- 1Division of Nephrology, Department of Internal Medicine, University of Michigan, Ann Arbor, MI, United States
- 2University of Michigan Medical School, Ann Arbor, MI, United States
Nephrotic syndrome is classically categorized by the histopathology with examples including focal segmental glomerulosclerosis (FSGS) and minimal change disease. Pediatric patients are also classified by whether their nephrotic syndrome is sensitive to, dependent on, or resistant to steroids. However, this traditional classification system overlooks the frequent clinical conundrum when, for example, one patient with FSGS responds briskly to steroids, and another quickly progresses to end stage kidney disease despite therapy. Two patients may have similar histopathologic appearances on kidney biopsy but entirely different clinical characteristics, rates of progression, and treatment responses. Transcriptional regulation of gene activation and posttranscriptional processing of mRNA may drive the unique and heterogeneous phenotypes which are incompletely understood in kidney disease and are a recent focus of research. Gene expression profiles provide insight on active transcriptional programs in tissues, are being used to understand biologic mechanisms of progressive chronic kidney disease, and may help to identify patients with shared mechanisms of kidney damage. This mini-review discusses clinically relevant techniques of bulk tissue and single cell transcriptomics, as well as strengths and limitations of each methodology. Further, we summarize recent examples in kidney research achieved through transcriptomics. This review offers an outlook on the role of transcriptomics in an integrative systems biology model with the goal of defining unique disease subgroups, finding targets for drug development, and aligning the right drug with the right patient.
Introduction
Nephrotic syndromes are kidney diseases which result in proteinuria, hypoalbuminemia, edema, and hyperlipidemia. Traditionally nephrotic syndrome is categorized into diagnoses by their histopathology (minimal change disease, focal segmental glomerulosclerosis (FSGS), membranous nephropathy) or their response to steroids, however pathology and clinical phenotype do not predict or uniquely correlate with rate of progression or response to therapy. The transcriptome refers to all of the RNA expressed from genes of a particular organism, tissue, or cell type. Understanding the transcriptome can help elucidate the crucial link between genotype and phenotype, as gene expression and control of gene expression can vary under different states of health and disease. In addition, progressive kidney diseases may upregulate particular common transcriptional pathways—allowing scientists to identify prognostic biomarkers and develop interventions targeted at these shared pathways in chronic kidney disease (CKD). By defining kidney diseases more granularly than their histopathology and clinical presentation and incorporating data from transcriptomics as well as genomics, proteomics, and metabolomics—scientists work toward advancing the field of nephrology toward targeted treatments for kidney disease.
In this mini-review, we briefly review techniques used to analyze the transcriptome, specifically comparing and contrasting techniques that use “bulk” methods profiling the mRNA expression from whole tissue (in our case kidney biopsy tissue) vs. “single cell” methods profiling the mRNA expression within individual cells extracted from tissue or biofluids, with the goal of describing how these techniques are being used to improve our understanding and treatment of kidney disease.
Bulk Transcriptomic Profiling
Brief Methods and Techniques
DNA and RNA microarray technology emerged in the mid-1990s (1, 2), facilitating large scale analyses of gene expression data. Microarray formation begins with a pre-specified set of nucleic acid probes which are designed to recognize a specific RNA sequence and are bound to glass slides. Target sequences from the patient samples are hybridized to the probes and are subsequently fluorescently labeled. Images are then analyzed and quantified for signal intensity—with increasing intensity proportional to the greater abundance of the transcripts and hybridization to the probes. These hybridization techniques are relatively inexpensive but the probes rely on an a priori understanding of the model organism's genome and transcriptome and must be regularly updated with known sequence data. When using microarray technology, whole tissues can be analyzed en masse or can be divided into compartments. In human kidney research, the glomerular compartment and the tubulointerstitial compartment are commonly separated through microdissection, and are then analyzed separately (3, 4).
Massive parallel sequencing, also called Next Generation Sequencing or deep sequencing, was subsequently developed in the early 2000s (5). This technique is now frequently used to study the transcriptome and referred to as RNA-sequencing (RNA-Seq) technology. RNA-Seq continues high output transcriptional analyses, and unlike microarray technology, it is unencumbered by the need for a prior understanding of the genomic sequence, but rather allows sequencing of all RNA in the sample. This allows for greater discovery of the transcriptome across the full genome, including identification of splice isoforms by directly sequencing the transcripts. An additional benefit to RNA-Seq technology is the greater detection range compared to microarrays: it can detect low levels of expression or very high levels with greater precision, unlike the microarray probes which become saturated. Different platforms can be used for sequencing, all of which sequence millions of short genome fragments in parallel. Ultimately, transcript reads are aligned back to a known genome in order to determine their differential expression (i.e., up- or down-regulation of transcripts) in tissues, or they can be used for transcript discovery and mapping when a reference genome does not exist (6).
Examples of Bulk Transcriptomics Analysis in Clinical Disease
A Novel Non-invasive Biomarker for Disease Progression: Urinary EGF
CKD is estimated to affect 11–13% of the overall population worldwide (7). While pediatric patients are a small proportion of the CKD and end stage kidney disease (ESKD) population, they suffer from a higher burden of hospitalizations, cardiovascular disease (8) and death (9) when compared to healthy children. Kidney Disease: Improving Global Outcomes (KDIGO) guidelines categorize stages of kidney function by a calculation of estimated glomerular filtration rate (eGFR) and an assessment of microalbuminuria/proteinuria (10). Estimated GFR is derived from serum creatinine, and in pediatrics accounts for height (11). The categories delineated by KDIGO, CKD 1–5, are associated with specific risks for progression to ESKD, and patients with CKD 1 and 2 have the lowest risk for progression. However, serum creatinine is notoriously a late marker for loss of renal function (12), and traditional formulas to estimate GFR perform poorly at the earlier stages of CKD (13). Thus, the challenge is to find patients who are at high risk to progress in early stages of their disease, so that interventions can be most efficacious. While histopathologic features on kidney biopsy can help predict outcomes independent of serum creatinine and estimated GFR (14, 15), the invasive and high risk nature of a kidney biopsy and high prevalence of CKD make this an untenable strategy for early detection. Developing early, non-invasive biomarkers is imperative to early detection and treatment.
