- 1Department of Pediatrics, University Hospitals Rainbow Babies & Children's Hospital, Cleveland, OH, United States
- 2Case Western Reserve University School of Medicine, Cleveland, OH, United States
- 3Department of Anesthesiology and Critical Care Medicine, University Hospitals Cleveland Medical Center, Cleveland, OH, United States
- 4Center for Reducing Health Disparities, MetroHealth Campus of Case Western Reserve University, Cleveland, OH, United States
- 5Center for Population Health Research, MetroHealth Campus of Case Western Reserve University, Cleveland, OH, United States
Objective: Technology-dependent children with medical complexity (CMC) are frequently admitted to the pediatric intensive care unit (PICU). The social risk factors for high PICU utilization in these children are not well described. The objective of this study was to describe the relationship between race, ethnicity, insurance status, estimated household income, and PICU admission following the placement of a tracheostomy and/or gastrostomy (GT) in CMC.
Study Design: This was a retrospective multicenter study of children <19 years requiring tracheostomy and/or GT placement discharged from a hospital contributing to the Pediatric Health Information System (PHIS) database between January 2016 and March 2019. Primary predictors included estimated household income, insurance status, and race/ethnicity. Additional predictor variables collected included patient age, sex, number of chronic complex conditions (CCC), history of prematurity, and discharge disposition following index hospitalization. The primary outcome was need for PICU readmission within 30 days of hospital discharge. Secondary outcomes included repeated PICU admissions and total hospital costs within 1 year of tracheostomy and/or GT placement.
Results: Patients requiring a PICU readmission within 30 days of index hospitalization for tracheostomy or GT placement accounted for 6% of the 20,085 included subjects. In multivariate analyses, public insurance [OR 1.28 (95% C.I. 1.12–1.47), p < 0.001] was associated with PICU readmission within 30 days of hospital discharge while living below the federal poverty threshold (FPT) was associated with a lower odds of 30-day PICU readmission [OR 0.7 (95% C.I. 0.51–0.95), p = 0.0267]. Over 20% (n = 4,197) of children required multiple (>1) PICU admissions within one year from index hospitalization. In multivariate analysis, Black children [OR 1.20 (95% C.I. 1.10–1.32), p < 0.001] and those with public insurance [OR 1.34 (95% C.I. 1.24–1.46), p < 0.001] had higher odds of multiple PICU admissions. Social risk factors were not associated with total hospital costs accrued within 1 year of tracheostomy and/or GT placement.
Conclusions: In a multicenter cohort study, Black children and those with public insurance had higher PICU utilization following tracheostomy and/or GT placement. Future research should target improving healthcare outcomes in these high-risk populations.
Introduction
Nearly one-third of health care costs for children are attributed to the one percent of children with medical complexity (CMC), in part due to their need for frequent hospitalizations (1, 2). In the United States (US), a subset of technology-dependent CMC rely upon life-sustaining medical technologies, including gastrointestinal and respiratory devices (3). Because of their medical fragility and the complication rate of medical devices, technology-dependent children are at risk for high hospital utilization, including frequent admissions and need for intensive care. An estimated 20% of admissions to a US pediatric intensive care unit (PICU) are children with a tracheostomy or gastrostomy tube (GT) (4).
The risk factors for PICU admission in technology-dependent children have not been well described but are likely similar to risk factors for general hospital admission including younger age and higher medical complexity (5). There is some evidence to suggest that technology-dependent children of minority race and those living in poverty with inadequate environmental conditions are at risk for repeated emergency department visits, prolonged hospital stays, and hospital readmission, and have a higher mortality (6–9).
The poor outcomes experienced by technology-dependent children could reflect the challenge families experience in caring for CMC at home (6). Families consistently report difficulties in both navigating the healthcare system and unmet health needs including lack of subspecialty care (10, 11). These challenges are likely much worse in socially and economically disadvantaged families. Optimal health outcomes for technology-dependent children require sophisticated care coordination centered within the medical home (10).
The relationship between poor living conditions and poor health in children is well documented (11). The impact of social factors including poverty, racism, inadequate insurance coverage, inadequate housing, food insecurity, and insufficient healthcare access may be most detrimental in technology-dependent children and mitigating the risk of PICU utilization requires understanding these risk factors. Yet little is known about the relationship between social factors and PICU utilization. Therefore, the primary objective of this study was to use a multicenter database of US children's hospitals to test the hypothesis that social factors including estimated household income, insurance status, and race predict high PICU utilization, defined as need for PICU readmission within 30-days of discharge from the hospital following initial placement of tracheostomy or GT. Secondary outcomes included repeated PICU admissions, and total hospital costs within 1 year following tracheostomy or GT placement.
Methods
Data Source
Data for this retrospective, multicenter cohort study was obtained from the Pediatric Health Information System (PHIS) a quality-controlled database of over 45 tertiary children's hospitals located in non-competing markets across the US associated with the Children's Hospital Association (Lenexa, KS). PHIS accounts for 15% of US pediatric hospitalizations and contains comprehensive patient-level data including demographics, admission and discharges dates, International Classification of Diseases, Tenth Revisions, Clinical Modification (ICD-10-CM, ICD-10-PCS) diagnosis and procedure codes, billing data, and disposition data (12). Although the database is de-identified, encrypted patient identification numbers allow investigators to track subjects across multiple hospitalizations. The study protocol was reviewed by the University Hospitals Institutional Review Board and deemed exempt from ethical approval and oversight (STUDY20201822).
Study Population
Children <19 years of age with a procedure code for a tracheostomy (ICD-10-PCS: 0B110F4, 0B113F4, 0B114F4) or GT (ICD-10-PCS: 0DH60UZ, 0DH63UZ, 0DH64UZ) discharged from a PHIS hospital from January 2016 through March 2019 were included in the study cohort as index hospitalizations and data collection continued for 365 days after discharge from index hospitalization, until March 2020 (6, 8).
