- 1Departments of Neurology and Anesthesia and Perioperative Care (Pain Management), University of California, San Francisco, San Francisco, CA, United States
- 2Department of Neurological Surgery, University of California, San Francisco, San Francisco, CA, United States
- 3Department of Psychology, University of California, Berkeley, Berkeley, CA, United States
Precision neuromodulation of central brain circuits is a promising emerging therapeutic modality for a variety of neuropsychiatric disorders. Reliably identifying in whom, where, and in what context to provide brain stimulation for optimal pain relief are fundamental challenges limiting the widespread implementation of central neuromodulation treatments for chronic pain. Current approaches to brain stimulation target empirically derived regions of interest to the disorder or targets with strong connections to these regions. However, complex, multidimensional experiences like chronic pain are more closely linked to patterns of coordinated activity across distributed large-scale functional networks. Recent advances in precision network neuroscience indicate that these networks are highly variable in their neuroanatomical organization across individuals. Here we review accumulating evidence that variable central representations of pain will likely pose a major barrier to implementation of population-derived analgesic brain stimulation targets. We propose network-level estimates as a more valid, robust, and reliable way to stratify personalized candidate regions. Finally, we review key background, methods, and implications for developing network topology-informed brain stimulation targets for chronic pain.
1. Introduction
The Centers for Disease Control and Prevention (CDC) estimates that chronic pain affects more people in the United States than heart disease, diabetes and cancer combined. Approximately 20% of the United States adult population suffers with chronic pain, with more than 19 million experiencing functionally disabling “high impact” chronic pain that limits life, work and social activity (1). Patients with chronic pain have highly variable and often inadequate responses to treatment, leading to trial-and-error based interventions that delay relief and increase reliance on potentially addictive opioid analgesics. Beginning in the 1950s, an emerging understanding of the role of specific brain regions in pain transmission and modulation motivated trials of deep brain stimulation (DBS) for severe and otherwise refractory pain conditions of various etiologies. More recently, preclinical pain research and neural circuitry models of pain processing in humans have substantially broadened the range of viable empirically-defined targets that may be used to treat chronic pain (2, 3). Although DBS of a variety of specific brain regions can dramatically reduce pain and improve quality of life for a subset of patients, therapeutic responses to stimulation are highly variable and there are currently no reliable methods for determining optimal stimulation parameters and locations for individual patients. The emergence of clinical transcranial magnetic stimulation (TMS) in the 1990s and more recent development of MRI-guided low-intensity focused ultrasound (LI-FUS) offer promising non-invasive alternatives to DBS (4–7). These new approaches promise to improve the safety and accessibility of therapeutic brain stimulation for pain, but face similar challenges in identifying in whom, where, and how to stimulate to achieve optimal pain relief.
Dysfunction in distributed brain networks is increasingly recognized as central to the pathophysiology of a variety of neuropsychiatric disorders and approaches to modulate these networks are now actively investigated as novel therapies (8–12). Across disorders, therapeutic targets are generally established anatomical regions informed by prior DBS and/or preclinical models, or regions identified through population-level comparisons between patients with the disorder and healthy adult “control” cohorts (8, 11–14). Although improving the precision of stimulation by targeting specific neuroanatomical regions that are abnormally activated or connected at the population level has been linked to improved treatment outcomes for a variety of disorders, there is more limited evidence that such an approach can be prospectively applied to individual patients (9, 15–17). In fact, there is accumulating evidence across neuropsychiatric disorders that variability in clinical response to neuromodulation is directly related to variability in the topology (i.e., the spatial organization and arrangement of network connections) of underlying brain networks (18–21).
Advances in precision neuroscience have recently enabled the estimation of reliable individual brain networks by repeatedly scanning research participants (22–25). Studies of these highly sampled individuals show that networks exhibit substantial interindividual variability and that individual networks meaningfully diverge from population average estimates (26–31). Moreover, neural responses to both acute experimental pain and spontaneous fluctuations in chronic pain are highly variable across individuals (32–34). Indeed, population-level brain imaging studies have largely failed to capture the complex and uniquely individual aspects of pain. This failure poses a considerable translational barrier to the development of network-informed biomarkers that can be used guide personalized neuromodulation for chronic pain (35, 36).
Effective pain management demands a personalized approach. We propose that a precision neuromodulation approach to network-informed target selection will be more robust to individual differences in brain network topologies than current one-target-fits-all approaches. Here, we review the neuroanatomical basis of pain in the context of stimulation for clinical pain relief and critique existing methods used to identify optimal targets for therapeutic neuromodulation. We conclude with a proposed biomarker development strategy motivated by recent advances in network neuroscience and graph theory that may improve personalized stimulation for pain and other neuropsychiatric disorders.
2. Review of pain circuits
Research across species indicates that pain is represented by a distributed network of brain regions involved in different aspects of pain processing, including ascending pain transmission circuits and descending opioidergic pain modulation circuits (32, 37–43). Early attempts to identify a single locus of pain perception in humans converged on the thalamus, as neither focal lesions nor stimulation of cortex could reliably modify painful sensations (44, 45) [but see (46, 47)]. Gate Control Theory (48) offered a mechanistic explanation for variability in pain perception as a dynamic central processes that exerts top-down influence over the patterned input of spinal pain transmission pathways. Gate control theory also began to highlight the paramount importance of motivational and affective features of the pain experience (49). This emerging understanding of pain as a multidimensional psychological construct was later formalized by Melzack as the Neuromatrix, subsequently “Pain Matrix”, a widely distributed neural network of interconnected somatosensory, limbic, and thalamocortical structures subserving parallel processing of sensory-discriminative, affective-motivational, and cognitive-evaluative domains of the pain experience (50, 51). In contrast to the largely modulatory role ascribed to the brain in Gate Control Theory, the Neuromatrix is foundational to our current understanding of pain as a complex experience emergent from patterned activity across a distributed network of brain regions (52).
Modern accounts of the Pain Matrix predict that sensory, affective, and cognitive domains of pain processing are represented by a specific subset of established “pain relevant” brain regions (51, 53, 54) (Figure 1A). In this reductionist view, sensory-discriminative regions include the sensory (i.e., ventral posterolateral and ventral posteromedial) thalamus, somatosensory cortex (S1/S2), and posterior insula (pIns). Affective-motivational regions include the amygdala, ventral striatum (VS), anterior insula (aIns), and anterior cingulate cortex (ACC). Cognitive-evaluative regions include the hippocampus, orbitofrontal cortex (OFC), and dorsolateral prefrontal cortex (dlPFC). Subcortical structures like the sensory thalamus and periaqueductal gray (PAG) with extensive reciprocal connections to distributed cortical regions and downstream outputs that are known to modulate spinal nociceptive transmission are included in most models as key ascending transmission and descending modulation hubs, respectively. Below, we review neuroimaging evidence for and against the involvement of specific brain regions and networks in pain before considering implications for clinical neuromodulation.
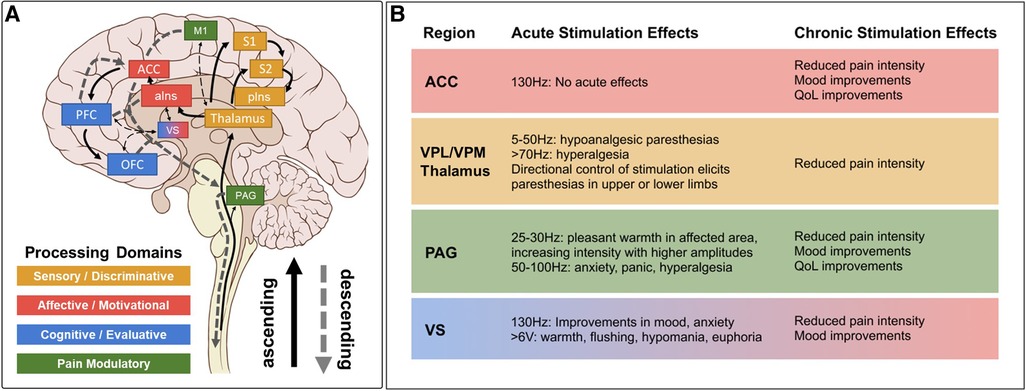
Figure 1. Pain matrix regions grouped by processing domain* with summarized DBS effects. (A) Brain regions implicated in different domains of pain perception superimposed on ascending pain transmission and descending pain modulation pathways. Several implicated brain regions without stimulation data in chronic pain (e.g., amygdala, hippocampus) are excluded for clarity, as are many connections between regions. Solid arrows indicate ascending projections. Dashed arrows indicate descending projections. (B) Summary of acute and chronic effects of stimulation at established DBS targets, color coded by processing domain as in A, with notes regarding specific parameter effects. Stimulation effects on relevant domains are summarized from references cited in Tables 1, 2. Clinical efficacy summaries can be found in Table 3. PAG, periaqueductal gray; S1, primary somatosensory cortex; S2, secondary somatosensory cortex; pIns, posterior insula; M1, primary motor cortex; aIns, anterior insula; ACC, anterior cingulate cortex; VS, ventral striatum; PFC, prefrontal cortex; OFC, orbitofrontal cortex; VPL, ventral posterolateral; VPM, ventral posteromedial; QoL, quality of life. *Note that we operationally define the term “domain” as a collection of related psychological processes or functions (e.g., somatosensation, emotion, cognition, memory, etc.) for consistency with the psychology and cognitive neuroscience literature (cited from Shirvalkar et al., (3)).
2.1. Evidence for the involvement of specific brain regions in pain processing
A large body of predominantly correlative brain imaging literature in humans has been used to link activity in specific brain regions with different domains of pain processing (39, 43, 55–57). In vivo imaging of brain-wide blood oxygen level dependent (BOLD) signals with functional magnetic resonance imaging (fMRI) is the most widely used method for mapping brain activation during complex behaviors (43, 58, 59). The BOLD signal is known to reflect underlying local field potentials (LFP), which in turn correspond to stimulus-induced oscillations in the range of 30–150 Hz (60). In general, BOLD responses to painful experimental stimuli in Pain Matrix regions are reliably correlated with perceived pain intensity and consistently modulated by contextual factors that impact self-reported pain (42, 53, 54, 61–63). A subset of studies highlight that behavioral manipulations targeting specific (e.g., sensory, affective, and cognitive) domains of pain processing elicit predictable changes in associated brain regions (38, 64–66). For example, ACC activity is selectively reduced by hypnotic suggestion to reduce pain unpleasantness, consistent with the proposed role of ACC in the affective domain of pain perception (67). Studies of placebo and opioid analgesia suggest that frontal regions implicated in affective and cognitive processing domains, including the ACC, dlPFC, and OFC, are also well suited to modulate activity across the network, potentially through top-down recruitment of antinociceptive circuits in the PAG (40, 61, 63, 68–71).
Some argue that the evolution in terminology from “Neuromatrix” to “Pain Matrix” is inappropriate, as most included brain regions support domain-general, rather than pain-specific, processing (50). For example, salience-matched painful and nonpainful sensory stimuli elicit largely indistinguishable patterns of network activity, which challenges the specificity of observed BOLD activations to pain (72, 73). Moreover, many regions reliably implicated in pain processing are vulnerable to a reverse inference problem, in which a specific mental state (e.g., pain) is inferred from observed patterns of brain activation (e.g., thalamus, S1) (74, 75). Indeed, pain-relevant regions like the dorsal anterior cingulate cortex (dACC) and anterior insula (aIns) are the most commonly activated brain regions across the fMRI literature, regardless of the condition or process studied (76–78). For example, dACC is implicated in a range of specific functions ranging from attentional control and language processing to emotional expression and learning, which, although pain relevant, are not pain-specific (79, 80). In the context of pain, dACC activity is commonly cited as a proxy of pain aversiveness, yet a substantial number of studies have demonstrated that increased dACC activity is likely anti-nociceptive through top-down connections with the PAG (40, 68, 69, 81–86). Furthermore, most contemporary circuit models of pain omit regions where pain-related BOLD activations are often seen, such as the cerebellum, and where stimulation can profoundly alter pain perception, notably the primary motor cortex (M1) (87, 88). Thus, simple structure-to-function mapping is fraught with potential biases in interpretation, which challenge links between specific regional dysfunction and processing domains commonly disrupted in chronic pain (89, 90).
More recent advances in fMRI analysis and machine learning have enabled the development and characterization of the so-called “Neural Pain Signature” (NPS). The NPS is a weighted combination of multivariate patterned BOLD activity across pain-relevant brain regions that can accurately discriminate between painful heat and non-painful warmth or social rejection (91). The discriminative performance of the NPS offers proof of principle that activity across distributed networks is more closely linked to the subjective experience of pain than activity in individual regions (91, 92). The primacy of networks to pain processing is further supported by the observation that stimulation of individual brain regions rarely elicits pain and lesions to these regions seldom lead to specific changes in pain processing (50). Together, these observations have motivated a gradual transition away from focusing on regional dysfunction in specific structures and toward evaluating brain-wide network-level dysfunction across interconnected and functionally related brain regions.
2.2. Functional brain networks involved in chronic pain
Network analysis with resting state fMRI (rs-fMRI) has characterized a variety of intrinsic resting state networks (RSNs) defined by shared temporal fluctuations in spontaneous BOLD activity among interconnected brain regions. RSNs are believed to reflect the intrinsic functional architecture of underlying brain circuits (93) and correspond with established processing domains with relevance to pain perception (38, 50, 94). RSNs are typically estimated using normalized univariate Pearson correlation coefficients of the BOLD signal during periods of unconstrained (i.e., task-free) rest, known as resting state functional connectivity (rsFC) (95, 96). Functional networks estimated using rsFC correspond with structural networks defined by white matter pathways (97, 98) and with patterns of coactivation observed while performing relevant cognitive tasks (99–102). Further, the low frequency (<0.1 Hz) BOLD signals used to estimate rsFC have a similar spatial correlation structure to intracranial low frequency (<4 Hz) and γ-range (40–100 Hz) power (103, 104). BOLD correlations also predict the spread of intracranial evoked activations (105), illustrating their correspondence to underlying anatomical and physiologic network architecture. Importantly, RSNs are linked to the pathophysiology of a variety of neuropsychiatric disorders (10, 20) and modulation of specific RSNs with stimulation has been linked to improvements in corresponding symptom domains (106).
Several canonical RSNs have been directly implicated in the pathogenesis of pain, including a “salience network” (aIns, dACC, temporo-parietal junction, and dlPFC) thought to be involved in externally directed attention toward exogenous painful stimuli, a “default mode” network (DMN; posterior cingulate/precuneus, medial prefrontal cortex, and lateral parietal lobe) putatively involved in mind wandering away from pain, and a pain-specific descending modulatory network defined based on rsFC with PAG, among many others (100, 101, 107). A leading network model of chronic pain predicts that structural pathology within and between hubs of defined RSNs are associated with trait-level risk of developing chronic pain. Specifically, impairments in DMN organization and connectivity with the antinociceptive system are thought to enhance implicit attention to pain (94, 108). However, DMN pathology is not specific to chronic pain. Rather, DMN dysfunction is a commonly cited network correlate of a range of typically comorbid disorders characterized by rumination and introspection (109–111). Thus, just as individual brain regions, including the dACC, are implicated in divergent pain-relevant functions (e.g., enhancing pain aversiveness and recruiting antinociceptive circuits), DMN is routinely implicated in both pain-protective processes (e.g., distraction) and in ruminative and self-referential processes that are thought to increase the risk for developing chronic pain (110, 112–114).
2.3. Relevance of circuit models to chronic pain
Although contemporary circuit models of pain processing clearly suffer from a lack specificity to pain, more concerning for their translational potential to brain-based therapeutics is the consistent failure of models derived from studies of acute experimental pain to generalize to chronic pain. Most prior research has relied on studies of evoked brain activity during noxious stimulation in healthy volunteers, which may lack validity in the chronic pain state (43, 115). Whereas the NPS can predict acute experimental pain with >90% sensitivity and specificity, the same algorithm performs poorly when applied to natural fluctuations in chronic pain, which are more closely linked to patterns of rsFC observed during prolonged tonic pain (91, 116). Prior work directly comparing responses to acute experimental pain between healthy adults and chronic pain populations highlight consistent involvement of similar key brain regions (i.e., the “Pain Matrix”) in both acute and chronic pain, but more recent large-scale meta-analyses failed to identify any coherent patterns of regional dysfunction that reliably distinguish patients with chronic pain from healthy control subjects (117, 118). Other studies that examined spontaneous fluctuations of clinical pain suggest that recruitment of separate brain regions involved in reward and stimulus valuation (e.g., nucleus accumbens and medial prefrontal cortex) may be unique to the chronic pain state and even predict the likelihood of transitioning from subacute to chronic pain (119–123). Apkarian and colleagues have interpreted this pattern of central changes in chronic pain as evidence for two central hypotheses: that chronic pain is associated with (1) plastic reorganization of circuits that fundamentally alters the central processing of pain and enriches the sensory experience with emotional and cognitive links, and (2) a transition in the locus of pain from an external threat to a highly salient internalized disease state (115).
2.4. Summary
Theoretical accounts linking patterns of neural activity with the experience of pain conclude that understanding pain networks in terms of nociceptive processing alone fails to address how sensorimotor aspects of pain are enriched with cognitive and affective features in the chronic pain state (38, 43, 64, 94, 115). Models based on the “Pain Matrix” offer compelling interpretations for observed patterns of brain activity and their modulation by contextual variables (124). However, these models fall short when translating insights from acute experimental pain to circuit-level dysfunction in chronic pain. With respect to analgesic brain stimulation, these models suggest several testable hypotheses about which brain regions should be targeted and through which domains brain stimulation may be effective. Combining network analysis with targeted brain stimulation has great potential to enrich correlative human brain imaging data with causal evidence (8, 14, 125). In subsequent sections, we integrate prior experience with brain stimulation for pain with the region and network-level hypotheses summarized below. Because stimulation can variably inhibit or excite brain activity, and because mechanisms underlying such tissue effects are poorly understood, we offer hypotheses for both phenomena (126, 127).
2.5. Testable Hypotheses
I. If brain stimulation inhibits local activity and creates a virtual lesion (127), then stimulation of loci within defined pain transmission circuits (e.g., thalamus and somatosensory cortex) should inhibit pain.