Using microarray technology on kidney biopsy tissue, Ju et al. identified urinary epidermal growth factor (EGF) as an independent predictor of CKD progression (16). EGF is largely expressed in the thick ascending limb of Henle and distal tubule cells of the kidney (17), has pro-proliferative and anti-apoptotic functions, and enhances regeneration of renal tubule cells and recovery from injury (18). Ju's study used kidney biopsy tissue from a European biobank (European Renal cDNA Bank: ERCB) to identify intra-renal transcripts associated with eGFR at the time of biopsy. This work found the EGF transcript to be amongst those with greatest predictive performance for patient eGFR at time of biopsy. EGF expression was also shown to be highly specific to kidney tissue when compared to non-kidney tissue expression data and has known biology relevant to CKD progression. Intra-renal mRNA expression and correlation with eGFR was validated in additional ERCB patients as well as in a North American cohort (Clinical Phenotyping Resource and Biobank Core). Following this validation in kidney tissue, urinary EGF protein was measured and normalized to urinary creatinine, similar to the urine protein to creatinine ratio used in clinical practice today. The uEGF/Cr ratio was interrogated as a potential non-invasive marker for tissue-based RNA expression in three independent cohorts. uEGF/Cr was found to significantly correlate with intrarenal EGF transcript expression and eGFR at time of biopsy. uEGF/Cr importantly was also associated with eGFR slope and added predictive power to standard models to predict progression to ESKD. This relationship has been validated in several other studies. Betz et al subsequently showed that a lower uEGF/Cr was associated with rapid decline in renal function in a cohort of patients with diabetes with normoalbuminuria and preserved eGFR (19). In a cohort of 117 children with confirmed Alport syndrome, Li et al demonstrated that children with Alport syndrome showed significantly lower uEGF/Cr when compared to 146 age-matched healthy children (20). Again in this population, uEGF/Cr correlated tightly with eGFR (r = 0.75, p < 0.001), eGFR slope, and “progressors”—those who advanced to a higher CKD stage within the study follow up—were more likely to have low uEGF/Cr. Most recently, in a cohort of 623 children, low uEGF/Cr was associated with increased risk of CKD progression (21).
In summary, low uEGF/Cr level is a robust predictor of eGFR in cross-section, and it has also been shown to be a promising early biomarker to predict progression of kidney disease across several cohorts and disease types, including children. In many kidney diseases, EGF may be a general marker for tubular health, not captured by serum creatinine and urine protein. In this example, transcriptomic data was used to identify a novel biologic process and then applied to develop a non-invasive marker which reliably captures intra-renal transcriptional activity, and predicts kidney disease progression.
A Novel Mechanism and Treatment Target: JAK-STAT in Diabetic Nephropathy
Transcriptomics has also found a role in elucidating mechanisms of disease which escape traditional animal models. While mouse models of diabetic nephropathy mimic early stage disease in humans, they fail to replicate the progressive histopathological, and biochemical changes that are classic for advanced diabetic nephopathy (22). Using microarray technology, Berthier et al. compared transcriptional patterns from kidney tissue of early diabetic disease, progressive kidney disease, and controls (kidney donor tissue and patients with minimal change disease), finding that Janus kinases-signal transducer and activator of transcription (JAK-STAT) signaling pathways are differentially expressed in different kidney tissue compartments (glomerular vs. tubulointerstitial), and at different stages of diabetic kidney disease (early vs. late and progressive) (23). In particular, Jak-2, a protein that is part of the JAK-STAT signaling pathway, expression was upregulated in the glomerular compartment of humans with early diabetic nephropathy, and in late diabetic nephropathy Jak-2 expression is downregulated in the glomerular department and upregulated in the tubulointerstitial compartment. Tubulointerstitial Jak-2 expression was strongly, inversely correlated with eGFR of patients with diabetic nephropathy. However, Jak-2 mRNA and protein expression was not enhanced in two mouse models of diabetes when compared to control mice, suggesting that differences in JAK-STAT pathway signaling between humans and mice may be one reason why mouse models fail to replicate advanced diabetic nephropathy (23). JAK inhibitors have been developed for other diseases, and this work, along with that of others (24, 25), laid the foundation for trials of JAK inhibition for diabetic nephropathy. A recent phase II trial of baricitinib, an oral inhibitor of JAK1 and JAK2, was found to decrease albuminuria at 24 weeks when compared to placebo in patients with diabetic kidney disease (26). Applying the knowledge gained from studies in diabetic nephropathy, and specifically relevant to pediatrics and nephrotic syndrome, components of the JAK-STAT signaling pathway have also been shown to be upregulated in humans with biopsy-proven FSGS, and that the changes in JAK-STAT are associated with key clinical features among patients with FSGS, suggesting a role in pathogenesis as well as a potential target for treatment (27).
In this example, transcriptomic data from kidney biopsy was used to identify a targetable disease pathway in diabetic nephropathy that led to a successful early stage clinical trial. Insights gained from this foundational work have been applied to other kidney diseases such as FSGS, as well as models of polycystic kidney disease (28) and unilateral ureteral obstruction models of renal fibrosis (29), potentially providing a treatment target in a variety of kidney diseases.
Strengths and Limitations of Bulk Tissue Transcriptomics
Bulk tissue transcriptomics has been used to identify biomarkers, novel mechanisms of disease, and potential targets for treatment. However, using tissue in bulk, such as tissue from a renal biopsy, to evaluate gene expression poses challenges because of the admixture of many cell types. While bulk methods advance our understanding of global transcriptional changes in the whole tissue, elucidating transcriptional changes in particular cell types remains a challenge. Bioinformatic methods have been developed to infer cell composition from bulk expression data based on unique cell markers, but characterizing single cell expression relies on inference based on marker gene expression levels, rather than direct measurement (30). Methods of amplifying transcripts from specific cell types are subject to biases from the amplification technique. Single cell transcriptomic techniques, discussed in detail in the second half of this review, are a recent advancement designed to address this issue.
