- 1Department of Orthopaedics, International Science and Technology Cooperation Base of Spinal Cord Injury, Tianjin Key Laboratory of Spine and Spinal Cord Injury, Tianjin Medical University General Hospital, Tianjin, China
- 2Department of Joint and Sport Medicine, Tianjin Union Medical Center, Nankai University Affiliated People’s Hospital, Tianjin, China
- 3Department of Spine Surgery, Tianjin Union Medical Center, Nankai University Affiliated People’s Hospital, Tianjin, China
Background: There is an urgent need to confirm biomarkers reflecting the pathogenesis and targeted drugs of lower back pain or/and sciatica in clinical practice. This study aimed to conduct a two sample bidirectional Mendelian randomization (MR) analysis to explore the causal link between 486 serum metabolites and lower back pain or/and sciatica.
Methods: All data come from two public shared databases of European ancestry and single nucleotide polymorphisms (SNPs) for lower back pain or/and sciatica acted as instrumental variables. The traditional inverse variance weighting (IVW) method, weighted-median method, MR-Egger methodand other methods were used to estimate causality. The horizontal pleiotropy, heterogeneities were also verified through the MR-Egger intercept test, Cochran's Q test, MR-PRESSO test and the leave-one-out sensitivity analysis. Reverse MR analysis was employed to evaluate the direct impact of metabolites on lower back pain or/and sciatica. Additionally, we conducted the colocalization analysis to reflect the causality deeply. Furthermore, metabolic pathway analysis was performed.
Results: 28 metabolites (18 known metabolites, 1 identified metabolites and 9 unknown metabolites) relevant to the risk of sciatica or/and lower back pain after using genetic variants as probes at PIVW < 0.05 were identifed. Among them, 8 serum metabolites decreased risk of sciatica or/and lower back pain significantly (P < 0.05), and 14 serum metabolites increased risk of sciatica or/and lower back pain significantly (P < 0.05). No reverse causal association was found between 28 metabolites and sciatica or/and lower back pain. Colocalization analysis results showed that the associations between sciatica or/and lower back pain and the 28 identified metabolites were not due to shared causal variant sites. Moreover, pathway enrichment analysis identifed 11 signifcant metabolic pathways, which are mainly involved in the pathological mechanism of sciatica or/and lower back pain (P < 0.05). There was no horizontal pleiotropy or heterogeneity in the other analyses.
Conclusion: Our analyses provided robust evidence of causal associations between blood metabolites on sciatica or/and lower back pain. However, the underlying mechanisms remain to be further investigated.
Introduction
Sciatica or/and lower back pain is not a medical diagnosis, but rather a symptom secondary to degenerative diseases of the lumbar spine such as disc herniation and spinal stenosis. In clinical practice, the vast majority of sciatica is related to nerve root compression caused by intervertebral disc herniation (IVDD), but mechanical compression alone cannot explain all phenomena. Sciatica, as a secondary symptom, involves complex processes such as decreased muscle strength, sensory loss and numbness, decreased reflexes, and abnormal sensations. It is currently believed that the inflammatory response between the nucleus pulposus and nerve roots plays an important role in discogenic sciatica or/and lower back pain (1, 2). Cytokines are a type of glycoprotein molecules produced by various cells and secreted into the extracellular space, possessing various biological functions. The role of cytokine mediated neuroimmune responses in sciatica has been widely studied (3, 4), pro-inflammatory cytokines can induce pain related behaviors (5), and anti-inflammatory cytokines can effectively alleviate hyperalgesia (6). However, it is unknown whether the differences in cytokine profiles are related to sciatica and pain intensity, and there is an urgent need to confirm biomarkers reflecting the intensity of sciatica in clinical practice.
However, with the development of metabolomics, the idea of identifying specific small molecule biomarkers in the serum of patients with sciatica or/and lower back pain and identifying potential causes has become possible (7, 8). Metabolomics is the systematic study of metabolic profiles in biological samples (cells, tissues, and body fluids). Usually, the low molecular weight of metabolites (<1,500 Da), which represent intermediate and/or final products of cell metabolism and cell cycle, may have different functions in different organisms. Metabolomics is an effective method for revealing the phenotype of biological molecules. This method can identify changes in small molecule metabolites in various diseases, which is of great help in understanding and diagnosing diseases. Several recent metabolic studies have documented numerous circulating markers, such as amino acids, sugars, and fats, in both human and animal models (9–11). However, due to restrictions in sample size and the presence of confounding factors, the direct impact of blood markers on sciatica or/and lower back pain remains unverified.
Mendelian randomization (MR) is a form of instrumental variable (IV) analysis that employs genetic variations as an IV to identify and measure causality (12). Due to its ability to mitigate the impact of potential confounding variables and reverse causality, MR has gained popularity in observational research in recent times. Moreover, non-experimental investigations frequently incorporate possible variables that may distort the results and the possibility of cause and effect being mistakenly identified (13–15). Thus, the objective of this study was to elucidate the genetic connection and potential causative link between 486 human serum metabolites and risks of sciatica or/and lower back pain through a bidirectional MR validation. Genome-wide association studies (GWAS) can uncover numerous genetic links, yet pinpointing causal variants as opposed to mere correlations continues to be a major hurdle. To refine these results, colocalization analysis provides evidence to ascertain if a single causal variant can affect multiple traits. Therefore, we carried out GWAS-GWAS colocalization analysis to detect and reduce false positives. This was accomplished by comparing gene loci across various GWAS, thereby ensuring a more precise identification of true genetic associations.
Methods
Our study is a Mendelian randomization study and we have conformed to the STROBE guidelines (see STROBE-MR-checklist in Supplementary Table S1). All published GWAS received ethical approval from the appropriate institutional review boards. This study utilized only summary-level data, eliminating the need for additional ethical approval.
Study design
This study used two sample bidirectional Mendelian randomization method to explore the causal relationship between 486 human serum metabolites and risks of sciatica or/and lower back pain. First, the association data of human serum metabolites (exposure factor) were obtained from a GWAS, so as to clarify the single nucleotide polymorphism (SNP) site associated with human serum metabolites, and treat it as gene instrumental variables estimation. Subsequently, another GWAS study was conducted to obtain association data with sciatica or/and lower back pain (outcome) and clarify the existence of relevant SNPs; Finally, through the screened SNPs and various statistical methods, we can comprehensively judge the causal relationship between 486 human serum metabolites and risks of sciatica or/and lower back pain.
Data sources
We obtained summary association data from the most extensive genetic investigation into human metabolism, which was openly accessible on the Metabolomics GWAS Server (website: http://metabolomics.helmholtz-muenchen.de/gwas/) (16). Utilizing a dataset comprising 7,824 individuals from two European population cohorts, a comprehensive set of 486 metabolites was measured using the MS (Metabolon) platform. Subsequently, GWAS analyses were conducted utilizing the genotype dataset imputed based on HapMap2. In the current study, we included 177 metabolites whose chemical identity had not been definitively determined, along with 309 confirmed metabolites. Additionally, these 309 known metabolites were further categorized into eight biochemical classes (peptides, energy, nucleotides, lipids, amino acids, cofactors and vitamins, carbohydrates, and xenobiotics) based on the Kyoto Encyclopedia of Genes and Genomes (KEGG) database. It is worth mentioning that comprehensive genotyping information for the two cohorts has been extensively described in previous research (17). Finally, the GWAS meta-analysis encompassed approximately 2.1 million SNPs. Data related to sciatica or/and lower back pain is derived from the GWAS dataset (https://gwas.mrcieu.ac.uk/datasets/finn-b-M13_LOWBACKPAINORANDSCIATICA/). The sample size of this dataset is 218,792 (ncase = 19,509, ncontrol = 199,283), including Europeans with a SNP count of 16,380,466.