II. Excitatory brain stimulation that activates brain loci associated with pain suppression, especially opioidergic PAG and regions with known connections to PAG (41), should inhibit pain.
III. Stimulation of loci from circuits linked to functionally distinct processing domains should have separable effects on the sensory, emotional, and cognitive aspects of pain.
IV. Network dysfunction models of chronic pain (13, 38, 94, 115, 128) predict that stimulation at various loci within a network should elicit similar pain outcomes.
3. Prior brain stimulation for chronic pain identifies multiple viable targets and mechanisms for pain relief
Various neuromodulation strategies are described for brain-based treatment of neuropsychiatric disorders and chronic pain. Deep brain stimulation (DBS) involves neurosurgical implantation of intracranial electrodes for direct electrical stimulation of specific brain regions and is currently FDA approved to treat movement disorders, epilepsy, and obsessive-compulsive disorder (OCD, through humanitarian exemption), with additional evidence of benefit in major depressive disorder (MDD) and chronic pain (129–132). While fundamental mechanisms of action of DBS for pain are not clearly defined, it is postulated that stimulation could modulate neural activity via a temporary lesion effect or through direct excitation of surrounding structures (126, 127). Transcranial magnetic stimulation (TMS) is a more recently developed non-invasive neuromodulation technique that utilizes magnetic fields to induce electrical currents beneath the skull (133). TMS can excite or inhibit superficial cortical structures depending on the frequency of stimulation (134–136). High frequency (i.e., >5 Hz) excitatory repetitive TMS (rTMS) is currently FDA-approved as a treatment for MDD at left dlPFC (137) and for OCD at dorsomedial PFC/ACC (138). There is accumulating evidence that high frequency rTMS at primary motor cortex (M1) can be effective for chronic neuropathic pain in select patients (6, 7, 139). MRI-guided low-intensity focused ultrasound (MRgFUS or LI-FUS) is an emerging form of non-invasive neuromodulation that is capable of precisely modulating brain activity in deep subcortical regions that are out of reach of conventional TMS probes (4, 5, 140, 141). Additional stimulation modalities such as transcranial direct current stimulation (tDCS) are described for chronic pain but are excluded from the present targeting discussion considering their more limited spatial precision.
3.1. Periaqueductal gray (PAG) and periventricular gray (PVG)
PAG is a midbrain nucleus that extends along the cerebral aqueduct from the locus coeruleus to the posterior commissure. PVG is the diencephalic extension of PAG rostral to the posterior commissure. Whereas PVG was the putative stimulation target in most early DBS trials, most preclinical antinociception literature focuses on PAG. Limited post-mortem evaluations found that similar analgesic responses could be obtained from stimulation in both regions, even within a single patient, and that final electrode locations often differed slightly from planned trajectories (i.e., PVG directed contacts within PAG, and vice versa) (142). We therefore combine our discussion of both regions in this section. PAG was the first brain region to be implicated in endogenous pain modulation and has subsequently been linked to additional homeostatic functions including autonomic control, aversive learning, and coordination of defensive escape/avoidance behaviors (143–151). A substantial body of preclinical evidence convincingly shows that PAG stimulation is antinociceptive (41, 83, 143, 144, 149, 152). Small case series of PAG/PVG DBS for chronic pain reported variable response rates, which may reflect the broad range of stereotactic hardware, targeting coordinates, stimulation parameters, and follow-up times in prior studies (Tables 1, 2). Stereotactic implantation of DBS electrodes is typically performed contralateral to pain, targeting the level of the superior colliculus 2–3 mm lateral to the third ventricle, corresponding with the expected location of the PVG (Table 2). In most prior studies, final electrode position is informed by intraoperative awake macrostimulation using a range of parameters titrated to analgesic effect (172, 178, 179). Subjectively, stimulation of the PAG/PVG elicits a warm sensation that displaces pain in the affected area, a response that has been used to guide final electrode position (160, 180). Other studies have used objective neurological signs such as head bobbing or ocular deviation for target determination (173, 177). Prior studies that reported frequency-dependent effects during macrostimulation found that lower frequency stimulation between 5 and 50 Hz elicited analgesia or pleasant paresthesia, whereas larger stimulation amplitudes and higher frequencies >50 Hz were associated with adverse experiences such as hyperalgesia or anxiety (Figure 1B) (177, 179). Although preclinical work strongly suggests that PAG-mediated analgesia is opioid dependent, clinical experience with PAG/PVG DBS suggests that stimulation may produce analgesia through both opioidergic and non-opioidergic mechanisms (186, 213, 214). Notably, PAG/PVG stimulation has also been reported to improve neuropsychiatric outcomes like mood, anxiety, and quality of life (176). Despite the use of intraoperative macrostimulation to elicit favorable prognostic symptoms and signs (which may account for structure-function variability across patients), stimulation of PAG/PVG nonetheless elicits highly variable and often incomplete analgesia (Table 3). The dissociable pain relieving and anxiogenic effects of low and high frequency acute stimulation are suggestive of distinct activation of antinociceptive vs escape/avoidance circuitry at different frequencies. To our knowledge, this has not been shown in humans. There may be alternative explanations (e.g., variability of local neuronal populations along the dorsoventral and rostrocaudal PAG/PVG axes) that are difficult to evaluate without the benefit of precise coordinates or detailed post-operative imaging from prior studies [but see (142)]. Taken together, the frequency dependence of analgesic PAG/PVG stimulation supports Hypothesis II, which together with widespread effects on sensory, affective, and cognitive pain processing domains suggests that PAG stimulation either enhances pain suppression or exerts widespread network effects.
3.2. Sensory thalamus
The sensory thalamus is composed of the ventral posterior lateral nucleus (VPL) and ventral posterior medial nucleus (VPM), which receive somatotopically organized afferent fibers from the spinothalamic tract and project to primary somatosensory cortex, as well as cognitive and limbic association areas (190). The central location of thalamus within pain transmission circuits made it an attractive early candidate for neuromodulation. Thalamic DBS emerged as one of the first viable applications for neuropathic pain in the 1970s but ultimately fell out of favor after two multicenter trials failed to meet efficacy criteria and industry partners largely abandoned their pursuit of FDA approval (189). These trials were subsequently criticized for the inclusion of heterogeneous chronic pain etiologies and lack of appropriate follow-up, which may have contributed to observed inefficacy (3). DBS target determination for thalamic stimulation takes advantage of the known mediodorsal somatotopic organization of the ventroposterior thalamus, such that VPM as the target for facial pain and the VPL for body or limb pain (154). Anatomically, VPM trajectories are typically 2 mm towards the midline and anterior to the posterior commissure relative to the VPL trajectory (Table 2). Several case series report acute analgesic effects from thalamic stimulation when pain is supplanted with paresthesia upon stimulation (Figure 1B). As with prior PAG/PVG DBS studies, this finding is often used to inform the final electrode position (153, 154, 158). However, despite the use of macrostimulation to ensure that stimulation effects localize to the painful anatomical region, personalized targeting alone is insufficient to ensure adequate analgesia (172, 179). Thalamic stimulation is generally ineffective for thalamic and central post-stroke pains, and across pain disorders, response rates and pain relief estimates are comparable to PAG/PVG DBS (Table 3). Adverse effects of stimulation ranged from reports of increased pain to dystonic movements caused by internal capsular stimulation (172, 179). The variability of outcomes with thalamic DBS suggests that stimulating pain transmission circuits, even when confirmed by positive sensory phenomena in the affected region, is insufficient to reliably modulate the overall experience of chronic pain, especially for neuropathic pain resulting from central (i.e., brain) injury (Hypothesis I).
3.3. Anterior cingulate cortex (ACC)
ACC is a heterogeneous midline cortical structure implicated in a range of emotional, cognitive, and motivational functions (79, 215). ACC exhibits a gradient of rsFC along its rostrocaudal axis ranging from affective and evaluative regions like OFC and amygdala anteriorly to action planning regions like frontal eye fields (FEF) and premotor cortex posteriorly (201, 202, 206). ACC DBS extends from prior experience with bilateral stereotactic cingulotomy for refractory pain, which was found to elicit profound and specific improvements in the affective and motivational aspects of chronic pain (216). ACC has more recently gained favor as a means of targeting the burden of suffering largely driven by the affective domain of chronic pain (2, 217). Spooner reported the first case of bilateral ACC DBS targeting an area 20 mm posterior to the anterior tip of the lateral ventricles in the midsection of the gyrus and reported slight improvements in pain visual analog scores (VAS) compared with PAG stimulation in the same patient (165). Intra-operative stimulation at 130 Hz did not yield any apparent sensory phenomena or pain relief (Figure 1B). Since then, most case series stimulating the ACC for neuropathic pain have applied high frequency (130 Hz) stimulation, noting variable initial responses and generalized loss of efficacy at longer follow-up times. Some of these studies have targeted the cingulum white matter bundle instead of overlying cortex (168). Patients receiving ACC DBS across multiple studies describe their pain as less emotionally unpleasant even if sensory/discriminative judgements about pain remain intact (unpublished personal communication to PS). Accordingly, ACC stimulation has been associated with significant improvements in several measures of quality of life and social functioning (167, 168). These findings support the hypothesis that disparate brain circuits may be involved in processing different domains of pain processing (Hypothesis III). Compared with more spatially restricted anatomical structures like PAG and Thalamus, ACC is a much larger and more structurally and functionally heterogeneous region, which may account for the slightly lower response rates and pain reduction effect sizes seen in prior studies (Table 3).
3.4. Ventral striatum (VS)/internal capsule (IC)
DBS of the ventral striatum and anterior limb of the internal capsule was motivated by prior work linking VS with the affective domain of pain processing and by the proven efficacy of VS/IC DBS for other comorbid neuropsychiatric conditions such as OCD and MDD (196, 198, 205). VS dysfunction and connectivity with prefrontal cortex is an established biomarker of pain chronification and is therefore a particularly attractive candidate stimulation location for patients with chronic pain (121–123, 218). However, a recent double-blind randomized sham-controlled trial targeting these regions did not meet its primary endpoint of greater than 50% pain intensity reduction in more than 50% of patients. As with prior ACC DBS, targeting VS/IC did show significant improvement in several outcome measures quantifying the affective dimension of pain (Figure 1B) (163). Results of ACC and VS DBS studies suggest that convergent effects on pain affect can result from stimulating separate areas of a subnetwork involved in affective processing (Hypothesis IV). However, it remains unclear whether patients that respond to stimulation at one site within a critical network would exhibit similar outcomes at the other site within that network, and whether stimulation of both regions simultaneously would have synergistic effects.
3.5. Primary motor cortex (M1)
Motor cortex stimulation (MCS) first emerged as a viable target for chronic pain after the serendipitous discovery that M1 stimulation reduced bursting activity in the ischemic penumbra of a feline thalamic stroke model (219). Despite early clinical success of MCS, pain outcomes are similarly unreliable as with other DBS targets (220–222). In the 1990s, TMS began to reproduce and predict potential benefits of MCS with non-invasive stimulation of M1 contralateral to pain (223–225). M1 continues to be among the most effective invasive and non-invasive stimulation targets for pain (6, 7, 139, 226), but is not yet FDA approved for this indication in the US. Although the exact mechanisms of pain relief from M1 stimulation are unknown, several hypotheses are proposed (88, 220). High-frequency excitatory M1 rTMS combined with brain imaging suggests that M1 stimulation elicits activity in a distributed network of brain regions implicated in pain processing, including: thalamus, PAG, insula, ACC, and the medial PFC region implicated in pain chronification (122, 227, 228). MCS excites PAG in preclinical models (229) and elicits endogenous opioid release in ACC and PAG in humans that is in turn correlated with pain relief (230). Analgesia from M1 TMS, like PAG/PVG DBS (in certain cases), is naloxone reversible (231, 232). There is some evidence that M1 stimulation is best for pain involving the contralateral face and arm and performs less well for lower extremity and widespread pain syndromes, suggesting somatotopic effects (233, 234). Overall, despite its omission from most circuitry models of chronic pain, M1 stimulation activates a network of pain-relevant brain regions that predict clinical improvement in pain scores. Most recently, this same region was shown to be a common network hub linking brain lesions that cause pain, suggesting that M1 may be a network target with diffuse influence over other implicated brain regions (128). Although this is an attractive hypothesis, the limited benefit of M1 for widespread and lower extremity pains argues for a more specific somatotopic mechanism.
3.6. Other targets
A variety of cortical regions have been explored as targets for chronic pain using high frequency rTMS. The left dorsolateral prefrontal cortex (dlPFC) is a neocortical structure involved in higher order cognitive and executive functions and component of the salience RSN. It is an established FDA-approved rTMS target for refractory major depressive disorder (MDD) and has been studied for a range of pain conditions (7, 137). Its direct role in pain processing is unclear, but activity in this region has been linked to selective attention to nociceptive stimuli (42). Meta-analyses of left dlPFC rTMS suggest no reliable benefit for chronic pain, although there is some evidence that left dlPFC may be effective for certain widespread pain conditions like fibromyalgia, mTBI headache, and pain with comorbid MDD (6, 139, 208, 235–237). One recent study evaluated rTMS at the dACC and posterior Insula (pIns) regions implicated in sensory and affective pain processing using a double-cone TMS coil design capable of stimulating deeper cortical structures (238). Although dACC stimulation reduced self-reported anxiety and pIns stimulation modulated experimental pain detection thresholds, neither target was clinically efficacious for chronic pain. This pattern of results offers further support for the assertion that regions implicated in nociceptive processing may not be viable analgesic targets in chronic pain and that modulation of emotional processing alone may not meaningfully improve the experience of pain. However, it is also possible that the relative loss of precision with rTMS at deeper targets may be contributing to the lack of clinical efficacy.
3.7. Multi-target stimulation
If the experience of pain relies on parallel processing in distributed brain networks, multidimensional pain relief may require simultaneous targeting of separate subnetworks. Motivated by earlier findings that thalamic DBS outcomes were better for patients with deafferentation pains (e.g., rhizotomy, spinal cord injury) and PAG/PVG DBS outcomes were better for nociceptive pain (e.g., cancer), Hosobuchi and colleagues hypothesized that simultaneous stimulation of both regions would lead to more comprehensive pain improvements (180). All eleven patients in the cohort reported satisfactory pain relief and continued to use DBS as their primary therapeutic modality at longer follow ups. Although limited by the absence of blinding and control stimulation, these findings suggest that simultaneous targeting of pain transmission and modulation pathways may be more effective than single-target stimulation. Combined acute stimulation of the PAG/PVG and the centromedian parafascicular (CMpf) nucleus of the thalamus, a thalamic nucleus implicated in attention and arousal, was show to relieve pain by an average of 41% within 30 min that lasted 2 h (209). A follow-up study by Hollingworth et al. investigated dual frequency DBS of the PAG/PVG and CMpf, demonstrating 100% response rate with 65.9% average VAS pain intensity reduction in three patients with medically refractory chronic pain at 3 years post implantation (Table 3) (170). While these results have yet to be replicated in larger sample sizes with blinded, randomized, and controlled study designs, they imply that simultaneously targeting regions involved in distinct aspects of pain processing may be more efficacious that single site DBS.
3.8. Summary
Despite the anatomical precision afforded by pain's somatotopic representation, stimulation of targets along defined transmission, central processing, and descending modulation pathways have failed to yield consistent relief across patients. Importantly, although prior case series of DBS have personalized electrode targets for individual patients using objective signs and subjective sensory reports during intra-operative macrostimulation, outcomes across all defined targets remain modest and unreliable (Table 3). Thus, although nociceptive stimuli may enter awareness through defined pathways, the multidimensional experience of pain that drives the burden of suffering may be difficult to capture by stimulating individual regions of interest to pain processing. Our review of prior brain stimulation highlights that while stimulation of individual regions rarely leads to complete relief, different domains of pain processing can be selectively manipulated by stimulating specific regions (e.g., affective domain improvements with ACC and VS stimulation). Importantly, emerging multi-target stimulation data suggest that stimulation of hubs from distinct networks may overcome some of the limitations of stimulating individual brain regions (13, 170, 180). However, even multi-target stimulation of popular brain regions only works for a minority of patients. Overall, prior experience with DBS and TMS for chronic pain supports two key observations: (1) no single brain region is universally efficacious for pain relief, yet (2) convergent relief can be obtained at a variety of stimulation locations. Taken together, these results highlight that a personalized network-informed approach to stimulation that seeks to simultaneously modulate multiple regions or subnetworks involved in distinct aspects of pain processing may lead to more reliable effects on the multi-dimensional experience of pain.
4. Network informed brain stimulation for neuropsychiatric disorders
Before turning to new targeting methods, it is first useful to review established fMRI-guided brain stimulation methods. The gradual development of rTMS targets for major depressive disorder (MDD), beginning with a single dysfunctional left dlPFC region and culminating in the development of rsFC-informed targets, highlights that network-informed stimulation can improve clinical outcomes. The selection of a consensus depression-relevant subgenual ACC (sgACC) region with which to explore rsFC with candidate rTMS targets was ultimately the key first step toward viable personalized neuromodulation for MDD (239). Although PAG and sensorimotor cortex are proposed as viable consensus targets for chronic pain (13, 128), rsFC with these regions has not yet been linked to improved stimulation outcomes. Below we discuss the strengths and weaknesses of existing targeting methods in MDD before proposing a novel approach that replaces a priori defined regions of interest with regions informed by individual network topologies.
4.1. TMS for depression supports network-informed targeting
The FDA-approved left dlPFC target for MDD was initially motivated by prior observations that left dlPFC lesions increase the risk of depression and that metabolism in this region increases with successful antidepressant treatment (136, 240, 241). Variable outcomes across early rTMS studies began to highlight that heterogeneity in clinical response was at least partially driven by variability in the underlying left dlPFC anatomical target, which is among the most structurally and functionally variable brain regions across individuals (27, 29, 242, 243). Subsequent studies sought to improve the consistency of stimulation across patients by targeting a region 5 cm anterior to the location where stimulation elicited a reliable finger twitch (137). Variability in head size motivated yet more precise measurements, guided by the 10–20 electroencephalogram (EEG) montage, to identify a patient-specific left frontal F3 point (244). Although the “Beam-F3 approach” has not been shown to be more clinically efficacious than the 5 cm point in head-to-head trials, it more consistently engages the left dlPFC and is therefore the method currently endorsed for clinical use in MDD by the Clinical TMS society (8, 245). More recent attempts to transition from coarse scalp landmarks to individual coordinates derived from group-level comparisons between MDD and healthy controls have unfortunately not led to any substantive improvement in clinical outcomes (8).