Single Cell Transcriptomic Profiling
While bulk sequencing is useful for identifying gene expression patterns across a whole tissue or tissue compartment, single cell sequencing techniques allow researchers to identify gene expression patterns of a single cell type within a whole tissue.
Brief Methods and Techniques
Microdissection and sorting by flow cytometry to process bulk tissue into single cells for RNA analysis are limited by the inability to reliably purify a single cell type and interrogate a single cell's transcriptome. In other words, the resulting transcriptomic data is an average of RNA transcripts from a mixed group of cells (31). Newer sequencing methods rely on dissociating the tissue and encapsulating single cells in individual spaces (aka “droplets”), where each space contains everything necessary to label and then subsequently sequence the individual RNA transcripts of that single cell (32). These are referred to as “droplet-based” techniques. Droplet-based sequencing technology allows researchers to utilize that expression data from individual cells to cluster related cell types from a heterogeneous tissue, like the kidney. In this way, researchers can identify rare cell populations, discover new cell types, and track dynamic cellular processes (31, 33). Researchers can follow major biological mechanisms to identify cell-specific gene expression that may mediate tissue development, differentiation, and disease progression, which is more challenging with bulk sequencing techniques (31, 34).
Single cell RNA sequencing involves five basic steps: single-cell isolation, cell lysis, reverse transcription of mRNA, amplification, and library generation (31, 35–37). In this review, we will specifically focus on the single cell droplet sequencing method named DropSeq. Figure 1A displays the steps of this technique. There are several mechanical and enzymatic methods used to dissociate live, single cells from tissue (31, 37). After single cell dissociation, a microfluidic device uses laminar flow to combine the suspension of single cells with a suspension of oligonucleotides (short sequences which can bind the mRNAs from that cell) attached to microparticles (beads) into single nanoliter droplets. Each bead has a unique identifier sequence to identify a single cell, and each oligonucleotide within a bead has a Unique Molecular Identifier (UMI) that identifies the single mRNA after amplification. After the droplets with one bead and one cell are created, the cells are lysed to release mRNA that is immediately captured by oligonucleotide primers. The new hybridized sequences are called Single Cell Transcriptomes attached to Microparticles (STAMPs). STAMPs are reverse transcribed, amplified, and sequenced in one reaction. Data analysis allows each mRNA transcript to be assigned by their cell barcode to a single cell type.
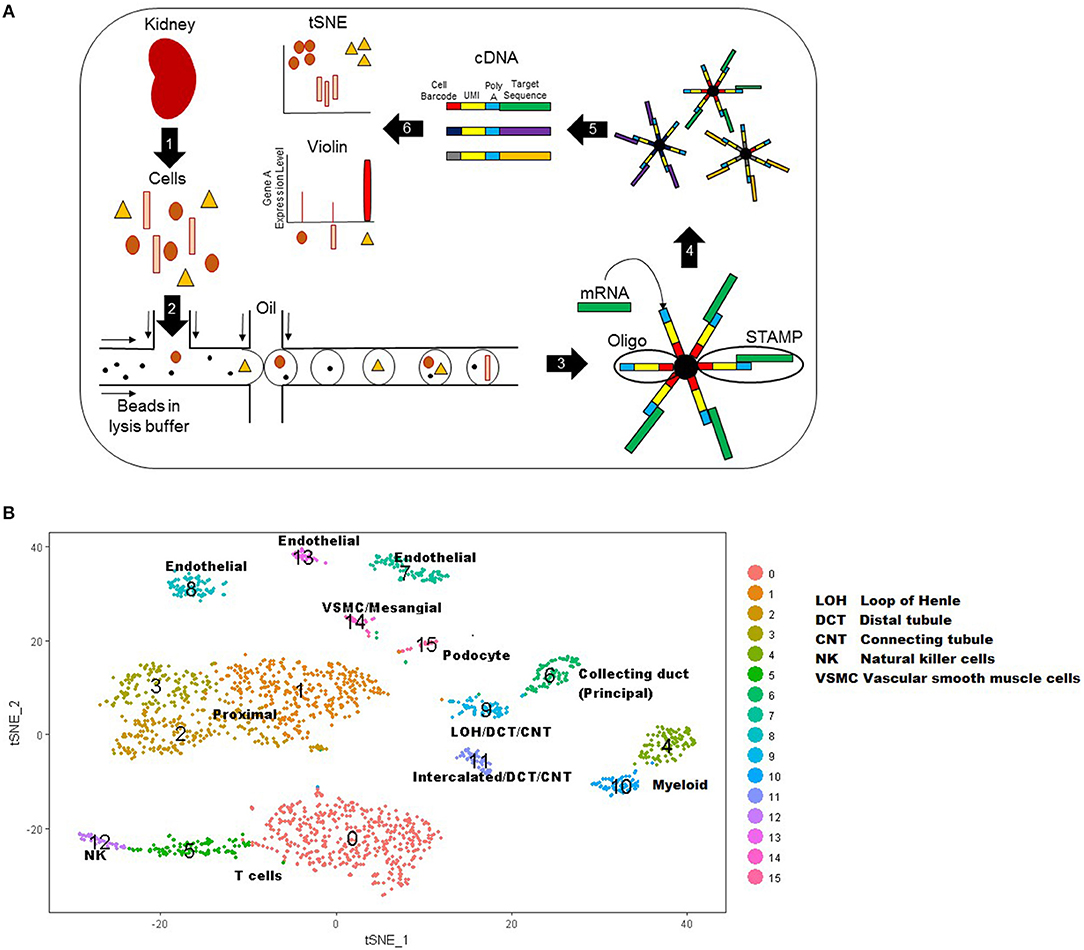
Figure 1. (A) Schematic of DropSeq workflow stepwise. Step 1: kidney biopsy tissue is digested into live single cells. Step 2 cells: cells, lysis buffer, and beads are injected into the microfluidic device where oil flowing across the stream pinches off droplets. Step 3: the lysed cell releases RNA for capture by bead primers. Step 4: droplets are broken to release beads with their cell-specific RNAs. Step 5: mRNA is reverse transcribed and cDNA is amplified for sequencing. Step 6: Data that has been mapped to the genome is analyzed to create a tSNE or Violin plot. UMI: Unique molecular identifier. STAMPs: Single Cell Transcriptomes attached to Microparticles. (B) T-Distributed Stochastic Neighbor Embedding (tSNE) plot representation of cell types in kidney tissue. Each cell is represented by a single point and points are grouped by similar gene expression profiles. In this plot, 15 clusters were identified and assigned a color as shown on the right. These 15 clusters were labeled as a specific cell type based on known genetic expression markers.
