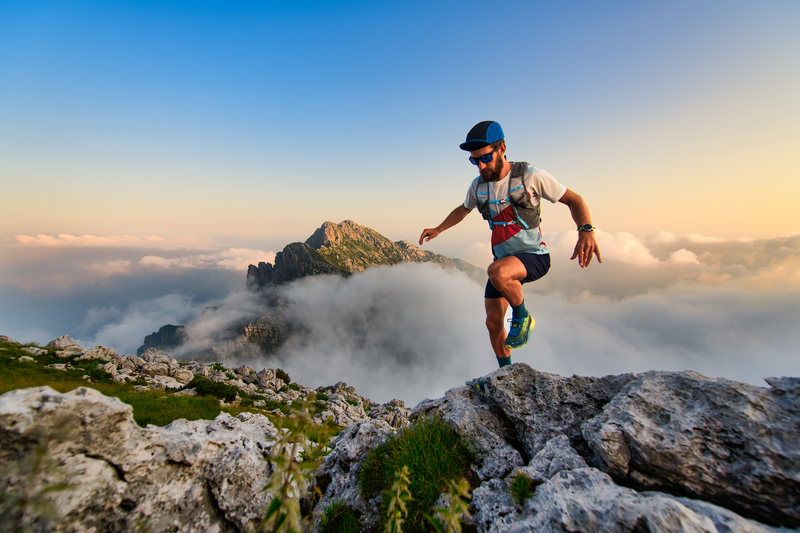
95% of researchers rate our articles as excellent or good
Learn more about the work of our research integrity team to safeguard the quality of each article we publish.
Find out more
ORIGINAL RESEARCH article
Front. Pain Res.
Sec. Cancer Pain
Volume 6 - 2025 | doi: 10.3389/fpain.2025.1567632
This article is part of the Research Topic AI in Cancer Pain management View all articles
The final, formatted version of the article will be published soon.
You have multiple emails registered with Frontiers:
Please enter your email address:
If you already have an account, please login
You don't have a Frontiers account ? You can register here
Introduction: Acute pain is common among oral cavity/oropharyngeal cancer (OCC/OPC) patients undergoing radiation therapy (RT). This study aimed to predict acute pain severity and opioid doses during RT using machine learning (ML), facilitating risk-stratification models for clinical trials.Methods: A retrospective study examined 900 OCC/OPC patients treated with RT during 2017-2023. Pain intensity was assessed using NRS (0-none, 10-worst) and total opioid doses were calculated using morphine equivalent daily dose (MEDD) conversion factors. Analgesics efficacy was assessed using combined pain intensity and total MEDD. ML predictive models were developed and validated, including Logistic Regression (LR), Support Vector Machine (SVM), Random Forest (RF), and Gradient Boosting Machine (GBM). Model performance was evaluated using discrimination and calibration metrics, while feature importance was investigated using bootstrapping.Results: For predicting pain intensity, the GBM demonstrated superior discrimination performance (AUROC 0.71, recall 0.39, and F1 score 0.48). For predicting the total MEDD, LR model outperformed other models (AUROC 0.67). For predicting analgesics efficacy, the SVM achieved the highest specificity (0.97), while the RF and GBM models achieved the highest AUROC (0.68). RF model emerged as the best calibrated model with an ECE of 0.02 and 0.05 for pain intensity and MEDD prediction, respectively. Baseline pain scores and vital signs demonstrated the most contributing features.ML models showed promise in predicting end-of-treatment pain intensity, opioid requirements and analgesics efficacy in OCC/OPC patients. Baseline pain score and vital signs are crucial predictors. Their implementation in clinical practice could facilitate early risk stratification and personalized pain management.
Keywords: Acute Pain, Opioid dose, radiation therapy, head and neck cancers, Oral cavity and oropharyngeal cancers, machine learning, artificial intelligence
Received: 27 Jan 2025; Accepted: 20 Mar 2025.
Copyright: © 2025 Salama, Humbert-Vidan, Godinich, Wahid, Elhabashy, Naser, He, Mohamed, Sahli, Hutcheson, Gunn, Rosenthal, Fuller and Moreno. This is an open-access article distributed under the terms of the Creative Commons Attribution License (CC BY). The use, distribution or reproduction in other forums is permitted, provided the original author(s) or licensor are credited and that the original publication in this journal is cited, in accordance with accepted academic practice. No use, distribution or reproduction is permitted which does not comply with these terms.
* Correspondence:
Vivian Salama, Cancer Institute, West Virginia University, Morgantown, United States
Amy C Moreno, University of Texas MD Anderson Cancer Center, Houston, 77030, Texas, United States
Disclaimer: All claims expressed in this article are solely those of the authors and do not necessarily represent those of their affiliated organizations, or those of the publisher, the editors and the reviewers. Any product that may be evaluated in this article or claim that may be made by its manufacturer is not guaranteed or endorsed by the publisher.
Research integrity at Frontiers
Learn more about the work of our research integrity team to safeguard the quality of each article we publish.