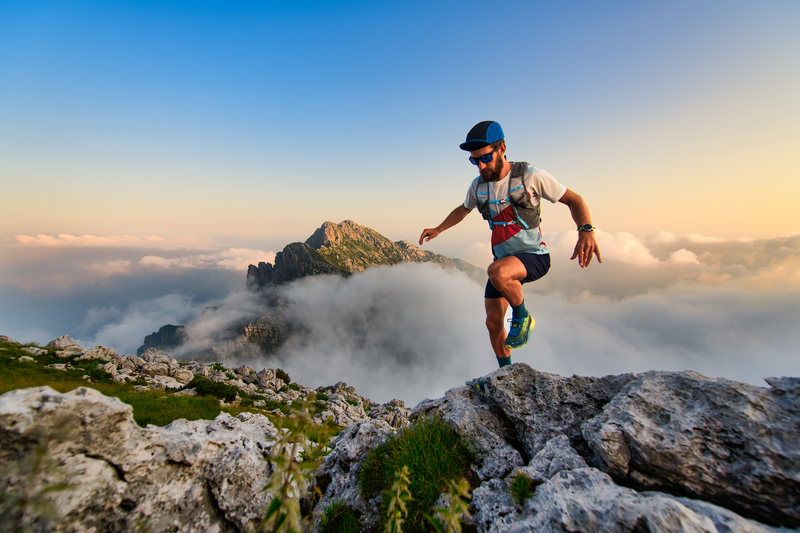
94% of researchers rate our articles as excellent or good
Learn more about the work of our research integrity team to safeguard the quality of each article we publish.
Find out more
EDITORIAL article
Front. Pain Res. , 19 October 2023
Sec. Pain Research Methods
Volume 4 - 2023 | https://doi.org/10.3389/fpain.2023.1298882
This article is part of the Research Topic Computational Methods for Pain Pharmacology View all 5 articles
Editorial on the Research Topic
Computational methods for pain pharmacology
As we venture into the year 2024, it becomes clear that we stand in front of a profound technological revolution. Recent developments in the field of data science and artificial intelligence have now made them apparent parts of our daily life. Advanced technologies are not only reshaping our daily experiences but also revolutionizing how we approach intricate challenges like pain management. The complexity of pain perception, the diversity of underlying mechanisms, and the necessity for tailored pharmacological interventions have fueled a growing interest in employing computational methods to enhance our understanding and treatment of pain.
Two articles in this Research Topic leveraged so-called “big data” to tackle clinically relevant problems. Holmes et al., have used different machine learning (ML) classifiers to differentiate pediatric and young adult subjects with post-traumatic headache (PTH) from healthy controls. Authors used data from different self- or caregiver-report questionnaires that reflect concussion symptoms, mental health, pain experience of the participants, as well as brain imaging data including volume measures of cortical and sub-cortical structures. Abovementioned data allowed researchers to predict PTH diagnosis with 85% accuracy, but more interestingly, they were able to establish the score in Child and Parent Fear of Pain Questionnaire to be the most important predictor of PTH, followed by changes in volumes of several brain areas, implicating their role in the neurobiological mechanism involved in the PTH development. These results highlight how much insight, one can get from the data by using computational tools, such as feature selection algorithms. Similarly, Lai et al., used an a priori algorithm to reveal the use patterns of Traditional Chinese Medicine (TCMs) as analgesic drugs in rheumatoid arthritis patients. This study had screened out the potential compatibility combination patterns of drugs for the treatment of rheumatoid arthritis and also set a perfect example of how the use of computational tools can bring better understanding of complex and vague phenomena such as the pharmacology of TCMs and the synergistic effects between various herbs.
This Research Topic also demonstrates how computational approaches are driving the development of personalized pain management strategies. By integrating patient-specific data, such as genetic profiles, physiological parameters, and pain phenotypes, computational models can assist clinicians in selecting the most suitable medications and dosages, optimizing outcomes, and minimizing adverse effects. Van der Wal et al., have employed nociception level monitor (NOL) during operation to dose the analgesics. NOL, based on random forest algorithm, constructed pain score from the following parameters: heart rate, heart rate variability amplitude of the finger photoplethysmogram, skin conductance level and their time derivatives. Whenever the patient reached a threshold pain score, they were given an analgesic. This method was compared with standard procedure, where analgesics are dosed based on hemodynamic response. Authors observed that in the NOL-guided group, fewer patients experienced severe pain after operation and less patients required post-operative pain treatment. Thus, application of NOL or other ML based algorithms for dose optimization may significantly reduce risk of opioid overuse.
