed the study, and drafted the initial manuscript. MC performed the supervision of this work. All authors reviewed, revised, and approved the final manuscript as submitted and agreed to be accountable for all aspects of the work.
The authors declare that the research was conducted in the absence of any commercial or financial relationships that could be construed as a potential conflict of interest.
The Reviewer SP has declared a past collaboration with several of the authors DM and BN to the handling editor.
All claims expressed in this article are solely those of the authors and do not necessarily represent those of their affiliated organizations, or those of the publisher, the editors and the reviewers. Any product that may be evaluated in this article, or claim that may be made by its manufacturer, is not guaranteed or endorsed by the publisher.
The Supplementary Material for this article can be found online at: https://www.frontiersin.org/articles/10.3389/fped.2021.804912/full#supplementary-material
1. Bousfiha A, Jeddane L, Picard C, Ailal F, Gaspar HB, Al-Herz W, et al. The (2017). IUIS phenotypic classification for primary immunodeficiencies. J Clin Immunol. (2018) 38:129–43. doi: 10.1007/s10875-017-0465-8
2. Chan AY, Leiding JW, Liu X, Logan BR, Burroughs LM, Allenspach EJ, et al. Hematopoietic cell transplantation in patients with primary immune regulatory disorders (PIRD): a primary immune deficiency treatment consortium (PIDTC) survey. Front Immunol. (2020) 11:239. doi: 10.3389/fimmu.2020.00239
3. Cowan MJ, Neven B, Cavazanna-Calvo M, Fischer A, Puck J. Hematopoietic stem cell transplantation for severe combined immunodeficiency diseases. Biol Blood Marrow Transplant. (2008) 14:73–5. doi: 10.1016/j.bbmt.2007.10.017
4. Speckmann C, Doerken S, Aiuti A, Albert MH, Al-Herz W, Allende LM, et al. A prospective study on the natural history of patients with profound combined immunodeficiency: an interim analysis. J Allergy Clin Immunol. (2020) 139:1302–10. doi: 10.1016/j.jaci.2016.07.040
5. Bhatt ST, Bednarski JJ. Immune reconstitution in pediatric patients following hematopoietic cell transplant for non-malignant disorders. Front Immunol. (2020) 11:e01988. doi: 10.3389/fimmu.2020.01988
6. Haddad E., Allakhverdi Z, Griffith LM, Cowan MJ, Notarangelo LD. Survey on re-transplantation criteria for severe combined immunodeficiency patients. J Allergy Clin Immunol. (2014) 133:597–9. doi: 10.1016/j.jaci.2013.10.022
7. Haddad E, Logan BR, Griffith LM, Buckley RH, Parrott RE, Prockop SE, et al. SCID genotype and 6-month post-transplant CD4 count predict survival and immune recovery: a PIDTC retrospective study. Blood. (2018) 132:1737–49. doi: 10.1182/blood-2018-03-840702
8. Heimall J, Logan BR, Cowan MJ, Notarangelo LD, Griffith LM, Puck JM, et al. Immune reconstitution and survival of 100 SCID patients post–hematopoietic cell transplant: a PIDTC natural history study. Blood. (2017) 130:2718–27. doi: 10.1182/blood-2017-05-781849
9. Lankester AC, Albert MH, Booth C, Gennery AR, Güngör T, Hönig M, et al. EBMT/ESID inborn errors working party guidelines for hematopoietic stem cell transplantation for inborn errors of immunity. Bone Marrow Transplant. (2021) 56:2052–62. doi: 10.1038/s41409-021-01378-8
10. Neven B, Leroy S, Decaluwe H, Le Deist F, Picard C, Moshous D, et al. Long-term outcome after hematopoietic stem cell transplantation of a single-center cohort of 90 patients with severe combined immunodeficiency. Blood. (2009) 113:4114–24. doi: 10.1182/blood-2008-09-177923
11. Hapuarachchi T, Lewis J, Callard RE. A mechanistic model for naive CD4 T cell homeostasis in healthy adults and children. Front Immunol. (2013). 4:e00366. doi: 10.3389/fimmu.2013.00366
12. Hoare RL, Veys P, Klein N, Callard R, Standing JF. Predicting CD4 T-cell reconstitution following pediatric hematopoietic stem cell transplantation. Clin Pharmacol Ther. (2017) 102:349–57. doi: 10.1002/cpt.621
13. Monolix. Monolix version 2019R1. Antony, France: Lixoft SAS (2019). Available online at: http://lixoft.com/products/monolix/ (accessed October, 2021).
14. R Core Team,. R: A Language Environment for Statistical Computing. R Foundation for Statistical Computing, Vienna, Austria (2018). Available online at: https://www.R-project.org/ (accessed March, 2021).
