- 1Department of Neurology, University of North Carolina at Chapel Hill School of Medicine, Chapel Hill, NC, United States
- 2Department of Anesthesiology, University of California, San Diego, San Diego, CA, United States
- 3Department of Psychiatry, San Francisco Weill Institute for Neurosciences, University of California, San Francisco, San Francisco, CA, United States
- 4Department of Psychiatry, University of California, San Diego, San Diego, CA, United States
- 5Department of Neurosciences, University of California, San Diego, San Diego, CA, United States
- 6Department of Psychiatry, San Diego & Center of Excellence in Stress and Mental Health, Veteran Affairs Health Care System, University of California, San Diego, San Diego, CA, United States
- 7Department of Psychiatry, San Diego & San Diego VA Medical Center, University of California, San Diego, San Diego, CA, United States
Chronic pain affects ~10–20% of the U.S. population with an estimated annual cost of $600 billion, the most significant economic cost of any disease to-date. Neuropathic pain is a type of chronic pain that is particularly difficult to manage and leads to significant disability and poor quality of life. Pain biomarkers offer the possibility to develop objective pain-related indicators that may help diagnose, treat, and improve the understanding of neuropathic pain pathophysiology. We review neuropathic pain mechanisms related to opiates, inflammation, and endocannabinoids with the objective of identifying composite biomarkers of neuropathic pain. In the literature, pain biomarkers typically are divided into physiological non-imaging pain biomarkers and brain imaging pain biomarkers. We review both types of biomarker types with the goal of identifying composite pain biomarkers that may improve recognition and treatment of neuropathic pain.
Introduction
Pain is a protective evolutionary function that involves “unpleasant sensory and emotional experiences associated with, or resembling that associated with, actual or potential tissue damage” (International Association for Study of Pain). Acute pain is an adaptive and essential survival behavior. Chronic pain is a pathological condition that poses a significant clinical, economic and social burden (1, 2). Chronic pain is the most common clinical complaint in the United States affecting ~10–20% of the U.S. population with an estimated annual cost of $600 billion, the most significant economic cost of any disease to-date (2–6).
Neuropathic pain is defined as pain that is “initiated or caused by a primary lesion or dysfunction in the nervous system” (7). Neuropathic pain can be divided into either having peripheral origin or central origin and can be further divided into acute or chronic pain, the latter defined as pain lasting for longer than 3 months (7).
Pain is a subjective sensory experience that cannot be directly measured nor quantified. Although pain is subjective and influenced by many physiological and psychological factors, measuring biomarkers of neuropathic pain provides an opportunity to identify objective markers of peripheral nerve damage and other pathology contributing to neuropathic pain. If used in combination, biomarkers related to pain mechanisms (including opiate, inflammation, and endocannabinoid mechanisms) offer the possibility to develop objective pain-related indicators that may improve diagnosis, treatment, and understanding of pain pathophysiology (8–11). The pursuit of pain biomarkers has followed two largely separate general directions: physiological vs. brain neuroimaging. Physiological pain biomarkers research has followed multiple lines of investigation including genetic, vesicular micro-RNA, metabolic/molecular, and stress markers. Neuroimaging biomarker research in neuropathic pain research was initially motivated by research into brain areas activated by painful stimuli and that vary with pain severity (10, 12–14). Brain activity that occurs in response to pain can also be observed in the absence of pain, which has led to conflicting evidence regarding brain activity related to pain. Thus, some researchers are developing biomarkers based on the mechanisms underlying pain and pain perception and biomarkers that may predict response to medication and pain treatments allowing for prediction of personalized treatment responses (10, 15, 16).
Toward the goal of identifying composite biomarkers for investigating neuropathic pain mechanisms and improving diagnosis and treatment response, we present a review of non-imaging and imaging pain biomarkers related to various neuropathic pain mechanisms, including opiate, inflammation, endocannabinoid mechanisms. In this review, we review mechanisms for neuropathic pain in general, but we focus on pain biomarkers for different types of peripheral neuropathies. Although various reviews of pain biomarkers exist, we focus on creating composite biomarkers through machine learning approaches that can most accurately identify people with neuropathic pain.
Opioid Pain Biomarkers
Opioid Mechanisms
Endogenous opioids are necessary for the expression of pain relief (17) and pain-induced aversion (18, 19). Blocking opioidergic transmission reduces dopamine release in the nucleus accumbens that accompanies pain relief (20). The endogenous opioid system consists of four opioid peptide families: β-endorphin, enkephalins, dynorphins, and nociceptin/orphanin and 4 families of receptors: mu, delta, kappa, and nociceptin (21, 22).
Opioid receptors are expressed by central and peripheral neurons, by neuroendocrine (pituitary, adrenals), immune, and ectodermal cells (23–25). All opioid receptor types mediate analgesia but have differing side effects, mostly due to their variable regional expression and functional activity in different parts of central and peripheral organ systems. Endogenous opioids are particularly concentrated in circuits involved in pain modulation (26).