Data Collection and Study Protocol
The primary outcome of interest was PICU utilization within 30 days of discharge from the index hospitalization. PICU utilization was defined by presence of the PICU “flag”, which identifies PICU level of care (excluding neonatal ICU care) through billing codes. Other outcomes examined included repeated (>1) PICU admissions and total accrued hospital costs within 365 days of discharge from index hospitalization, hospital length of stay (LOS) at index admission, 30-day hospital readmission, and discharge disposition for children requiring repeated hospital admissions within the one-year observation period. Because hospital readmissions and total hospital costs were outcomes of interest, children who died on the index admission or subsequent readmissions, and children with missing cost data were excluded.
The primary sociodemographic predictors included patient income, race/ethnicity, and insurance. The median household income provided by PHIS was obtained by linking patients' ZIP codes to data from the US census bureau. The 2016 federal poverty threshold (FPT) for a family of four with two children was $24,339 (13). For this study, subjects were categorized into four groups based on the FPT and its percentage multiples, which are commonly used as eligibility criterion for various federal programs (14). Race and ethnicity were categorized White, Black, Asian, Hispanic or Latino, or “other,” which included Pacific Islander, American Indian or Alaskan Native, other race, or mixed race. Insurance status was categorized into private, public or “other,” which included patients classified as charity, self-pay, no hospital bill associated with subject, or “other payor.” Additional predictor variables for the outcomes of interest included age, analyzed as a categorical variable, sex, number of chronic complex conditions (CCC) using the classification system proposed by Feudtner et al., history of prematurity or low birthweight, and discharge disposition at index hospitalization, categorized as discharge to home, discharge to home with health services, or discharge to another healthcare facility (Supplementary Table 1) (15, 16). Children with missing predictor variables including income, race/ethnicity, insurance, sex, or discharge disposition were excluded (Figure 1).
Statistical Analysis
The demographics of study subjects at index hospitalization were summarized using descriptive statistics. All variables were categorical and presented as frequencies and percentages. Univariate associations between predictor variables and outcomes of interest including PICU readmission within 30 days of index hospitalization, >1 PICU admission within 365 days of index hospitalization, 30-day hospital readmission, discharge disposition at index admission, and discharge disposition after repeated admissions, were analyzed using chi-square tests. Variables associated with these outcomes in univariate analysis (p < 0.05) and the primary predictors of interest (race, ethnicity, median household income and insurance status) regardless of statistical significance, were included in multivariate logistic regression analyses. For the continuous outcomes of hospital LOS at index admission and total hospital costs within 365 days of index hospitalization, we observed that these outcomes were positively skewed and violated the normality assumption. Therefore, we assessed the relationship between predictor variables and the continuous outcomes using univariate and multivariate generalized linear models using the gamma distribution with a log-link. Presence of multi-collinearity between the predictors was checked by the variation inflation factor [VIF] and no substantial multi-collinearity (defined as VIF > 5) was observed. For the outcome of discharge disposition after repeat hospital admissions, we included the population of patients who experienced a 365-day readmission, excluding those with an unknown discharge disposition on subsequent discharges (n = 22), for a final cohort of 14,959 patients. For patients experiencing multiple discharges during the one-year observation period, we categorized them based on the highest level of care used (Healthcare facility > Home with health services > Home). In the multinomial logistic regression analysis, we used “Home” as the comparison category. For all analyses, p < 0.05 were considered statistically significant and odds ratios were considered significant if the 95% confidence intervals did not include 1. All analyses were conducted using R software, version 4.0.3.
Results
There were 20,085 children <19 years of age who were admitted for a tracheostomy or GT placement to one of 49 PHIS hospitals during the study period. Demographics and clinical characteristics at index hospitalization are found in Table 1. Most children were <1 year of age (58%), White (62%), and had public insurance (63%). Over two-thirds of children lived in a low-income ZIP code where the median household income was <200% of the FPT. Nearly all children (n = 19,876) were classified as having a chronic complex condition, with technology-dependence (93%), gastrointestinal (89%), and neurologic/neuromuscular (34%) being the most common categories (Supplementary Table 2). Only 15% of children in this cohort were discharged home with health services, and there were differences in patient demographics and clinical characteristics based on discharge disposition (Supplementary Tables 1, 3).
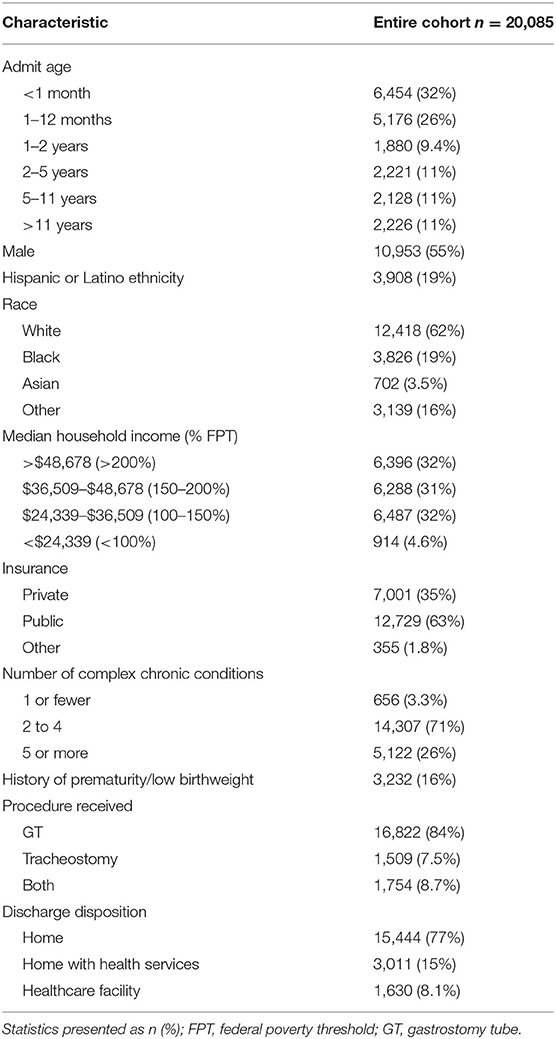
Table 1. Patient demographics and clinical characteristics at index admission in a cohort of technology-dependent children.