15. De Leeuw J. Journal of statistical software. Wiley Interdiscip Rev Comput Stat. (2009) 1:128–9. doi: 10.1002/wics.10
16. Li Q, Dahl DB, Vannucci M, Joo H, Tsai JW. Bayesian model of protein primary sequence for secondary structure prediction. PLoS ONE. (2014) 9:e0109832. doi: 10.1371/journal.pone.0109832
17. Maity AK, Bhattacharya A, Mallick BK, Baladandayuthapani V. Bayesian data integration and variable selection for pan-cancer survival prediction using protein expression data. Biometrics. (2020) 76:316–25. doi: 10.1111/biom.13132
18. Lankester AC, Neven B, Mahlaoui N, von Asmuth EGJ, Courteille V, Alligon M, et al. Hematopoietic cell transplantation in severe combined immunodeficiency: the SCETIDE 2006-2014 European cohort. J Allergy Clin Immunol. (2021). doi: 10.1016/j.jaci.2021.10.017
19. Schuetz C, Neven B, Dvorak CC, Leroy S, Ege MJ, Pannicke U, et al. SCID patients with ARTEMIS vs. RAG deficiencies following HCT: increased risk of late toxicity in ARTEMIS-deficient SCID. Blood. (2014) 123:281–9. doi: 10.1182/blood-2013-01-476432
20. Koenig M, Huenecke S, Salzmann-Manrique E, Esser R, Quaritsch R, Steinhilber D, et al. Multivariate analyses of immune reconstitution in children after allo-SCT: risk-estimation based on age-matched leukocyte sub-populations. Bone Marrow Transplant. (2010) 45:613–21. doi: 10.1038/bmt.2009.204
21. Mellgren K, Nierop AFM, Abrahamsson J. Use of multivariate immune reconstitution patterns to describe immune reconstitution after allogeneic stem cell transplantation in children. Biol Blood Marrow Transplant. (2019) 25:2045–53. doi: 10.1016/j.bbmt.2019.06.018
22. Skert C, Perucca S, Chiarini M, Giustini V, Sottini A, Ghidini C, et al. Sequential monitoring of lymphocyte subsets and of T-and-B cell neogenesis indexes to identify time-varying immunologic profiles in relation to graft-versus-host disease and relapse after allogeneic stem cell transplantation. PLoS ONE. (2017) 12:e0175337. doi: 10.1371/journal.pone.0175337
23. Ogonek J, Juric MK, Ghimire S, Varanasi PR, Holler E, Greinix H, et al. Immune reconstitution after allogeneic hematopoietic stem cell transplantation. Front Immunol. (2016) 7:00507. doi: 10.3389/fimmu.2016.00507
24. Admiraal R, Lindemans CA, Van Kesteren C, Bierings MB, Versluijs AB, Nierkens S, et al. Excellent T-cell reconstitution and survival depend on low ATG exposure after pediatric cord blood transplantation. Blood. (2016) 128:2734–41. doi: 10.1182/blood-2016-06-721936
25. Drylewicz J, Schellens IMM, Gaiser R, Nanlohy NM, Quakkelaar ED, Otten H, et al. Rapid reconstitution of CD4 T cells and NK cells protects against CMV-reactivation after allogeneic stem cell transplantation. J Transl Med. (2016) 14:1–9. doi: 10.1186/s12967-016-0988-4
Keywords: Bayesian prediction algorithm, immune reconstitution, severe combined immunodeficiency (SCID), hematopoietic stem cell transplantation, CD34+ selection
Citation: Diana J-S, Bouazza N, Couzin C, Castelle M, Magnani A, Magrin E, Rosain J, Treluyer J-M, Picard C, Moshous D, Blanche S, Neven B and Cavazzana M (2022) Bayesian Modeling Immune Reconstitution Apply to CD34+ Selected Stem Cell Transplantation for Severe Combined Immunodeficiency. Front. Pediatr. 9:804912. doi: 10.3389/fped.2021.804912
Received: 29 October 2021; Accepted: 21 December 2021;
Published: 15 February 2022.
Edited by:
Fabio Candotti, Centre Hospitalier Universitaire Vaudois (CHUV), SwitzerlandReviewed by:
Andrew R. Gennery, Newcastle University, United KingdomCopyright © 2022 Diana, Bouazza, Couzin, Castelle, Magnani, Magrin, Rosain, Treluyer, Picard, Moshous, Blanche, Neven and Cavazzana. This is an open-access article distributed under the terms of the Creative Commons Attribution License (CC BY). The use, distribution or reproduction in other forums is permitted, provided the original author(s) and the copyright owner(s) are credited and that the original publication in this journal is cited, in accordance with accepted academic practice. No use, distribution or reproduction is permitted which does not comply with these terms.
*Correspondence: Jean-Sebastien Diana, amVhbi1zZWJhc3RpZW4uZGlhbmFAcGFzdGV1ci5mcg==
Disclaimer: All claims expressed in this article are solely those of the authors and do not necessarily represent those of their affiliated organizations, or those of the publisher, the editors and the reviewers. Any product that may be evaluated in this article or claim that may be made by its manufacturer is not guaranteed or endorsed by the publisher.
Research integrity at Frontiers
Learn more about the work of our research integrity team to safeguard the quality of each article we publish.