Genetic variants
Based on the requirements of MR analysis, genetic variants as instrumental variables (IVs) need to fulfill the following three fundamental assumptions: (I) a robust correlation exists between instrumental variables and the exposure factor X; (II) instrumental variables are not linked to any confounding factors affecting the association between exposure and outcome; and (III) instrumental variables do not influence outcome Y, except for a possible indirect impact through their association with exposure X (18–20). Figure 1 depicts the fundamental assumptions and workflow of Mendelian randomization (MR). To identify instrumental variables (IVs) for the 486 metabolites, certain procedures were undertaken to ensure the validity of the first assumption. Initially, genetic variants were extracted using association thresholds of P < 1 × 10–5, which are commonly employed in MR analysis to capture greater variability when limited SNPs are available for exposure. Subsequently, independent variants were identified through a clumping procedure implemented in R software, with a linkage-disequilibrium threshold of r2 < 0.01 within a 500 kb window based on the European 1,000 Genomes Project Phase 3 reference panel. Instrumental SNPs were selected by excluding palindromic SNPs with a middle allele frequency (MAF). MR Steiger filters were used to exclude SNPs with an incorrect causal direction. Finally, in order to assess the strength of the selected instruments quantitatively, we calculated the explained variance (R2) and the F statistic for each metabolite. Typically, a threshold of F > 10 is recommended for further MR analysis.
MR statistical analyses
In this study, five statistical methods, simple mode, weighted mode, inverse varianceweighted analysis (IVW), weighted median method and MR-Egger regression, were used to analyze the causal association between human serum metabolites and risks of sciatica or/and lower back pain. As the most well-established research approach in Mendelian randomization, the IVW analysis method (utilizing a random effects model when heterogeneity exists and a fixed effects model when there is no heterogeneity) conducts weighted analysis on genetic variants that satisfy the criteria of valid instrumental variables. The weighted midpoint approach necessitates that a minimum of 50% of SNPs fulfill the requirement of being effective instrumental variables. Once the SNPs are arranged in order of their weights, the analysis outcome is derived from the median of the associated distribution function. MR-Egger regression analysis can still gauge the causal impact of the outcome on the condition that the incorporated SNPs exhibit gene pleiotropy. The slope of the regression is the causal effect estimate of human serum metabolites on the trend of sciatica or/and lower back pain. However, due to its low test efficiency and wide confidence interval results, MR-Egger analysis is often used as a sensitivity analysis for other statistical results. MR-PRESSO is a different innovative MR technique that has the capability to identify outliers and adjust for horizontal pleiotropy, thus yielding an accurate estimation (15). All outcomes are presented as odds ratios (OR) along with their corresponding 95% confidence intervals (CI), and a p-value of less than 0.05 is deemed statistically significant. The statistical analysis, employing the Two Sample MR 0.5.6 and MR-PRESSO packages, was conducted using R software version 3.6.0. The leave-one-out method was employed to assess the sensitivity of the Mendelian randomization findings. After sequentially removing the incorporated SNPs, the IVW technique was employed to compute the effect of analysis on the remaining all SNPs, and the impact of an individual SNP on the estimation of causal judgment was determined accordingly. The asymmetry plot from the funnel plot was utilized to identify any heterogeneity, and a symmetric plot confirmed the lack of heterogeneity. An additional indication that horizontal multiplicity is absent can be observed through the symmetry of the funnel plot. Additionally, the false positive rate rose because of the numerous exposure factors and various exposure phenotypes in GWAS originating from the same sample set. To mitigate this, we utilized the false discovery rate (FDR < 0.05) to adjust the MR results.
Reverse MR analysis
A reverse MR analysis was performed to evaluate if sciatica or/and lower back pain influences the levels of candidate serum metabolites. The MR analysis was conducted following the previously described methodology.
Colocalization analysis
We performed a colocalization analysis using the coloc R package to investigate whether the association between the identified metabolites and sciatica or/and lower back pain was due to a shared causal variant (21). This involved analyzing regional loci within 1,000 kb on either side of the lead SNP in the exposure data, thereby reducing the likelihood of reinforcing false associations between the two phenotypes. Within a Bayesian framework, the coloc method assessed posterior probabilities for five hypotheses (H0, H1, H2, H3, H4) at each variant locus: (1) no association with either trait; (2) association with only trait 1; (3) association with only trait 2; (4) associations with both traits due to different causal variants; and (5) both traits sharing a common causal variant (22). We used default priors (p1 = 1 × 10–4, p2 = 1 × 10–4, p12 = 1 × 10–5) for the colocalization analysis. The presence of a posterior probability over 80% for H4 (PP4) under various prior and window conditions was considered strong evidence of colocalization.
Metabolic pathway analysis
To investigate the roles of the identified metabolites, MetaboAnalyst 5.0 (https://www.metaboanalyst.ca/) (23) was employed for conducting metabolic pathway analysis. In order to obtain extensive and reliable pathway analysis outcomes, all metabolites significantly associated with sciatica or/and lower back pain (with a P-value < 0.05) were included. For this purpose, two databases, namely the Small Molecule Pathway database (SMPDB) and the KEGG database, were utilized. The significance level for the pathway analysis was set at 0.10.
Results
Influence of 486 serum metabolites on sciatica or/and lower back pain (forward MR)
Determination of IVs
We conducted a two-sample MR analysis to assess the causal relationship between genetically determined metabolites and sciatica or/and lower back pain using four distinct pairs of GWAS summary data. The instrumental variables (IVs) generated for the 480 metabolites ranged from 3 to 481 SNPs, with fructose having the fewest IVs (3 SNPs), and 2-methoxyacetaminophen sulfate* having the most IVs (481 SNPs). Furthermore, all IVs were adequately effective for conducting MR analysis on the 480 metabolites (F-statistic >10) (see Supplementary Table S2).
Association between serum metabolites and sciatica or/and lower back pain
The IVW approach was utilized to validate the causal relationship between the 480 metabolites and sciatica or/and lower back pain. In total, 28 distinct metabolites were confirmed with a PIVW value of less than 0.05 and FDR < 0.05, out of which 18 were previously identified metabolites (tyrosine, malate, pentadecanoate (15:0), benzoate, aspartate, 1,5-anhydroglucitol (1,5-AG), 1-palmitoylglycerol (1-monopalmitin), levulinate (4-oxovalerate), glycine, 3-methylxanthine, C-glycosyltryptophan*, adrenate (22:4n6), alpha-hydroxyisovalerate, N-acetylthreonine, 1-stearoylglycerophosphocholine, 2-stearoylglycerophosphocholine*, hydroquinone sulfate, 1-myristoylglycerophosphocholine), 1 identified metabolites (X-11445–5-alpha-pregnan-3beta,20alpha-disulfate) and 9 unknown metabolites (X-03088, X-11820, X-11852, X-12040, X-12189, X-12261, X-12726, X-12850, X-14632) in Table 1. The P values obtained from IVW analysis for the aforementioned estimates were below 0.05, and the findings from MREgger and WM methods exhibited consistent effects. This suggests the robustness of the two causal discoveries mentioned above (X-12189 and X-12261). To be more specific, X-03088 (OR: 0.66, 95% CI: 0.48–0.91, P = 0.0105), 3-methylxanthine (OR: 0.81, 95% CI: 0.66–0.99, P = 0.0424), adrenate (22:4n6) (OR: 0.59, 95% CI: 0.38–0.93, P = 0.0214), X-11820 (OR: 0.76, 95% CI: 0.60–0.96, P = 0.0227), X-12189 (OR: 0.95, 95% CI: 0.90–0.99, P = 0.0289), X-12261 (OR: 0.88, 95% CI: 0.82–0.95, P = 0.0013), N-acetylthreonine (OR: 0.64, 95% CI: 0.41–1.00, P = 0.0486), and X-12726 (OR: 0.86, 95% CI: 0.76–0.98, P = 0.0193) decreased risk of sciatica or/and lower back pain significantly; tyrosine (OR: 1.94, 95% CI: 1.15–3.25, P = 0.0127), pentadecanoate (15:0) (OR: 0.66, 95% CI: 0.48–0.91, P = 0.0373), aspartate (OR: 1.72, 95% CI: 1.01–2.92, P = 0.0472), 1,5-anhydroglucitol (1,5-AG) (OR: 1.38, 95% CI: 1.04–1.84, P = 0.0251), 1-palmitoylglycerol (1-monopalmitin) (OR: 1.55, 95% CI: 1.01–2.36, P = 0.0438), glycine (OR: 1.38, 95% CI: 1.10–1.74, P = 0.0064), C-glycosyltryptophan* (OR: 2.25, 95% CI: 1.17–4.29, P = 0.0144), X-11445-5-alpha-pregnan-3beta,20alpha-disulfate (OR: 1.14, 95% CI: 1.01–1.29, P = 0.0328), X-12040 (OR: 1.04, 95% CI: 1.00–1.09, P = 0.0497), 1-stearoylglycerophosphocholine (OR: 1.61, 95% CI: 1.12–2.30, P = 0.0093), X-12850 (OR: 1.42, 95% CI: 1.02–1.97, P = 0.0399), hydroquinone sulfate (OR: 1.09, 95% CI: 1.00–1.18, P = 0.0426), 1-myristoylglycerophosphocholine (OR: 1.53, 95% CI: 1.02–2.28, P = 0.0382), and X-14632 (OR: 1.17, 95% CI: 1.02–1.34, P = 0.0261) increased risk of sciatica or/and lower back pain significantly.