Displaying Data From Single Cell Techniques
One of the most common graphical representations of single cell data is a T-Distributed Stochastic Neighbor Embedding (tSNE) plot, which is useful for visualizing cell populations (38). In a tSNE plot, cells are represented by dots and are grouped by similar gene expression profiles (Figure 1B). The data is represented as isolated clusters and the grouped cell types (e.g., podocytes vs. proximal tubular cells) can be identified based on known gene expression markers. Another plot commonly used to display single cell data is a violin plot. This plot displays the expression level of a given gene (y axis) by cell type clusters (cluster group along the x-axis).
Strengths and Limitations of Single Cell Techniques
The advantages of single cell techniques are the ability to identify rare and new cell types and trace developmental relationships between heterogeneous cells (e.g., which cells produce similar proteins, transporters or hormone receptors) and cellular states (e.g., how cells change with health and disease) (34). Methodologically, DropSeq is a high-resolution technique that reduces technical variability and reduces amplification bias (32, 36, 39). Other advantages include relatively inexpensive set-up, which is cost-efficient for large numbers of heterogeneous cell samples (36, 37). One potential disadvantage of DropSeq is an event called a doublet; two cells are combined in one droplet, thereby all subsequent RNA transcripts are identified as originating from a single cell, rather than two cells. Additionally, capture efficiency is on average only 5% of input cells: data loss occurs when a cell is injected into a droplet without a bead. Another disadvantage of DropSeq technique includes potential apoptosis or membrane damage from the single cell dissociation, which may alter the transcriptional profile of studied cells or release mitochondrial RNA transcripts falsely elevating total transcript expression (40). These undesired events can obscure the mapping of raw reads to the genome. Techniques have been developed to overcome harsh tissue digestion methods (41, 42) and false elevations in transcript numbers (40), and there are analytical ways to clean raw data, removing mitochondrial mRNA. Although DropSeq can identify a cell type through differential mRNA expression of a single cell, there is no way to directly identify cell types based on shape, size, lineage, or location within the tissue (32).
Evolving Technology
One of the largest quality improvements made on DropSeq is a technique termed 10X genomics. 10x genomics is a completely automated technique that allows for higher sequencing depth and speed as well as increased yield, which is suitable for more sensitive, smaller samples (43, 44). However, this technology comes at a cost (34): 10X genomics is 3 times more expensive than DropSeq analysis (45). C1 Fluidigm is single cell RNA sequencing using a chip instead of a droplet design to capture cells for higher throughput (31, 36). Another variation (Cell Expression by Linear Amplification and Sequencing: CEL-seq) creates a more efficient process by using in vitro transcription to combine barcoding and amplication steps into a single reaction (46–49). Table 1 describes the strengths, limitations, and recent applications of these technologies as well as bulk techniques.
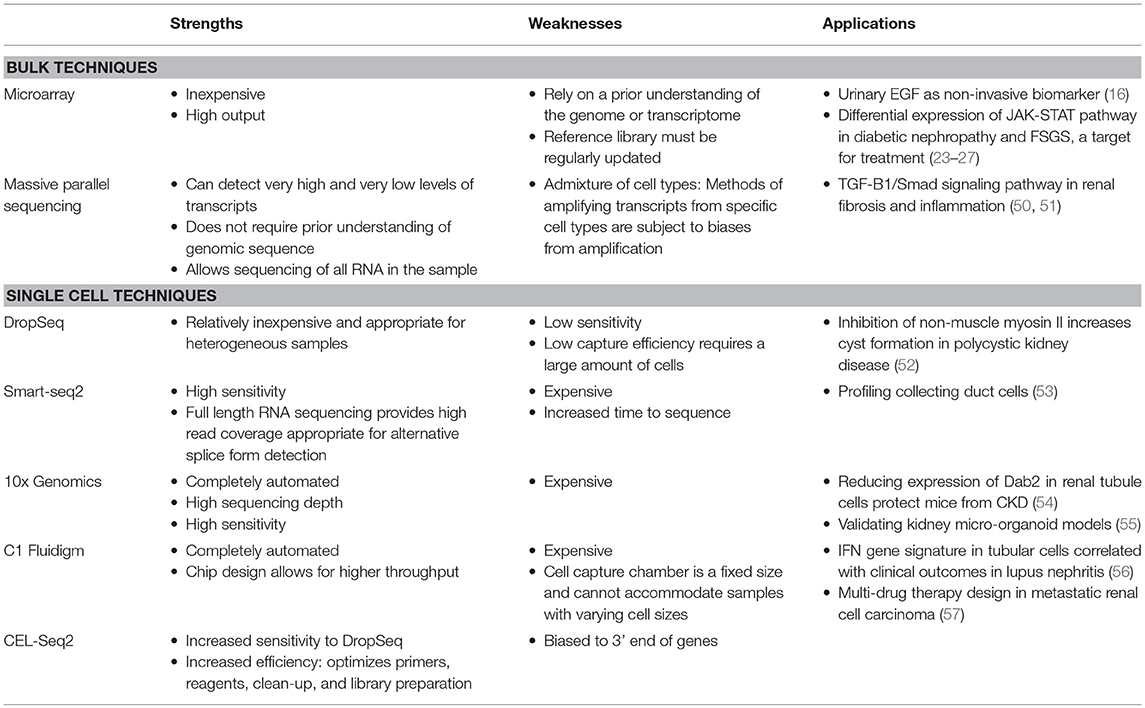
Table 1. Strengths, weaknesses, and recent applications of bulk and single transcriptomic techniques in kidney disease.