Only 6% (n = 1,264) of children required a PICU readmission within 30 days of discharge from the index hospitalization (Table 2). In an adjusted multivariate model, public insurance was associated with higher odds of 30-day PICU readmission [OR 1.28 (95% C.I. 1.12–1.47), p < 0.001], while living below the FPT was associated with a lower odds of 30-day PICU readmission [OR 0.7 (95% C.I. 0.51–0.95), p = 0.0267].
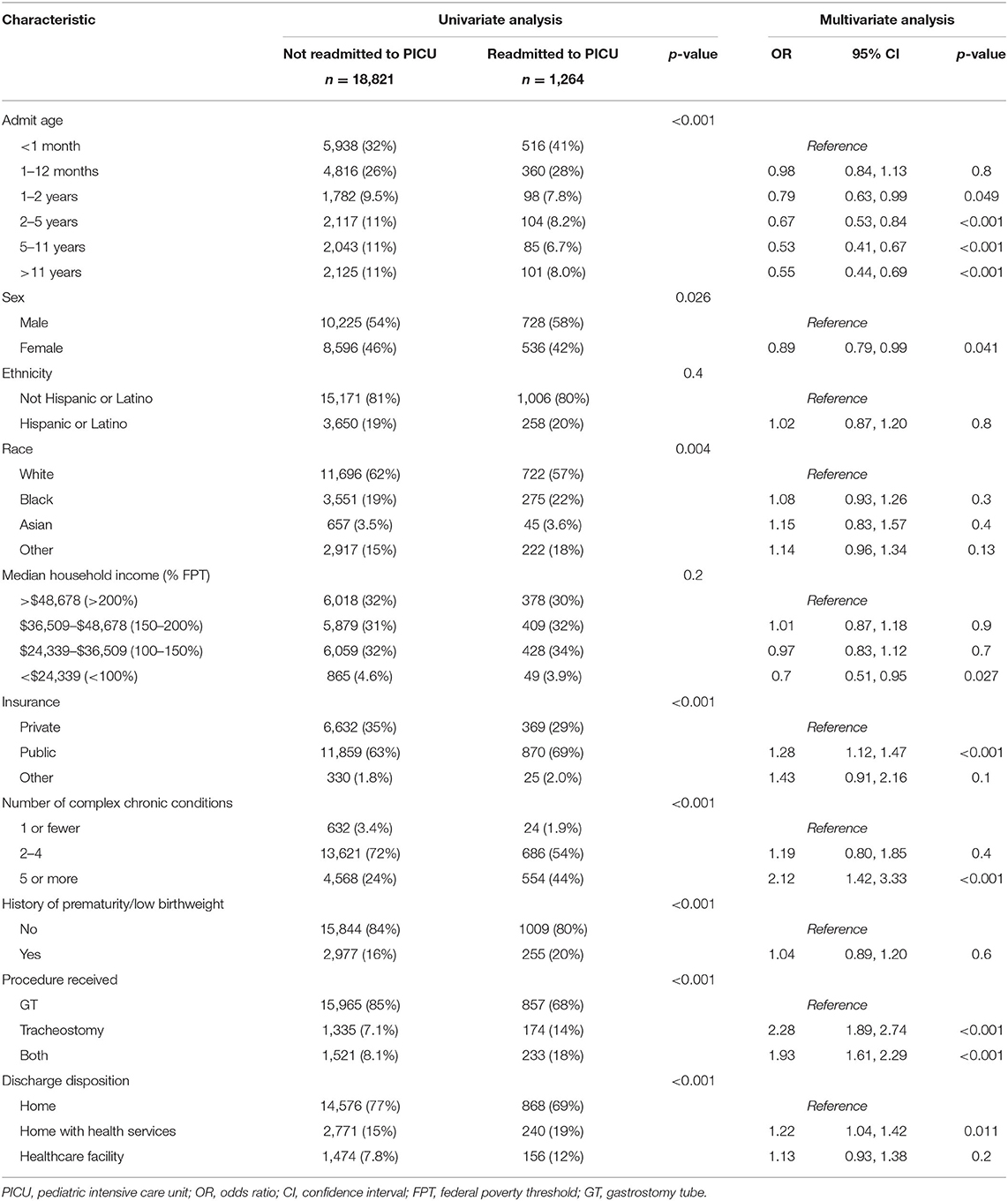
Table 2. Univariate and multivariate analysis of characteristics associated with a 30-day PICU readmission in a cohort of technology-dependent children.
Over 20% (n = 4,197) of children required multiple (>1) PICU admissions within one year from the index hospitalization (Table 3). In univariate analysis, there were differences in age, race, insurance, number of chronic complex conditions, history of prematurity or low birthweight, procedure received, and discharge disposition between children who did and did not require multiple PICU hospitalizations. In multivariate analysis, Black children [OR 1.20 (95% C.I. 1.10–1.32), p < 0.001] and those with public insurance [OR 1.34 (95% C.I. 1.24–1.46), p < 0.001] had higher odds of multiple PICU admissions. Estimated household income level was not an independent predictor of high PICU utilization in this cohort of children.