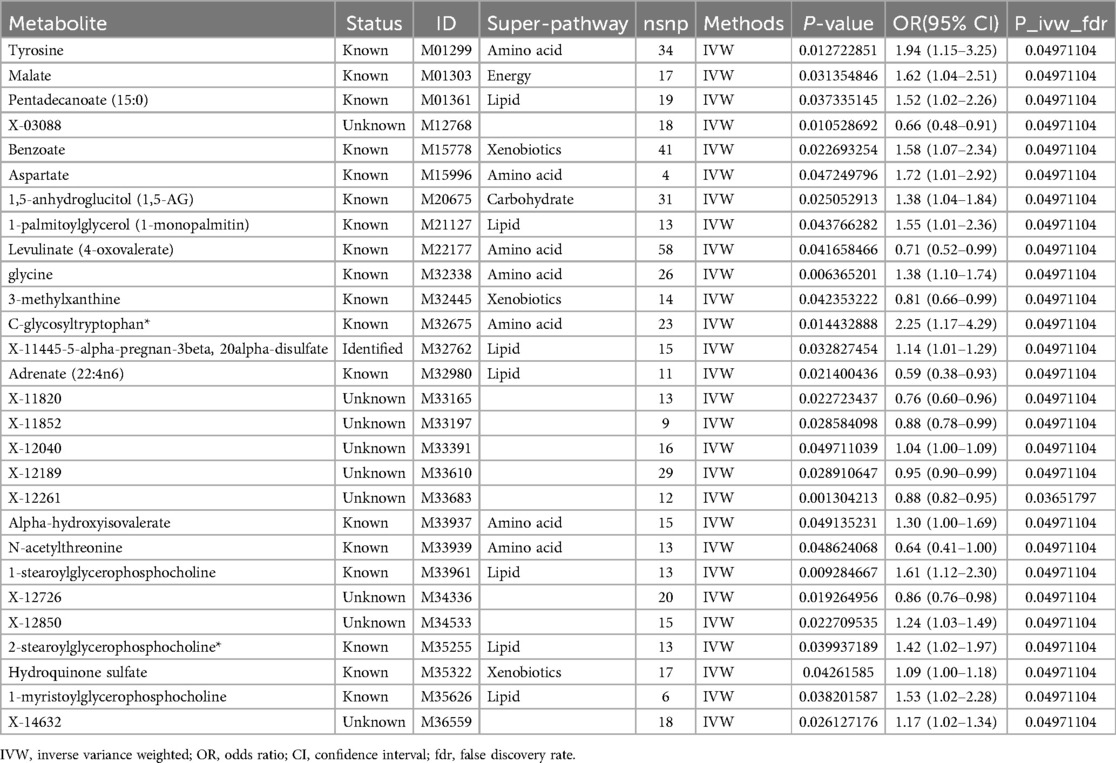
Table 1. Causal effect of 28 metabolites on the risk of sciatica or/and lower back pain derived from IVW.
Heterogeneity analysis and pluripotency analysis
The comparison between serum metabolites and sciatica or/and lower back pain was examined using Cochran's Q test for heterogeneity. The results indicated that there was no significant heterogeneity, with IVW and MR Egger. Consequently, the fixed-effects model was chosen for MR analysis. Only two comparisons [adrenate (22:4n6) and X-12189 on sciatica or/and lower back pain] showed horizontal pleiotropy causing that P values were <0.05 in MR-Egger and IVW Cochran's Q tests. The analysis outcomes for heterogeneity and pluripotency are displayed in Table 2. To evaluate the MR analysis results, five methods were employed, and scatter plots were produced (Figure 2; Supplementary Figure S1). Among these methods, MR-Egger was utilized to assess the pluripotency of IVs. The intercept of MR-Egger did not significantly differ from the zero intercept of IVW, indicating the absence of horizontal pluripotency. Furthermore, as demonstrated by the funnel plot (Figure 3; Supplementary Figure S2), there was no apparent symmetry in the variation of effect size around the estimated point. This lack of symmetry can be attributed to the limited sample size of the SNP and should not be interpreted as an absence of horizontal pleiotropy.
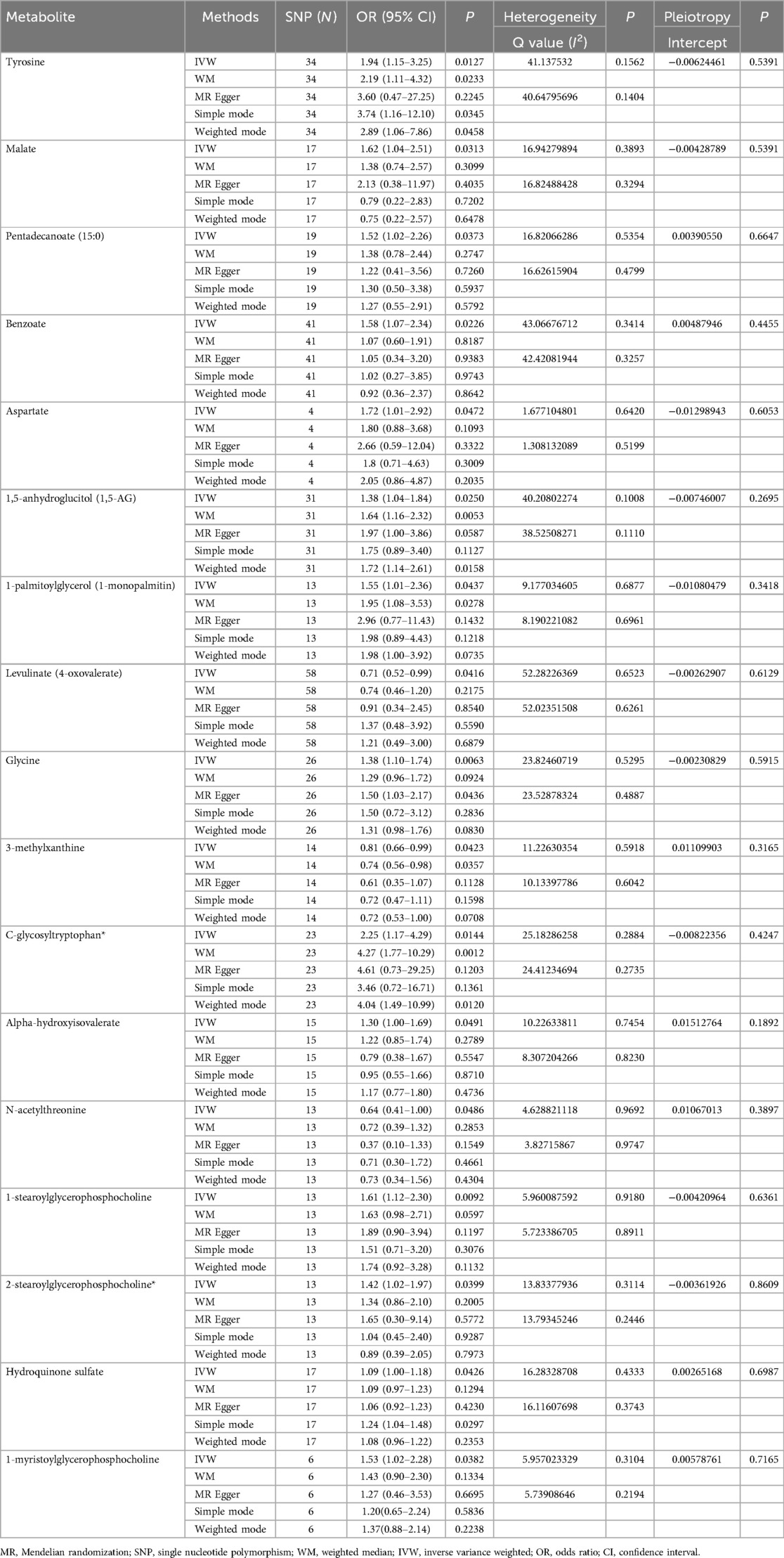
Table 2. Five MR models estimate the causal relationships between 17 known metabolites and the risk of sciatica or/and lower back pain and tests for heterogeneity and horizontal pleiotropy.