Opioid Pain Biomarkers
Beta-endorphin levels in the CSF, blood and saliva have been investigated as possible pain biomarkers. Plasma Beta-endorphin has been used to investigate age responses to experimental pain (27). Patients with chronic neuropathic pain due to trauma or surgery have been shown to have lower levels of Beta-endorphin in the CSF (28). Plasma and CSF Beta-endorphin have been investigated in patients with trigeminal neuralgia (29). Interestingly, Beta-endorphin in peripheral blood was related to levels in CSF; furthermore, the levels of Beta-endorphin were inversely correlated with the severity of pain symptoms (29). While chronic low back pain typically involves non-neuropathic pain mechanisms, it is interesting that plasma Beta-endorphin levels have been shown to be a promising biomarker for chronic back pain (30). In other non-neuropathic pain conditions, mu opioid receptors expressed on immune B cells was found to be a biomarker for chronic pain in fibromyalgia and osteoarthritis. In this study, the percentage of mu opioid receptors positive B cells was statistically lower in patients with moderate to severe pain than in pain-free subjects or mild pain subjects (31). In a heterogenous group of patients with pain, a composite biomarker was identified that uses emergent properties in genetics to separate patients with pain requiring extremely high opioid doses from controls (32). Negative studies for opiate mechanism pain biomarkers have shown that salivary Beta-endorphin is not a biomarker for neuropathic chronic pain propensity (33).
Functional brain imaging performed on patients with non-neuropathic primary dysmenorrhea with mu-opioid receptor A118G polymorphism has been used to investigate pain sensitivity and opioid-analgesic treatment related to function in the descending pain modulatory system. Specifically, the functional connectivity of the descending pain modulatory system dependence upon mu-opioid receptor A118G polymorphisms was investigated. This study found that patient groups with different alleles for the A118G polymorphisms exhibited varying functional connectivity between the anterior cingulate cortex and periaqueductal gray (34).
Although magnetic resonance imaging provides information regarding structural and metabolic changes that provide insight into pain perception of the CNS, magnetic resonance imaging cannot image opioid function in cells in vivo at the molecular level. Such important opioid function information can be obtained through positron emission tomography and can be used to investigate pain opioid mechanisms (35).
Inflammation Pain Biomarkers
Peripheral Neuroinflammation Mechanisms
While the development of neuropathic pain has long been ascribed to the known contributors of central sensitization (action potential kinetics, phenotypic transformation, receptor density zone reorganization and long-term potentiation), the role of neuroinflammation regarding the initiation and maintenance of neuropathic pain has evolved tremendously over the last decade. Pro-inflammatory cytokines have been implicated in the generation of neuropathic pain states at both peripheral and central nervous system sites (36, 37). Neuroinflammation of the peripheral nervous system is triggered by inciting damage to the peripheral nerves, either by trauma, metabolic disturbances (i.e., diabetes), viral infection (i.e., HIV) or surgical lesions leading to sprouting of new pain-sensitive fibers (such as A-β fibers), excessive neuronal firing, and hypersensitization of primary afferent peripheral neurons. During a peripheral nerve injury, local cytokines recruits macrophages which secrete components of the complement cascade, coagulation factors, proteases, hydrolases, interferons, and other cytokines that ultimately facilitate degradation and phagocytosis of the pathogen and injured tissue. Peripheral neuroinflammatory mechanisms affect the damaged neuron and neighboring afferent neurons sharing the same innervation territory (38–45).
Central Neuroinflammation Mechanisms
Peripheral nerve injury causes neuroinflammation in the spinal cord (41, 46–48). The neuroinflammation is triggered by hyperactivity of the injured primary afferent peripheral sensory neuron which increases neurotransmitters and neuromodulators, causing hyperactivity of postsynaptic nociceptive neuronal hyperactivity as well as the release of several inflammatory activators. A result of this lumbar spinal inflammation process is disruption of the blood-spinal cord barrier leading to increased permeability, which then leads to infiltration of immune cells such as T lymphocytes, macrophages, mast cells, and neutrophils from the periphery into the spinal cord and dorsal root ganglion (46). These mechanisms contribute to further release of inflammatory mediators which contribute to alterations in post-synaptic receptors. This neurotransmitter increase leads to hyperactivity of post-synaptic nociceptive neurons in the spinal cord and altered signaling up to the thalamus and cortex that may contribute to central sensitization and pain hypersensitivity (41, 45, 49). Nerve injury typically involves neuro-immune interaction involving glia (50–52). Glia are known to provide functional microenvironment modulating neuronal signal transduction, synaptic pruning, and neuroplasticity that contributes to central sensitization.
Inflammation Pain Biomarkers
Cytokines as Pain Biomarkers
Concentrations of CSF proinflammatory cytokines are increased in multiple neuropathic pain states (53). Most studies of pain syndromes have found elevations of proinflammatory and anti-inflammatory cytokines in painful conditions compared with healthy controls; furthermore, frequently higher levels of proinflammatory markers are associated with greater pain (54). Other markers such as soluble intercellular adhesion molecule-1 (sICAM-1), for example, have also been demonstrated to correlate with pain. sICAM-1 measured in serum correlates with patients' self-reported pain levels in various pain conditions (back pain, polyneuropathy, post-herpetic neuralgia, orofacial pain, mixed pain, and musculoskeletal pain), distinguishing these patients from those with no or mild pain (55).