Finally, a great proportion of computational work focuses on creating functional models of biological phenomena. Neilan et al., have developed a 3-D computational model of neurons in the central amygdala (CeA) based on the laboratory data. CeA is a heterogenous nucleus including dozens of unique cell types with different functions based on intra-CeA connectivity and localization along the anterior-posterior axis. Generally, neurons expressing protein kinase C-delta (PKCδ) have a pro-nociceptive role in CeA “pain output”, while somatostatin (SST) positive CeA neurons have an anti-nociceptive role. However, there is considerable evidence for expression differences in PKCδ and SST as well as other CeA cell-types based on their location within the nucleus. Nociceptive inputs to these cells differ as well. Authors developed the first computational model of PKCδ and SST neurons in the amygdala, based on cell-type physiology data. Their model simulates the behaviors and interactions of individual PKCδ and SST neurons and is capable of capturing morphological features as well as spatial heterogeneity in the location and connectivity of PKCδ and SST neurons throughout the CeA. One of the advantages of the 3-D model is its ability to target cells in different locations within the CeA to investigate the potential for spatially separate populations to differently impact nociception. The value of computational modeling is its ability to address these questions quickly prior to investment in resource-heavy wet lab experiments. Based on the simulations, authors suggest that manipulation of the anterior vs. posterior CeA causes a disproportional impact on nociceptive output from two populations of CeA neurons, a phenomenon recently demonstrated in mice (1).
In conclusion, the research topic “Computational Methods for Pain Pharmacology” underscores the transformative role of computational methods in revolutionizing pain management and research. From unraveling the intricacies of pain neurobiology to accelerating drug discovery and enabling personalized treatments, computational approaches are pushing the boundaries of our understanding and capabilities. As technology continues to evolve and interdisciplinary collaborations flourish, the future holds the promise of more effective, safer, and patient-centric pain relief strategies.
JM: Writing – original draft. MK: Writing – review & editing.
JM was supported by Polish National Agency of Academic Exchange BPN/BEK/2021/1/00152 and SciLifeLab RED fellowship scholarship. MK is supported by Medical Research Council (UK) NIRG grant (MR/W004739/1) held by Kirsty Bannister and Polish National Science Centre Miniatura grant (2022/06/X/NZ5/01381) held by MK.
The authors declare that the research was conducted in the absence of any commercial or financial relationships that could be construed as a potential conflict of interest.
All claims expressed in this article are solely those of the authors and do not necessarily represent those of their affiliated organizations, or those of the publisher, the editors and the reviewers. Any product that may be evaluated in this article, or claim that may be made by its manufacturer, is not guaranteed or endorsed by the publisher.
Keywords: computational methods, pain, machine learning, artificial intelligence, big data, data mining
Citation: Mlost J and Kucharczyk M (2023) Editorial: Computational methods for pain pharmacology. Front. Pain Res. 4:1298882. doi: 10.3389/fpain.2023.1298882
Received: 22 September 2023; Accepted: 11 October 2023;
Published: 19 October 2023.
Edited and Reviewed by: Yue Lupeng, Chinese Academy of Sciences (CAS), China
© 2023 Mlost and Kucharczyk. This is an open-access article distributed under the terms of the Creative Commons Attribution License (CC BY). The use, distribution or reproduction in other forums is permitted, provided the original author(s) and the copyright owner(s) are credited and that the original publication in this journal is cited, in accordance with accepted academic practice. No use, distribution or reproduction is permitted which does not comply with these terms.
*Correspondence: Jakub Mlost amFrdWIubWxvc3RAc2NpbGlmZWxhYi5zZQ==
Disclaimer: All claims expressed in this article are solely those of the authors and do not necessarily represent those of their affiliated organizations, or those of the publisher, the editors and the reviewers. Any product that may be evaluated in this article or claim that may be made by its manufacturer is not guaranteed or endorsed by the publisher.
Research integrity at Frontiers
Learn more about the work of our research integrity team to safeguard the quality of each article we publish.