Examples of Single Cell Transcriptomics Analysis in Clinical Disease
Developing a Kidney Cell Atlas to Discover New Kidney Cell Types
Without an accessible all-encompassing classification system for kidney cell types, researchers have been relying on fragmented systems based on one aspect of cell identity: location, function, or genetic profile. To combine these classification systems to create an all-inclusive cell atlas, Park et al used droplet-based single-cell RNA sequencing to catalog mouse kidney cell types from over 57,000 cells from seven healthy mice (58). Analysis revealed 16 distinct cell clusters, and each cluster's identity was assigned by performing differential gene expression analysis and comparing those unique genes to known cell type specific markers. They also identified novel markers for the cell types. To confirm that human mendelian kidney diseases show cell type specificity translatable to murine cell types, a literature search was conducted for mouse homolog genes to human genes associated with monogenic inheritance of a specific phenotype (e.g., nephrotic syndrome or renal tubular acidosis). The mouse homolog genes identified were present in the same cell-specific clusters as human genes. For example, mouse homolog genes to human genes causing podocyte dysfunction and nephrotic syndrome were identified in the murine podocyte cluster and, similarly, mouse homolog genes to human genes causing intercalated cell dysfunction, and renal tubular acidosis were identified in the murine intercalated cell cluster. The murine kidney cell atlas will be integral in understanding of novel kidney cell types and disease pathogenesis. For example, three murine cell clusters did not express genes of a known cell type, prompting further investigation to classify these new cell types. In one cluster, genes known to be expressed in both intercalated cells and principle cells were present, suggesting a new transitional cell type. To confirm that these transitional cells originated from intercalated cells, Notch cell signaling was induced in intercalated cells, resulting in production of cells expressing both transitional and principle cell markers. To understand the functional consequence of this new transitional cell type in CKD, bicarbonate levels and transitional cell expression were measured in mouse model of CKD. Increased transitional cells were associated with worsening metabolic acidosis in the murine kidney. These data showed that intercalated to principle cell transition was dependent on Notch signaling and may be in the causal pathway of metabolic acidosis in CKD, which is an area for further study.
In this example, single cell data was used to comprehensively identify cell types within mouse kidney tissue. It allowed direct comparison of mouse and human kidney cell types to assess similarities and differences, critical for using mouse models to study human disease. It also identified novel cell types with potential implications for identifying biological processes, previously not appreciated in humans. The human cell atlas (www.humancellatlas.org) project is working to create reference maps of all human cells.
Understanding Novel Disease Transcriptional Signatures
Single cell transcriptomic techniques have been combined with another recent technologic advance: the ability to derive kidney organoids, a simplified cohort of human kidney cell types induced from pluripotent stem cells, that could be used as a new model system (vs. animal models) to study human disease. For example, Harder et al has shown through single cell transcriptional profiling of podocytes from kidney organoids, that organoid podocyte lineage cells have two distinct transcriptional states (59). These podocyte transcriptional states are comparable to developing human kidneys: the genes that defined the two different human kidney organoid podocyte clusters were the same genes that defined the two different developing human kidney podocyte clusters. These two distinct transcriptional states of organoid podocytes may represent two developmental stages of podocyte cells, supporting the hypothesis that transcriptional plasticity may be used as a compensatory mechanism in glomerular disease. To further understand the effect of two developmental podocyte stages on glomerular disease, an early glomerular epithelial (EGE) gene signature and mature podocyte gene signature were created from the clusters. Interestingly, many genes in the EGE signature resembled genes dysregulated in diseased glomeruli. Specifically, three genes LYPD1, PRSS23, and CDH6 were differentially regulated in EGE cells and strongly associated with increased proteinuria and loss of kidney function from prior analyses of glomerular tissue in two human kidney disease cohorts (59). These data suggest that podocyte loss or dedifferentiation to an earlier cell type, as suggested by increased EGE gene expression, is associated with CKD, making these newly identified genes involved in glomerular pathology potential targets for investigation.
Organoids have also been used in combination with single cell transcriptomics to better understand specific pathology, such as polycystic kidney disease. Cyst size and total kidney volume in polycystic kidney disease are associated with disease progression and mortality. However, phenotypes in polycystic kidney disease differ by family and mutation, which is an area of continued research. To interrogate different phenotypes of polycystic kidney disease, Czerniecki et al. edited genes of kidney organoids to produce cysts. DropSeq analysis of these gene-edited models showed that inhibition of non-muscle myosin II increased cyst formation, and this process is potentially regulated though polycystin-1, a pathway for future investigation (52).
These examples show that analysis of cell specific transcriptional changes in cell development, health, and disease, can lead to identification of novel genes and pathways that may be relevant to human disease.
Conclusions and Future Directions
Bulk and single cell transcriptomic profiling techniques each have unique contributions to advancing our understanding of kidney disease. These techniques can help us identify cell types and mechanisms relevant to disease processes that thus far have largely been defined by histopathology, which does not well-capture the underlying biologic heterogeneity of CKD and nephrotic syndromes. As demonstrated in the examples provided in this mini-review, interrogations of the kidney transcriptome have helped researchers understand mechanisms and biomarkers of disease. Exciting applications now and in the near future include further interrogation of these discoveries in animal models, targeting these disease pathways with medications with the goal of delaying progression of CKD, and helping researchers identify subgroups of patients who might be most likely to respond to specific pharmacologic therapies. These approaches may benefit all patients with kidney disease, but in particular pediatric patients with a higher likelihood of a germline genomic mutations have much to potentially gain. Translation of this technology to clinical practice in nephrology is not yet available, however application of similar techniques in this bench to bedside paradigm has revolutionized the field of oncology. Ultimately, the goal of applying transcriptomic analyses to blood, urine, and kidney biopsy specimens of individual patients to better provide targeted treatments for their nephrotic syndrome and CKD is part of the future of nephrology.