Cytokines in Peripheral Neuropathy
Cytokines have also been demonstrated to be potent mediators of pain in peripheral neuropathy. In one peripheral neuropathy study, gene expression of pro- and anti-inflammatory cytokines was shown to be increased in patients compared to controls (56). Another study found neuropathic pain group was found to have higher serum levels of several markers including C-Reactive Protein (CRP) and Tumor Necrosis Factor (TNF)-α compared with two control groups. Furthermore, patients with painful neuropathy had higher sICAM-1 and CRP levels when compared to painless neuropathy (57). A meta-analysis comprehensively assessed the relationship between serum TNF-α levels and diabetic peripheral neuropathy in patients with type 2 diabetes, demonstrating increased serum TNF-α levels in patients with diabetic neuropathy compared to type 2 diabetic patients without neuropathy and compared with controls (58). Il-17 is significantly upregulated in rat models of neuropathic pain, and mRNA expression levels of IL-1β and IL-6 are significantly enhanced in the spinal dorsal horn compared with controls (59). Moreover, functional recovery from neuropathic pain following a peripheral nerve injury relies on downregulation of IL-1 β and TNF- α responses (60).
Substance P and Neuropeptides Pain Biomarkers
Another key pro-inflammatory neuropeptide, Substance P, is known to initiate biological inflammatory effects (61). In painful trigeminal neuralgia, levels of Substance P and other neuropeptides (CGRP and VIP) in the cerebrospinal fluid and blood of patients were found to have higher levels than that of controls; furthermore, blood levels of these markers correlated with those of the CSF (29). Another study investigating non-neuropathic experimental pain found altered substance P levels and dynamics when comparing older and younger adults (27).
Imaging Neuroinflammation Biomarkers
Compromised BBB can be identified with gadolinium-enhanced MRI as is seen in the setting of white matter lesions in multiple sclerosis. CNS-infiltration of circulating immune cells, such as monocyte infiltration into brain parenchyma, can be tracked with iron oxide nanoparticles and MRI. Pathological consequences of neuroinflammation such as apoptosis can be imaged with PET [99mTc] Annexin V or with iron accumulation with using MRI T2* relaxometry. These imaging techniques can be used to image human neuroinflammation which have potential to impact patient care in the foreseeable future (62, 63). Integrated positron emission tomography-magnetic resonance imaging and the radioligand 11C-PBR28 for the translocator protein (TSPO) can be used to image regional brain volumes with glial activation. Given the putative role of activated glia in the establishment and or maintenance of persistent pain, pathophysiology, and management of a variety of persistent pain conditions the results from this technique are important to consider when considering imaging techniques for measuring CNS inflammatory effects of pain (64).
Endocannabinoid Pain Biomarkers
Endocannabinoid Mechanisms
There are three classifications of cannabinoids: phytocannabinoids (plant-derived), endocannabinoids (in human or animal tissues), and synthetic cannabinoids. Similar to the opioid system, versions of the endocannabinoid (ECB) system have been found in the vast majority of species with a nervous system (65). In particular, the ECB ligands 2-AG and AEA have been found throughout the animal kingdom (66). The ECB system regulates physiology across most organ systems and operates independently and interacts with the inflammatory system, the opiate system, the Vaniloid (TRP) system, and with nuclear transcription factors (67–72). The ECB system works as a part of a negative feedback loop that regulates neurotransmitter and neuropeptide release in the nervous system. Endocannabinoid ligands are generated on-demand in response to high levels of activity and produce short-term inhibitory effects via their actions as retrograde transmitters at presynaptic inhibitory G protein-coupled receptors (2).
The two most prevalent endocannabinoid ligands that bind endocannabinoid receptors are anandamide (AEA) and 2-arachidonoylglycerol (2-AG) (67). The 2-AG basal level is ~1,000 times greater than AEA in the brain (73). The enzyme acylphosphatidylethanolamine-phospholipase D is involved in the formation of AEA, and the enzyme diacylglycerol lipase is involved in 2-AG formation (74). Once synthesized and released, endocannabinoids are removed from the extracellular space through an endocannabinoid membrane transporter, subsequently AEA is hydrolyzed by the enzyme fatty acid amide hydrolase (FAAH), and 2-AG is degraded by cytosolic monoacylglycerol lipase (MAGL).
There are two G protein-coupled ECB receptors (CBR1 and CBR2). CBR1 receptors are highly expressed on presynaptic neurons in the brain, spinal cord, and dorsal root ganglion. CBR2 receptors are primarily expressed in immune cells (including myeloid, macrophage, lymphoid, and mast cells) (75). AEA maintains basal endocannabinoid tone and has a high selectivity for the CBR1 receptor over the peripheral CBR2 receptor.