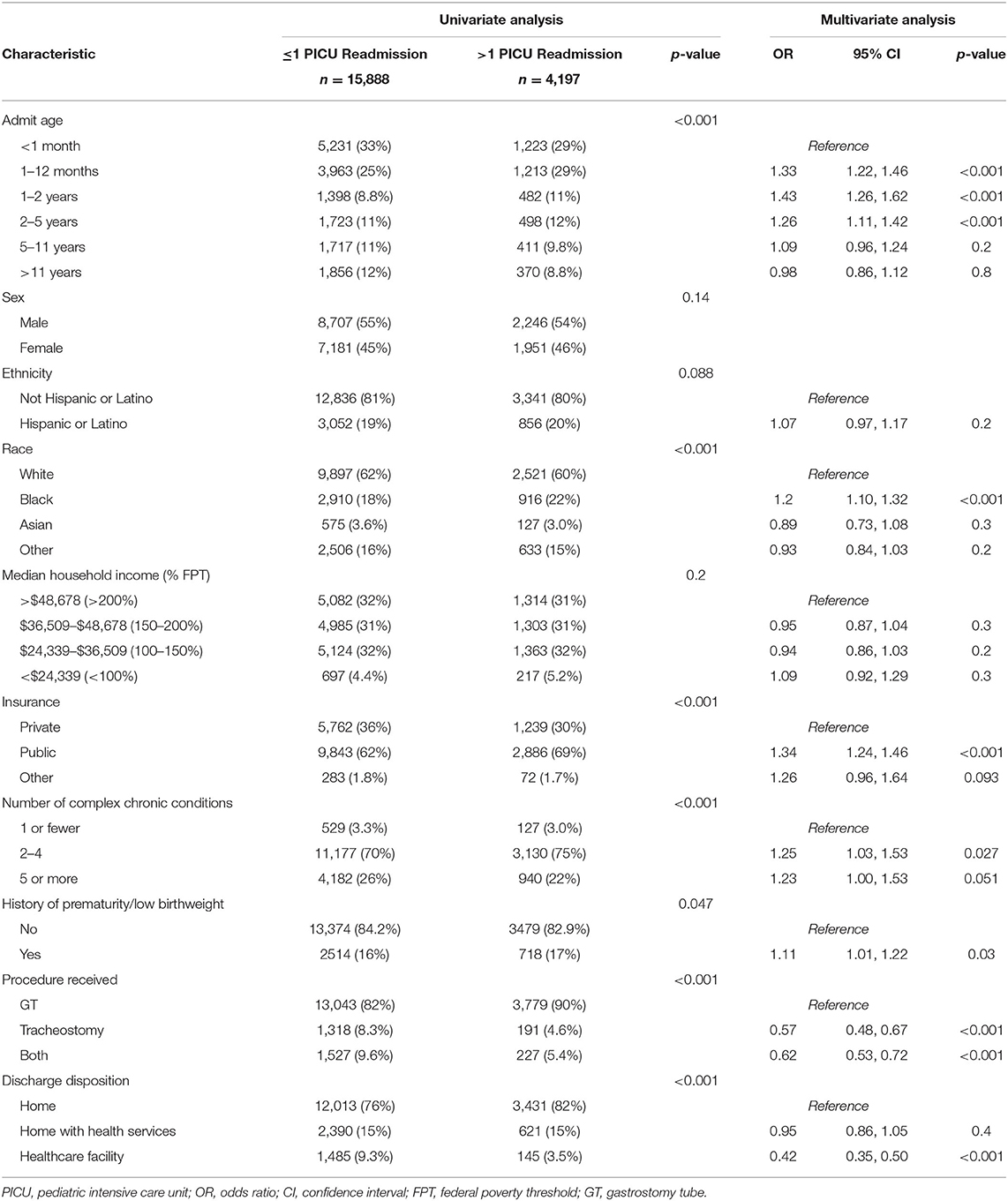
Table 3. Univariate and multivariate analysis of characteristics associated with >1 365-day PICU readmission in a cohort of technology-dependent children.
Race, ethnicity, household income and insurance were not associated with total hospital costs accrued in the 14,981 children who were admitted within one year of the index hospitalization following tracheostomy/GT placement (Table 4). In these children, multivariate models demonstrate that discharge disposition at index admission was the strongest predictor of discharge disposition upon subsequent hospitalizations; the impact of race/ethnicity, insurance status and estimated household income was negligible in comparison (Supplementary Table 4).
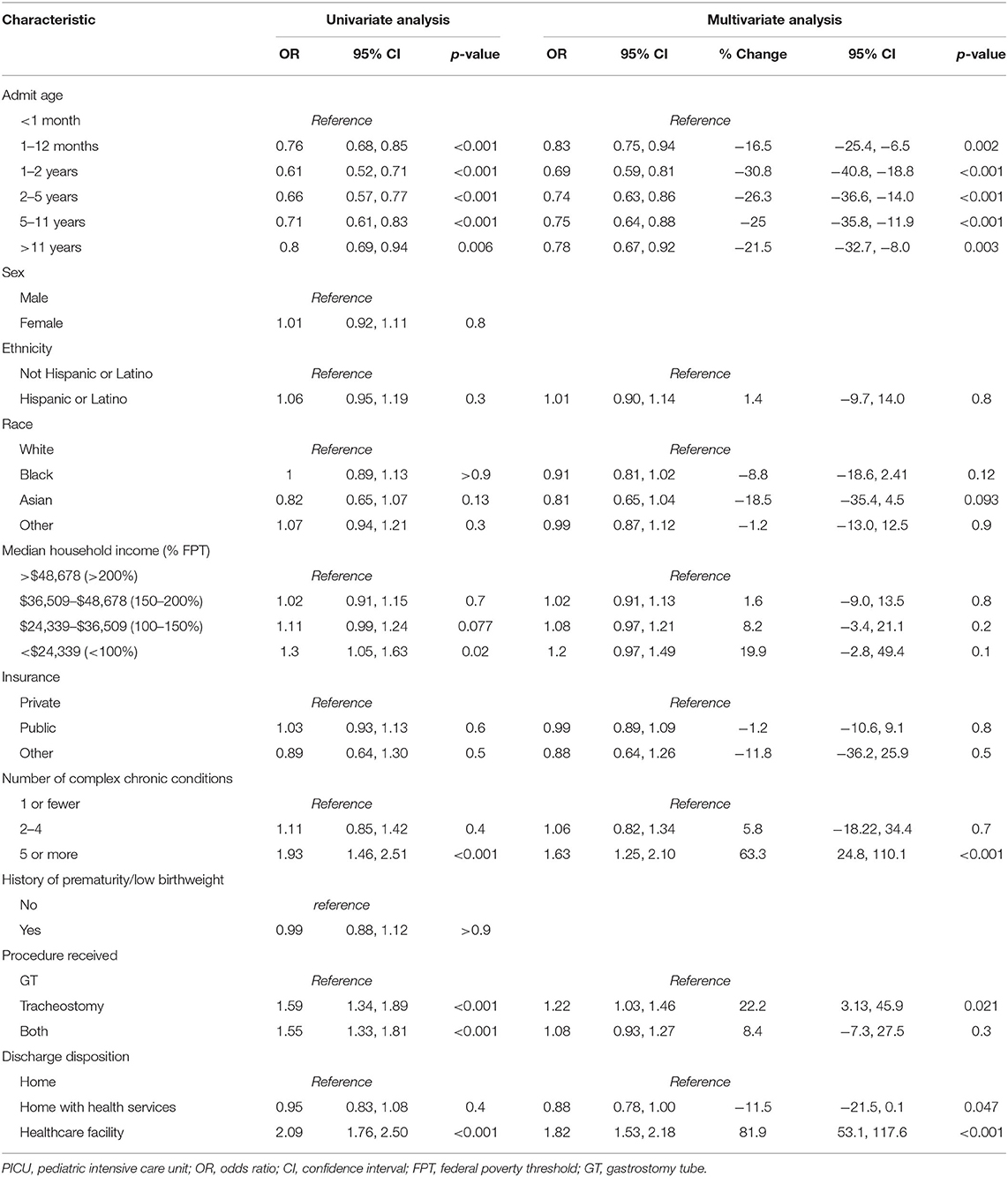
Table 4. Univariate and multivariate analysis of characteristics predictive of total hospital costs 1 year after tracheostomy/GT placement.