Author Contributions
All authors listed have made a substantial, direct and intellectual contribution to the work, and approved it for publication.
Funding
AO was supported by an institutional training grant funded by the NIH/NIDDK, grant number: 5 T32 DK 7378-39. The granting agency had no role in the conduct of this study. There is no other funding relevant to this manuscript. LH was supported by an individual career development grant from NIH/NIDDK, 5 K08 DK115891.
Conflict of Interest Statement
The authors declare that the research was conducted in the absence of any commercial or financial relationships that could be construed as a potential conflict of interest.
Acknowledgments
The authors would like to thank Rajasree Menon for generating an example tSNE plot.
References
1. Schena M, Shalon D, Davis RW, Brown PO. Quantitative monitoring of gene expression patterns with a complementary DNA microarray. Science. (1995) 270:467–70. doi: 10.1126/science.270.5235.467
2. Schena M, Shalon D, Heller R, Chai A, Brown PO, Davis RW. Parallel human genome analysis: microarray-based expression monitoring of 1000 genes. Proc Natl Acad Sci USA. (1996) 93:10614–9. doi: 10.1073/pnas.93.20.10614
3. Cohen CD, Frach K, Schlöndorff D, Kretzler M. Quantitative gene expression analysis in renal biopsies: a novel protocol for a high-throughput multicenter application. Kidney Int. (2002) 61:133–40. doi: 10.1046/j.1523-1755.2002.00113.x
4. Cohen CD, Gröne HJ, Gröne EF, Nelson PJ, Schlöndorff D, Kretzler M. Laser microdissection and gene expression analysis on formaldehyde-fixed archival tissue. Kidney Int. (2002) 61:125–32. doi: 10.1046/j.1523-1755.2002.00112.x
5. Brenner S, Johnson M, Bridgham J, Golda G, Lloyd DH, Johnson D, et al. Gene expression analysis by massively parallel signature sequencing (MPSS) on microbead arrays. Nat Biotechnol. (2000) 18:630–4. doi: 10.1038/76469
6. Pepke S, Wold B, Mortazavi A. Computation for ChIP-seq and RNA-seq studies. Nat Methods. (2009) 6:S22–32. doi: 10.1038/nmeth.1371
7. Hill NR, Fatoba ST, Oke JL, Hirst JA, O'Callaghan CA, Lasserson DS, et al. Global prevalence of chronic kidney disease - a systematic review and meta-analysis. PLoS ONE. (2016) 11:e0158765. doi: 10.1371/journal.pone.0158765
8. United States Renal Data System. Annual Data Report 2018. Available online at: https://www.usrds.org/adr.aspx (accessed December 9, 2018).
9. Kaspar CD, Bholah R, Bunchman TE. A review of pediatric chronic kidney disease. Blood Purif. (2016) 41:211–7. doi: 10.1159/000441737
10. Kaspar CD, Bholah R, Bunchman TE. KDIGO 2012 clinical practice guideline for the evaluation and management of chronic kidney disease. Kidney Int Suppl. (2013) 3:136–50. doi: 10.1038/kisup.2012.72
11. Schwartz GJ, Muñoz A, Schneider MF, Mak RH, Kaskel F, Warady BA, et al. New equations to estimate GFR in children with CKD. J Am Soc Nephrol. (2009) 20:629–37. doi: 10.1681/ASN.2008030287
12. McMahon GM, Waikar SS. Biomarkers in nephrology: core curriculum 2013. Am J Kidney Dis. (2013) 62:165–78. doi: 10.1053/j.ajkd.2012.12.022
13. Michels WM, Grootendorst DC, Verduijn M, Elliott EG, Dekker FW, Krediet RT. Performance of the cockcroft-gault, MDRD, and new CKD-EPI formulas in relation to GFR, age, and body size. Clin J Am Soc Nephrol. (2010) 5:1003–9. doi: 10.2215/CJN.06870909
14. Diaz Encarnacion MM, Griffin MD, Slezak JM, Bergstralh EJ, Stegall MD, Velosa JA, et al. Correlation of quantitative digital image analysis with the glomerular filtration rate in chronic allograft nephropathy. Am J Transplant. (2004) 4:248–56. doi: 10.1046/j.1600-6143.2003.00311.x
15. Barbour SJ, Espino-Hernandez G, Reich HN, Coppo R, Roberts IS, Feehally J, et al. The MEST score provides earlier risk prediction in lgA nephropathy. Kidney Int. (2016) 89:167–75. doi: 10.1038/ki.2015.322
16. Ju W, Nair V, Smith S, Zhu L, Shedden K, Song PXK, et al. Tissue transcriptome-driven identification of epidermal growth factor as a chronic kidney disease biomarker. Sci Transl Med. (2015) 7:316ra193. doi: 10.1126/scitranslmed.aac7071
17. Salido EC, Lakshmanan J, Fisher DA, Shapiro LJ, Barajas L. Expression of epidermal growth factor in the rat kidney. an immunocytochemical and in situ hybridization study. Histochemistry. (1991) 96:65–72. doi: 10.1007/BF00266763
18. Humes HD, Cieslinski DA, Coimbra TM, Messana JM, Galvao C. Epidermal growth factor enhances renal tubule cell regeneration and repair and accelerates the recovery of renal function in postischemic acute renal failure. J Clin Invest. (1989) 84:1757–61. doi: 10.1172/JCI114359
19. Betz BB, Jenks SJ, Cronshaw AD, Lamont DJ, Cairns C, Manning JR, et al. Urinary peptidomics in a rodent model of diabetic nephropathy highlights epidermal growth factor as a biomarker for renal deterioration in patients with type 2 diabetes. Kidney Int. (2016) 89:1125–35. doi: 10.1016/j.kint.2016.01.015