Variability in the specific location of the left dlPFC stimulation target across prior studies ultimately proved fruitful for subsequent mechanistic studies seeking to characterize network parameters associated with favorable clinical outcomes (14, 17, 106, 239, 246). Across prior rTMS trials, rsFC between the stimulated left dlPFC target and a region of sgACC routinely implicated in depression was reliably correlated with improvement in symptoms (125, 247, 248). This key finding motivated more recent attempts to use rsFC with sgACC to prospectively identify the “best” dlPFC region to stimulate for depression (15, 17). However, there are notable caveats to this approach. First, prior studies have compared rsFC estimates within a restricted dlPFC search space. It is therefore unclear whether the dlPFC target identified using rsFC with sgACC is the best treatment location for MDD, or whether there may be alternative brain regions outside of dlPFC where stimulation is more efficacious. Second, correlations between clinical outcomes and rsFC in most prior studies were generated using a large normative sample of healthy adults without depression, from a standardized open-source “connectome” dataset (58, 239, 249). In other words, although coordinates used to generate rsFC estimates were derived from individual patients, the resulting rsFC parameter for each set of coordinates was estimated using a separate large, unaffected sample group.
The use of large normative samples to explore network properties of neuromodulation targets is motivated by limitations of the fMRI BOLD signal, which is characterized by low signal-to-noise ratio (SNR), poor signal coverage in a variety of structures of interest (especially orbitofrontal cortex and inferior temporal lobes), and low temporal resolution (1.5–2 s in most studies), which together pose significant barriers to generating reliable and valid estimates for individual patients (58, 59). Averaging across large samples improves statistical power, which is linked to more stable parameter estimates but assumes homogeneous structure-function relationships across patients (250–252). Using large normative datasets to generate rsFC estimates has dramatically advanced our understanding of the network-level correlates of behavior and yielded powerful new methods to understand a variety of neuropsychiatric conditions that may be better understood as “circuitopathies” (241, 249, 253, 254). However, the same group that first identified anticorrelation with sgACC as a predictor of left dlPFC rTMS efficacy also showed that the estimated dlPFC target derived from individual subject data diverged from the group average prediction (255). Although subsequent studies testing the generalizability of estimates derived from the normative connectome highlight that datasets from affected clinical populations yield similar estimates (17, 106, 128, 254) and that the direction and magnitude of sgACC rsFC estimates derived from individual subject data are similar to population estimates (15), it remains unclear whether population level estimates of network organization will translate to prospective target selection for individual patients.
4.2. Precision neuroscience may inform personalized stimulation
Although single-subject fMRI applications have been fraught with potential methodological confounds, more recent technical developments in MRI acquisition and preprocessing have improved both the SNR and temporal resolution of acquired fMRI data. Specifically, multi-band fMRI acquisition protocols, and more recently, multi-echo fMRI (among other preprocessing advancements) have been shown to improve the reliability of single subject network estimates (24, 256). Serial imaging studies of highly sampled individuals suggest that approximately 10 min of data are needed to estimate reliable networks in individual patients using these methods (256), compared with approximately 45 min using standard acquisition techniques (23, 257). These emerging single-subject applications, often termed precision fMRI (pfMRI), provide a viable counterargument to recent high-profile indictments against the inferential power of population-level fMRI analyses, which suggest that thousands of patients are required to identify reliable brain behavior relationships (23, 252, 258). Together with pervasive heterogeneity in preprocessing and deficient statistical methods across the fMRI literature (259, 260), such power concerns are thought to be contributing to a widespread generalizability crisis in neuroscience (261). However, it seems equally likely that noisy and unreliable estimates at the group level are a direct consequence of averaging across important individual differences in brain organization (23, 262, 263). Indeed, brain networks are highly variable from person to person (26–28, 243), but generally consistent within individuals (22, 24, 25, 30, 31). Recent work combining individualized network estimates with MRI-guided dlPFC rTMS underscores that targeting the same anatomical area engages different functional regions and RSNs in different patients, providing further support for the assertion that individual estimates are more likely to yield reliable targeting estimates for neuromodulation (19, 23). The critical importance of personalized targeting finds additional support in the cognitive neuroscience literature, where targeting TMS with individual subject fMRI data leads to significantly greater effect sizes across a range of behavioral experiments compared with anatomical, group-level functional, and scalp landmark based targeting approaches (264).
4.3. Applications of precision neuromodulation
There are few clinical targeting implementations that validate group-level estimates with individual subject data (11, 15) and fewer still that have used baseline rsFC to guide prospective targeting for individual patients (9, 16, 19). To our knowledge, only one group has successfully used individual subject fMRI data to prospectively generate a targeting prediction for subsequent TMS (9, 16). Although emerging data from the Stanford Neuromodulation Therapy (SNT) protocol suggests that personalized targeting estimates may improve outcomes for patients with MDD, the authors incorporate two parallel advances to conventional TMS protocols that limit attribution of benefit to personalized targeting. Patients receiving SNT receive a novel and higher dose intermittent theta burst TMS protocol that alone may explain the impressive remission rates, greater than 75%, seen in the study. This approach was compared with sham stimulation, but not active stimulation of a control target, leaving considerable uncertainty regarding the specific contribution of personalized targeting to the overall result. Moreover, although the SNT method uses individual patient fMRI data to generate targeting estimates, the search space for the personalized target is constrained by both the key sgACC region and planned dlPFC stimulation region, such that the targeting algorithm refines dlPFC placement to optimize sgACC connectivity but does not allow for identification of alternative targets outside of the established dlPFC search space. Thus, while the method offers compelling evidence that precision is key to optimize targeting, there are currently no reliable methods to identify optimal sites from a brain-wide list of potential candidates.
4.4. Summary, caveats, and emerging applications to chronic pain
Experience with hypothesis-driven target selection in MDD suggests that rsFC with a single region of a priori interest (i.e., sgACC) can explain a substantial amount of variability in treatment outcomes. Prior work suggests that using a single depression-relevant sgACC region to generate rsFC estimates may also be a viable means to generate prospective, rsFC-based targeting estimates for individual patients. Results linking rsFC between efficacious TMS and DBS targets across neuropsychiatric disorders suggests that identifying a network of relief (i.e., rsFC patterns that link all effective sites across stimulation modalities and exclude ineffective ones) should facilitate more consistent stimulation outcomes for patients with a variety of disorders (13). However, this model would predict that sgACC should be an effective DBS target, yet only 4 of 6 patients obtained benefit in the first sgACC DBS study (265). Moreover, a larger multi-center sgACC DBS trial was halted for underperformance relative to the preceding open label trial. Remission rates for the sgACC DBS target in subsequent open label reports were around 30% (266, 267). Even more difficult to reconcile are the markedly higher remission rates seen in the SNT rTMS trial that used sgACC to identify a personalized left dlPFC target. As above, results from SNT may reflect the higher “dose” of electrical stimulation inherent to the theta-burst protocol, or unique stimulation parameters of rTMS relative to DBS, but may also reflect the specific rsFC method used to derive the dlPFC target. Rather than using rsFC with the consensus sgACC region of interest, a hierarchical agglomerative clustering algorithm was used to identify unique functional sgACC subunits based on patterns of brain-wide connectivity. Next, optimal dlPFC connectivity with each subunit was used to inform the final rTMS target. Such an approach may overcome structure-function limitations inherent to using a single group-average sgACC region for all patients. It is also possible that whole-brain agglomerative clustering may be unintentionally identifying dlPFC nodes based on as-yet unknown global network properties that have little to do with specific connections between sgACC and dlPFC.
In chronic pain, PAG and sensorimotor cortex (M1/S1) are proposed as viable candidate network hubs for rsFC-based targeting, akin to the established sgACC region in MDD (13, 128). However, as reviewed in Section 3, stimulation at both PAG and M1 elicits variable analgesia (Table 3). Although prior work predicts that M1 rTMS should be more efficacious than left dlPFC rTMS for patients with chronic pain expressly because M1, but not dlPFC, is significantly anticorrelated with PAG in the normative connectome dataset, there are reports of clinical pain benefit from left dlPFC rTMS for individual patients and dozens of reports of rTMS at M1 showing modest pain relief and relatively low responder rates, especially for widespread and lower extremity pain conditions (6, 7, 139). Incidentally, the M1 stimulation target with best evidence for clinical efficacy across pain conditions already overcomes several targeting limitations posed by other neuropsychiatric disorders. Targeting M1 requires elicitation of an observable motor response in the affected (contralateral) extremity for each patient, similar to personalization with intraoperative macrostimulation in PAG and Thalamic DBS [see (6, 139, 220) for review]. M1 stimulation reliably engages a distributed network of pain-relevant regions (227, 228, 230) and has recently been shown to modulate between network connectivity between the DMN and salience networks (268), highlighting multiple viable network mechanisms for analgesia. Moreover, interindividual variability in representations of acute pain are greatest in midline prefrontal regions commonly implicated in the transition to chronic pain (32, 34) and lowest in regions like M1 (29). Despite these advantages, response rates for M1 TMS are highly variable, modest, and often short-lived. The lack of viable network informed targets for chronic pain and failure of even the best contemporary targeting models in other disorders for a substantial subset of patients highlights the clear need for alternative strategies to identify more robust and generalizable network-informed imaging biomarkers for brain stimulation (36).
We propose that our emerging understanding of the profound interindividual variability in functional networks demands a personalized approach that uses individual subject fMRI data to generate predictions of individually optimized stimulation targets (23, 269, 270). The dearth of reliable neural correlates of chronic pain in clinical populations and the observation that both intrinsic RSNs and patterns of evoked activity to pain are profoundly variable across individuals suggest that any attempt to identify a single optimal brain region for analgesic stimulation will fail for a substantial number of patients. Although it is attractive to consider the possibility that a small number of viable targets may be generalizable across patients, seeking to manipulate single brain regions or even single networks may be overly reductive, as this approach largely ignores the substantial contribution of dynamic interactions between brain regions to perception and behavior (99, 100). Considering the apparent limitations of selecting brain regions based on rsFC with a subset of defined anatomical regions of interest, we hypothesize that a truly network-informed approach to stimulation should instead consider the overall topology of the network to identify personalized regions where stimulation is most likely to have a widespread impact on pain-relevant regions and subnetworks. This approach fundamentally reframes the characteristics of an “ideal target” away from specific neuroanatomical regions defined at the population level and toward estimates that carry more information about the overall structure and organization of individual brains (i.e., topology) to characterize the functional role of each candidate region within the overall network. Below we elaborate on this approach and review novel network analysis methods that may be more robust to variability in network topology across individuals.
5. Modeling network dysfunction in pain to inform biomarker development
An emerging network neuroscience literature using mathematics from graph theory has established powerful methods to characterize integrated local and global network organization unconstrained by the specific anatomical location of the component parts of the system. A variety of complex systems—ranging from transportation and telecommunications infrastructure to metabolic and neural networks—are intuitively represented and analyzed using mathematics from graph theory (271–276). Graphs are constructed from nodes consisting of individual units in the system (e.g., airports, neurons) and edges consisting of connections between units (e.g., flights, axons). The key advance of graph theory over conventional approaches to estimating rsFC (i.e., with RSNs) is representing brain regions as nodes and rsFC between pairs of regions as edges in a graph in order to facilitate the computation of a variety of neurobiologically meaningful summary estimates that simultaneously capture properties of individual regions and their relationship to the overall network topology (277). To generate a network graph, rsFC between all pairs of nodes (i.e., regions) is used to construct a correlation matrix, which is then normalized, thresholded, and binarized to construct a network graph that visually represents the entire system (Figures 2A–E). A variety of widely available open-source toolkits are then used to estimate region and network level parameters from the network graph (271, 277, 278). Notably, graph theory can be used to represent a broad range of related network properties, including structural connections estimated from white matter integrity between regions, functional connections estimate with rsFC between regions, and regional transcriptomes, among other emerging applications (271). Here, we primarily focus on functional networks defined with rsFC for consistency. A comprehensive summary of the graph terms relevant for network neuroscience is beyond the scope of the current review, but fall into measures of integration, segregation, and centrality. We suggest that the reader review (277) for a detailed description of network terms and their functional significance and (279) for a summary of graph theory applications in chronic pain.
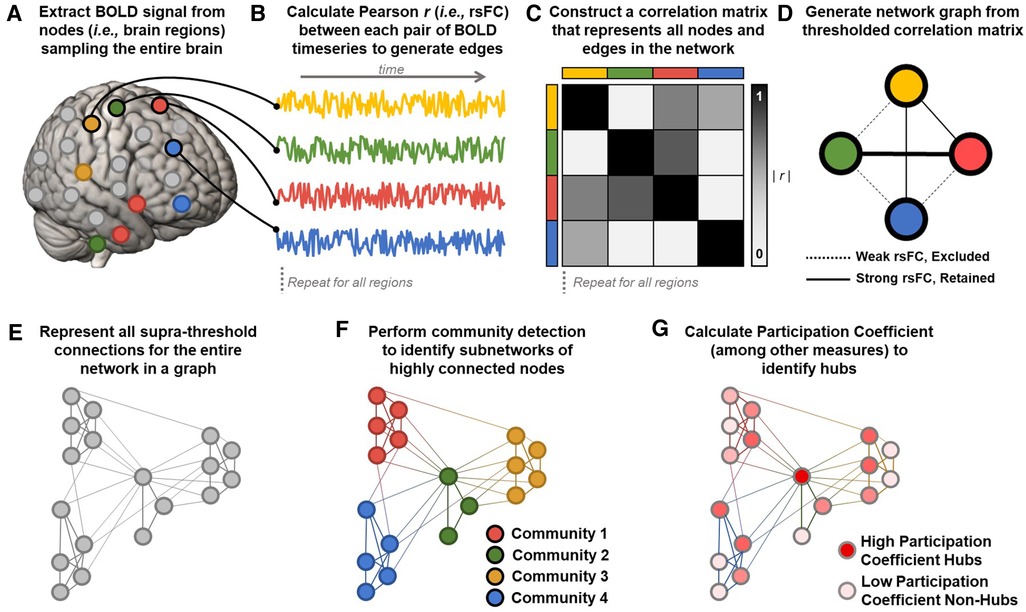
Figure 2. Calculating graphs from fMRI data to estimate network parameters. (A) Resting state blood-oxygen-level-dependent (BOLD) signals are extracted from a parcellation of nodes sampling the entire brain. (B) Representative BOLD timeseries from each node are used to calculate Pearson correlation coefficients (r) between each pair of nodes to generate edges reflecting resting state functional connectivity (rsFC) between regions. (C) Z-transformed rsFC between all possible pairs of regions is represented as a correlation matrix. (D) The most significant functional connections in the graph (typically the top 2%–10% of rsFC values) are used to construct a network graph consisting of above threshold nodes and edges. (E) An example of an entire brain network topology represented as a graph, generated using the methods outlined in A–D. (F) Community detection algorithms (e.g., Infomap, Louvain) assign each node to a community (i.e., module) consisting of color-coded groups of nodes that are more strongly connected with each other than with nodes in other communities. Network modularity can be calculated by comparing within- and between-module connections. In this example, four communities are detected, with colors corresponding to the four pain processing subnetworks shown in Figure 1A. Note that community detection algorithms are data-driven and need not correspond pre-defined sensory, affective, cognitive, and regulatory subnetworks. (G) Once nodes are assigned to communities, additional parameters like participation coefficient can be calculated to identify nodes connected to many different communities. In this example, a central node from the pain modulatory subnetwork (green in panel F) is maximally connected to all four communities and is an ideal candidate connector or integrator hub (dark red). Peripheral nodes in each subnetwork are least hub-like (light pink).
Of particular interest to studies of large scale network organization and precision neuromodulation is the concept of modularity, which describes the functional segregation or clustering of nodes (i.e., brain regions) into subnetworks (i.e., modules or communities) that have stronger connections with nodes in the same module than with nodes in other modules (Figure 2F) (272, 274, 277, 280, 281). Community detection algorithms (e.g., Louvain, Infomap) are applied to thresholded network graphs to identify synchronous subnetworks characterized by many within-module connections and fewer between-module connections (281, 282). Modularity and community structure are universal organizing principles of complex biological systems (190, 275, 278, 281). Highly modular networks exhibit functional segregation, and subnetworks estimated from rsFC data reliably correspond with separate canonical RSNs involved in different aspects of cognition (e.g., somatosensory, limbic, attention, and default mode networks) (100, 101). Critically, network graphs produce valid and reliable estimates at the single subject level (23, 101, 283) and prior work has shown that modularity has prognostic value in predicting individual differences in treatment outcomes (284).
The decomposition of brain networks into functionally specialized modules enables the identification of highly connected hub nodes that are well situated to support integrative processing across the entire network (272, 280, 283). Hub nodes with high participation coefficient (PC), also termed the “diverse club”, have the most diverse connectivity across the network's functional modules and are therefore well-suited to integrate activity between distinct subnetworks (280, 283, 285). Participation coefficient takes a maximal value for nodes that have an equal number of connections to each separate module in the network (Figure 2G). In humans, brain regions with hub-like characteristics facilitate integrative processing across distributed functional subnetworks and support modular network organization (285). Nodes with high PC also promote dynamic network reorganization to accommodate evolving task demands and their recruitment predicts task performance (286, 287). Damage to nodes with high PC impairs the modular architecture of human brain networks (288) and is a common predictor of disease severity across neuropsychiatric disorders ranging from Alzheimer's disease to chronic pain (289). Further, targeted disruption of network hubs with personalized fMRI-guided TMS markedly impairs cognitive and integrative function, providing additional causal evidence of the importance of hubs to large scale network integration (257).