Children who were Black or of “other” race, had low household incomes, and public insurance all had higher odds of longer hospital LOS during their index admission. In multivariable regression analyses, Black children experienced a 27% longer LOS compared to White children [OR 1.27 (95% C.I. 1.21–1.33), p < 0.001], children living below the poverty threshold had a 22% longer LOS compared to children living at >200% FPT [OR 1.22 (95% C.I. 1.11–1.33), p < 0.001], and children with public insurance had an 11% longer LOS than children with private insurance [OR 1.11 (95% C.I. 1.06–1.15), p < 0.001] (Supplementary Table 5).
Within 30 days of the index hospitalization, 34% (n = 6,754) of children were readmitted to the hospital (Supplementary Table 6). In the multivariable model, Hispanic ethnicity [OR 1.10 (95% C.I. 1.02–1.19), p = 0.018], Black race [OR 1.23 (95% C.I. 1.14–1.34), p < 0.001), and public insurance [OR 1.31 (95% C.I. 1.22–1.40), p < 0.001] were associated with higher odds of 30-day hospital readmission. In this model, children with an estimated household income between 100 and 150% FPT had lower odds of 30-day hospital readmission [OR 0.88 (95% C.I. 0.81–0.95), p < 0.001).
Discussion
Technology-dependent children are at high risk for hospital admission and PICU utilization because of their medical fragility and complications inherent in their medical devices. The overall objective of this study was to understand if social risk factors for high PICU utilization existed in this patient population. To our knowledge, this is the first study to show differential PICU utilization based on insurance or race in a cohort of medically complex children.
There are several important observations made in this study. First, over two-thirds of children in this study were living in low-income households. While we did not show any clinically meaningful differences in PICU utilization based on estimated household income, the financial implications of supporting a medically complex child should nonetheless be considered when counseling families. Families consistently report financial and logistical complications in caring for medically complex children (17–20). In an observational study of 167 CMC with high rates of technology dependence, over half of families reported financial difficulties related to their child's health, and two-thirds reported that a family member had decreased work hours to care for their child (20). The burden on families is likely underestimated, as only 15% of children in our cohort were discharged home from the index hospital admission with skilled nursing care, a percentage that decreased to 8.7% following subsequent hospital discharges. In this study, we did not find socioeconomic factors to be strongly associated with discharge disposition, however. Prospective studies are needed to understand how family financial stress impacts the risk for acute illness in their technology-dependent child.
Second, compared to children of other races, Black children had unfavorable health outcomes following placement of a tracheostomy and/or GT. They had longer hospital LOS during the index hospitalization, 20% higher odds of 30-day hospital readmission, and 20% higher odds of multiple PICU admissions within one year of the index hospital stay. Differential quality of healthcare based on patient race is a well described pervasive flaw in our healthcare system (21). However, there are few studies investigating differences in the risk of pediatric critical illness or in PICU utilization based on race (22–24). There are numerous studies describing racial/ethnic disparities in neonatal intensive care. A recently published systematic review including 41 articles outlined pervasive differences in access to high quality healthcare systems, healthcare delivery, and neonatal patient outcomes, based on race (25). Studies show high risk Black infants are less likely to be referred to neonatal follow up clinics, and Black mothers report experiencing indifferent and ineffective communication from medical staff, impacting effective family-centered care (26–29). There is also adult literature to suggest that Black families are more likely to choose life-sustaining medical technology for their kin following cardiac arrest, trauma, and stroke, but suffer from more adverse events, worse patient experiences, and less improvement in functional outcomes when receiving home health care (30–35). Our study design precludes understanding why technology-dependent Black children have higher hospital and PICU utilization. But like the neonatal and adult populations, if hospital processes and healthcare systems are inadequate to educate, engage and assist Black families caring for medically complex children, and if the pervasive institutional racism isn't eliminated, then repeated admissions are likely (21).
Third, like Black race, public insurance was also associated with high PICU utilization including 30-day PICU readmission and multiple PICU admissions within a year of index hospitalization. Public insurance has repeatedly been associated with high hospital utilization in pediatric patients (24, 36, 37). Upon hospital discharge, parents of publicly insured children are more likely to make errors in discharge plans, perhaps related to the prevalence of low literacy in this population (38). In the community, many publicly insured children receive primary care in resource limited settings where high patient volumes may limit the face time with providers (39). Similarly, children with public insurance are less likely to have a medical home, and less likely to receive high-quality family-centered care, factors which will undoubtedly affect technology- dependent children (40, 41).
Fourth, it was unexpected that lower household income was not associated with higher PICU readmission rates. Multiple markers of low socioeconomic status, including household income, have consistently been associated with increased risk for repeated hospital admissions (42, 43). There are several reasons why income, race and insurance status may have had different influence on our study outcomes, which are likely reflective of the differential impact these variables have on population health, and study design limitations. “Social determinants of health” is an imprecise concept describing the interplay between inequalities in social position and inequalities in health. Defining social position is equally challenging and is conceptualized as a person's standing and power within social hierarchies, influenced by multiple factors including but not limited to, socioeconomic status, education, employment, gender, and race (44). Studies like this one reinforce that while race, income and insurance status are related, they are distinct measures that should be considered differently when designing interventions to mitigate their negative effect (45). Study design limitations also likely influenced our results. Hospitals within the PHIS database are primarily academic teaching centers located in large cities with higher rates of public insurance and lower median household income, compared to national data (46). Institution of medical technology is likely to occur at these tertiary hospitals, but low acuity readmissions to community hospitals for suburban and rural families will be missed by the PHIS database.