The CBR1 receptor is the most abundant G protein-coupled receptor in the brain and one of the most abundant in both the peripheral and central nervous system. CBR1 are expressed primarily on presynaptic peripheral and central nerve terminals. CBR1 is the central receptor responsible for the behavioral and psychotropic effects of the “high” caused by THC (65, 72). The CBR1 receptor is also present in multiple immune cells, making it important when considering anti-inflammatory properties of endocannabinoids (68, 75).
The CBR2 receptor is largely present on peripheral immune cells and participates in regulation of the immune system. The principal endogenous ligand for the CBR2 receptor is 2-AG. In the brain, stimulation of CBR2 receptors does not produce cannabis-like effects (72, 74). The anti-inflammatory effects of exogenous cannabinoids are mediated by the endocannabinoid system, likely through CB2Rs in the periphery that have immunomodulatory functions (76).
In neural and non-neural systems, in response to tissue injury or excessive nociception, the ECB system generally suppresses inflammation, suppresses sensitization, and suppresses pain (68, 71, 72, 77); however, ECB activity on the nociception system can be complicated, with ECB antinociceptive or pronociceptive depending on the site of expression and the underlying physiological brain state (2).
Endocannabinoid Pain Biomarkers
ECB biomarkers have been used to monitor neuropathic pain. Increases in circulating AEA concentrations occur in patients with neuropathic pain complex regional pain syndrome (CRPS) when compared to controls without pain (78). Increases in circulating 2-AG concentrations have also been reported in neuromyelitis optica (79). Circulating concentrations of AEA and 2-AG have been shown to correlate with the numbers of daily severe headaches (80). ECB biomarkers also are present for non-neuropathic pain conditions: bladder pain (81), fibromyalgia (78, 82), cold pain sensitivity (83), osteoarthritis (84), knee pain (85), and back pain (86). Clinical Cannabinoid Deficiency Syndrome has been linked to migraines, neuromuscular pain, and gastrointestinal disorders (87).
Endocannabinoid Interactions With Inflammation and Opioids
When considering ECB pain biomarkers, it is important to consider that the ECB system interacts significantly with inflammation mechanisms as well as opioid mechanisms. The ECB system regulates inflammation at multiple levels and generally inhibits inflammation. In preclinical and laboratory investigation, it has been shown that the ECB system inhibits pro- inflammatory cytokines while increasing production of anti-inflammatory cytokines. The ECB system also inhibits immune cell activation, immune cell proliferation and migration, and can increase immune cell apoptosis via multiple mechanisms (23, 67, 88–93).
CBR1 receptors are 10 times more concentrated than mu-opioid receptors in the brain, and cannabinoid receptors co-localize with opioid receptors in many regions involved in pain circuitry including the dorsal horn of the spinal cord and in the supra-spinal periaqueductal gray and rostro-ventral medulla (67, 72, 94). ECB and opioid pain biomarkers potentially can be identified via endogenous opioid function which can be assumed when comparing pain sensitivity in the presence of opioid blocking treatment, such as naloxone vs. placebo. Higher endogenous opioid function is associated with decreased benefit from opioid treatments such as morphine (95). Exercise induced analgesia involves both opioid and endocannabinoid mechanisms (96). Exercise induced increases of endocannabinoid ligands such as AEA are blocked by naltrexone. This indicates that opioids are involved in the increase of endocannabinoid ligands following exercise (97). Intrathecal morphine decreases circulating levels of endocannabinoids demonstrating how the opioid and endocannabinoid systems are linked (98). Lower endogenous opioid function is associated with greater analgesia from pain treatment with opiates. One study demonstrated that low endocannabinoid activity is also associated with greater analgesia from pain treatment with opiates (99).
Pathogenic alterations in the distribution of microbial species within the gut (gut dysbiosis) is associated with neuropathic pain in a variety of clinical conditions. One study found that reductions in the diversity and increases in the ratios of two microbial species (ratios of Blautia and Clostridium to Lachnospira) may contribute to HIV-associated neuropathic pain (100). This may be particularly relevant in the context of the endocannabinoid system, as the endocannabinoid system regulates homeostasis of multiple organ systems, including the gut. Because dysregulation of the gut-brain axis can result in chronic inflammation and neuroinflammation, endocannabinoids have anti-oxidant, and anti-inflammatory properties relevant to modulation of inflammation that occurs along the gut-brain axis (101, 102).
Non-Imaging Pain Biomarkers
Genetic Biomarkers
In a systematic review and meta-analysis of 29 studies on potential genetic variants associated with neuropathic pain identified (103), 28 genes were significantly associated with neuropathic pain, many involved in neurotransmission, immune response, and metabolism. Genetic variants in HLA genes, COMT, OPRM1, TNFA, IL6, and GCH1, were found to have an association with neuropathic pain in more than one study. In the meta-analysis, polymorphisms in HLA-DRB1*13, HLA-DRB1*04, HLA-DQB1*03, HLA-A*33, and HLA-B*44 were associated with significantly increased risk of developing neuropathic pain, whereas HLA-A*02 reduced risk of neuropathic pain.