Notably, whereas “hubs” of the pain matrix described in Section 2 are defined based on a priori functional attributions to implicated brain regions (i.e., regions of particular importance), hubs in a network graph are derived from the observed correlational structure of the studied network. There is substantial overlap between network hubs identified using data-driven community detection algorithms and putative Pain Matrix hubs, including ACC and aIns, which may reflect the fact that many pain-relevant regions are in higher-order association areas (280, 283). Importantly, the overlap between graph hubs and Pain Matrix hubs provides a viable alternative fifth network hypothesis with which to understand clinical stimulation effects at prior DBS and TMS targets. Because network “hubs” are more likely to be members of many distinct pain-relevant subnetworks, stimulating hub regions should have widespread effects on the multidimensional experience of chronic pain. Thus, we hypothesize that clinical benefit at any given target may reflect the “hub-ness” of the underlying target region.
5.1. Evidence for impaired network integration and hub disruption in chronic pain
The application of graph theoretical methods to chronic pain has begun to reveal widespread changes in network topology that are shared across pain etiologies but notably distinct from healthy populations (279). Pain networks defined based on intracranial physiologic responses to pain are inherently modular and segregate into subnetworks that reflect known pain processing domains (290). A variety of established graph theory terms describing network topology and the roles of individual nodes (e.g., Thalamus) have been shown to correlate with clinical pain metrics, including pain intensity, duration, and pain-related disability, suggesting that graph terms are behaviorally relevant (279).
Studies evaluating specific graph theory parameters across chronic pain conditions and species suggest that disruption of modular network organization is associated with emergence of the chronic pain state (291, 292). Direct comparisons of the full range of available graph terms between chronic pain populations and healthy cohorts and between different chronic pain conditions are limited by substantial variability in methods and reporting across the small number of available studies; however, there is an emerging consensus that global network changes and integrated local/global estimates like participation coefficient can more reliably distinguish patients with chronic pain from healthy controls. Specifically, chronic pain of various etiologies is associated with characteristic disruptions in the way that network hubs interact with multiple functional modules, leading to an emerging hypothesis that chronic pain is a state of dysfunctional network integration (291, 292). Global summary terms like the hub disruption index (HDI) and whole brain degree rank order disruption (KD) were developed to quantify how graphs constructed in individual subjects diverge from a normative comparison population. Consistent HDI and KD changes have been observed in patients with chronic pain of various etiologies and in rodents with nerve-injury induced neuropathic pain, suggesting that, across species and pain conditions, well-connected regions become less well-connected in chronic pain. Overall, HDI and KD analyses indicate that regions with hub-like characteristics (e.g., high participation coefficient or network centrality) are less hub-like, indicating that the emergence of chronic pain represents a fundamental shift in the identity of important network hubs within a modular network architecture. Taken together, prior work suggests that network-informed stimulation at personalized regions with hub-like characteristics should restore network integration and reduce chronic pain. However, applications of graph theory to clinical pain conditions are still in their infancy and it seems likely that a weighted combination of different graph parameters may be required to identify reliable stimulation sites in chronic pain (293).
6. Summary and future directions
The graph theory framework outlined above represents a fundamental reframing of precision medicine away from specific neuroanatomical targets defined at the population level toward data-driven, individualized local and global network estimates that are more reliably implicated in the pathogenesis of chronic pain. As with prior region and RSN models, combining network graphs with targeted stimulation can begin to support a causal link between the characteristics of specific network nodes (i.e., brain regions), global network organization, and the experience of chronic pain. We propose that studies of clinical neuromodulation should routinely employ pre/post brain imaging measures to facilitate network analysis and better ascertain mechanisms of clinically efficacious brain stimulation for individual patients, consistent with IMMPACT recommendations for clinical trials in chronic pain (57, 294, 295).
One key advantage of the graph theory approach over measurements of activity and connectivity derived from the BOLD signal is that it dramatically expands the types of information that can be estimated from a network. New applications of graph theory in network neuroscience are continually evolving. Whereas static network graphs provide a snapshot of network organization, recent advances in dynamic network analysis are beginning to show how network topologies evolve and change with learning and intervention (286, 296–298). Dynamic time-varying graph analyses may in turn facilitate a variety of analyses ranging from estimation of node promiscuity (i.e., membership in multiple distinct functional modules over time) to linking dynamic reorganization of networks to experiential states, such as high and low pain (99). Dynamic analyses may also be used to explore the state-dependence of neuromodulation targets depending on the context of stimulation, which builds upon the who and where questions of stimulation with additional information about when stimulation is most likely to be effective.
Associating specific network topologies with clinically-relevant states (e.g., high and low pain) will also facilitate more advanced analyses of network controllability, which describe how stimulating a subset of key nodes might drive a network toward a desired state (i.e., low-pain) (299–302). Such an approach could be undertaken through off-line identification of specific regions that reliably transition a given patient into a low pain state (i.e., identifying network characteristics of the pain-relieving node) or by recording real-time pain ratings during brain imaging to determine whether fluctuations in clinical pain are accompanied by temporally linked dynamic shifts in network organization. It is likely that combining these two levels of analysis will be required to build a comprehensive precision network model capable of predicting which regions are most likely to induce favorable state transitions for individual patients (e.g., from high to low pain).
Although graph theory has previously linked network reconfiguration dynamics with behavior following learning or intervention (284, 287), to our knowledge it has never been used to evaluate candidate brain regions for therapeutic stimulation. We hypothesize that deploying precision network neuroscience and graph theory to trials of therapeutic brain stimulation will enable a deeper understanding of the networks that contribute to the experience of pain and facilitate the identification of more effective personalized stimulation targets. The parallel emergence of valid and reliable methods for single subject fMRI and an expanding array of graph theory tools for network neuroscience offer great promise to advance our understanding of the network correlates of pain and relief. However, taking full advantage of the considerable opportunities presented by ongoing trials of neuromodulation for pain will require careful attention to methods for data collection, analysis, interpretation, and dissemination. To conclude, we offer recommendations for future neuromodulation research to facilitate network analyses and ongoing development of circuit-based treatments for pain.
7. Recommendations for future research
7.1. Data collection
1. Collect sufficient data to generate precision network estimates.
a. At minimum, studies should acquire high resolution T1-weighted anatomical sequences for co-registration, diffusion tensor imaging (DTI) sequences for estimating structural white matter connections, and resting state fMRI (rs-fMRI) sequences for estimating functional brain networks, ideally at a field strength of 3 T (36, 57, 295). A discussion of the full range of sequences and parameters is beyond the scope of the present article, but we provide some “minimum necessary” acquisition guidelines below. We suggest that investigators establishing new imaging protocols follow guidelines from large consortia like the Adolescent Brain Child Development (ABCD) study, which has ready-to-use protocols for most Siemens, GE, and Philips scanners (303).
i. Anatomical: 3D T1-weighted images with at least 1 mm × 1 mm × 1 mm resolution (approx. 5–7 min acquisition time).
ii. White Matter (DTI): at minimum including one unweighted image (i.e., T2 or b = 0) and at least 6 diffusion-weighted images with orthogonally oriented gradients. We recommend using a high angular resolution diffusion imaging (HARDI) protocol with at least 60 orthogonal diffusion directions and multiple gradient strengths for improved white matter tract tracing performance (approx. 6–10 min acquisition time).
iii. Functional MRI: Optimization of fMRI parameters often involves trade-offs between spatial and temporal resolution, with specific protocols customized to the population and brain regions of interest. Most contemporary fMRI protocols have spatial resolution of 2–3.5 mm3 and temporal resolution (defined by the TR, or repetition time, to acquire one full brain volume) of around 2 s (304). Precision network estimates require ∼45 min of data using conventional rs-fMRI sequences (i.e., TRs of 2–3 s) and ∼10–15 min using multi-band fMRI (which reduces TR to ∼800 ms) and multi-echo fMRI (which acquires up to 4 separate brain volumes per TR, each with different SNR characteristics) (23, 256).
2. Collect data over multiple sessions or during real-time symptom ratings.
a. Linking experiential pain states with dynamic network graphs can improve understanding of brain-behavior relationships (99).
7.2. Data analysis
1. Follow consensus guidelines for data preprocessing and analysis to facilitate comparison with prior work (305–307).
2. Use appropriate parameters to generate valid network graphs.
a. Use standard parcellations to define nodes (i.e., brain regions) that ensure adequate coverage of brain areas of interest and correspond with functional units of brain organization (101, 308).
b. Apply rsFC thresholds to ensure that edges are sufficiently sparse to generate meaningful and interpretable graphs, typically including only the top 10% of connections (101, 278, 281).
7.3. Data interpretation
1. Follow consensus guidelines for reporting data acquisition and analysis parameters for functional brain imaging studies (309).
2. Ground interpretations in extant network models of pain perception such that each study can support or refute available hypotheses to guide community consensus (38, 43, 94, 115, 310).
Data availability statement
The original contributions presented in the study are included in the article, further inquiries can be directed to the corresponding author.
Author contributions
JM developed the hypothesis, refined the theory, wrote and edited the manuscript, and created the figures. PS and MD’E provided the framework for the theory, refined the theory, and edited the manuscript. IK provided input to the theory, performed literature review, created the tables, and edited the manuscript. All authors contributed to the article and approved the submitted version.
Funding
This work is supported by National Institutes of Neurological Disorders and Stroke R25 Grant NS070680 (JM); National Institutes of Neurological Disorders and Stroke UH3 NS109556 (PS) and 1UH3 NS115631 (PS).
Acknowledgments
We thank Ankit Khambhati and Ioannis Pappas for formative discussions about the method and Allan Basbaum for his comments on a prior draft of this manuscript.
Conflict of interest
The authors declare that the research was conducted in the absence of any commercial or financial relationships that could be construed as a potential conflict of interest.
Publisher's note
All claims expressed in this article are solely those of the authors and do not necessarily represent those of their affiliated organizations, or those of the publisher, the editors and the reviewers. Any product that may be evaluated in this article, or claim that may be made by its manufacturer, is not guaranteed or endorsed by the publisher.
References
1. Dahlhamer J. Prevalence of chronic pain and high-impact chronic pain among adults—United States, 2016. Morb Mortal Wkly Rep. (2018) 67:1001–6. doi: 10.15585/mmwr.mm6736a2
2. Frizon LA, Yamamoto EA, Nagel SJ, Simonson MT, Hogue O, Machado AG. Deep brain stimulation for pain in the modern era: a systematic review. Neurosurgery. (2020) 86(2):191–202. doi: 10.1093/neuros/nyy552
3. Shirvalkar P, Veuthey TL, Dawes HE, Chang EF. Closed-loop deep brain stimulation for refractory chronic pain. Front Comput Neurosci. (2018) 12:18. doi: 10.3389/fncom.2018.00018
4. Arulpragasam AR, van ‘t Wout-Frank M, Barredo J, Faucher CR, Greenberg BD, Philip NS. Low intensity focused ultrasound for non-invasive and reversible deep brain neuromodulation-A paradigm shift in psychiatric research. Front Psychiatry. (2022) 13:825802. doi: 10.3389/fpsyt.2022.825802
5. Di Biase L, Falato E, Caminiti ML, Pecoraro PM, Narducci F, Di Lazzaro V. Focused ultrasound (FUS) for chronic pain management: approved and potential applications. Neurol Res Int. (2021) 2021:1–16. doi: 10.1155/2021/8438498
6. Lefaucheur JP, Aleman A, Baeken C, Benninger DH, Brunelin J, Di Lazzaro V, et al. Evidence-based guidelines on the therapeutic use of repetitive transcranial magnetic stimulation (rTMS): an update (2014–2018). Clin Neurophysiol. (2020) 131(2):474–528. doi: 10.1016/j.clinph.2019.11.002
7. O’Connell NE, Marston L, Spencer S, Desouza LH, Wand BM. Non-invasive brain stimulation techniques for chronic pain. Cochrane Database Syst Rev. (2018) 2018:4. doi: 10.1002/14651858.CD008208.pub3
8. Cash RFH, Weigand A, Zalesky A, Siddiqi SH, Downar J, Fitzgerald PB, et al. Using brain imaging to improve spatial targeting of transcranial magnetic stimulation for depression. Biol Psychiatry. (2021) 90(10):689–700. doi: 10.1016/j.biopsych.2020.05.033
9. Cole EJ, Phillips AL, Bentzley BS, Stimpson KH, Nejad R, Barmak F, et al. Stanford neuromodulation therapy (SNT): a double-blind randomized controlled trial. Am J Psychiatry. (2022) 179(2):132–41. doi: 10.1176/appi.ajp.2021.20101429
10. Drysdale AT, Grosenick L, Downar J, Dunlop K, Mansouri F, Meng Y, et al. Resting-state connectivity biomarkers define neurophysiological subtypes of depression. Nat Med. (2017) 23:28–38. doi: 10.1038/nm.4246
11. Horn A, Reich M, Vorwerk J, Li N, Wenzel G, Fang Q, et al. Connectivity predicts deep brain stimulation outcome in Parkinson disease. Ann Neurol. (2017) 82:67–78. doi: 10.1002/ana.24974
12. Li N, Baldermann JC, Kibleur A, Treu S, Akram H, Elias GJB, et al. A unified connectomic target for deep brain stimulation in obsessive-compulsive disorder. Nat Commun. (2020) 11:3364. doi: 10.1038/s41467-020-16734-3
13. Fox MD, Buckner RL, Liu H, Mallar Chakravarty M, Lozano AM, Pascual-Leone A. Resting-state networks link invasive and noninvasive brain stimulation across diverse psychiatric and neurological diseases. Proc Natl Acad Sci U S A. (2014) 111(41):E4367–75. doi: 10.1073/pnas.1405003111
14. Siddiqi SH, Schaper FLWVJ, Horn A, Hsu J, Padmanabhan JL, Brodtmann A, et al. Brain stimulation and brain lesions converge on common causal circuits in neuropsychiatric disease. Nat Hum Behav. (2021) 5(12):1707–16. doi: 10.1038/s41562-021-01161-1
15. Cash RFH, Zalesky A, Thomson RH, Tian Y, Cocchi L, Fitzgerald PB. Subgenual functional connectivity predicts antidepressant treatment response to transcranial magnetic stimulation: independent validation and evaluation of personalization. Biol Psychiatry. (2019) 86(2):e5–7. doi: 10.1016/j.biopsych.2018.12.002
16. Cole EJ, Stimpson KH, Bentzley BS, Gulser M, Cherian K, Tischler C, et al. Stanford accelerated intelligent neuromodulation therapy for treatment-resistant depression. Am J Psychiatry. (2020) 177(8):716–26. doi: 10.1176/appi.ajp.2019.19070720
17. Weigand A, Horn A, Caballero R, Cooke D, Stern AP, Taylor SF, et al. Prospective validation that subgenual connectivity predicts antidepressant efficacy of transcranial magnetic stimulation sites. Biol Psychiatry. (2018) 84(1):28–37. doi: 10.1016/j.biopsych.2017.10.028
18. Khambhati AN, Shafi A, Rao VR, Chang EF. Long-term brain network reorganization predicts responsive neurostimulation outcomes for focal epilepsy. Sci Transl Med. (2021) 13(608):eabf6588. doi: 10.1126/scitranslmed.abf6588
19. Lynch CJ, Elbau IG, Ng TH, Wolk D, Zhu S, Ayaz A, et al. Automated optimization of TMS coil placement for personalized functional network engagement. Neuron. (2022) 110:3263–77. doi: 10.1016/j.neuron.2022.08.012
20. Scangos KW, Khambhati AN, Daly PM, Owen LW, Manning JR, Ambrose JB, et al. Distributed subnetworks of depression defined by direct intracranial neurophysiology. Front Hum Neurosci. (2021) 15:746499. doi: 10.3389/fnhum.2021.746499
21. Scangos KW, Makhoul GS, Sugrue LP, Chang EF, Krystal AD. State-dependent responses to intracranial brain stimulation in a patient with depression. Nat Med. (2021) 27:229–31. doi: 10.1038/s41591-020-01175-8
22. Finn ES, Shen X, Scheinost D, Rosenberg MD, Huang J, Chun MM, et al. Functional connectome fingerprinting: identifying individuals using patterns of brain connectivity. Nat Neurosci. (2015) 18(11):1664–71. doi: 10.1038/nn.4135
23. Gratton C, Kraus BT, Greene DJ, Gordon EM, Laumann TO, Nelson SM, et al. Defining individual-specific functional neuroanatomy for precision psychiatry. Biol Psychiatry. (2020) 88(1):28–39. doi: 10.1016/j.biopsych.2019.10.026
24. Poldrack RA. Precision neuroscience: dense sampling of individual brains. Neuron. (2017) 95(4):727–9. doi: 10.1016/j.neuron.2017.08.002
25. Poldrack RA, Laumann TO, Koyejo O, Gregory B, Hover A, Chen M-Y, et al. Long-term neural and physiological phenotyping of a single human. Nat Commun. (2015) 6:8885. doi: 10.1038/ncomms9885
26. Gordon EM, Laumann TO, Adeyemo B, Gilmore AW, Nelson SM, Dosenbach NUF, et al. Individual-specific features of brain systems identified with resting state functional correlations. NeuroImage. (2017) 146:918–39. doi: 10.1016/j.neuroimage.2016.08.032
27. Gordon EM, Laumann TO, Adeyemo B, Petersen SE. Individual variability of the system-level organization of the human brain. Cerebral Cortex. (2017) 27(1):386–99. doi: 10.1093/cercor/bhv239
28. Gratton C, Laumann TO, Nielsen AN, Greene DJ, Gordon EM, Gilmore AW, et al. Functional brain networks are dominated by stable group and individual factors, not cognitive or daily variation. Neuron. (2018) 98(2):439–452.e5. doi: 10.1016/j.neuron.2018.03.035
29. Kong R, Li J, Orban C, Sabuncu MR, Liu H, Schaefer A, et al. Spatial topography of individual-specific cortical networks predicts human cognition, personality, and emotion. Cerebral Cortex. (2019) 29(6):2533–51. doi: 10.1093/cercor/bhy123
30. Laumann TO, Gordon EM, Adeyemo B, Snyder AZ, Joo SJ, Chen M-Y, et al. Functional system and areal organization of a highly sampled individual human brain. Neuron. (2015) 87(3):657–70. doi: 10.1016/j.neuron.2015.06.037
31. Seitzman BA, Gratton C, Laumann TO, Gordon EM, Adeyemo B, Dworetsky A, et al. Trait-like variants in human functional brain networks. Proc Natl Acad Sci U S A. (2019) 116(45):22851–61. doi: 10.1073/pnas.1902932116
32. Kohoutovamp L, Atlas LY, Bamp C, Buhle JT, Geuter S, Jepma M, et al. Individual variability in brain representations of pain. Nat Neurosci. (2022) 25:749–59. doi: 10.1038/s41593-022-01081-x
33. Mayr A, Jahn P, Stankewitz A, Deak B, Winkler A, Witkovsky V, et al. Patients with chronic pain exhibit individually unique cortical signatures of pain encoding. Hum Brain Mapp. (2022) 43(5):1676–93. doi: 10.1002/hbm.25750
34. Zunhammer M, Spisák T, Wager TD, Bingel U, Atlas L, Benedetti F, et al. Meta-analysis of neural systems underlying placebo analgesia from individual participant fMRI data. Nat Commun. (2021) 12:1. doi: 10.1038/s41467-021-21179-3
35. Cho C, Deol HK, Martin LJ. Bridging the translational divide in pain research: biological, psychological and social considerations. Front Pharmacol. (2021) 12:1–15. doi: 10.3389/fphar.2021.603186
36. Woo C-W, Chang LJ, Lindquist MA, Wager TD. Building better biomarkers: brain models in translational neuroimaging. Nat Neurosci. (2017) 20:365–77. doi: 10.1038/nn.4478
37. Basbaum AI, Bautista DM, Scherrer G, Julius D. Cellular and molecular mechanisms of pain. Cell. (2009) 139(2):267–84. doi: 10.1016/j.cell.2009.09.028
38. Bushnell MC, Čeko M, Low LA. Cognitive and emotional control of pain and its disruption in chronic pain. Nat Rev Neurosci. (2013) 14:502–11. doi: 10.1038/nrn3516
39. Duerden EG, Albanese MC. Localization of pain-related brain activation: a meta-analysis of neuroimaging data. Hum Brain Mapp. (2013) 34(1):109–49. doi: 10.1002/hbm.21416
40. Eippert F, Bingel U, Schoell ED, Yacubian J, Klinger R, Lorenz J, et al. Activation of the opioidergic descending pain control system underlies placebo analgesia. Neuron. (2009) 63(4):533–43. doi: 10.1016/j.neuron.2009.07.014
41. Fields H. State-dependent opioid control of pain. Nat Rev Neurosci. (2004) 5(7):565–75. doi: 10.1038/nrn1431
42. Peyron R, Laurent B, García-Larrea L. Functional imaging of brain responses to pain. A review and meta-analysis (2000). Neurophysiol Clin. (2000) 30(5):263–88. doi: 10.1016/S0987-7053(00)00227-6
43. Tracey I, Bushnell MC. How neuroimaging studies have challenged US to rethink: is chronic pain a disease? J Pain. (2009) 10(11):1113–20. doi: 10.1016/j.jpain.2009.09.001
44. Head BYH, Holmes G. Sensory disturbances from cerebral lesions (followup to 1911 brain paper). Lancet. (1912) 179:144–52. doi: 10.1016/S0140-6736(01)64942-0
45. Penfield W, Boldrey E. Somatic motor and sensory representation in man. Brain. (1937) 60:389–443. doi: 10.1093/brain/60.4.389
46. Mazzola L, Isnard J, Peyron R, Mauguière F. Stimulation of the human cortex and the experience of pain: wilder Penfield’s observations revisited. Brain. (2012) 135(2):631–40. doi: 10.1093/brain/awr265
47. Ostrowsky K, Magnin M, Ryvlin P, Isnard J, Guenot M, Mauguière F. Representation of pain and somatic sensation in the human insula: a study of responses to direct electrical cortical stimulation. Cerebral Cortex. (2002) 12(4):376–85. doi: 10.1093/cercor/12.4.376
48. Melzack R, Wall PD. Pain mechanism: a new theory. Science. (1965) 150(3699):971–9. doi: 10.1126/science.150.3699.971
49. Melzack R, Casey KL. Sensory, motivational, and central control determinants of pain: a new conceptual model. In: Kenshalo D, editor. The skin senses. Springfield, IL: Charles C. Thomas (1968). p. 423–39.