This study has additional limitations important to consider. First, we used an observational design in this study, so we are unable to establish a causal relationship between our exposure variables and PICU utilization. Second, it is a retrospective database study dependent upon high-quality database governance of participating tertiary academic children's hospitals. While generalizability of our findings may be limited, PHIS data is likely reflective of the care provided at over 200 children's hospitals and pediatric facilities within general hospitals that provide most critical care services for US children (12, 47, 48). Third, the identification of our study cohort with ICD codes could be inaccurate, however previous studies have suggested identification of tracheostomy and GT placement using this methodology has high sensitivity and specificity (49, 50). Fourth, the sociodemographic predictor variables may be inaccurate. Race and ethnicity may be misclassified in our patients, but prior work suggests that administrative data is adequate for identifying Hispanic and non-Hispanic ethnicity, and White and Black race (51). PHIS provides an estimated household income based on ZIP code, which is an imperfect, but likely accurate measure of socioeconomic status. Previous studies have identified ZIP codes as an adequate surrogate for neighborhood-based deprivation (52, 53). Fifth, readmission rates are likely underestimated, as children receiving care at hospitals not within the PHIS database are not included. Lastly, database studies do not provide any information on specific clinical reasons why children are readmitted, ability to identify clinical confounders is limited, and results can only be viewed as hypothesis generating.
Conclusion
This large multicenter cohort study of technology-dependent children demonstrated differences in PICU and hospital utilization based on race and insurance status. While future prospective studies are necessary to validate our findings, they suggest that socially vulnerable children and their families are over-burdened by hospitalizations following institution of medical technology. Aggressive interventions to prevent multiple, high-risk, resource-heavy hospital admissions and readmissions must be started by anchor healthcare institutions, including the tertiary children's hospitals represented in this study, even before we understand why these differences exist.
Data Availability Statement
The data analyzed in this study is subject to the following licenses/restrictions: Data for this article was obtained from the Pediatric Health Information Systems Database, which is available to members of the Children's Hospital Association (Lenexa, KS). Requests to access these datasets should be directed to childrenshospitals.org.
Author Contributions
KS conceptualized and designed the study. AB completed the data curation and statistical analysis. PP and JT made contributions to the study analysis and interpretation of the results. KS drafted the initial manuscript. AB, PP, and JT reviewed and edited the manuscript. All authors gave final approval to the final version of the manuscript and agree to be accountable for all aspects of the work.
Funding
Dr. Slain, Dr. Thornton, and the Center for Reducing Health Disparities are supported by NIH U54MD002265 and UL1TR002548.
Conflict of Interest
The authors declare that the research was conducted in the absence of any commercial or financial relationships that could be construed as a potential conflict of interest.
Publisher's Note
All claims expressed in this article are solely those of the authors and do not necessarily represent those of their affiliated organizations, or those of the publisher, the editors and the reviewers. Any product that may be evaluated in this article, or claim that may be made by its manufacturer, is not guaranteed or endorsed by the publisher.
Supplementary Material
The Supplementary Material for this article can be found online at: https://www.frontiersin.org/articles/10.3389/fped.2021.721353/full#supplementary-material
Abbreviations
CMC, children with medical complexity; US, United States; PICU, pediatric intensive care unit; GT, gastrostomy tube; PHIS, Pediatric Health Information System; ICD-10-CM and ICD-10-PCS, International Classification of Diseases, Tenth Revision, Clinical Modification and Procedure Classification System; LOS, length of stay, FPT, federal poverty threshold; CCC, chronic complex condition; IQR, interquartile range; OR, odds ratio; CI, confidence interval.
References
1. Kuo DZ, Houtrow AJ. Council on children with disabilities. Recognit Manage Med Complexity Pediatr. (2016) 138:6. doi: 10.1542/peds.2016-3021
2. Cohen E, Berry JG, Camacho X, Anderson G, Wodchis W, Guttmann A. Patterns and costs of health care use of children with medical complexity. Pediatrics. (2012) 130:e1463–70. doi: 10.1542/peds.2012-0175
3. Spratling R. Defining technology dependence in children and adolescents. West J Nurs Res. (2015) 37:634–51. doi: 10.1177/0193945914526002
4. Heneghan JA, Reeder RW, Dean JM, Meert KL, Berg RA, Carcillo J, et al. Characteristics and outcomes of critical illness in children with feeding and respiratory technology dependence. Pediatr Crit Care Med. (2019) 20:417–25. doi: 10.1097/PCC.0000000000001868
5. Berry JG, Graham DA, Graham RJ, Zhou J, Putney HL, O'Brien JE, et al. Predictors of clinical outcomes and hospital resource use of children after tracheotomy. Pediatrics. (2009) 124:563–72. doi: 10.1542/peds.2008-3491
6. Friesen TL, Zamora SM, Rahmanian R, Bundogji N, Brigger MT. Predictors of pediatric tracheostomy outcomes in the United States. Otolaryngol Head Neck Surg. (2020) 163:591–9. doi: 10.1177/0194599820917620
7. Liao K, Chorney SR, Brown AB, Brooks RL, Sewell A, Bailey C, et al. The impact of socioeconomic disadvantage on pediatric tracheostomy outcomes. Laryngoscope. (2021). doi: 10.1002/lary.29576. [Epub ahead of print].