20. Li B, Zhang Y, Wang F, Nair V, Ding F, Xiao H, et al. Urinary epidermal growth factor as a prognostic marker for the progression of Alport syndrome in children. Pediatr Nephrol. (2018) 33:1731–9. doi: 10.1007/s00467-018-3988-1
21. Azukaitis KJW, Kirchner M, Nair V, Smith M, Fan Z, Thurn-Valsassina D, et al. Low levels of urinary epidermal growth factor predict chronic kidney disease progression in children. Kidney Int. (2019) 96:214–24. doi: 10.1016/j.kint.2019.01.035
22. Breyer MD, Böttinger E, Brosius FC, Coffman TM, Harris RC, Heilig CW, et al. Mouse models of diabetic nephropathy. J Am Soc Nephrol. (2005) 16:27–45. doi: 10.1681/ASN.2004080648
23. Berthier CC, Zhang H, Schin M, Henger A, Nelson RG, Yee B, et al. Enhanced expression of Janus kinase-signal transducer and activator of transcription pathway members in human diabetic nephropathy. Diabetes. (2009) 58:469–77. doi: 10.2337/db08-1328
24. Hodgin JB, Nair V, Zhang H, Randolph A, Harris RC, Nelson RG, et al. Identification of cross-species shared transcriptional networks of diabetic nephropathy in human and mouse glomeruli. Diabetes. (2013) 62:299–308. doi: 10.2337/db11-1667
25. Marrero MB, Banes-Berceli AK, Stern DM, Eaton DC. Role of the JAK/STAT signaling pathway in diabetic nephropathy. Am J Physiol Renal Physiol. (2006) 290:F762–8. doi: 10.1152/ajprenal.00181.2005
26. Tuttle KR, Brosius FC, Adler SG, Kretzler M, Mehta RL, Tumlin JA, et al. JAK1/JAK2 inhibition by baricitinib in diabetic kidney disease: results from a Phase 2 randomized controlled clinical trial. Nephrol Dial Transplant. (2018) 33:1950–9. doi: 10.1093/ndt/gfx377
27. Tao J, Mariani L, Eddy S, Maecker H, Kambham N, Mehta K, et al. JAK-STAT signaling is activated in the kidney and peripheral blood cells of patients with focal segmental glomerulosclerosis. Kidney Int. (2018) 94:795–808. doi: 10.1016/j.kint.2018.05.022
28. Fragiadaki M, Lannoy M, Themanns M, Maurer B, Leonhard WN, Peters DJ, et al. STAT5 drives abnormal proliferation in autosomal dominant polycystic kidney disease. Kidney Int. (2017) 91:575–86. doi: 10.1016/j.kint.2016.10.039
29. Pang M, Ma L, Gong R, Tolbert E, Mao H, Ponnusamy M, et al. A novel STAT3 inhibitor, S3I-201, attenuates renal interstitial fibroblast activation and interstitial fibrosis in obstructive nephropathy. Kidney Int. (2010) 78:257–68. doi: 10.1038/ki.2010.154
30. Ju W, Greene CS, Eichinger F, Nair V, Hodgin JB, Bitzer M, et al. Defining cell-type specificity at the transcriptional level in human disease. Genome Res. (2013) 23:1862–73. doi: 10.1101/gr.155697.113
31. Wu H, Humphreys BD. The promise of single-cell RNA sequencing for kidney disease investigation. Kidney Int. (2017) 92:1334–42. doi: 10.1016/j.kint.2017.06.033
32. Klein AM, Mazutis L, Akartuna I, Tallapragada N, Veres A, Li V, et al. Droplet barcoding for single-cell transcriptomics applied to embryonic stem cells. Cell. (2015) 161:1187–201. doi: 10.1016/j.cell.2015.04.044
33. Miyamoto DT, Zheng Y, Wittner BS, Lee RJ, Zhu H, Broderick KT, et al. RNA-Seq of single prostate CTCs implicates noncanonical Wnt signaling in antiandrogen resistance. Science. (2015) 349:1351–6. doi: 10.1126/science.aab0917
34. Haque A, Engel J, Teichmann SA, Lönnberg T. A practical guide to single-cell RNA-sequencing for biomedical research and clinical applications. Genome Med. (2017) 9:75. doi: 10.1186/s13073-017-0467-4
35. Zheng GX, Terry JM, Belgrader P, Ryvkin P, Bent ZW, Wilson R, et al. Massively parallel digital transcriptional profiling of single cells. Nat Commun. (2017) 8:14049. doi: 10.1038/ncomms14049
36. Ziegenhain C, Vieth B, Parekh S, Reinius B, Guillaumet-Adkins A, Smets M, et al. Comparative analysis of single-cell RNA sequencing methods. Mol Cell. (2017) 65:631–43 e4. doi: 10.1016/j.molcel.2017.01.023
37. Macosko EZ, Basu A, Satija R, Nemesh J, Shekhar K, Goldman M, et al. Highly parallel genome-wide expression profiling of individual cells using nanoliter droplets. Cell. (2015) 161:1202–14. doi: 10.1016/j.cell.2015.05.002
38. Andrews TS, Hemberg M. Identifying cell populations with scRNASeq. Mol Aspects Med. (2018) 59:114–22. doi: 10.1016/j.mam.2017.07.002
39. Alles J, Karaiskos N, Praktiknjo SD, Grosswendt S, Wahle P, Ruffault PL, et al. Cell fixation and preservation for droplet-based single-cell transcriptomics. BMC Biol. (2017) 15:44. doi: 10.1186/s12915-017-0383-5
40. Kretzler M, Menon R. Single-cell sequencing the glomerulus, unraveling the molecular programs of glomerular filtration, one cell at a time. J Am Soc Nephrol. (2018) 29:2036–8. doi: 10.1681/ASN.2018060626
41. Adam M, Potter AS, Potter SS. Psychrophilic proteases dramatically reduce single-cell RNA-seq artifacts: a molecular atlas of kidney development. Development. (2017) 144:3625–32. doi: 10.1242/dev.151142