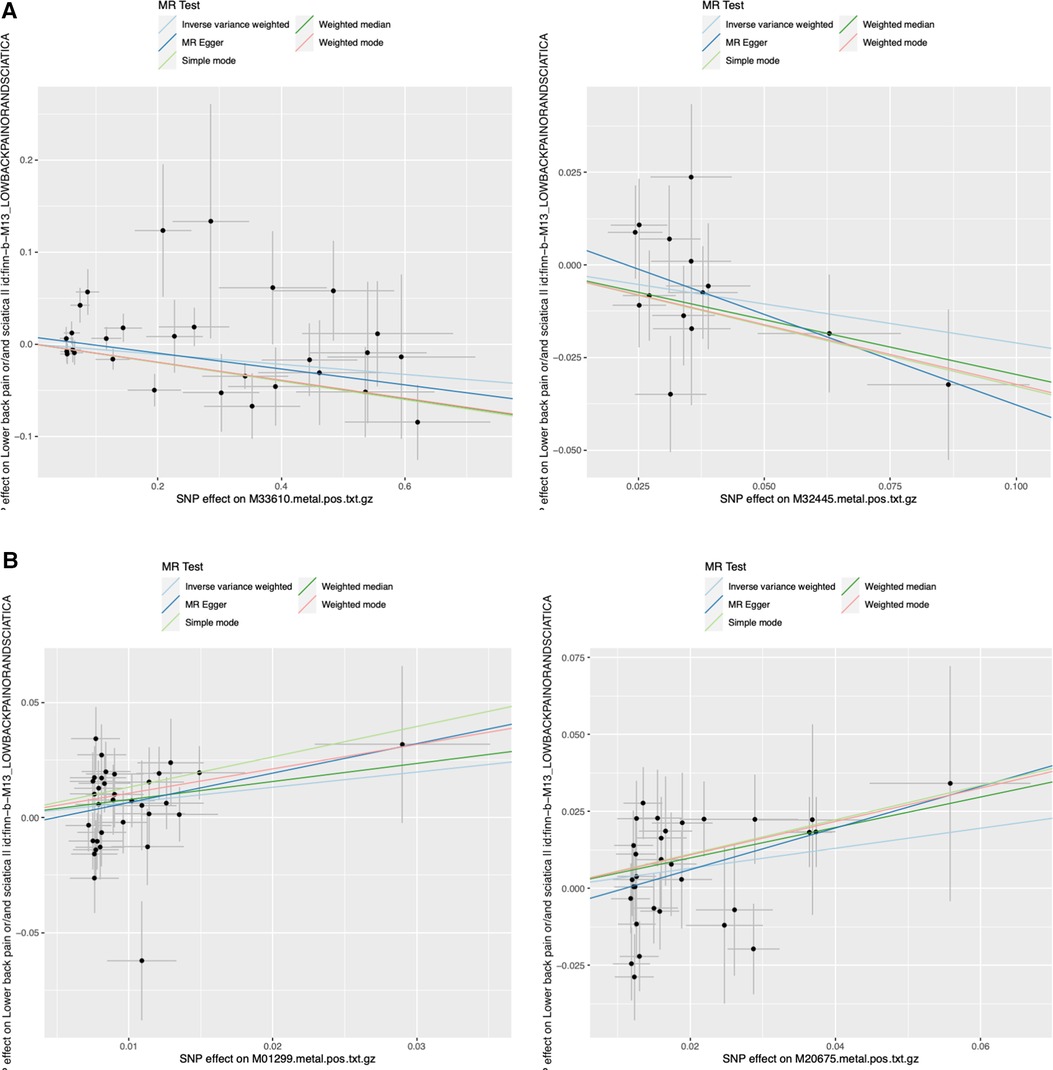
Figure 2. Representative scatter plots of the 5MR models for 22 screened metabolites with potential causal relationship with sciatica or/and lower back pain. MR, Mendelian randomization; SNP, single nucleotide polymorphism. (A) 2 metabolites (M33610 and M32445) decreased risk of sciatica or/and lower back pain significantly; (B) 2 metabolites (M01299 and M20675) increased risk of sciatica or/and lower back pain significantly.
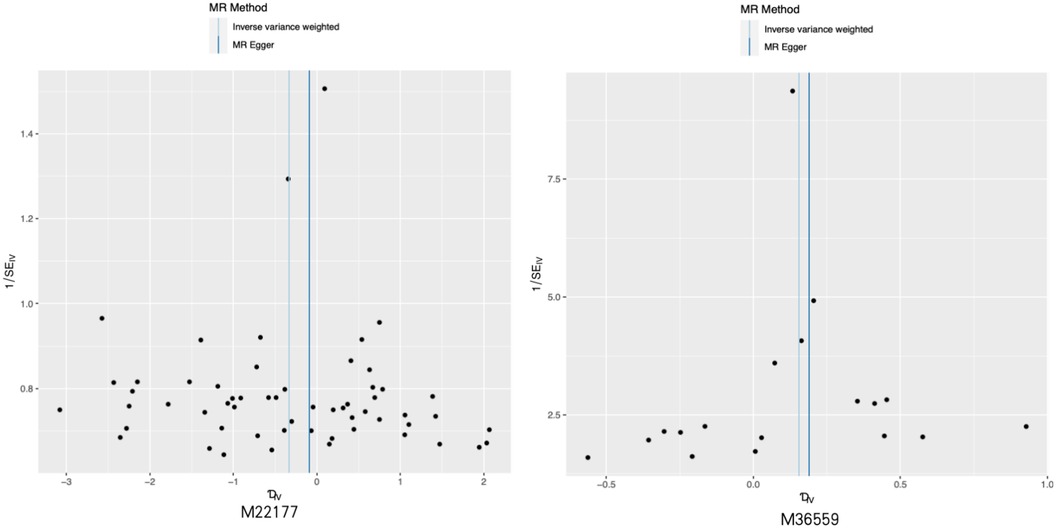
Figure 3. Representative funnel plot maps of 28 metabolites with potential causal relationship with sciatica or/and lower back pain. MR, Mendelian randomization.
Leave-one-out analysis
We performed an analysis called leave-one-out to compute the MR result of the remaining IVs when each one was removed individually. Removing each SNP did not have a significant impact on the overall error line, as shown in Figure 4; Supplementary Figure S3. Hence, the correlation analysis results from the two-sample MR study were relatively dependable.
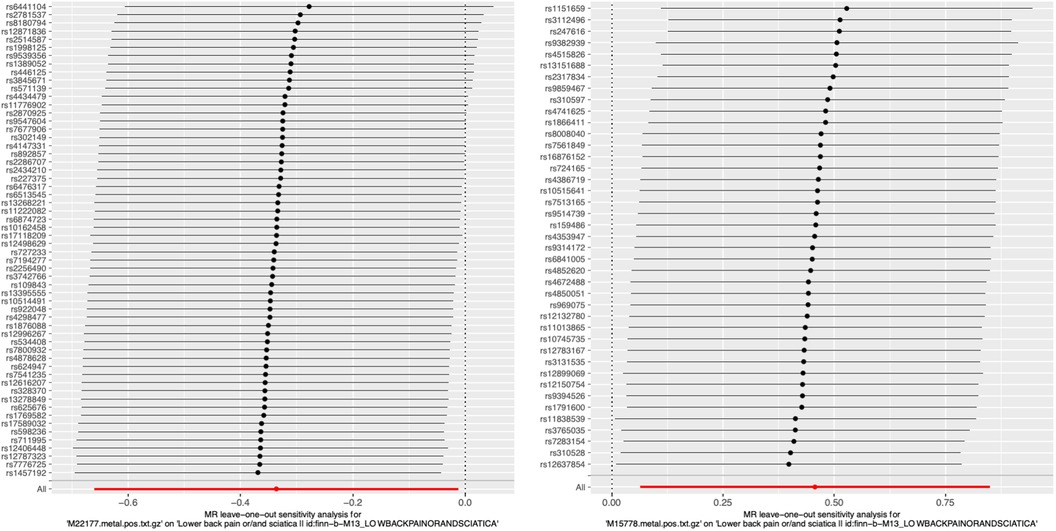
Figure 4. Representative leave-one-out forest maps of 28 metabolites with potential causal relationship with sciatica or/and lower back pain. MR, Mendelian randomization.