8. Goldin AB, Heiss KF, Hall M, Rothstein DH, Minneci PC, Blakely ML, et al. Emergency department visits and readmissions among children after gastrostomy tube placement. J Pediatr. (2016) 174:139–145.e132. doi: 10.1016/j.jpeds.2016.03.032
9. Cristea AI, Ackerman VL, Davis SD, Carroll AE, Downs SM, Yu Z, et al. Median household income: association with mortality in children on chronic ventilation at home secondary to bronchopulmonary dysplasia. Pediatr Allergy Immunol Pulmonol. (2015) 28:41–6. doi: 10.1089/ped.2014.0406
10. Sobotka SA, Lynch E, Quinn MT, Awadalla SS, Agrawal RK, Peek ME. Unmet respite needs of children with medical technology dependence. Clin Pediatr. (2019) 58:1175–86. doi: 10.1177/0009922819870251
11. Council on Community Pediatrics. Poverty and child health in the United States. Pediatrics. (2016) 137:e20160339. doi: 10.1542/peds.2016-0339
12. Benneyworth BD, Bennett WE, Carroll AE. Cross-sectional comparison of critically ill pediatric patients across hospitals with various levels of pediatric care. BMC Res Notes. (2015) 8:693. doi: 10.1186/s13104-015-1550-9
13. United States Census Bureau. American Community Survey: 2014 ACS 1-Year Estimates. Suitland, MD: United States Census Bureau (2014).
14. US Department of Health and Human Services ASPE. Frequently Asked Questions Related to the Poverty Guidelines and Poverty. Available online at: https://aspe.hhs.gov/frequently-asked-questions-related-poverty-guidelines-and-poverty#differences (accessed May 23, 2021).
15. Williams K, Thomson D, Seto I, Contopoulos-Ioannidis DG, Ioannidis JP, Curtis S, et al. Standard 6: age groups for pediatric trials. Pediatrics. (2012) 129(Suppl. 3):S153–160. doi: 10.1542/peds.2012-0055I
16. Feudtner C, Feinstein JA, Zhong W, Hall M, Dai D. Pediatric complex chronic conditions classification system version 2: updated for ICD-10 and complex medical technology dependence and transplantation. BMC Pediatr. (2014) 14:199. doi: 10.1186/1471-2431-14-199
17. Page BF, Hinton L, Harrop E, Vincent C. The challenges of caring for children who require complex medical care at home: ‘the go between for everyone is the parent and as the parent that's an awful lot of responsibility'. Health Expect. (2020) 23:1144–54. doi: 10.1111/hex.13092
18. Edwards JD, Panitch HB, Constantinescu A, Miller RL, Stone PW. Survey of financial burden of families in the US with children using home mechanical ventilation. Pediatr Pulmonol. (2018) 53:108–16. doi: 10.1002/ppul.23917
19. Parish SL, Shattuck PT, Rose RA. Financial burden of raising CSHCN: association with state policy choices. Pediatrics. (2009) 124(Suppl. 4):S435–42. doi: 10.1542/peds.2009-1255P
20. Thomson J, Shah SS, Simmons JM, Sauers-Ford HS, Brunswick S, Hall D, et al. Financial and social hardships in families of children with medical complexity. J Pediatr. (2016) 172:187–93.e181. doi: 10.1016/j.jpeds.2016.01.049
21. Institute of Medicine (US) Committee on Understanding and Eliminating Racial and Ethnic Disparities in Health Care. In: Smedley BD, Stith AY, Nelson AR, editors. Unequal Treatment: Confronting Racial and Ethnic Disparities in Health Care. Washington, DC: The National Academies Press (2003).
22. Turner D, Simpson P, Li SH, Scanlon M, Quasney MW. Racial disparities in pediatric intensive care unit admissions. South Med J. (2011) 104:640–6. doi: 10.1097/SMJ.0b013e3182296e52
23. Slain KN, Wurtz MA, Rose JA. US children of minority race are less likely to be admitted to the pediatric intensive care unit after traumatic injury, a retrospective analysis of a single pediatric trauma center. Inj Epidemiol. (2021) 8:14. doi: 10.1186/s40621-021-00309-x
24. Kane JM, Hall M, Cecil C, Montgomery VL, Rakes LC, Rogerson C, et al. Resources and costs associated with repeated admissions to PICUs. Crit Care Explor. (2021) 3:e0347. doi: 10.1097/CCE.0000000000000347
25. Sigurdson K, Mitchell B, Liu J, Morton C, Gould JB, Lee HC, et al. Racial/ethnic disparities in neonatal intensive care: a systematic review. Pediatrics. (2019) 144:e20183114. doi: 10.1542/peds.2018-3114
26. Barfield WD, Clements KM, Lee KG, Kotelchuck M, Wilber N, Wise PH. Using linked data to assess patterns of early intervention (EI) referral among very low birth weight infants. Matern Child Health J. (2008) 12:24–33. doi: 10.1007/s10995-007-0227-y
27. Hintz SR, Gould JB, Bennett MV, Gray EE, Kagawa KJ, Schulman J, et al. Referral of very low birth weight infants to high-risk follow-up at neonatal intensive care unit discharge varies widely across California. J Pediatr. (2015) 166:289–95. doi: 10.1016/j.jpeds.2014.10.038
28. Martin AE, D'Agostino JA, Passarella M, Lorch SA. Racial differences in parental satisfaction with neonatal intensive care unit nursing care. J Perinatol. (2016) 36:1001–7. doi: 10.1038/jp.2016.142
29. Sigurdson K, Morton C, Mitchell B, Profit J. Disparities in NICU quality of care: a qualitative study of family and clinician accounts. J Perinatol. (2018) 38:600–7. doi: 10.1038/s41372-018-0057-3
30. Woo KK, Can A, Chang DW. Racial differences in the utilization of guideline-recommended and life-sustaining procedures during hospitalizations for out-of-hospital cardiac arrest. J Racial Ethn Health Dispar. (2020) 7:403–12. doi: 10.1007/s40615-019-00668-8
31. Faigle R, Urrutia VC, Cooper LA, Gottesman RF. Racial differences in utilization of life-sustaining vs curative inpatient procedures after stroke. JAMA Neurol. (2016) 73:1151–3. doi: 10.1001/jamaneurol.2016.1914
32. Hanna K, Palmer J, Castanon L, Zeeshan M, Hamidi M, Kulvatunyou N, et al. Racial and ethnic differences in limiting life-sustaining treatment in trauma patients. Am J Hosp Palliat Care. (2019) 36:974–9. doi: 10.1177/1049909119847970
33. Narayan MC, Scafide KN. Systematic review of racial/ethnic outcome disparities in home health care. J Transcult Nurs. (2017) 28:598–607. doi: 10.1177/1043659617700710
34. Jones RC, Creutzfeldt CJ, Cox CE, Haines KL, Hough CL, Vavilala MS, et al. Racial and ethnic differences in health care utilization following severe acute brain injury in the United States. J Intensive Care Med. (2020) 10:885066620945911. doi: 10.1177/0885066620945911
35. Krishnamoorthy V, Hough CL, Vavilala MS, Komisarow J, Chaikittisilpa N, Lele AV, et al. Tracheostomy after severe acute brain injury: trends and variability in the USA. Neurocrit Care. (2019) 30:546–54. doi: 10.1007/s12028-019-00697-5
36. Edwards JD, Lucas AR, Boscardin WJ, Dudley RA. Repeated critical illness and unplanned readmissions within 1 year to PICUs. Crit Care Med. (2017) 45:1276–84. doi: 10.1097/CCM.0000000000002439
37. Berry JG, Toomey SL, Zaslavsky AM, Jha AK, Nakamura MM, Klein DJ, et al. Pediatric readmission prevalence and variability across hospitals. JAMA. (2013) 309:372–80. doi: 10.1001/jama.2012.188351
38. Glick AF, Farkas JS, Nicholson J, Dreyer BP, Fears M, Bandera C, et al. Parental management of discharge instructions: a systematic review. Pediatrics. (2017) 140:e20164165. doi: 10.1542/peds.2016-4165