To detect genetic associations, particularly those of small effect size, a study must be sufficiently statistically powered to detect those differences. Most genetic studies of neuropathic pain have typically analyzed cohorts with <1,000 cases, which has resulted in only suggestive associations (103, 104). One reason that genetic studies in neuropathic pain lack sufficient sample sizes is the costs associated with studying these large cohorts (105).
Micro-RNA
Micro-RNA Mechanisms
MicroRNA are small non-coding RNA molecules that contain about 22 nucleotides and are found in plants, animals, and some viruses (106). Identification of the first microRNA occurred in 1993, and currently more than 2,000 human microRNAs have been recognized (107). MicroRNA function in RNA silencing and post-transcriptional gene expression regulation. Base-pairing occurs between microRNA and complementary sequences of mRNA molecules leading to silencing of mRNA by (i) cleavage of the mRNA, (ii) destabilization of the mRNA by shortening the poly(A) tail, and (iii) inefficient translation of mRNA into proteins by ribosomes. Each microRNA species regulates multiple genes creating a complex regulatory network (108, 109).
MicroRNA-mRNA interactions allow for modification of gene expression by controlling translation in response to signaling events. Disease states or perturbations in cellular homeostasis can lead to aberrant microRNA expression (110). Numerous studies suggest the involvement of microRNAs in key biological processes including development and cellular homeostasis, and their altered expression is associated with various pathological conditions including cancer, immune disease, inflammatory disease as well as pain mechanisms (106, 111, 112).
Micro-RNA Are Stable in Blood Circulation
It is well-established that microRNAs are present in the serum and plasma of humans and stable (as part of RNase resistant molecular complexes or within vesicles or exosomes) such that retrospective studies can be performed using banked samples (106, 110, 113). Horizontal transfer of circulating microRNAs between cells is a novel mode of intercellular communication (109).
Micro-RNA-Based Biomarkers of Chronic Pain
The discovery of stable microRNAs in circulation has generated enormous interest in exploring their utility as potential non-invasive biomarkers (110, 114). The induction and chronification of pain are associated with many expressional changes in pain-related proteins regulated by microRNA. Thus, microRNAs are useful as diagnostic and prognostic biomarkers in pain medicine. MicroRNAs have been found to be involved in the onset and progression of several human chronic pain conditions by means of gene repression (107, 114–116). MicroRNA signatures specific to different pain conditions, and their reversal on treatment can be beneficial in patient stratification, prognosis and in bridging pre-clinical, and clinical results (113).
Dysregulations in microRNAs have been reported in several pain disorders in humans in both affected tissues and the circulation (107, 117): Neuropathic pain (117, 118), peripheral neuropathy (119, 120), complex regional pain syndrome (109, 117, 118), cystitis-induced chronic pain (118), osteoarthritis (121), irritable bowel disorder (117, 118), fibromyalgia (117, 118), and migraine (107, 111, 122).
Micro-RNA Biomarkers of Chronic Pain Treatment
Presence of circulating microRNAs within exosomes opens up novel avenues for targeting treatments for chronic pain conditions. Such approaches can provide insights on the molecular underpinnings regarding therapeutic targets, treatment doses, and patient eligibility for different treatments (113, 118, 123).
Selectively inhibiting or supplementing a microRNA contributing to pathogenesis is being pursued as a therapeutic strategy for a variety of disorders. Studies from rodent pain models and from patients have now implicated a role for microRNAs in mediating various aspects of pain processing. These non-coding RNAs can provide mechanistic insights into the pathways modulated and could serve as therapeutic targets (110). Drug treatments alter microRNAs in humans and in various animal models. Thus, microRNAs can be predictive biomarkers for therapeutic intervention as well as prognostic markers for treatment response (106).
Micro-RNA Biomarkers of Painful Peripheral Neuropathies
MicroRNA modulated inflammation has a major role in the induction and maintenance of neuropathic pain. Inflammation-regulating microRNA profiles in patients with peripheral neuropathies have been characterized. In patients with polyneuropathies of different etiologies the expression of miR-21-5p, miR-146a, and miR-155 were upregulated. In painful neuropathies, tissues from skin biopsies from the lower leg, where neuropathic changes are most common, had reduced miR-146a and miR-155 expression compared to the thigh; furthermore, peripheral neuropathies are associated with aberrant microRNA expression in the sural nerve and in the skin (119). In sural nerve biopsies of patients with peripheral neuropathies miR-132-3p expression was more than doubled in white blood cells of neuropathy patients compared to healthy controls as well as in painful compared with non-painful neuropathy (120). MiRNA's (miR-155) have been found to be upregulated in polyneuropathies, and miR-21 is increased in painful neuropathies.
Stress
Allostatic Load
Allostatic load indices, an index that measures the effects on the body by chronic stress, have been demonstrated to predict morbidity and mortality. Allostatic load has been studied in pain with allostatic load model covariates including age, sex, education, smoking status, alcohol consumption, activity level, depression, and common comorbid health conditions. Allostatic load pain models have shown a positive relationship between pain severity and allostatic load (124).