50. Iannetti GD, Mouraux A. From the neuromatrix to the pain matrix (and back). Exp Brain Res. (2010) 205(1):1–12. doi: 10.1007/s00221-010-2340-1
51. Melzack R. From the gate to the neuromatrix. Pain. (1999) 6:S121–6. doi: 10.1016/S0304-3959(99)00145-1
52. Mesulam M. Large-scale neurocognitive networks and distributed processing for attention, language, and memory. Ann Neurol. (1990) 28:597–613. doi: 10.1002/ana.410280502
53. Apkarian AV, Bushnell MC, Treede RD, Zubieta JK. Human brain mechanisms of pain perception and regulation in health and disease. Eur J Pain. (2005) 9(4):463–84. doi: 10.1016/j.ejpain.2004.11.001
54. Tracey I. Nociceptive processing in the human brain. Curr Opin Neurobiol. (2005) 15(4):478–87. doi: 10.1016/j.conb.2005.06.010
55. Lee MC, Tracey I. Unravelling the mystery of pain, suffering, and relief with brain imaging. Curr Pain Headache Rep. (2010) 14(2):124–31. doi: 10.1007/s11916-010-0103-0
56. Talbot JD, Marret S, Evans AC, Meyer E, Bushnell MC, Duncan GH. Multiple representations of pain in human cerebral cortex. Science. (1991) 251(4999):1355–8. doi: 10.1126/science.2003220
57. Tracey I, Woolf CJ, Andrews NA. Composite pain biomarker signatures for objective assessment and effective treatment. Neuron. (2019) 101(5):783–800. doi: 10.1016/j.neuron.2019.02.019
58. Buckner RL, Krienen FM, Yeo BTT. Opportunities and limitations of intrinsic functional connectivity MRI. Nat Neurosci. (2013) 16:832–7. doi: 10.1038/nn.3423
59. Logothetis NK. What we can do and what we cannot do with fMRI. Nature. (2008) 453(7197):869–78. doi: 10.1038/nature06976
60. Logothetis NK, Pauls J, Augath M, Trinath T, Oeltermann A. Neurophysiological investigation of the basis of the fMRI signal. Nature. (2001) 412(6843):150–7. doi: 10.1038/35084005
61. Atlas LY, Bolger N, Lindquist MA, Wager TD. Brain mediators of predictive cue effects on perceived pain. J Neurosci. (2010) 30(39):12964–77. doi: 10.1523/JNEUROSCI.0057-10.2010
62. Keltner JR, Furst A, Fan C, Redfern R, Inglis B, Fields HL. Isolating the modulatory effect of expectation on pain transmission: a functional magnetic resonance imaging study. J Neurosci. (2006) 26(16):4437–43. doi: 10.1523/JNEUROSCI.4463-05.2006
63. Ploghaus A, Becerra L, Borras C, Borsook D. Neural circuitry underlying pain modulation: expectation, hypnosis, placebo. Trends Cogn Sci. (2003) 7(5):197–200. doi: 10.1016/S1364-6613(03)00061-5
64. Price DD. Psychological and neural mechanisms of the affective dimension of pain. Science. (2000) 288(5472):1769–72. doi: 10.1126/science.288.5472.1769
65. Rainville P. Brain mechanisms of pain affect and pain modulation. Curr Opin Neurobiol. (2002) 12(2):195–204. doi: 10.1016/S0959-4388(02)00313-6
66. Wiech K, Ploner M, Tracey I. Neurocognitive aspects of pain perception. Trends Cogn Sci. (2008) 12(8):306–13. doi: 10.1016/j.tics.2008.05.005
67. Rainville P, Duncan G, Price D, Carrier B, Bushnell M. Pain affect encoded in human anterior cingulate but not somatosensory cortex. Science. (1997) 277:968–71. doi: 10.1126/science.277.5328.968
68. An X, Bandler R, Ongür D, Price JL. Prefrontal cortical projections to longitudinal columns in the midbrain periaqueductal gray in macaque monkeys. J Comp Neurol. (1998) 401:455–79. 10.1002/(SICI)1096-9861(19981130)401:4<455::AID-CNE3>3.0.CO;2-69826273
69. Bingel U, Lorenz J, Schoell E, Weiller C, Büchel C. Mechanisms of placebo analgesia: RACC recruitment of a subcortical antinociceptive network. Pain. (2006) 120:8–15. doi: 10.1016/j.pain.2005.08.027
70. Ploner M, Lee MC, Wiech K, Bingel U, Tracey I. Prestimulus functional connectivity determines pain perception in humans. Proc Natl Acad Sci U S A. (2010) 107(1):355–60. doi: 10.1073/pnas.0906186106
71. Wager TD, Atlas LY, Leotti LA, Rilling JK. Predicting individual differences in placebo analgesia: contributions of brain activity during anticipation and pain experience. J Neurosci. (2011) 31(2):439–52. doi: 10.1523/JNEUROSCI.3420-10.2011
72. Downar J, Crawley AP, Mikulis DJ, Davis KD. A multimodal cortical network for the detection of changes in the sensory environment. Nat Neurosci. (2000) 3:277–83. doi: 10.1038/72991
73. Mouraux A, Diukova A, Lee MC, Wise RG, Iannetti GD. A multisensory investigation of the functional significance of the “pain matrix”. NeuroImage. (2011) 54(3):2237–49. doi: 10.1016/j.neuroimage.2010.09.084
74. D’Esposito M, Ballard D, Aguirre GK, Zarahn E. Human prefrontal cortex is not specific for working memory: a functional MRI study. NeuroImage. (1998) 8(3):274–82. doi: 10.1006/nimg.1998.0364
75. Poldrack RA. Inferring mental states from neuroimaging data: from reverse inference to large-scale decoding. Neuron. (2011) 72(5):692–7. doi: 10.1016/j.neuron.2011.11.001
76. Ferraro S, Klugah-Brown B, Tench CR, Yao S, Nigri A, Demichelis G, et al. Dysregulated anterior insula reactivity as robust functional biomarker for chronic pain—meta-analytic evidence from neuroimaging studies. Hum Brain Mapp. (2022) 43(3):998–1010. doi: 10.1002/hbm.25702
77. Vogt BA, Sikes RW. The medial pain system, cingulate cortex, and parallel processing of nociceptive information. Prog Brain Res. (2000) 122:223–35. doi: 10.1016/S0079-6123(08)62141-X
78. Yarkoni T, Poldrack RA, Nichols TE, Van Essen DC, Wager TD. Large-scale automated synthesis of human functional neuroimaging data. Nat Methods. (2011) 8(8):665–70. doi: 10.1038/nmeth.1635
79. Shackman AJ, Salomons TV, Slagter HA, Fox AS, Winter JJ, Davidson RJ. The integration of negative affect, pain and cognitive control in the cingulate cortex. Nat Rev Neurosci. (2011) 12(3):154–67. doi: 10.1038/nrn2994
80. Vogt BA. Pain and emotion interactions in subregions of the cingulate gyrus. Nat Rev Neurosci. (2005) 6:533–44. doi: 10.1038/nrn1704
81. Cheriyan J, Sheets PL. Altered excitability and local connectivity of mPFC-PAG neurons in a mouse model of neuropathic pain. J Neurosci. (2018) 38:4829–39. doi: 10.1523/JNEUROSCI.2731-17.2018
82. Fuchs PN, Peng YB, Boyette-Davis JA, Uhelski ML. The anterior cingulate cortex and pain processing. Front Integr Neurosci. (2014) 8:1–10. doi: 10.3389/fnint.2014.00035
83. Gu L, Uhelski ML, Anand S, Romero-Ortega M, Kim YT, Fuchs PN, et al. Pain inhibition by optogenetic activation of specific anterior cingulate cortical neurons. PLoS One. (2015) 10(2):1–17. doi: 10.1371/journal.pone.0117746
84. Johansen JP, Fields HL, Manning BH. The affective component of pain in rodents: direct evidence for a contribution of the anterior cingulate cortex. Proc Natl Acad Sci U S A. (2001) 98(14):8077–82. doi: 10.1073/pnas.141218998
85. Petrovic P, Kalso E, Petersson KM, Ingvar M. Placebo and opioid analgesia—imaging a shared neuronal network. Science. (2002) 295:1737–40. doi: 10.1126/science.1067176
86. Wager TD, Scott DJ, Zubieta J-K. Placebo effects on human mu-opioid activity during pain. Proc Natl Acad Sci U S A. (2007) 104(26):11056–61. doi: 10.1073/pnas.0702413104
87. Coombes SA, Misra G. Pain and motor processing in the human cerebellum. Pain. (2016) 157(1):117–27. doi: 10.1097/j.pain.0000000000000337
88. Ramos-Fresnedo A, Perez-Vega C, Domingo RA, Cheshire WP, Middlebrooks EH, Grewal SS. Motor cortex stimulation for pain: a narrative review of indications, techniques, and outcomes. Neuromodulation. (2022) 25(2):211–21. doi: 10.1016/j.neurom.2021.10.025
89. Lieberman MD, Eisenberger NI. The dorsal anterior cingulate cortex is selective for pain: results from large-scale reverse inference. Proc Natl Acad Sci U S A. (2015) 112(49):15250–5. doi: 10.1073/pnas.1515083112
90. Wager TD, Atlas LY, Botvinick MM, Chang LJ, Coghill RC, Davis KD, et al. Pain in the ACC? Proc Natl Acad Sci U S A. (2016) 113:18. doi: 10.1073/pnas.1600282113
91. Wager TD, Atlas LY, Lindquist MA, Roy M, Woo C-W, Kross E. An fMRI-based neurologic signature of physical pain. N Engl J Med. (2013) 368(15):1388–97. doi: 10.1056/nejmoa1204471
92. Zunhammer M, Bingel U, Wager TD. Placebo effects on the neurologic pain signature A meta-analysis of individual participant functional magnetic resonance imaging data supplemental content. JAMA Neurol. (2018) 75(11):1321–30. doi: 10.1001/jamaneurol.2018.2017
93. Fox MD, Raichle ME. Spontaneous fluctuations in brain activity observed with functional magnetic resonance imaging. Nat Rev Neurosci. (2007) 8:700–11. doi: 10.1038/nrn2201
94. Kucyi A, Davis KD. The dynamic pain connectome. Trends Neurosci. (2015) 38(2):86–95. doi: 10.1016/j.tins.2014.11.006
95. Biswal B, Yetkin FZ, Haughton VM, Hyde JS. Functional connectivity in the motor cortex of resting human brain using echo-planar MRI. Magn Reson Med. (1995) 34(4):537–41. doi: 10.1002/mrm.1910340409
96. Fox MD, Snyder AZ, Vincent JL, Corbetta M, Van Essen DC, Raichle ME. The human brain is intrinsically organized into dynamic, anticorrelated functional networks. Proc Natl Acad Sci U S A. (2005) 5:9673–8. 10.1073pnas.0504136102
97. Honey CJ, Sporns O, Cammoun L, Gigandet X, Thiran JP, Meuli R, et al. Predicting human resting-state functional connectivity from structural connectivity. Proc Natl Acad Sci U S A. (2009) 106(6):2035–40. 10.1073pnas.081116810619188601
98. Park H-J, Friston K. Structural and functional brain networks: from connections to cognition. Science. (2013) 342(6158):1238411. doi: 10.1126/science.1238411
99. Calhoun VD, Miller R, Pearlson G, Adalı T. The chronnectome: time-varying connectivity networks as the next frontier in fMRI data discovery. Neuron. (2014) 84(2):262–74. doi: 10.1016/j.neuron.2014.10.015
100. Cole MW, Bassett DS, Power JD, Braver TS, Petersen SE. Intrinsic and task-evoked network architectures of the human brain. Neuron. (2014) 83(1):238–51. doi: 10.1016/j.neuron.2014.05.014
101. Power JD, Cohen AL, Nelson SM, Wig GS, Barnes KA, Church JA, et al. Functional network organization of the human brain. Neuron. (2011) 72(4):665–78. doi: 10.1016/j.neuron.2011.09.006
102. Smith SM, Fox PT, Miller KL, Glahn DC, Fox PM, Mackay CE, et al. Correspondence of the brain’s functional architecture during activation and rest. Proc Natl Acad Sci U S A. (2009) 106(31):13040–5. doi: 10.1073/pnas.0905267106
103. He BJ, Snyder AZ, Zempel JM, Smyth MD, Raichle ME. Electrophysiological correlates of the brain’s intrinsic large-scale functional architecture. Proc Natl Acad Sci U S A. (2008) 105(41):16039–44. doi: 10.1073/pnas.0807010105
104. Nir Y, Mukamel R, Dinstein I, Privman E, Harel M, Fisch L, et al. Interhemispheric correlations of slow spontaneous neuronal fluctuations revealed in human sensory cortex. Nat Neurosci. (2008) 11(9):1100–8. doi: 10.1038/nn.2177
105. Keller CJ, Bickel S, Entz L, Ulbert I, Milham MP, Kelly C, et al. Intrinsic functional architecture predicts electrically evoked responses in the human brain. Proc Natl Acad Sci U S A. (2011) 108(25):10308–13. doi: 10.1073/pnas.1019750108
106. Siddiqi SH, Taylor SF, Cooke D, Pascual-Leone A, George MS, Fox MD. Distinct symptom-specific treatment targets for circuit-based neuromodulation. Am J Psychiatry. (2020) 177(5):435–46. doi: 10.1176/appi.ajp.2019.19090915
107. Cohen JR, D’Esposito M. The segregation and integration of distinct brain networks and their relationship to cognition. J Neurosci. (2016) 36(48):12083–94. doi: 10.1523/JNEUROSCI.2965-15.2016
108. Baliki MN, Mansour AR, Baria AT, Apkarian AV. Functional reorganization of the default mode network across chronic pain conditions. PloS One. (2014) 9(9):e106133. doi: 10.1371/journal.pone.0106133
109. Brandl F, Weise B, Mulej Bratec S, Jassim N, Hoffmann Ayala D, Bertram T, et al. Common and specific large-scale brain changes in major depressive disorder, anxiety disorders, and chronic pain: a transdiagnostic multimodal meta-analysis of structural and functional MRI studies. Neuropsychopharmacology. (2022) 47:1071–80. doi: 10.1038/s41386-022-01271-y
110. Hamilton JP, Farmer M, Fogelman P, Gotlib IH. Depressive rumination, the default-mode network, and the dark matter of clinical neuroscience. Biol Psychiatry. (2015) 78(4):224–30. doi: 10.1016/j.biopsych.2015.02.020
111. Kaiser RH, Andrews-Hanna JR, Wager TD, Pizzagalli DA. Large-Scale network dysfunction in Major depressive disorder: a meta-analysis of resting-state functional connectivity. JAMA Psychiatry. (2015) 72(6):603–11. doi: 10.1001/jamapsychiatry.2015.0071
112. Mohan A, Roberto AJ, Mohan A, Lorenzo A, Jones K, Carney MJ, et al. The significance of the default mode network (DMN) in neurological and neuropsychiatric disorders: a review. Yale J Biol Med. (2016) 89(1):49–57.27505016
113. Reddan MC, Wager TD. Brain systems at the intersection of chronic pain and self-regulation. Neurosci Lett. (2019) 702:24–33. doi: 10.1016/j.neulet.2018.11.047
114. Whitfield-Gabrieli S, Ford JM. Default mode network activity and connectivity in psychopathology. Annu Rev Clin Psychol. (2012) 8:49–76. doi: 10.1146/annurev-clinpsy-032511-143049
115. Apkarian AV, Baliki MN, Geha PY. Towards a theory of chronic pain. Prog Neurobiol. (2009) 87(2):81–97. doi: 10.1016/j.pneurobio.2008.09.018
116. Lee JJ, Kim HJ, Čeko M, Park BY, Lee SA, Park H, et al. A neuroimaging biomarker for sustained experimental and clinical pain. Nat Med. (2021) 27(1):174–82. doi: 10.1038/s41591-020-1142-7
117. Tanasescu R, Cottam WJ, Condon L, Tench CR, Auer DP. Functional reorganisation in chronic pain and neural correlates of pain sensitisation: a coordinate based meta-analysis of 266 cutaneous pain fMRI studies. Neurosci Biobehav Rev. (2016) 68:120–33. doi: 10.1016/j.neubiorev.2016.04.001
118. Xu A, Larsen B, Henn A, Baller EB, Scott JC, Sharma V, et al. Brain responses to noxious stimuli in patients with chronic pain: a systematic review and meta-analysis. JAMA Network Open. (2021) 4(1):e2032236. doi: 10.1001/jamanetworkopen.2020.32236
119. Apkarian AV, Baliki MN, Farmer MA. Predicting transition to chronic pain. Curr Opin Neurol. (2013) 26(4):360–7. doi: 10.1097/WCO.0b013e32836336ad
120. Baliki MN, Chang PC, Baria AT, Centeno MV, Apkarian AV. Resting-state functional reorganization of the rat limbic system following neuropathic injury. Sci Rep. (2014) 4:6186. doi: 10.1038/srep06186