Influence of sciatica or/and lower back pain on the candidate serum metabolites (reverse MR)
Reverse MR analysis was subsequently conducted to assess the impact of sciatica and/or lower back pain on the 28 candidate serum metabolites identified in the forward MR analysis. SNPs that met a significance threshold of P < 1 × 10−5 were selected as genetic instruments closely linked to sciatica and/or lower back pain and utilized for LD clumping. However, no association was found between genetic predisposition to sciatica and/or lower back pain and changes in the concentrations of the candidate metabolites in the IVW analysis, as well as in the simple mode, weighted mode, weighted median, and MR-Egger analyses (Table 3).
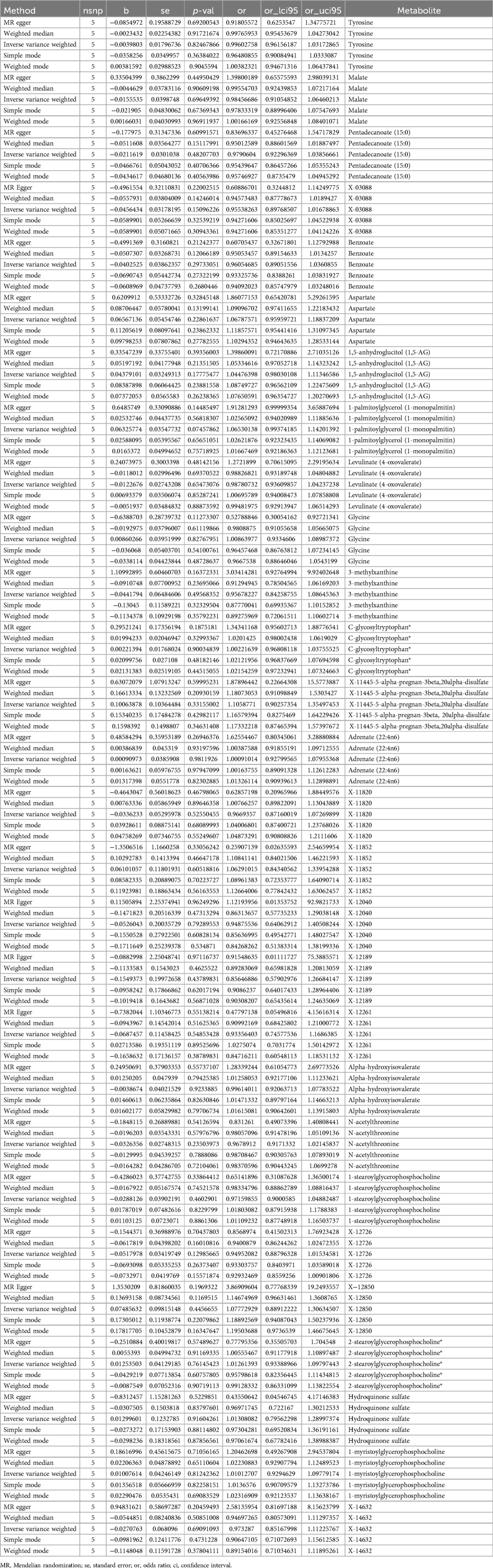
Table 3. Complementary reverse MR analyses of sciatica or/and lower back pain for causal association with blood metabolites.
Colocalization analysis
For the 28 identified metabolites linked to sciatica or/and lower back pain risk in this study, we performed a colocalization analysis to evaluate their relationship with sciatica or/and lower back pain outcomes. This analysis used exposure data from a 1,000 kb region around the lead SNP to ensure that the observed associations were not influenced by the same causal variant within that area. This strategy aimed to reduce the chance of a false association between the two phenotypes (see Table 4). The results of the colocalization analysis showed that the associations between sciatica or/and lower back pain and the 28 identified metabolites were not due to shared causal variant sites.
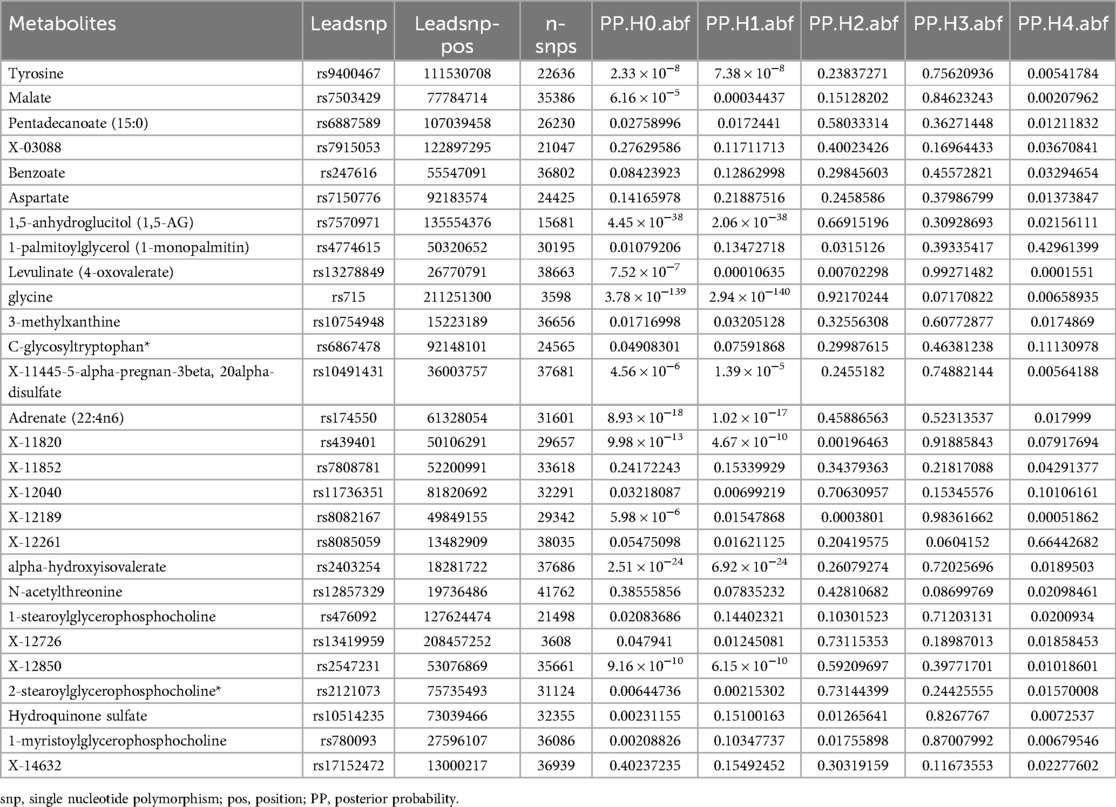
Table 4. The colocalization analysis illustrating the associations between 28 metabolites and sciatica or/and lower back pain.
Metabolic pathway analysis
The metabolic pathway analysis identifed 11 signifcant metabolic pathways in sciatica or/and lower back pain (Figure 5). Our results show that the Aminoacyl-tRNA biosynthesis (p = 0.00010925), Glyoxylate and dicarboxylate metabolism (p = 0.0024154), Phenylalanine, tyrosine and tryptophan biosynthesis (p = 0.010293), Ubiquinone and other terpenoid-quinone biosynthesis (0.023046), Phenylalanine metabolism (p = 0.025582), Arginine biosynthesis (p = 0.035677), Nicotinate and nicotinamide metabolism (p = 0.038188), Histidine metabolism (p = 0.040694), Pantothenate and CoA biosynthesis (p = 0.048184) pathways were identified to be linked with the underlying mechanism of sciatica or/and lower back pain (Table 5).