Cortisol and Dehydroepiandrosterone
Cortisol is a proposed stress-related pain biomarker (125). DHEA and DHEAS are neurosteroids that modulate inhibitory GABA receptors and excitatory NMDA receptors, producing complex neuronal effects (126). In animal studies, DHEA and DHEAS levels have been proposed as a biomarker for pain (127, 128). In multivariable regression analysis, gender, age, and pain perception in the shoulder and upper limbs were significantly related to serum DHEAS (129). In another study plasma DHEAS levels were lower compared with persons with chronic neck pain compared with controls with no pain (130). One study found that the odds of having depressive symptoms increased with higher cortisol/DHEA-S ratios among people living with HIV on treatment, suggesting altered neuroactive steroid metabolism may contribute to the pathophysiological mechanisms of depression in people living with HIV (131). A study of male war veterans found that reductions in DHEA levels were associated with muscle soreness and were positively associated with chest pain (132). Self-reported back pain measures in female war veterans were inversely correlated with DHEA and DHEA-S (126); those reporting moderate to severe low back pain demonstrated significantly lower DHEA-S levels compared to those with no or mild lower back pain.
Allopregnanolone
Allopregnanolone is a neuroactive steroid derived from progesterone that is synthesized within the nervous tissue. Allopregnanolone interacts with GABA-A receptors making it important in neuroprotection particularly in cases of ischemia and peripheral neuropathy. Plasma allopregnanolone immunoreactivity has been associated with decreased pain sensitivity in humans which may be mediated by hypothalamic-pituitary-axis function (133). Allopregnanolone levels have also been inversely associated with low back pain and chest pain (132). In addition, allopregnanolone levels have been inversely associated with muscle soreness, chest pain, and aggregate total pain among war veterans (134).
Saliva
Biomarkers in saliva may be useful as they are easily measurable without requiring a needlestick or invasive methods (135). Salivary biomarkers, such as salivary cortisol, salivary α-amylase, secretory IgA (sIgA), testosterone, glutamate, or tumor necrosis factor receptor type II (TNF-RII) has been proposed as possible pain biomarkers (136–138). In particular, sIgA and TNF-RII as useful salivary markers of pain given their high intra-individual reproducibility (139, 140).
Other
QST and Skin Biopsy and Peripheral Nerve Imaging
Quantitate sensory testing and skin punch biopsy results are potential peripheral neuropathic pain biomarkers, in particular for diabetic peripheral neuropathy (141, 142). Markers for peripheral nerve fiber degeneration and regeneration, microvasculature characteristics, and peripheral angiogenesis have been investigated as biomarkers for diabetic peripheral neuropathic pain (142). A review of MRI imaging of the sciatic nerve and its branches provides convincing evidence that diabetic peripheral neuropathy is associated with increase nerve cross sectional area, T2-weighted hyperintense and hypointense lesions, evidence of nerve edema, decreased fractional anisotropy and increased apparent diffusion coefficient. These nerve abnormalities are potential markers of pain in diabetic neuropathy (143).
Physiological Markers
Skin conductance responses and alterations in electrocardiograms have been used to predict pain level ratings with high sensitivity and moderate specificity (144). Pupillary dilatation in response to noxious stimuli is thought to be related to locus coeruleus responses to nociceptive stimuli (145).
Fatty Acids and Linoleic Acid Derivative
Ornithine levels have also been found to be elevated in patients with persistent muscle pain (146). Significant correlations have also been seen for plasma concentrations of the linoleic acid derivatives 9- and 13-hydroxy-octadecadienoic acid among patients with neck pain (147).
Neurotrophic and Neurotransmitter Pain Biomarkers
Neurotrophic factors (BDNF, NGF, NT3, TrkA) and erythropoietin with the erythropoietin receptor are up-regulated in patients with peripheral neuropathy (56). Neurotransmitters in serum have been proposed as pain biomarkers, including neuropeptide Y and BDNF (148–150) as well as Dopamine (151). The catecholamine product metanephrine has also been proposed as a pain biomarker (152).
Brain Imaging Pain Biomarkers
Pain Brain Circuits
In the past decade, the focus of brain imaging investigation of pain mechanisms has shifted from investigating individual regions of the brain to investigating brain circuits, see Figure 1. One of the most important brain circuits is the default mode network which is associated with daydreaming (154, 155). The executive network is the brain circuit used when not daydreaming but instead attending to the outside world (156). The ascending pain network includes the anatomical pathway that conveys the nociceptive input from the peripheral nervous system to the spinal cord and the brain (157). The descending modulation network involves brain regions that connect to the brainstem and then down to the dorsal horn and increase or decrease ascending pain signals depending on the behavioral state of the individual (158). The salience network is a large-scale brain network of the human brain that is primarily composed of the anterior insula and dorsal anterior cingulate cortex. It is involved in detecting and filtering salient stimuli, as well as in recruiting relevant functional networks (157, 159).