121. Baliki MN, Geha PY, Fields HL, Apkarian AV. Article predicting value of pain and analgesia: nucleus accumbens response to noxious stimuli changes in the presence of chronic pain. Neuron. (2010) 66(1):149–60. doi: 10.1016/j.neuron.2010.03.002
122. Baliki MN, Petre B, Torbey S, Herrmann KM, Huang L, Schnitzer TJ, et al. Corticostriatal functional connectivity predicts transition to chronic back pain. Nat Neurosci. (2012) 15(8):1117–9. doi: 10.1038/nn.3153
123. Hashmi JA, Baliki MN, Huang L, Baria AT, Torbey S, Hermann KM, et al. Shape shifting pain: chronification of back pain shifts brain representation from nociceptive to emotional circuits. Brain. (2013) 136(9):2751–68. doi: 10.1093/brain/awt211
124. Erpelding N, Davis KD. Neural underpinnings of behavioural strategies that prioritize either cognitive task performance or pain. Pain. (2013) 154(10):2060–71. doi: 10.1016/j.pain.2013.06.030
125. Fox MD, Halko MA, Eldaief MC, Pascual-Leone A. Measuring and manipulating brain connectivity with resting state functional connectivity magnetic resonance imaging (fcMRI) and transcranial magnetic stimulation (TMS). NeuroImage. (2012) 62(4):2232–43. doi: 10.1016/j.neuroimage.2012.03.035
126. Levy RM. Deep brain stimulation for the treatment of intractable pain. Neurosurg Clin N Am. (2003) 14(3):389–99. doi: 10.1016/S1042-3680(03)00036-6
127. Vaidya AR, Pujara MS, Petrides M, Murray EA, Fellows LK. Lesion studies in contemporary neuroscience. Trends Cogn Sci. (2019) 23(8):653–71. doi: 10.1016/j.tics.2019.05.009
128. Kim NY, Taylor JJ, Kim YW, Borsook D, Joutsa J, Li J, et al. Network effects of brain lesions causing central poststroke pain. Ann Neurol. (2022) 92(5):834–45. doi: 10.1002/ana.26468
129. Gadot R, Najera R, Hirani S, Anand A, Storch E, Goodman WK, et al. Efficacy of deep brain stimulation for treatment-resistant obsessive-compulsive disorder: systematic review and meta-analysis. J Neurol Neurosurg Psychiatry. (2022) 93:1166–73. doi: 10.1136/jnnp-2021-328738
130. Goodman WK, Storch EA, Cohn JF, Sheth SA. Deep brain stimulation for intractable obsessive-compulsive disorder: progress and opportunities. Am J Psychiatry. (2020) 177(3):200–3. doi: 10.1176/appi.ajp.2020.20010037
131. Scangos KW, Khambhati AN, Daly PM, Makhoul GS, Sugrue LP, Zamanian H, et al. Closed-loop neuromodulation in an individual with treatment-resistant depression. Nat Med. (2021) 27:1696–1700. doi: 10.1038/s41591-021-01480-w
132. Sheth SA, Bijanki KR, Metzger B, Allawala A, Pirtle V, Adkinson JA, et al. Deep brain stimulation for depression informed by intracranial recordings. Biol Psychiatry. (2022) 92(3):246–51. doi: 10.1016/j.biopsych.2021.11.007
133. Hallett M. Transcranial magnetic stimulation: a primer. Neuron. (2007) 55(2):187–99. doi: 10.1016/j.neuron.2007.06.026
134. Burke MJ, Fried PJ, Pascual-Leone A. Transcranial magnetic stimulation: neurophysiological and clinical applications. In: D'Esposito M, Grafman JH, editors. Handbook of clinical neurology. The Frontal Lobes. Cambridge, MA: Elsevier (2019). p. 73–92. doi: 10.1016/B978-0-12-804281-6.00005-7
135. Maeda F, Keenan JP, Tormos JM, Topka H, Pascual-Leone A. Modulation of corticospinal excitability by repetitive transcranial magnetic stimulation. Clin Neurophysiol. (2000) 111(5):800–5. doi: 10.1016/S1388-2457(99)00323-5
136. Pascual-leone A, Valls-solé J, Wassermann EM, Hallett M. Responses to rapid-rate transcranial magnetic stimulation of the human motor cortex. Brain. (1994) 117(4):847–58. doi: 10.1093/brain/117.4.847
137. Carpenter LL, Janicak PG, Aaronson ST, Boyadjis T, Brock DG, Cook IA, et al. Transcranial magnetic stimulation (tms) for major depression: a multisite, naturalistic, observational study of acute treatment outcomes in clinical practice. Depress Anxiety. (2012) 29(7):587–96. doi: 10.1002/da.21969
138. Carmi L, Alyagon U, Barnea-Ygael N, Zohar J, Dar R, Zangen A. Clinical and electrophysiological outcomes of deep TMS over the medial prefrontal and anterior cingulate cortices in OCD patients. Brain Stimul. (2018) 11(1):158–65. doi: 10.1016/j.brs.2017.09.004
139. Leung A, Shirvalkar P, Chen R, Kuluva J, Vaninetti M, Bermudes R, et al. Transcranial magnetic stimulation for pain, headache, and comorbid depression: INS-NANS expert consensus panel review and recommendation. Neuromodulation. (2020) 23(3):267–90. doi: 10.1111/ner.13094
140. Philip NS, Arulpragasam AR. Reaching for the unreachable: low intensity focused ultrasound for non-invasive deep brain stimulation. Neuropsychopharmacology. (2023) 48:251–2. doi: 10.1038/s41386-022-01386-2
141. Todd N, McDannold N, Borsook D. Targeted manipulation of pain neural networks: the potential of focused ultrasound for treatment of chronic pain. Neurosci Biobehav Rev. (2020) 115:238–50. doi: 10.1016/j.neubiorev.2020.06.007
142. Baskin DS, Mehler WR, Hosobuchi Y, Richardson DE, Adams JE, Flitter MA. Autopsy analysis of the safety, efficacy and cartography of electrical stimulation of the central gray in humans. Brain Res. (1986) 371(2):231–6. doi: 10.1016/0006-8993(86)90358-6
143. Basbaum AI, Fields HL. Endogenous pain control mechanisms: review and hypothesis. Ann Neurol. (1978) 4(5):451–62. doi: 10.1002/ana.410040511
144. Basbaum AI, Fields HL. Endogenous pain control systems: brainstem spinal pathways and endorphin circuitry. Annu Rev Neurosci. (1984) 7:309–38. doi: 10.1146/annurev.ne.07.030184.001521
145. Carrive P. The periaqueductal gray and defensive behavior: functional representation and neuronal organization. Behav Brain Res. (1993) 58(1–2):27–47. doi: 10.1016/0166-4328(93)90088-8
146. Eippert F, Tracey I. Pain and the PaG: learning from painful mistakes. Nat Neurosci. (2014) 17:1438–9. doi: 10.1038/nn.3844
147. Huang J, Gadotti VM, Chen L, Souza IA, Huang S, Wang D, et al. A neuronal circuit for activating descending modulation of neuropathic pain. Nat Neurosci. (2019) 22(10):1659–68. doi: 10.1038/s41593-019-0481-5
148. Menant O, Andersson F, Zelena D, Chaillou E. The benefits of magnetic resonance imaging methods to extend the knowledge of the anatomical organisation of the periaqueductal gray in mammals. J Chem Neuroanat. (2016) 77:110–20. doi: 10.1016/j.jchemneu.2016.06.003
149. Reynolds DV. Surgery in the rat during electrical analgesia induced by focal brain stimulation. Science. (1969) 164(3878):444–5. doi: 10.1126/science.164.3878.444
150. Rozeske RR, Jercog D, Karalis N, Chaudun F, Khoder S, Girard D, et al. Prefrontal-periaqueductal gray-projecting neurons mediate context fear discrimination. Neuron. (2018) 97(4):898–910.e6. doi: 10.1016/j.neuron.2017.12.044
151. Uhelski ML, Morris-Bobzean SA, Dennis TS, Perrotti LI, Fuchs PN. Evaluating underlying neuronal activity associated with escape/avoidance behavior in response to noxious stimulation in adult rats. Brain Res. (2012) 1433:56–61. doi: 10.1016/j.brainres.2011.11.016
152. LaBuda CJ, Fuchs PN. Attenuation of negative pain affect produced by unilateral spinal nerve injury in the rat following anterior cingulate cortex activation. Neuroscience. (2005) 136(1):311–22. doi: 10.1016/j.neuroscience.2005.07.010
153. Hosobuchi Y, Adams JE, Rutkin B. Chronic thalamic stimulation for the control of facial anesthesia dolorosa. Arch Neurol. (1973) 29(3):158–61. doi: 10.1001/archneur.1973.00490270040005
154. Abreu V, Vaz R, Rebelo V, Rosas MJ, Chamadoira C, Gillies MJ, et al. Thalamic deep brain stimulation for neuropathic pain: efficacy at three years’ follow-up. Neuromodulation. (2017) 20(5):504–13. doi: 10.1111/ner.12620
155. Pereira EAC, Boccard SG, Linhares P, Chamadoira C, Rosas MJ, Abreu P, et al. Thalamic deep brain stimulation for neuropathic pain after amputation or brachial plexus avulsion. Neurosurg Focus. (2013) 35(3):E7. doi: 10.3171/2013.7.FOCUS1346
156. Katayama Y, Yamamoto T, Kobayashi K, Kasai M, Oshima H, Fukaya C. Motor cortex stimulation for phantom limb pain: comprehensive therapy with spinal cord and thalamic stimulation. Stereotact Funct Neurosurg. (2001) 77(1–4):159–62. doi: 10.1159/000064593
157. Yamamoto T, Katayama Y, Obuchi T, Kano T, Kobayashi K, Oshima H, et al. Thalamic sensory relay nucleus stimulation for the treatment of peripheral deafferentation pain. Stereotact Funct Neurosurg. (2006) 84(4):180–3. doi: 10.1159/000094958
158. Bittar RG, Otero S, Carter H, Aziz TZ. Deep brain stimulation for phantom limb pain. J Clin Neurosci. (2005) 12(4):399–404. doi: 10.1016/j.jocn.2004.07.013
159. Green A, Owen S, Davies P, Moir L, Aziz T. Deep brain stimulation for neuropathic cephalalgia. Cephalalgia. (2006) 26(5):561–7. doi: 10.1111/j.1468-2982.2005.01068.x
160. Green AL, Wang S, Owen SLF, Xie K, Bittar RG, Stein JF, et al. Stimulating the human midbrain to reveal the link between pain and blood pressure. Pain. (2006) 124(3):349–59. doi: 10.1016/j.pain.2006.05.005
161. Richardson DE. Pain reduction by electrical brain stimulation in man. J Neurosurg. (1977) 47:178–83. doi: 10.3171/jns.1977.47.2.0178
162. Nandi D, Smith H, Owen S, Joint C, Stein J, Aziz T. Peri-ventricular grey stimulation versus motor cortex stimulation for post stroke neuropathic pain. J Clin Neurosci. (2002) 9(5):557–61. doi: 10.1054/jocn.2001.1042
163. Lempka SF, Malone DA, Hu B, Baker KB, Wyant A, Ozinga JG, et al. Randomized clinical trial of deep brain stimulation for poststroke pain: DBS for pain. Ann Neurol. (2017) 81(5):653–63. doi: 10.1002/ana.24927
164. Hunsche S, Sauner D, Runge MJR, Lenartz D, El Majdoub F, Treuer H, et al. Tractography-guided stimulation of somatosensory fibers for thalamic pain relief. Stereotact Funct Neurosurg. (2013) 91(5):328–34. doi: 10.1159/000350024
165. Spooner J, Yu H, Kao C, Sillay K, Konrad P. Neuromodulation of the cingulum for neuropathic pain after spinal cord injury: case report. J Neurosurg. (2007) 107(1):169–72. doi: 10.3171/JNS-07/07/0169
166. Boccard SGJ, Fitzgerald JJ, Pereira EAC, Moir L, Van Hartevelt TJ, Kringelbach ML, et al. Targeting the affective component of chronic pain: a case series of deep brain stimulation of the anterior cingulate cortex. Neurosurgery. (2014) 74(6):628–37. doi: 10.1227/NEU.0000000000000321
167. Boccard SGJ, Prangnell SJ, Pycroft L, Cheeran B, Moir L, Pereira EAC, et al. Long-term results of deep brain stimulation of the anterior cingulate cortex for neuropathic pain. World Neurosurg. (2017) 106:625–37. doi: 10.1016/j.wneu.2017.06.173
168. Levi V, Cordella R, D’Ammando A, Tringali G, Dones I, Messina G, et al. Dorsal anterior cingulate cortex (ACC) deep brain stimulation (DBS): a promising surgical option for the treatment of refractory thalamic pain syndrome (TPS). Acta Neurochir. (2019) 161(8):1579–88. doi: 10.1007/s00701-019-03975-5
169. Louppe J-M, Nguyen J-P, Robert R, Buffenoir K, de Chauvigny E, Riant T, et al. Motor cortex stimulation in refractory pelvic and perineal pain: report of two successful cases. Neurourol Urodyn. (2013) 32(1):53–7. doi: 10.1002/nau.22269
170. Hollingworth M, Sims-Williams H, Pickering A, Barua N, Patel N. Single electrode deep brain stimulation with dual targeting at dual frequency for the treatment of chronic pain: a case series and review of the literature. Brain Sci. (2017) 7(12):9. doi: 10.3390/brainsci7010009
171. Abdallat M, Saryyeva A, Blahak C, Wolf ME, Weigel R, Loher TJ, et al. Centromedian–parafascicular and somatosensory thalamic deep brain stimulation for treatment of chronic neuropathic pain: a contemporary series of 40 patients. Biomedicines. (2021) 9(7):731. doi: 10.3390/biomedicines9070731
172. Boccard SGJ, Pereira EAC, Moir L, Aziz TZ, Green AL. Long-term outcomes of deep brain stimulation for neuropathic pain. Neurosurgery. (2013) 72(2):221–31. doi: 10.1227/NEU.0b013e31827b97d6
173. Owen SLF, Green AL, Nandi D, Bittar RG, Wang S, Aziz TZ. Deep brain stimulation for neuropathic pain. Neuromodulation. (2006) 9(2):100–6. doi: 10.1111/j.1525-1403.2006.00049.x
174. Owen SLF, Green AL, Stein JF, Aziz TZ. Deep brain stimulation for the alleviation of post-stroke neuropathic pain. Pain. (2006) 120(1–2):202–6. doi: 10.1016/j.pain.2005.09.035
175. Coffey RJ. Deep brain stimulation for chronic pain: results of two multicenter trials and a structured review. Pain Med. (2001) 2(3):183–92. doi: 10.1046/j.1526-4637.2001.01029.x
176. Gray AM, Pounds-Cornish E, Eccles FJR, Aziz TZ, Green AL, Scott RB. Deep brain stimulation as a treatment for neuropathic pain: a longitudinal study addressing neuropsychological outcomes. J Pain. (2014) 15(3):283–92. doi: 10.1016/j.jpain.2013.11.003
177. Hamani C, Schwalb JM, Rezai AR, Dostrovsky JO, Davis KD, Lozano AM. Deep brain stimulation for chronic neuropathic pain: long-term outcome and the incidence of insertional effect. Pain. (2006) 125(1):188–96. doi: 10.1016/j.pain.2006.05.019
178. Nandi D, Aziz T, Carter H, Stein J. Thalamic field potentials in chronic central pain treated by periventricular gray stimulation – a series of eight cases. Pain. (2003) 101(1):97–107. doi: 10.1016/S0304-3959(02)00277-4
179. Rasche D, Rinaldi PC, Young RF, Tronnier VM. Deep brain stimulation for the treatment of various chronic pain syndromes. Neurosurg Focus. (2006) 21(6):1–8. doi: 10.3171/foc.2006.21.6.10
180. Hosobuchi Y. Combined electrical stimulation of the periaqueductal gray matter and sensory thalamus. Stereotact Funct Neurosurg. (1983) 46(1–4):112–5. doi: 10.1159/000101249
181. Boccard SGJ, Pereira EAC, Aziz TZ. Deep brain stimulation for chronic pain. J Clin Neurosci. (2015) 22(10):1537–43. doi: 10.1016/j.jocn.2015.04.005
182. Ahmed MA, Mohamed SA, Sayed D. Long-term antalgic effects of repetitive transcranial magnetic stimulation of motor cortex and serum beta-endorphin in patients with phantom pain. Neurol Res. (2011) 33(9):953–8. doi: 10.1179/1743132811Y.0000000045
183. Boadas-Vaello P, Castany S, Homs J, Álvarez-Pérez B, Deulofeu M, Verdú E. Neuroplasticity of ascending and descending pathways after somatosensory system injury: reviewing knowledge to identify neuropathic pain therapeutic targets. Spinal Cord. (2016) 54(5):330–40. doi: 10.1038/sc.2015.225
184. Boyer L, Dousset A, Roussel P, Dossetto N, Cammilleri S, Piano V, et al. rTMS in fibromyalgia: a randomized trial evaluating QoL and its brain metabolic substrate. Neurology. (2014) 82:1231–8. doi: 10.1212/WNL.0000000000000280