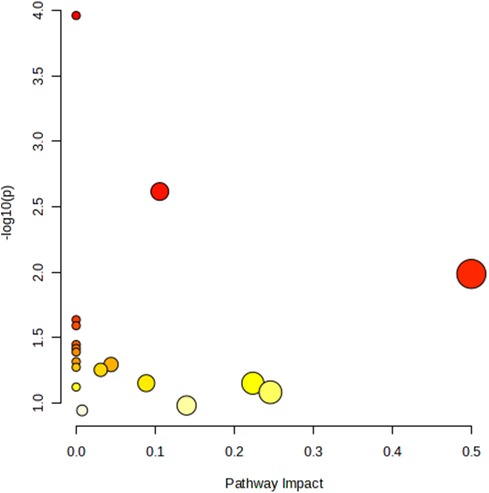
Figure 5. Metabolic pathway analysis and KEGG pathway enrichment analysis of 18 known metabolites by MetaboAnalyst5.0.
Discussion
Principal findings
In this bidirectional comparison between two sets of samples, sciatica or/and lower back pain was found to have a suggestive connection with levels of some serum metabolites. We identifed 28 metabolites (18 known metabolites, 1 identified metabolites and 9 unknown metabolites) relevant to the risk of sciatica or/and lower back pain after using genetic variants as probes at PIVW < 0.05 and FDR < 0.05. Among them, 8 serum metabolites decreased risk of sciatica or/and lower back pain significantly (P < 0.05), and 14 serum metabolites increased risk of sciatica or/and lower back pain significantly (P < 0.05). Our study did not identify any blood metabolites with bidirectional effects. Furthermore, such GWAS-GWAS co-localization analysis provided robust evidence of the causal relationships unaffected by overlapping SNPs. Pathway enrichment analysis identifed 11 signifcant metabolic pathways, which are mainly involved in the pathological mechanism of sciatica or/and lower back pain (P < 0.05).
Previous studies and potential mechanisms
The pathological mechanism of sciatica or/and lower back pain is complex. On one hand, previous research has shown that pro-inflammatory cytokines can induce and exacerbate inflammatory reactions and neurogenic pain. Animal experiments have confirmed that the imbalance between pro-inflammatory and anti-inflammatory factors, as well as the conversion of anti-inflammatory cytokines to pro-inflammatory cytokines, can also increase the sensitivity of neurogenic pain (24). The characteristic of inflammatory pain is an increase in sensitivity to mechanical or thermal stimulation of the affected tissue. After tissue damage, local macrophages produce inflammatory reactions and further expand through migration of blood cells. Different inflammatory agents and tissue acidification work together to induce and sustain the progression of pain and hyperalgesia. On the other hand, discogenic sciatica or/and lower back pain is more likely due to the protrusion of the nucleus pulposus into the epidural space, which increases the sensitivity of sensory neurons to various stimuli. Pain is not always caused by nerve pain receptors, damage to nerve axons, and abnormal stimulation of the dorsal root ganglia (DRG) and dorsal horn of the spinal cord can also generate ectopic nerve impulses to induce pain. The sensory neurons of DRG are stimulated, increasing their overall activity while also lowering their threshold for various stimuli (25). DRG is adjacent to the spinal cord, innervating the limbs and core tissues, and transmitting electrical signals to the central nervous system (CNS) (26). These neurons can detect various stimuli and transmit proprioceptions, cold and hot sensations, mechanical pain, and injury sensations. The heightened function of primary sensory neurons enhances the release of neurotransmitters (like glutamate) and neuromodulators (including substance P, calcitonin gene-related peptide, and brain-derived neurotrophic factor) within the spinal cord. This results in an excessive activation of postsynaptic nociceptive neurons, a phenomenon known as central sensitization (27). Central sensitization is accountable for secondary pain experienced beyond the original injury site. Additionally, the activation of mitogen-activated protein kinase (MAPK) plays a crucial role in central sensitization and contributes to the development of pain hypersensitivity responses (28).
It can be observed from this that amino acid neurotransmitters play a crucial role in influencing the development of neuropathic pain in specific contexts. For instance, in a rat model of chronic constrictive injury, electroacupuncture (EA) relieves neuropathic pain by decreasing the release of excitatory amino acid transmitters like glutamate, glutamine, and aspartate, while enhancing the release of inhibitory amino acid transmitters such as glycine, gamma-aminobutyric acid (GABA), and taurine within the spinal cord (29). Glutamate, being one of the most abundant neurotransmitters in the spinal dorsal horn, assumes a key role in facilitating excitatory signaling associated with pain (30, 31). When utilizing a microdialysis method to collect spinal cord dialysates from rats with a spinal nerve ligation model, researchers noticed reduced levels of aspartate and glutamate in the dialysates obtained from rats treated with EA compared to sham EA controls. Based on these findings, Ma et al. concluded that EA has the ability to alleviate neuropathic pain in this experimental model by partially inhibiting the levels of aspartate and glutamate within the spinal dorsal horn (32).
In this MR analysis, specific amino acid metabolites such as tyrosine, aspartate, and glycine were identified as neurotransmitters associated with sciatica or/and lower back pain—findings consistent with previous research. Kobayashi et al. emphasize the significance of comprehending the vascular system's morphological characteristics that supply the dorsal root ganglion (DRG), as well as the neurogenic regulation of intraganglionic blood flow, in understanding the mechanisms behind low back pain and sciatica. This study confirmed the presence of dopaminergic nerves within the vasculature of the DRGs, which rely on the amino acid tyrosine from the bloodstream (33). Abnormalities in neurotransmitters consisted of elevated levels of glutamate and reduced levels of GABA. Watson's findings provide evidence that an imbalanced ratio of excitatory and inhibitory neurotransmitters, particularly in brain regions such as the insula, is present in chronic pain conditions. This imbalance contributes to heightened central pain processing and heightened sensitivity to pain (34). Maintaining glutamate homeostasis and regulating microglia activation are both significant factors in the progression and persistence of neuropathic pain. Yang et al. conducted a study and validated that the administration of ultra-low dose naloxone, either alone or in conjunction with morphine, had the ability to modify the levels of excitatory amino acids (EAAs) such as glutamate and aspartate. Additionally, they observed changes in the expression of tumor necrosis factor-α (TNF-α) and its receptors (TNFR1 and TNFR2) in the dorsal horn of the spinal cord in rats with partial transection of the sciatic nerve (35). Wang et al. discovered that the levels of certain serum metabolites related to linoleic acid metabolism, alanine, aspartate, and glutamate, glycerophospholipids, and the citrate cycle, exhibited a significant decrease following treatment with Lactobacillus paracasei S16 in LDH mice. Conversely, the metabolite associated with purine metabolism demonstrated a significant increase. These findings indicate that the administration of Lactobacillus paracasei S16 can ameliorate inflammatory responses, modulate gut microbiota, and influence serum metabolomics in an LDH mouse model, thereby contributing to the alleviation of low back pain (36). Multiple investigations have demonstrated that the natural neurosteroid allopregnanolone (AP) exhibits analgesic, neuroprotective, antidepressant, and anxiolytic effects. These beneficial effects are attributed to AP's ability to modulate GABAA receptors, glycine receptors, and L- and T-type calcium channels. This indicates that AP, with its high therapeutic potential and favorable toxicological profile, holds promise in developing effective and safe strategies for managing chronic neuropathic pain (37). Dysfunction of spinal strychnine-sensitive glycine receptors is associated with the development of chronic pain conditions and movement disorders. Therefore, enhancing the activity of glycine receptors could potentially serve as a treatment approach for such disorders. The ventral horn of the spinal cord, which is responsible for pain-induced motor reflexes and muscle tone regulation, contains the highest levels of glycine in the central nervous system. 4-Bromopropofol, acting through glycine receptors, has the potential to serve as a starting point for developing non-sedative, non-addictive muscle relaxants and analgesics for the treatment of low back pain (38,38). Furthermore, the correlation between the aforementioned serum metabolites, as confirmed by our MR study, aligns with previous research findings. Additionally, our MR study identified another 25 serum metabolites that have not been reported in the existing literature but hold potential as biomarkers for future investigations into the mechanisms and drug targets associated with lower back pain and/or sciatica.