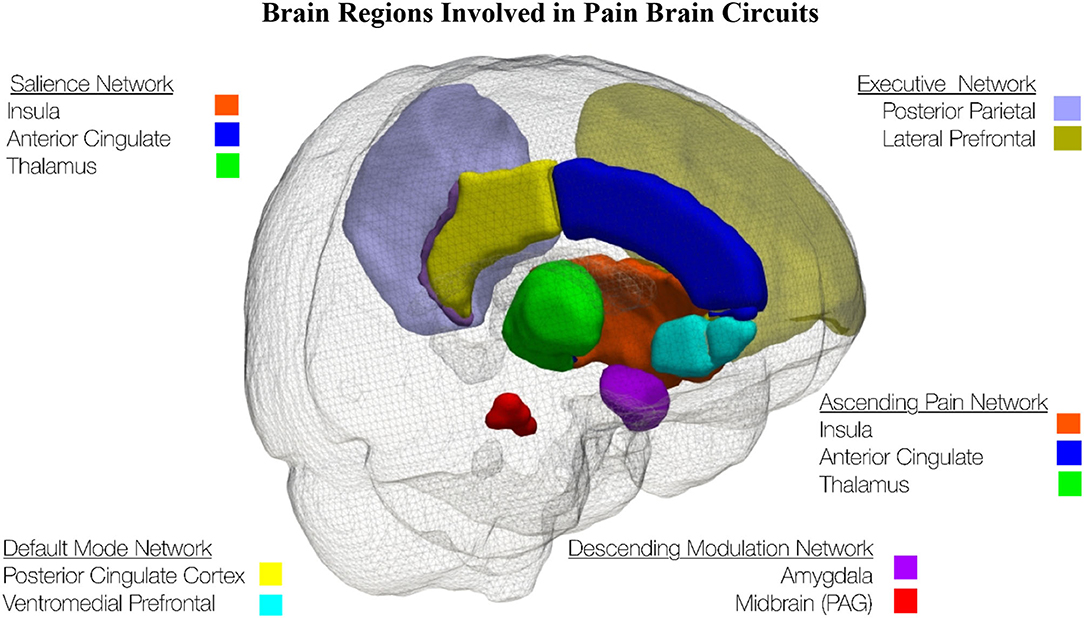
Figure 1. This schematic of the left side of the brain shows brain regions involved in pain brain circuits. The brain regions were extracted from the Hammers Maximum Probability Atlas (153).
Brain Circuit Changes in Chronic Pain
Chronic Pain Reduced Gray Matter Volumes
One of the most established chronic pain brain imaging biomarkers is reduced regional gray matter volumes in brain regions related to pain processing (160–164). One of the early reports of reduced brain gray matter volumes in chronic pain was reported in chronic back pain (160). Despite that brain atrophy has been identified as a potential cause of chronic pain in neurologic dementia disease (165), it has been demonstrated that chronic pain causes brain atrophy (164). Subsequent studies identified characteristic patterns of gray matter atrophy in different chronic pain conditions (161–163).
Presence of Chronic Pain
It has been shown that in multiple chronic pain conditions that there is increased connectivity between the salience network and the default mode network and decreased activity in the default mode network (166–171). This exciting biomarker for chronic pain indicates that the chronic pain experience is disrupting the normal daydreaming default mode network with an abnormal interaction with the salience network which monitors stressful events like the presence of painful stimulation.
Pain Rumination
Pain rumination occurs when people negatively dwell on their pain experience. Interestingly, this negative cognitive dwelling on the pain experience appears as increased activity in the default mode network (172). This increase in default mode activity is distinct from the decreased activity observed in the default mode network when chronic pain increases cross-talk between the salience network and the default mode network (166).
Pain Mind Wandering
It is plausible to envision that healthy mind wandering away from thinking about the pain experience is the opposite of pain rumination (172, 173). The dynamic pain connectome is a model that helps to understand the pain experience that include the salience network, default mode network, ascending pain system, and descending pain modulation pathway (157, 159). The dynamic pain connectome model was derived from brain imaging data in healthy subjects who had mind-wandering away from a painful stimulus. This work found that most brain regions were activated by noxious stimuli whether the mind wandered away from the stimulus or not. The responses of the salience and default mode networks and connectivity with antinociceptive areas showed mind wandering brain activity that included a clear distinction between trials in which subjects attended to pain vs. mind-wandered from pain (159, 173).
Transition to Chronic Pain
Increased functional connectivity between the medial prefrontal cortex–nucleus accumbens at the beginning of back pain predicts that patients will go on to develop chronic back pain; while patients with decreased connectivity in this circuit went on to recover from back pain (174, 175). Structural brain imaging in subacute back pain patients was followed longitudinally for 3 years as they either recovered from or transitioned to chronic pain. Furthermore, these results indicate that persistence of chronic pain is predetermined by corticolimbic neuroanatomical factors (176).
Placebo Mechanisms
There is substantial overlap between the circuits involved in human placebo analgesia and those that mediate multiple forms of context-based modulation of pain behavior in rodents, including forebrain-brainstem pathways and opioid and cannabinoid systems in particular. This suggests that placebo effects are a set of adaptive mechanisms that shape nociceptive signaling (177, 178). Subcortical limbic volume asymmetry, sensorimotor cortical thickness, and functional coupling of prefrontal regions, anterior cingulate, and periaqueductal gray are predictive of placebo response (179). One study found that placebo and nocebo effects are generated through differential engagement of the periaqueductal gray-rostral ventromedial medulla pathway, which likely influences pain experience by modulating activity at the dorsal horn level (180).