185. Corlier J, Tadayonnejad R, Wilson AC, Lee JC, Marder KG, Ginder ND, et al. Repetitive transcranial magnetic stimulation treatment of major depressive disorder and comorbid chronic pain: response rates and neurophysiologic biomarkers. Psychol Med. (2023) 53:823–32. doi: 10.1017/S0033291721002178
186. Duncan GH, Bushnell CM, Marchand S. Deep brain stimulation: a review of basic research and clinical studies. Pain. (1991) 45(1):49–59. doi: 10.1016/0304-3959(91)90164-S
187. Farrell S, Green A, Aziz T. The current state of deep brain stimulation for chronic pain and its context in other forms of neuromodulation. Brain Sci. (2018) 8(8):158. doi: 10.3390/brainsci8080158
188. Galhardoni R, Correia GS, Araujo H, Yeng LT, Fernandes DT, Kaziyama HH, et al. Repetitive transcranial magnetic stimulation in chronic pain: a review of the literature. Arch Phys Med Rehabil. (2015) 96(4):S156–72. doi: 10.1016/j.apmr.2014.11.010
189. Gardner J. A history of deep brain stimulation: technological innovation and the role of clinical assessment tools. Soc Stud Sci. (2013) 43(5):707–28. doi: 10.1177/0306312713483678
190. Gybels J, Kupers R, Nuttin B. Therapeutic stereotactic procedures on the thalamus for pain. Acta Neurochir. (1993) 124(1):19–22. doi: 10.1007/BF01400709
191. Hirayama A, Saitoh Y, Kishima H, Shimokawa T, Oshino S, Hirata M, et al. Reduction of intractable deafferentation pain by navigation-guided repetitive transcranial magnetic stimulation of the primary motor cortex. Pain. (2006) 122(1):22–7. doi: 10.1016/j.pain.2005.12.001
192. Knotkova H, Hamani C, Sivanesan E, Le Beuffe MFE, Moon JY, Cohen SP, et al. Neuromodulation for chronic pain. Lancet. (2021) 397(10289):2111–24. doi: 10.1016/S0140-6736(21)00794-7
193. Lorenz J, Minoshima S, Casey KL. Keeping pain out of mind: the role of the dorsolateral prefrontal cortex in pain modulation. Brain. (2003) 126(5):1079–91. doi: 10.1093/brain/awg102
194. Lozano AM, Lipsman N. Probing and regulating dysfunctional circuits using deep brain stimulation. Neuron. (2013) 77(3):406–24. doi: 10.1016/j.neuron.2013.01.020
195. Mallory GW, Abulseoud O, Hwang S-C, Gorman DA, Stead SM, Klassen BT, et al. The nucleus accumbens as a potential target for central poststroke pain. Mayo Clin Proc. (2012) 87(10):1025–31. doi: 10.1016/j.mayocp.2012.02.029
196. Malone DA, Dougherty DD, Rezai AR, Carpenter LL, Friehs GM, Eskandar EN, et al. Deep brain stimulation of the ventral capsule/ventral striatum for treatment-resistant depression. Biol Psychiatry. (2009) 65(4):267–75. doi: 10.1016/j.biopsych.2008.08.029
197. Mark VH, Ervin FR. Role of thalamotomy in treatment of chronic severe pain. Postgrad Med. (1965) 37(5):563–71. doi: 10.1080/00325481.1965.11695514
198. Modell JG, Mountz JM, Curtis GC, Greden JF. Neurophysiologic dysfunction in basal ganglia/limbic striatal and thalamocortical circuits as a pathogenetic mechanism of obsessive-compulsive disorder. J Neuropsychiatry Clin Neurosci. (1989) 1:27–36. doi: 10.1176/jnp.1.1.27
199. Moisset X, Lanteri-Minet M, Fontaine D. Neurostimulation methods in the treatment of chronic pain. J Neural Transm. (2020) 127(4):673–86. doi: 10.1007/s00702-019-02092-y
200. Monosov IE, Haber SN, Leuthardt EC, Jezzini A. Anterior cingulate cortex and the control of dynamic behavior in primates. Curr Biol. (2020) 30(23):R1442–54. doi: 10.1016/j.cub.2020.10.009
201. Morecraft RJ, Stilwell-Morecraft KS, Cipolloni PB, Ge J, McNeal DW, Pandya DN. Cytoarchitecture and cortical connections of the anterior cingulate and adjacent somatomotor fields in the rhesus monkey. Brain Res Bull. (2012) 87(4–5):457–97. doi: 10.1016/j.brainresbull.2011.12.005
202. Morecraft RJ, Tanji J. Cingulofrontal interactions and the cingulate motor areas. In: Vogt BA, editor. Cingulate neurobiology and disease. New York: Oxford (2009). p. 113–44.
203. Nardone R, Höller Y, Langthaler PB, Lochner P, Golaszewski S, Schwenker K, et al. RTMS Of the prefrontal cortex has analgesic effects on neuropathic pain in subjects with spinal cord injury. Spinal Cord. (2017) 55(1):20–5. doi: 10.1038/sc.2016.87
204. Nüssel M, Zhao Y, Knorr C, Regensburger M, Stadlbauer A, Buchfelder M, et al. Deep brain stimulation, stereotactic radiosurgery and high-intensity focused ultrasound targeting the limbic pain matrix: a comprehensive review. Pain Ther. (2022) 11(2):459–76. doi: 10.1007/s40122-022-00381-1
205. Nuttin B, Cosyns P, Demeulemeester H, Gybels J, Meyerson B. Electrical stimulation in anterior limbs of internal capsules in patients with obsessive-compulsive disorder. Lancet. (1999) 354(9189):1526. doi: 10.1016/S0140-6736(99)02376-4
206. Ongur D. The organization of networks within the orbital and medial prefrontal cortex of rats, monkeys and humans. Cerebral Cortex. (2000) 10(3):206–19. doi: 10.1093/cercor/10.3.206
207. Parastarfeizabadi M, Kouzani AZ. Advances in closed-loop deep brain stimulation devices. J Neuroeng Rehabil. (2017) 14(1):79. doi: 10.1186/s12984-017-0295-1
208. Short BE, Borckardt JJ, Anderson BS, Frohman H, Beam W, Reeves ST, et al. Ten sessions of adjunctive left prefrontal rTMS significantly reduces fibromyalgia pain: a randomized, controlled pilot study. Pain. (2011) 152(11):2477–84. doi: 10.1016/j.pain.2011.05.033
209. Sims-Williams H, Matthews JC, Talbot PS, Love-Jones S, Brooks JC, Patel NK, et al. Deep brain stimulation of the periaqueductal gray releases endogenous opioids in humans. NeuroImage. (2017) 146:833–42. doi: 10.1016/j.neuroimage.2016.08.038
210. Son B, Kim D, Kim H, Lee S. Simultaneous trial of deep brain and motor cortex stimulation in chronic intractable neuropathic pain. Stereotact Funct Neurosurg. (2014) 92(4):218–26. doi: 10.1159/000362933
211. Taylor JJ, Borckardt JJ, George MS. Endogenous opioids mediate left dorsolateral prefrontal cortex rTMS-induced analgesia. Pain. (2012) 153(6):1219–25. doi: 10.1016/j.pain.2012.02.030
212. Yu R, Gollub RL, Spaeth R, Napadow V, Wasan A, Kong J. Disrupted functional connectivity of the periaqueductal gray in chronic low back pain. Neuroimage Clin. (2014) 6:100–8. doi: 10.1016/j.nicl.2014.08.019
213. Adams JE. Naloxone reversal of analgesia produced by brain stimulation in the human. Pain. (1976) 2(2):161–6. doi: 10.1016/0304-3959(76)90111-1
214. Akil H, Richardson DE, Hughes J, Barchas JD. Enkephalin-like material elevated in ventricular cerebrospinal fluid of pain patients after analgetic focal stimulation. Science. (1978) 201(4354):463–5. doi: 10.1126/science.663668
215. Vogt BA, Paxinos G. Cytoarchitecture of mouse and rat cingulate cortex with human homologies. Brain Struct Funct. (2014) 219(1):185–92. doi: 10.1007/s00429-012-0493-3
216. Foltz EL, White LE. Pain “relief” by frontal cingulumotomy. J Neurosurg. (1962) 19:89–100. doi: 10.3171/jns.1962.19.2.0089
217. Machado AG, Baker KB, Plow E, Malone DA. Cerebral stimulation for the affective component of neuropathic pain. Neuromodulation. (2013) 16(6):514–8. doi: 10.1111/j.1525-1403.2012.00517.x
218. Vachon-Presseau E, Tétreault P, Petre B, Huang L, Berger SE, Torbey S, et al. Corticolimbic anatomical characteristics predetermine risk for chronic pain. Brain. (2016) 139(7):1958–70. doi: 10.1093/brain/aww100
219. Tsubokawa T, Katayama Y, Yamamoto T, Hirayama T, Koyama S. Treatment of thalamic pain by chronic motor cortex stimulation. Pacing Clin Electrophysiol. (1991) 14(1):131–4. doi: 10.1111/j.1540-8159.1991.tb04058.x
220. Nguyen JP, Nizard J, Keravel Y, Lefaucheur JP. Invasive brain stimulation for the treatment of neuropathic pain. Nat Rev Neurol. (2011) 7(12):699–709. doi: 10.1038/nrneurol.2011.138
221. Nuti C, Peyron R, Garcia-Larrea L, Brunon J, Laurent B, Sindou M, et al. Motor cortex stimulation for refractory neuropathic pain: four year outcome and predictors of efficacy. Pain. (2005) 118(1):43–52. doi: 10.1016/j.pain.2005.07.020
222. Tsubokawa T, Katayama Y, Yamamoto T, Hirayama T, Koyama S. Chronic motor cortex stimulation in patients with thalamic pain. J Neurosurg. (1993) 78(3):393–401. doi: 10.3171/jns.1993.78.3.0393
223. André-Obadia N, Mertens P, Gueguen A, Peyron R, Garcia-Larrea L. Pain relief by rTMS: differential effect of current flow but no specific action on pain subtypes. Neurology. (2008) 71(11):833–40. doi: 10.1212/01.wnl.0000325481.61471.f0
224. Barker A, Jalinous R, Freeston I. Non-invasive magnetic stimulation of human motor cortex. Lancet. (1985) 1:1106–7. doi: 10.1515/eng-2018-0022
225. Polson MJR, Barker AT, Freeston IL. Stimulation of nerve trunks with time-varying magnetic fields. Med Biol Eng Comput. (1982) 20(2):243–4. doi: 10.1007/BF02441362
226. Lefaucheur JP, André-Obadia N, Antal A, Ayache SS, Baeken C, Benninger DH, et al. Evidence-based guidelines on the therapeutic use of repetitive transcranial magnetic stimulation (rTMS). Clin Neurophysiol. (2014) 125(11):2150–206. doi: 10.1016/j.clinph.2014.05.021
227. Bestmann S, Baudewig J, Siebner HR, Rothwell JC, Frahm J. Subthreshold high-frequency TMS of human primary motor cortex modulates interconnected frontal motor areas as detected by interleaved fMRI-TMS. NeuroImage. (2003) 20(3):1685–96. doi: 10.1016/j.neuroimage.2003.07.028
228. Garcia-Larrea L, Peyron R, Mertens P, Gregoire MC, Lavenne F, Bonnefoi F, et al. Positron emission tomography during motor cortex stimulation for pain control. Stereotact Funct Neurosurg. (1997) 68:141–8. doi: 10.16309/j.cnki.issn.1007-1776.2003.03.004
229. Pagano RL, Fonoff ET, Dale CS, Ballester G, Teixeira MJ, Britto LRG. Motor cortex stimulation inhibits thalamic sensory neurons and enhances activity of PAG neurons: possible pathways for antinociception. Pain. (2012) 153(12):2359–69. doi: 10.1016/j.pain.2012.08.002
230. Maarrawi J, Peyron R, Mertens P, Costes N, Magnin M, Sindou M, et al. Motor cortex stimulation for pain control induces changes in the endogenous opioid system. Neurology. (2007) 69(9):827–34. doi: 10.1212/01.wnl.0000269783.86997.37
231. De Andrade DC, Mhalla A, Adam F, Texeira MJ, Bouhassira D. Neuropharmacological basis of rTMS-induced analgesia: the role of endogenous opioids. Pain. (2011) 152(2):320–6. doi: 10.1016/j.pain.2010.10.032
232. Hosobuchi Y, Adams JE, Linchitz R. Pain relief by electrical stimulation of the central gray matter in humans and its reversal by naloxone. Science. (1977) 197(4299):183–6. doi: 10.1126/science.301658
233. Lefaucheur JP, Hatem S, Nineb A, Ménard-Lefaucheur I, Wendling S, Keravel Y, et al. Somatotopic organization of the analgesic effects of motor cortex rTMS in neuropathic pain. Neurology. (2006) 67(11):1998–2004. doi: 10.1212/01.wnl.0000247138.85330.88
234. Lefaucheur J-P, Drouot X, Menard-Lefaucheur I, Zerah F, Bendib B, Cesaro P, et al. Neurogenic pain relief by repetitive transcranial magnetic cortical stimulation depends on the origin and the site of pain. J Neurol Neurosurg Psychiatry. (2004) 75:612–6. doi: 10.1136/jnnp.2003.022236
235. Che X, Cash RFH, Luo X, Luo H, Lu X, Xu F, et al. High-frequency rTMS over the dorsolateral prefrontal cortex on chronic and provoked pain: a systematic review and meta-analysis. Brain Stimul. (2021) 14(5):1135–46. doi: 10.1016/j.brs.2021.07.004
236. Leung A, Metzger-Smith V, He Y, Cordero J, Ehlert B, Song D, et al. Left dorsolateral prefrontal Cortex rTMS in alleviating MTBI related headaches and depressive symptoms. Neuromodulation. (2018) 21(4):390–401. doi: 10.1111/ner.12615
237. Tzabazis A, Aparici CM, Rowbotham MC, Schneider MB, Etkin A, Yeomans DC. Shaped magnetic field pulses by multi-coil repetitive transcranial magnetic stimulation (rTMS) differentially modulate anterior cingulate cortex responses and pain in volunteers and fibromyalgia patients. Mol Pain. (2013) 9(1):1–9. doi: 10.1186/1744-8069-9-33
238. Galhardoni R, Da Silva VA, García-Larrea L, Dale C, Baptista AF, Barbosa LM, et al. Insular and anterior cingulate cortex deep stimulation for central neuropathic pain disassembling the percept of pain. Neurology. (2019) 92(18):E2165–75. doi: 10.1212/WNL.0000000000007396
239. Fox MD, Buckner RL, White MP, Greicius MD, Pascual-Leone A. Efficacy of transcranial magnetic stimulation targets for depression is related to intrinsic functional connectivity with the subgenual cingulate. Biol Psychiatry. (2012) 72(7):595–603. doi: 10.1016/j.biopsych.2012.04.028
240. George MS, Wassermann EM, Williams WA, Callahan A, Ketter TA, Basser P, et al. Daily repetitive transcranial magnetic stimulation (rTMS) improves mood in depression. Neuroreport. (1995) 6(14):1853–6. doi: 10.1097/00001756-199510020-00008
241. Padmanabhan JL, Cooke D, Joutsa J, Siddiqi SH, Ferguson M, Darby RR, et al. A human depression circuit derived from focal brain lesions. Biol Psychiatry. (2019) 86(10):749–58. doi: 10.1016/j.biopsych.2019.07.023
242. Goldman-Rakic PS. Architecture of the prefrontal cortex and the central executive. Ann N Y Acad Sci. (1995) 769(1):71–84. doi: 10.1111/j.1749-6632.1995.tb38132.x
243. Mueller S, Wang D, Fox MD, Yeo BTT, Sepulcre J, Sabuncu MR, et al. Individual variability in functional connectivity architecture of the human brain. Neuron. (2013) 77(3):586–95. doi: 10.1016/j.neuron.2012.12.028
244. Beam W, Borckardt JJ, Reeves ST, George MS. An efficient and accurate new method for locating the F3 position for prefrontal TMS applications. Brain Stimul. (2009) 2(1):50–4. doi: 10.1016/j.brs.2008.09.006
245. Mir-Moghtadaei A, Caballero R, Fried P, Fox MD, Lee K, Giacobbe P, et al. Concordance between BeamF3 and MRI-neuronavigated target sites for repetitive transcranial magnetic stimulation of the left dorsolateral prefrontal cortex. Brain Stimul. (2015) 8(5):965–73. doi: 10.1016/j.brs.2015.05.008
246. Iwabuchi SJ, Auer DP, Lankappa ST, Palaniyappan L. Baseline effective connectivity predicts response to repetitive transcranial magnetic stimulation in patients with treatment-resistant depression. Eur Neuropsychopharmacol. (2019) 29(5):681–90. doi: 10.1016/j.euroneuro.2019.02.012
247. Drevets WC, Price JL, Simpson JR, Todd RD, Reich T, Vannier M, et al. Subgenual prefrontal cortex abnormalities in mood disorders. Nature. (1997) 386:824–7. doi: 10.1038/386824a0