In this study, the metabolic pathway analysis showed that Aminoacyl-tRNA biosynthesis, Glyoxylate and dicarboxylate metabolism, Phenylalanine, tyrosine and tryptophan biosynthesis, Phenylalanine metabolism, Ubiquinone and other terpenoid-quinone biosynthesis, Arginine biosynthesis, Nicotinate and nicotinamide metabolism, Histidine metabolism, Pantothenate and CoA biosynthesis pathways are mainly associated with sciatica or/and lower back pain (P < 0.05). Of them, Phenylalanine metabolism, Phenylalanine, tyrosine and tryptophan biosynthesis, Glycine, serine and threonine metabolism (P > 0.05), Tyrosine metabolism (P > 0.05), and Alanine, aspartate and glutamate metabolism (P > 0.05) were all belongs to amino acid neurotransmitter pathway which had been proven to be closely related to the pathogenesis of sciatica by our MR results and previous literatures. Altogether, these findings suggest that amino acid neurotransmitter pathway played an important role in the biological mechanism of asciatica or/and lower back pain.
Strengths and limitations
Our research has many positive aspects. This analysis is the initial thorough MR investigation of lower back pain or/and sciatica with blood metabolites, examining proof of the bidirectional cause-and-effect relationship between blood metabolites on lower back pain or/and sciatica. Additionally, the MR methodology minimized the possibility of confounding variables and other biases influencing the reported bias. A substantial sample size and GWAS SNPs provided statistical reliability for evaluating causality. These measures enhance the validity of our conclusions. Third, both exposure and outcome were from the European population, avoiding population bias. Moreover, the samples of exposure and outcome in this study are from different databases, which reduces the possible bias in the effect sizes caused by the overlap of exposure and outcome samples. Co-localization analysis was also undertaken, reinforcing the credibility of our results.
However, our research does have certain limitations. Primarily, the majority of these GWAS data originate from populations in Europe. It is crucial to ascertain whether the conclusions we outlined remain applicable to other individuals. Secondly, we employed FDR multiple analysis correction for all 486 serum metabolites, and the all p-values after FDR corrections are greater than 0.05 (see Supplementary Table S3), which is because a large number of samples undergoing FDR correction simultaneously can result in false negatives. The primary objective of our study was to identify potential biomarkers for lower back pain and/or sciatica, as well as potential targets for treatment. However, implementing strict corrections such as Bonferroni or FDR correction could have resulted in the exclusion of significant findings. Therefore, this study only adjusted the P-values of 28 identified serum metabolites for FDR, and the adjusted P-values were all less than 0.05, which is consistent with previous results. Thus, we believe that such positive results are meaningful. Lastly, while this study encompassed a relatively extensive range of metabolites, the functions and mechanisms of some of these metabolites in the context of the disease were not entirely elucidated. Consequently, our interpretation of the results obtained from this MR analysis was limited.
Conclusions
In conclusion, the comprehensive findings from this study indicate that there are 28 serum metabolites, including 18 known metabolites, 1 identified metabolite, and 9 unknown metabolites, which might have a causal role in the development of lower back pain and/or sciatica. These findings were derived from a large GWAS summary statistics database. Additionally, this research identified 11 significant metabolic pathways that could be relevant to the underlying pathology of lower back pain and/or sciatica. Overall, our results provide valuable insights into the potential use of certain metabolites as biomarkers for investigating targeted drug therapies for human diseases. However, further studies are necessary to validate these findings.
Data availability statement
The original contributions presented in the study are included in the article/Supplementary Material, further inquiries can be directed to the corresponding authors.
Author contributions
Y-MR: Writing – original draft, Writing – review & editing. W-YH: Writing – original draft, Writing – review & editing. B-YF: Writing – original draft, Writing – review & editing. Y-HD: Writing – original draft, Writing – review & editing. Y-BS: Writing – original draft, Writing – review & editing. TY: Writing – original draft, Writing – review & editing. H-JZ: Writing – original draft, Writing – review & editing. T-WS: Writing – original draft, Writing – review & editing. M-QT: Writing – original draft, Writing – review & editing.
Funding
The author(s) declare financial support was received for the research, authorship, and/or publication of this article. This research is supported by Tianjin Science and Technology Major Special Project (21ZXJBSY00080), Tianjin Natural Science Foundation’s Diversified Investment Youth Project (21JCQNJC01300), and National Natural Science Foundation Youth Program (82102563 and 82102671).
Conflict of interest
The authors declare that the research was conducted in the absence of any commercial or financial relationships that could be construed as a potential conflict of interest.
Publisher's note
All claims expressed in this article are solely those of the authors and do not necessarily represent those of their affiliated organizations, or those of the publisher, the editors and the reviewers. Any product that may be evaluated in this article, or claim that may be made by its manufacturer, is not guaranteed or endorsed by the publisher.
Supplementary material
The Supplementary Material for this article can be found online at: https://www.frontiersin.org/articles/10.3389/fpain.2024.1370704/full#supplementary-material
Supplementary Figure S1 | All rest scatter plots of the 5MR models for 22 screened metabolites with potential causal relationship with sciatica or/and lower back pain.
Supplementary Figure S2 | All rest funnel plot maps of 28 metabolites with potential causal relationship with sciatica or/and lower back pain.
Supplementary Figure S3 | All rest leave-one-out forest maps of 28 metabolites with potential causal relationship with sciatica or/and lower back pain.
Supplementary Table S1 | The STROBE-MR reporting specification.
Supplementary Table S2 | The information on instrumental variables and SNPs identified by the Mendelian randomization estimates.
Supplementary Table S3 | The all p-values after FDR corrections of all 486 serum metabolites.
Abbreviations
SCI, spinal cord injury; MR, Mendelian randomization; GWAS, genome wide association study; SNP, single nucleotide polymorphism; IVW, inverse varianceweighted analysis; LD, linkage disequilibrium; PMN, polymorphonuclear leukocytes; H2O2, hydrogen peroxide; MMPs, matrix metalloproteinases; PCA, principal component analysis.
References
1. Koes BW, van Tulder MW, Peul WC. Diagnosis and treatment of sciatica. Br Med J. (2007) 334:1313–7. doi: 10.1136/bmj.39223.428495.BE
2. Violante FS, Mattioli S, Bonfiglioli R. Low-back pain. Handb Clin Neurol. (2015) 131:397–410. doi: 10.1016/B978-0-444-62627-1.00020-2s
3. Wuertz K, Haglund L. Inflammatory mediators in intervertebral disk degeneration and discogenic pain. Global Spine J. (2013) 3:175–84. doi: 10.1055/s-0033-1347299
4. Freemont AJ. The cellular pathobiology of the degenerate intervertebral disc and discogenic back pain. Rheumatology (Oxford). (2009) 48:5–10. doi: 10.1093/rheumatology/ken396
5. Brisby H, Olmarker K, Larsson K, Nutu M, Rydevik B. Proinflammatory cytokines in cerebrospinal fluid and serum in patients with disc herniation and sciatica. Eur Spine J. (2002) 11:62–6. doi: 10.1007/s005860100306