39. Cunningham P, May J. Medicaid patients increasingly concentrated among physicians. Track Rep. (2006) 1–5.
40. Zickafoose JS, Gebremariam A, Clark SJ, Davis MM. Medical home disparities between children with public and private insurance. Acad Pediatr. (2011) 11:305–10. doi: 10.1016/j.acap.2011.03.006
41. Bleser WK, Young SI, Miranda PY. Disparities in patient- and family-centered care during US children's health care encounters: a closer examination. Acad Pediatr. (2017) 17:17–26. doi: 10.1016/j.acap.2016.06.008
42. Bensken WP, Alberti PM, Koroukian SM. Health-related social needs and increased readmission rates: findings from the nationwide readmissions database. J Gen Intern Med. (2021) 36:1173–80. doi: 10.1007/s11606-021-06646-3
43. Auger KA, Teufel RJ II, Harris JM II, Gay JC, Del Beccaro MA, Neuman MI, et al. Children's hospital characteristics and readmission metrics. Pediatrics. (2017) 139:e20161720. doi: 10.1542/peds.2016-1720
44. Graham H. Social determinants and their unequal distribution: clarifying policy understandings. Milbank Q. (2004) 82:101–24. doi: 10.1111/j.0887-378X.2004.00303.x
45. Nuru-Jeter AM, Michaels EK, Thomas MD, Reeves AN, Thorpe RJ Jr, LaVeist TA. Relative roles of race versus socioeconomic position in studies of health inequalities: a matter of interpretation. Annu Rev Public Health. (2018) 39:169–188. doi: 10.1146/annurev-publhealth-040617-014230
46. Sills MR, Hall M, Colvin JD, Macy ML, Cutler GJ, Bettenhausen JL, et al. Association of social determinants with children's hospitals' preventable readmissions performance. JAMA Pediatr. (2016) 170:350–358. doi: 10.1001/jamapediatrics.2015.4440
47. Leyenaar JK, Ralston SL, Shieh MS, Pekow PS, Mangione-Smith R, Lindenauer PK. Epidemiology of pediatric hospitalizations at general hospitals and freestanding children's hospitals in the United States. J Hosp Med. (2016) 11:743–9. doi: 10.1002/jhm.2624
48. Simon TD, Berry J, Feudtner C, Stone BL, Sheng X, Bratton SL, et al. Children with complex chronic conditions in inpatient hospital settings in the United States. Pediatrics. (2010) 126:647–55. doi: 10.1542/peds.2009-3266
49. Lahiri S, Mayer SA, Fink ME, Lord AS, Rosengart A, Mangat HS, et al. Mechanical ventilation for acute stroke: a multi-state population-based study. Neurocrit Care. (2015) 23:28–32. doi: 10.1007/s12028-014-0082-9
50. Bennett TD, DeWitt PE, Dixon RR, Kartchner C, Sierra Y, Ladell D, et al. Development and prospective validation of tools to accurately identify neurosurgical and critical care events in children with traumatic brain injury. Pediatr Crit Care Med. (2017) 18:442–51. doi: 10.1097/PCC.0000000000001120
51. Smith N, Iyer RL, Langer-Gould A, Getahun DT, Strickland D, Jacobsen SJ, et al. Health plan administrative records versus birth certificate records: quality of race and ethnicity information in children. BMC Health Serv Res. (2010) 10:316. doi: 10.1186/1472-6963-10-316
52. Krieger N, Williams DR, Moss NE. Measuring social class in US public health research: concepts, methodologies, and guidelines. Annu Rev Public Health. (1997) 18:341–78. doi: 10.1146/annurev.publhealth.18.1.341
Keywords: healthcare disparities, intensive care units, pediatric, tracheostomy, gastrostomy, race
Citation: Slain KN, Barda A, Pronovost PJ and Thornton JD (2021) Social Factors Predictive of Intensive Care Utilization in Technology-Dependent Children, a Retrospective Multicenter Cohort Study. Front. Pediatr. 9:721353. doi: 10.3389/fped.2021.721353
Received: 06 June 2021; Accepted: 18 August 2021;
Published: 13 September 2021.
Edited by:
Michael C. Spaeder, University of Virginia, United StatesReviewed by:
Jason Espinoza, Riley Hospital for Children, United StatesA. Ioana Cristea, Riley Hospital for Children, United States
Copyright © 2021 Slain, Barda, Pronovost and Thornton. This is an open-access article distributed under the terms of the Creative Commons Attribution License (CC BY). The use, distribution or reproduction in other forums is permitted, provided the original author(s) and the copyright owner(s) are credited and that the original publication in this journal is cited, in accordance with accepted academic practice. No use, distribution or reproduction is permitted which does not comply with these terms.
*Correspondence: Katherine N. Slain, katherine.slain@uhhospitals.org