42. Lacar B, Linker SB, Jaeger BN, Krishnaswami SR, Barron JJ, Kelder MJE, et al. Nuclear RNA-seq of single neurons reveals molecular signatures of activation. Nat Commun. (2016) 7:11022. doi: 10.1038/ncomms12020
43. Behjati S, Tarpey PS. What is next generation sequencing? Arch Dis Child Educ Pract Ed. (2013) 98:236–8. doi: 10.1136/archdischild-2013-304340
44. Tang F, Barbacioru C, Wang Y, Nordman E, Lee C, Xu N, et al. mRNA-Seq whole-transcriptome analysis of a single cell. Nat Methods. (2009) 6:377–82. doi: 10.1038/nmeth.1315
45. Del Fabbro C, Scalabrin S, Morgante M, Giorgi FM. An extensive evaluation of read trimming effects on Illumina NGS data analysis. PLoS ONE. (2013) 8:e85024. doi: 10.1371/journal.pone.0085024
46. Kolodziejczyk AA, Kim JK, Svensson V, Marioni JC, Teichmann SA. The technology and biology of single-cell RNA sequencing. Mol Cell. (2015) 58:610–20. doi: 10.1016/j.molcel.2015.04.005
47. Hashimshony T, Wagner F, Sher N, Yanai I. CEL-Seq: single-cell RNA-Seq by multiplexed linear amplification. Cell Rep. (2012) 2:666–73. doi: 10.1016/j.celrep.2012.08.003
48. Hashimshony T, Senderovich N, Avital G, Klochendler A, de Leeuw Y, Anavy L, et al. CEL-Seq2: sensitive highly-multiplexed single-cell RNA-Seq. Genome Biol. (2016) 17:77. doi: 10.1186/s13059-016-0938-8
49. Jaitin DA, Kenigsberg E, Keren-Shaul H, Elefant N, Paul F, Zaretsky I, et al. Massively parallel single-cell RNA-seq for marker-free decomposition of tissues into cell types. Science. (2014) 343:776–9. doi: 10.1126/science.1247651
50. Brennan EP, Morine MJ, Walsh DW, Roxburgh SA, Lindenmeyer MT, Brazil DP, et al. Next-generation sequencing identifies TGF-beta1-associated gene expression profiles in renal epithelial cells reiterated in human diabetic nephropathy. Biochim Biophys Acta. (2012) 1822:589–99. doi: 10.1016/j.bbadis.2012.01.008
51. Lan HY, Chung AC. TGF-beta/Smad signaling in kidney disease. Semin Nephrol. (2012) 32:236–43. doi: 10.1016/j.semnephrol.2012.04.002
52. Czerniecki SM, Cruz NM, Harder JL, Menon R, Annis J, Otto EA, et al. High-throughput screening enhances kidney organoid differentiation from human pluripotent stem cells and enables automated multidimensional phenotyping. Cell Stem Cell. (2018) 22:929–40 e4. doi: 10.1016/j.stem.2018.04.022
53. Chen L, Lee JW, Chou CL, Nair AV, Battistone MA, Păunescu TG, et al. Transcriptomes of major renal collecting duct cell types in mouse identified by single-cell RNA-seq. Proc Natl Acad Sci USA. (2017) 114:E9989-98. doi: 10.1073/pnas.1710964114
54. Qiu C, Huang S, Park J, Park Y, Ko YA, Seasock MJ, et al. Renal compartment-specific genetic variation analyses identify new pathways in chronic kidney disease. Nat Med. (2018) 24:1721–31. doi: 10.1038/s41591-018-0194-4
55. Kumar SV, Er PX, Lawlor KT, Motazedian A, Scurr M, Ghobrial I, et al. Kidney micro-organoids in suspension culture as a scalable source of human pluripotent stem cell-derived kidney cells. Development. (2019) 146:dev172361. doi: 10.1242/dev.172361
56. Der E, Ranabothu S, Suryawanshi H, Akat KM, Clancy R, Morozov P, et al. Single cell RNA sequencing to dissect the molecular heterogeneity in lupus nephritis. JCI Insight. (2017) 2:e93009. doi: 10.1172/jci.insight.93009
57. Kim KT, Lee HW, Lee HO, Song HJ, Jeong da E, Shin S, et al. Application of single-cell RNA sequencing in optimizing a combinatorial therapeutic strategy in metastatic renal cell carcinoma. Genome Biol. (2016) 17:80. doi: 10.1186/s13059-016-0945-9
58. Park J, Shrestha R, Qiu C, Kondo A, Huang S, Werth M, et al. Single-cell transcriptomics of the mouse kidney reveals potential cellular targets of kidney disease. Science. (2018) 360:758–63. doi: 10.1126/science.aar2131
Keywords: transcriptomics, single cell transcriptomics, nephrotic syndrome, glomerular disease, chronic kidney disease
Citation: Oliverio AL, Bellomo T and Mariani LH (2019) Evolving Clinical Applications of Tissue Transcriptomics in Kidney Disease. Front. Pediatr. 7:306. doi: 10.3389/fped.2019.00306
Received: 16 April 2019; Accepted: 09 July 2019;
Published: 23 July 2019.
Edited by:
Frederick Jeffrey Kaskel, Children's Hospital at Montefiore, United StatesReviewed by:
Saritha Ranabothu, University of Arkansas for Medical Sciences, United StatesAndrew Mallett, Royal Brisbane and Women's Hospital, Australia
Copyright © 2019 Oliverio, Bellomo and Mariani. This is an open-access article distributed under the terms of the Creative Commons Attribution License (CC BY). The use, distribution or reproduction in other forums is permitted, provided the original author(s) and the copyright owner(s) are credited and that the original publication in this journal is cited, in accordance with accepted academic practice. No use, distribution or reproduction is permitted which does not comply with these terms.
*Correspondence: Laura H. Mariani, bG1hcmlhbmkmI3gwMDA0MDt1bWljaC5lZHU=