6. Mendes Fonseca M, Santa-Cecilia F, Kusuda R, Ferreira D, Bezerra F, Ferreira M, et al. (153) the interleukin 27 (IL-27) protects mice from neuropathic pain development through up-regulation of antiinflammatory cytokine IL-10. J Pain. (2017) 18(4):S14–5. doi: 10.1016/j.jpain.2017.02.059
7. Zhou C, Shi X, Huang H, Zhu Y, Wu Y. Montelukast attenuates neuropathic pain through inhibiting p38 mitogen-activated protein kinase and nuclear factor-kappa B in a rat model of chronic constriction injury. Anesth Analg. (2014) 118(5):1090–6. doi: 10.1213/ANE.0000000000000174
8. Li H-L, Wu H, Zhang B-B, Shi H-L, Wu X-J. MAPK Pathways are involved in the inhibitory effect of berberine hydrochloride on gastric cancer MGC 803 cell proliferation and IL-8 secretion in vitro and in vivo. Mol Med Rep. (2016) 14:1430–8. doi: 10.3892/mmr.2016.5361
9. Johnson CH, Ivanisevic J, Siuzdak G. Metabolomics: beyond biomarkers and towards mechanisms. Nat Rev Mol Cell Biol. (2016) 17:451–9. doi: 10.1038/nrm.2016.25
10. Lains I, Gantner M, Murinello S, Lasky-Su JA, Miller JW, Friedlander M, et al. Metabolomics in the study of retinal health and disease. Prog Retin Eye Res. (2019) 69:57–79. doi: 10.1016/j.preteyeres.2018.11.002
11. Yin X, Chan LS, Bose D, Jackson AU, VandeHaar P, Locke AE, et al. Genome-wide association studies of metabolites in Finnish men identify disease-relevant loci. Nat Commun. (2022) 13:1644. doi: 10.1038/s41467-022-29143-5
12. Holmes M, Ala-Korpela M, Smith G. Mendelian Randomization in cardiometabolic disease: challenges in evaluating causality. Nat Rev Cardiol. (2017) 14:577–90. doi: 10.1038/nrcardio.2017.78
13. Lawlor DA, Harbord RM, Sterne JA, Timpson N, Smith GD. Mendelian Randomization: using genes as instruments for making causal inferences in epidemiology. Stat Med. (2008) 27:1133–63. doi: 10.1002/sim.3034
14. Smith GD, Hemani G. Mendelian Randomization: genetic anchors for causal inference in epidemiological studies. Hum Mol Genet. (2014) 23:R89–98. doi: 10.1093/hmg/ddu328
15. Smith GD, Ebrahim S. Data dredging, bias, or confounding. Br Med J. (2002) 325(7378):1437–8. doi: 10.1136/bmj.325.7378.1437
16. Shin S-Y, Fauman EB, Petersen A-K, Krumsiek J, Santos R, Huang J, et al. An atlas of genetic influences on human blood metabolites. Nat Genet. (2014) 46:543–50. doi: 10.1038/ng.2982
17. Suhre K, Shin S-Y, Petersen A-K, Mohney RP, Meredith D, Wägele B, et al. Human metabolic individuality in biomedical and pharmaceutical research. Nature. (2011) 477:54–60. doi: 10.1038/nature10354
18. van Kippersluis H, Rietveld CA. Pleiotropy-robust Mendelian randomization. Int J Epidemiol. (2018) 47(4):1279–88. doi: 10.1093/ije/dyx002
19. Chen L, Yang H, Li H, He C, Yang L, Lv G. Insights into modifable risk factors of cholelithiasis: a Mendelian randomization study. Hepatology. (2022) 75(4):785–96. doi: 10.1002/hep.32183
20. Verbanck M, Chen C-Y, Neale B, Do R. Detection of widespread horizontal pleiotropy in causal relationships inferred from Mendelian randomization between complex traits and diseases. Nat Genet. (2018) 50:693–8. doi: 10.1038/s41588-018-0099-7
21. Giambartolomei C, Vukcevic D, Schadt EE, Franke L, Hingorani AD, Wallace C, et al. Bayesian Test for colocalisation between pairs of genetic association studies using summary statistics. PLoS Genet. (2014) 10(5):e1004383. doi: 10.1371/journal.pgen.1004383
22. Foley CN, Staley JR, Breen PG, Sun BB, Kirk PD, Burgess S, et al. A fast and efficient colocalization algorithm for identifying shared genetic risk factors across multiple traits. Nat Commun. (2021) 12:764. doi: 10.1038/s41467-020-20885-8
23. Chong J, Soufan O, Li C, Caraus I, Li S, Bourque G, et al. Metaboanalyst 4.0: towards more transparent and integrative metabolomics analysis. Nucleic Acids Res. (2018) 46:W486–94. doi: 10.1093/nar/gky310
24. McKelvey R, Berta T, Old E, Ji R-R, Fitzgerald M. Neuropathic pain is constitutively suppressed in early life by anti-inflammatory neuroimmune regulation. J Neurosci. (2015) 35:457–66. doi: 10.1523/JNEUROSCI.2315-14.2015
25. Zimmermann M. Pathobiology of neuropathic pain. Eur J Pharmacol. (2001) 429:23–37. doi: 10.1016/S0014-2999(01)01303-6
26. Devor M, Jänig W, Michaelis M. Modulation of activity in dorsal root ganglion neurons by sympathetic activation in nerve-injured rats. J Neurophysiol. (1994) 71:38–47. doi: 10.1152/jn.1994.71.1.38
27. Woolf CJ, Salter MW. Neuronal plasticity: increasing the gain in pain. Science. (2000) 288:1765–9. doi: 10.1126/science.288.5472.1765
28. Karim F, Wang CC, Gereau RW. Metabotropic glutamate receptor subtypes 1 and 5 are activators of extracellular signal-regulated kinase signaling required for inflammatory pain in mice. J Neurosci. (2001) 21:3771–9. doi: 10.1523/JNEUROSCI.21-11-03771.2001
29. Yan L-p, Wu X-t, Yin Z-y, Ma C. [Effect of electroacupuncture on the levels of amino acid neurotransmitters in the spinal cord in rats with chronic constrictive injury]. Zhen Ci Yan Jiu. (2011) 36(5):0.
30. Chiechio S, Nicoletti F. Metabotropic glutamate receptors and the control of chronic pain. Curr Opin Pharmacol. (2012) 12:28–34. doi: 10.1016/j.coph.2011.10.010
31. Ma S, Zheng X, Zheng T, Huang F, Jiang J, Luo H, et al. Amitriptyline influences the mechanical withdrawal threshold in bone cancer pain rats by regulating glutamate transporter GLAST. Mol Pain. (2019) 15(0). doi: 10.1177/1744806919855834
32. Ma C, Li C-x, Yi J-l, Yan L-p. [Effects of electroacupuncture on glutamate and aspartic acid contents in the dorsal root ganglion and spinal cord in rats with neuropathic pain]. Zhen Ci Yan Jiu. (2008) 33(4):0.
33. Kobayashi S, Mwaka ES, Baba H, Kokubo Y, Yayama T, Kubota M, et al. Microvascular system of the lumbar dorsal root ganglia in rats. Part II: neurogenic control of intraganglionic blood flow. J Neurosurg Spine. (2010) 12:203–9. doi: 10.3171/2009.8.SPINE08895
34. Watson CJ. Insular balance of glutamatergic and GABAergic signaling modulates pain processing. Pain. (2016) 157:2194–207. doi: 10.1097/j.pain.0000000000000615
35. Yang C-P, Cherng C-H, Wu C-T, Huang H-Y, Tao P-L, Lee S-O, et al. Intrathecal ultra-low dose naloxone enhances the antihyperalgesic effects of morphine and attenuates tumor necrosis factor-α and tumor necrosis factor-α receptor 1 expression in the dorsal horn of rats with partial sciatic nerve transection. Anesth Analg. (2013) 117:1493–502. doi: 10.1213/ANE.0000000000000020
36. Wang Z, Wu H, Chen Y, Chen H, Wang X, Yuan W. Lactobacillus paracasei S16 alleviates lumbar disc herniation by modulating inflammation response and gut microbiota. Front Nutr. (2021) 8:701644. doi: 10.3389/fnut.2021.701644
37. Patte-Mensah C, Meyer L, Taleb O, Mensah-Nyagan AG. Potential role of allopregnanolone for a safe and effective therapy of neuropathic pain. Prog Neurobiol. (2014) 113:70–8. doi: 10.1016/j.pneurobio.2013.07.004
Keywords: lower back pain, sciatica, Mendelian randomization, serum metabolites, single nucleotide polymorphisms
Citation: Ren Y-M, Hou W-Y, Fan B-Y, Duan Y-H, Sun Y-B, Yang T, Zhang H-J, Sun T-W and Tian M-Q (2024) Causality of genetically determined serum metabolites on lower back pain or/and sciatica: a comprehensive Mendelian randomized study. Front. Pain Res. 5:1370704. doi: 10.3389/fpain.2024.1370704
Received: 20 January 2024; Accepted: 12 September 2024;
Published: 25 September 2024.
Edited by:
John Michael Streicher, University of Arizona, United StatesReviewed by:
Changyu Jiang, Duke University, United StatesDayu Pan, Johns Hopkins University, United States
Copyright: © 2024 Ren, Hou, Fan, Duan, Sun, Yang, Zhang, Sun and Tian. This is an open-access article distributed under the terms of the Creative Commons Attribution License (CC BY). The use, distribution or reproduction in other forums is permitted, provided the original author(s) and the copyright owner(s) are credited and that the original publication in this journal is cited, in accordance with accepted academic practice. No use, distribution or reproduction is permitted which does not comply with these terms.
*Correspondence: Bao-You Fan, YmFveW91MTAzMEAxNjMuY29t; Tian-Wei Sun, c3R3dW1jQDE2My5jb20=
†These authors have contributed equally to this work