248. Hiser J, Koenigs M. The multifaceted role of the ventromedial prefrontal cortex in emotion, decision making, social cognition, and psychopathology. Biol Psychiatry. (2018) 83(8):638–47. doi: 10.1016/j.biopsych.2017.10.030
249. Fox MD. Mapping symptoms to brain networks with the human connectome. N Engl J Med. (2018) 379(23):2237–45. doi: 10.1056/NEJMra1706158
250. Cremers HR, Wager TD, Yarkoni T. The relation between statistical power and inference in fMRI. PloS One. (2017) 12(11):e0184923. doi: 10.1371/journal.pone.0184923
251. Grady CL, Rieck JR, Nichol D, Rodrigue KM, Kennedy KM. Influence of sample size and analytic approach on stability and interpretation of brain-behavior correlations in task-related fMRI data. Hum Brain Mapp. (2021) 42(1):204–19. doi: 10.1002/hbm.25217
252. Marek S, Tervo-Clemmens B, Calabro FJ, Montez DF, Kay BP, Hatoum AS, et al. Reproducible brain-wide association studies require thousands of individuals. Nature. (2022) 603:654–60. doi: 10.1038/s41586-022-04492-9
253. Boes AD, Prasad S, Liu H, Liu Q, Pascual-Leone A, Caviness VS Jr, et al. Network localization of neurological symptoms from focal brain lesions. Brain. (2015) 138(10):3061–75. doi: 10.1093/brain/awv228
254. Joutsa J, Moussawi K, Siddiqi SH, Abdolahi A, Drew W, Cohen AL, et al. Brain lesions disrupting addiction map to a common human brain circuit. Nat Med. (2022) 28:1249–55. doi: 10.1038/s41591-022-01834-y
255. Fox MD, Liu H, Pascual-Leone A. Identification of reproducible individualized targets for treatment of depression with TMS based on intrinsic connectivity. NeuroImage. (2013) 66:151–60. doi: 10.1016/j.neuroimage.2012.10.082
256. Lynch CJ, Power JD, Scult MA, Dubin M, Gunning FM, Liston C. Rapid precision functional mapping of individuals using multi-echo fMRI. Cell Rep. (2020) 33(12):108540. doi: 10.1016/j.celrep.2020.108540
257. Lynch CJ, Breeden AL, Gordon EM, Cherry JBC, Turkeltaub PE, Vaidya CJ. Precision inhibitory stimulation of individual-specific cortical hubs disrupts information processing in humans. Cerebral Cortex. (2019) 29(9):3912–21. doi: 10.1093/cercor/bhy270
258. Elliott ML, Knodt AR, Ireland D, Morris ML, Poulton R, Ramrakha S, et al. What is the test-retest reliability of common task-functional MRI measures? New empirical evidence and a meta-analysis. Psychol Sci. (2020) 31(7):792–806. doi: 10.1177/0956797620916786
259. Vul E, Harris C, Winkielman P, Pashler H. Puzzlingly high correlations in fMRI studies of emotion, personality, and social cognition. Perspect Psychol Sci. (2009) 4(3):274–90. doi: 10.1111/j.1745-6924.2009.01125.x
260. Vul E, Pashler H. Voodoo and circularity errors. NeuroImage. (2012) 62(2):945–8. doi: 10.1016/j.neuroimage.2012.01.027
261. Yarkoni T. The generalizability crisis. Behav Brain Sci. (2022) 45:e1. doi: 10.1017/S0140525X20001685
262. Ince RAA, Kay JW, Schyns PG. Within-participant statistics for cognitive science. Trends Cogn Sci. (2022) 26(8):626–30. doi: 10.1016/j.tics.2022.05.008
263. Thiebaut de Schotten M, Shallice T. Identical, similar or different? Is a single brain model sufficient? Cortex. (2017) 86:172–5. doi: 10.1016/j.cortex.2016.12.002
264. Sack AT, Cohen Kadosh R, Schuhmann T, Moerel M, Walsh V, Goebel R. Optimizing functional accuracy of TMS in cognitive studies: a comparison of methods. J Cogn Neurosci. (2009) 21(2):207–21. doi: 10.1162/jocn.2009.21126
265. Mayberg HS, Lozano AM, Voon V, McNeely HE, Seminowicz D, Hamani C, et al. Deep brain stimulation for treatment-resistant depression. Neuron. (2005) 45(5):651–60. doi: 10.1016/j.neuron.2005.02.014
266. McNeely HE, Mayberg HS, Lozano AM, Kennedy SH. Neuropsychological impact of Cg25 deep brain stimulation for treatment-resistant depression: preliminary results over 12 months. J Nerv Ment Dis. (2008) 196(5):405–10. doi: 10.1097/NMD.0b013e3181710927
267. Riva-Posse P, Choi KS, Holtzheimer PE, McIntyre CC, Gross RE, Chaturvedi A, et al. Defining critical white matter pathways mediating successful subcallosal cingulate deep brain stimulation for treatment-resistant depression. Biol Psychiatry. (2014) 76(12):963–9. doi: 10.1016/j.biopsych.2014.03.029
268. Jung J, Bungert A, Bowtell R, Jackson SR. Modulating brain networks with transcranial magnetic stimulation over the primary motor cortex: a concurrent TMS/fMRI study. Front Hum Neurosci. (2020) 14:31. doi: 10.3389/fnhum.2020.00031
269. Cocchi L, Zalesky A. Personalized transcranial magnetic stimulation in psychiatry. Biol Psychiatry Cogn Neurosci Neuroimaging. (2018) 3(9):731–41. doi: 10.1016/j.bpsc.2018.01.008
270. Dubin MJ, Liston C, Avissar MA, Ilieva I, Gunning FM. Network-guided transcranial magnetic stimulation for depression. Curr Behav Neurosci Rep. (2017) 4(1):70–7. doi: 10.1007/s40473-017-0108-7
271. Bassett DS, Sporns O. Network neuroscience. Nat Neurosci. (2017) 20(3):353–64. doi: 10.1038/nn.4502
272. Bertolero MA, Yeo BTT, D’Esposito M. The modular and integrative functional architecture of the human brain. Proc Natl Acad Sci U S A. (2015) 112(49):E6798–807. doi: 10.1073/pnas.1510619112
273. Bullmore E, Sporns O. Complex brain networks: graph theoretical analysis of structural and functional systems. Nat Rev Neurosci. (2009) 10:186–98. doi: 10.1038/nrn2575
274. Guimerà R, Amaral LA. Cartography of complex networks: modules and universal roles. J Stat Mech. (2005) 2005(P02001):nihpa35573. doi: 10.1088/1742-5468/2005/02/P02001
275. Jeong H, Mason SP, Barabási A-L, Oltvai ZN. Lethality and centrality in protein networks. Nature. (2001) 411:41–2. doi: 10.1038/35075138
276. Sporns O, Betzel RF. Modular brain networks. Annu Rev Psychol. (2016) 67:613–40. doi: 10.1146/annurev-psych-122414-033634
277. Rubinov M, Sporns O. Complex network measures of brain connectivity: uses and interpretations. NeuroImage. (2010) 52(3):1059–69. doi: 10.1016/j.neuroimage.2009.10.003
278. Bassett DS, Porter MA, Wymbs NF, Grafton ST, Carlson JM, Mucha PJ. Robust detection of dynamic community structure in networks. Chaos. (2013) 23(1):013142. doi: 10.1063/1.4790830
279. Lenoir D, Cagnie B, Verhelst H, Pauw RD. Graph measure based connectivity in chronic pain patients: a systematic review. Pain Physician. (2021) 24:E1037–58.34704714
280. Bertolero MA, Yeo BTT, D’Esposito M. The diverse club. Nat Commun. (2017) 8:1277. doi: 10.1038/s41467-017-01189-w
281. Newman MEJ. Modularity and community structure in networks. Proc Natl Acad Sci U S A. (2006) 103(23):8577–82. doi: 10.1073/pnas.0601602103
282. Blondel VD, Guillaume J-L, Lambiotte R, Lefebvre E. Fast unfolding of communities in large networks. J Stat Mech. (2008) 2008(10):10008. doi: 10.1088/1742-5468/2008/10/P10008
283. Power JD, Schlaggar BL, Lessov-Schlaggar CN, Petersen SE. Evidence for hubs in human functional brain networks. Neuron. (2013) 79(4):798–813. doi: 10.1016/j.neuron.2013.07.035
284. Gallen CL, D’Esposito M. Brain modularity: a biomarker of intervention-related plasticity. Trends Cogn Sci. (2019) 23(4):293–304. doi: 10.1016/j.tics.2019.01.014
285. Bertolero MA, Yeo BTT, Bassett DS, D’Esposito M. A mechanistic model of connector hubs, modularity and cognition. Nat Hum Behav. (2018) 2(10):765–77. doi: 10.1038/s41562-018-0420-6
286. Bassett DS, Wymbs NF, Porter MA, Mucha PJ, Carlson JM, Grafton ST. Dynamic reconfiguration of human brain networks during learning. Proc Natl Acad Sci U S A. (2011) 108(18):7641–6. doi: 10.1073/pnas.1018985108
287. Bassett DS, Yang M, Wymbs NF, Grafton ST. Learning-induced autonomy of sensorimotor systems. Nat Neurosci. (2015) 18:744–51. doi: 10.1038/nn.3993
288. Gratton C, Nomura EM, Pérez F, D’Esposito M. Focal brain lesions to critical locations cause widespread disruption of the modular organization of the brain. J Cogn Neurosci. (2012) 24(6):1275–85. doi: 10.1162/jocn_a_00222
289. Crossley NA, Mechelli A, Scott J, Carletti F, Fox PT, McGuire P, et al. The hubs of the human connectome are generally implicated in the anatomy of brain disorders. Brain. (2014) 137(8):2382–95. doi: 10.1093/brain/awu132
290. Fauchon C, Meunier D, Faillenot I, Pomares FB, Bastuji H, Garcia-Larrea L, et al. The modular organization of pain brain networks: an fMRI graph analysis informed by intracranial EEG. Cereb Cortex Commun. (2020) 1(1):tgaa088. doi: 10.1093/texcom/tgaa088
291. De Pauw R, Aerts H, Siugzdaite R, Meeus M, Coppieters I, Caeyenberghs K, et al. Hub disruption in patients with chronic neck pain: a graph analytical approach. Pain. (2020) 161(4):729–41. doi: 10.1097/j.pain.0000000000001762
292. Mansour A, Baria AT, Tetreault P, Vachon-Presseau E, Chang PC, Huang L, et al. Global disruption of degree rank order: a hallmark of chronic pain. Sci Rep. (2016) 6:34853. doi: 10.1038/srep34853
293. Guimerà R, Nunes Amaral LA. Functional cartography of complex metabolic networks. Nature. (2005) 433:895–900. doi: 10.1038/nature03288
294. Edwards RR, Dworkin RH, Turk DC, Angst MS, Dionne R, Freeman R, et al. Patient phenotyping in clinical trials of chronic pain treatments: IMMPACT recommendations. Pain. (2016) 157(9):1851–71. doi: 10.1097/j.pain.0000000000000602
295. Smith SM, Dworkin RH, Turk DC, Baron R, Polydefkis M, Tracey I, et al. The potential role of sensory testing, skin biopsy, and functional brain imaging as biomarkers in chronic pain clinical trials: IMMPACT considerations. J Pain. (2017) 18(7):757–77. doi: 10.1016/j.jpain.2017.02.429
296. Kao C-H, Khambhati AN, Bassett DS, Nassar MR, McGuire JT, Gold JI, et al. Functional brain network reconfiguration during learning in a dynamic environment. Nat Commun. (2020) 11:1682. doi: 10.1038/s41467-020-15442-2
297. Khambhati AN, Mattar MG, Wymbs NF, Grafton ST, Bassett DS. Beyond modularity: fine-scale mechanisms and rules for brain network reconfiguration. NeuroImage. (2018) 166:385–99. doi: 10.1016/j.neuroimage.2017.11.015
298. Khambhati AN, Medaglia JD, Karuza EA, Thompson-Schill SL, Bassett DS. Subgraphs of functional brain networks identify dynamical constraints of cognitive control. PLoS Comput Biol. (2018) 14(7):e1006234. doi: 10.1371/journal.pcbi.1006234
299. Gu S, Pasqualetti F, Cieslak M, Telesford QK, Yu AB, Kahn AE, et al. Controllability of structural brain networks. Nat Commun. (2015) 6:8414. doi: 10.1038/ncomms9414
300. Liu Y-Y, Slotine J-J, Barabási A-L. Controllability of complex networks. Nature. (2011) 473:167–73. doi: 10.1038/nature10011
301. Pasqualetti F, Zampieri S, Bullo F. Controllability metrics, limitations and algorithms for complex networks. IEEE Trans Control Netw Syst. (2014) 1(1):40–52. doi: 10.1109/TCNS.2014.2310254
302. Stiso J, Khambhati AN, Menara T, Kahn AE, Stein JM, Das SR, et al. White matter network architecture guides direct electrical stimulation through optimal state transitions. Cell Rep. (2019) 28(10):2554–2566.e7. doi: 10.1016/j.celrep.2019.08.008
303. Dale A, Jernigan T, Brown S. Adolescent brain cognitive development (ABCD) neuroimaging parameters. Imaging protocols (2023). Available at: https://abcdstudy.org/images/Protocol_Imaging_Sequences.pdf.
304. Soares JM, Magalhães R, Moreira PS, Sousa A, Ganz E, Sampaio A, et al. A Hitchhiker’s guide to functional magnetic resonance imaging. Front Neurosci. (2016) 10. doi: 10.3389/fnins.2016.00515
305. Esteban O, Markiewicz CJ, Blair RW, Moodie CA, Isik AI, Erramuzpe A, et al. fMRIPrep: a robust preprocessing pipeline for functional MRI. Nat Methods. (2019) 16(1):111–6. doi: 10.1038/s41592-018-0235-4
306. Gorgolewski KJ, Auer T, Calhoun VD, Craddock RC, Das S, Duff EP, et al. The brain imaging data structure, a format for organizing and describing outputs of neuroimaging experiments. Sci Data. (2016) 3:160044. doi: 10.1038/sdata.2016.44
307. Poldrack RA, Baker CI, Durnez J, Gorgolewski KJ, Matthews PM, Munafò MR, et al. Scanning the horizon: towards transparent and reproducible neuroimaging research. Nat Rev Neurosci. (2017) 18(2):115–26. doi: 10.1038/nrn.2016.167
308. Lawrence RM, Bridgeford EW, Myers PE, Arvapalli GC, Ramachandran SC, Pisner DA, et al. Standardizing human brain parcellations. Sci Data. (2021) 8:78. doi: 10.1038/s41597-021-00849-3
309. Poldrack RA, Fletcher PC, Henson RN, Worsley KJ, Brett M, Nichols TE. Guidelines for reporting an fMRI study. Neuroimage. (2008) 40(2):409–14. doi: 10.1016/j.neuroimage.2007.11.048
310. Davis KD, Aghaeepour N, Ahn AH, Angst MS, Borsook D, Brenton A, et al. Discovery and validation of biomarkers to aid the development of safe and effective pain therapeutics: challenges and opportunities. Nat Rev Neurol. (2020) 16:381–400. doi: 10.1038/s41582-020-0362-2
311. Ten Brinke TR, Arnts H, Schuurman R, van den Munckhof P. Directional sensory thalamus deep brain stimulation in poststroke refractory pain. BMJ Case Rep. (2020) 13:e233254. doi: 10.1136/bcr-2019-233254
312. Ray CD, Burton CV. Deep brain stimulation for severe, chronic pain. Acta Neurochir Suppl (Wien). (1980) 30:289–93. doi: 10.1007/978-3-7091-8592-6_35
Keywords: neuromodulation, chronic pain, network neuroscience, graph theory, precision medicine, pain, deep brain stimulation (DBS), transcranial magnetic stimulation (TMS)
Citation: Motzkin JC, Kanungo I, D’Esposito M and Shirvalkar P (2023) Network targets for therapeutic brain stimulation: towards personalized therapy for pain. Front. Pain Res. 4:1156108. doi: 10.3389/fpain.2023.1156108
Received: 1 February 2023; Accepted: 19 May 2023;
Published: 8 June 2023.
Edited by:
Rocco Cavaleri, Western Sydney University, AustraliaReviewed by:
Scott Holmes, Harvard Medical School, United StatesKazuaki Yamamoto, Toronto Western Hospital, Canada
© 2023 Motzkin, Kanungo, D'Esposito and Shirvalkar. This is an open-access article distributed under the terms of the Creative Commons Attribution License (CC BY). The use, distribution or reproduction in other forums is permitted, provided the original author(s) and the copyright owner(s) are credited and that the original publication in this journal is cited, in accordance with accepted academic practice. No use, distribution or reproduction is permitted which does not comply with these terms.
*Correspondence: Prasad Shirvalkar cHJhc2FkLnNoaXJ2YWxrYXJAdWNzZi5lZHU=