Pain States and Traits and Resilience
Individuals have a set point around which different biological attributes can fluctuate transiently into different states. However, if one remains in a different state other than their set point for a considerable period (e.g., induced by a disease), this different state is considered a new set point. In pain research it is important to consider trait and state pains to gain an understanding of not only an individual's current pain state but also more broadly to their trait pain, which may be more reflective of their general condition (181). Resilience is a trait that is highly associated with chronic pain–related health outcomes. The neural correlates of both pain and trait resilience are critical to understand the brain–behavior relationship in chronic pain; yet, neural correlates of resilience in chronic pain states are unknown (182). Therefore, regional BOLD (Blood Oxygen Level Dependent) variability and circuit connectivity have potential to provide predictive power for pain resilience or vulnerability to chronic pain and treatment efficacy (182, 183).
Imaging Biomarkers for Diabetic Peripheral Neuropathic Pain
Two reviews on mechanisms and imaging biomarkers for diabetic neuropathic pain review that diabetic peripheral neuropathy and associated pain have structural and functional central nervous system changes in the spinal cord, subcortex, and cortex (184, 185). Diabetic peripheral neuropathy has been associated with changes in the thalamus. A decreased thalamic NAA/creatinine ratio is suggestive of thalamic neuronal dysfunction (186, 187), and thalamic microvascular perfusion changes have also been observed (188). Smaller spinal cord cross-sectional area has been observed in those with diabetic peripheral neuropathy (189, 190). In fact, in diabetic peripheral neuropathy, diffusion tensor imaging techniques found posterior column damage in the cervical spinal cord (191). Diabetic neuropathic pain is related to decreased NAA in the thalamus (187), increased thalamic vascularity (188), and spinal cord posterior column damage (191).
Diabetic neuropathic pain is associated with increased regional brain gray matter volume loss localized to brain regions involved in somatosensory perception (192); furthermore, in diabetic neuropathic pain, increased total gray matter atrophy is associated with impaired ability to walk (193). Diabetic neuropathic pain has been shown to be related to aberrant default mode functional connectivity (171), decreased functional connectivity between the thalamus and cortex (194), and decreased functional connectivity in attention networks (195). Altered fMRI activation responses to experimental heat pain in limbic and striatal brain circuits are related to the duration of diabetic neuropathic pain (196). Diabetic neuropathic pain is related to a double dissociation such that neuropathic pain intensity is more associated with thalamus-insular cortex functional connectivity and nerve deficits are more related to thalamus-somatosensory cortex functional connectivity (197). Diabetic neuropathic pain is also associated with decreased functional connectivity between the thalamus and amygdala (198), decreased gray matter volumes and decreased white matter connectivity in pain processing and pain modulation brain regions (199), decreased somatosensory cortical thickness related to cortical function dysfunction (200), increased activity in the anterior cingulate cortex (201), as well as ventrolateral periaqueductal gray functional connectivity is altered and correlates with magnitude of spontaneous pain and allodynic pain (202).
Imaging Biomarkers for HIV Peripheral Neuropathic Pain
Structural brain imaging has revealed changes to the brain associated with HIV peripheral neuropathy. Total cortical volume is smaller with HIV distal neuropathic pain (203). In fact, in HIV distal neuropathic pain the posterior cingulate cortex is the cortical region that was found to be smaller (204). In another sample of people living with HIV, subjective symptoms of HIV peripheral neuropathy were associated with smaller precuneus volumes which overlap with the posterior cingulate cortex (205). Smaller brain volumes for HIV distal neuropathic pain are consistent with a general pattern that brain volumes are reduced for a variety of chronic pain conditions (161, 162). Interestingly, the midbrain, thalamus and posterior cingulate cortex volumes are all reduced in HIV distal neuropathic paresthesia (206). It has been suggested that brain atrophy associated with HIV distal neuropathic paresthesia may precede brain atrophy associated with HIV distal neuropathic pain (206).
More recent multi-modal brain imaging research has revealed structural brain changes associated with HIV peripheral neuropathy (207). HIV peripheral neuropathy is correlated with decreased white matter integrity running from the midbrain to the somatosensory cortex. HIV peripheral neuropathy severity is also associated with decreased generalized fractional anisotropy along the tracts of the external capsule in both hemispheres, appearing to lead along the lateral thalamus to sensorimotor cortex. A similar correlation is found in the superior bilateral cingulum. These results indicate ascending deafferentation in HIV peripheral neuropathy extends further downstream from damaged peripheral nerves than seen previously, into the cortex.
HIV-associated distal neuropathic pain is associated with decreased fMRI resting state functional connectivity in the default mode network and increased functional connectivity in the salience network (208). Decreased connectivity between the medial prefrontal cortex and posterior cingulate cortex and stronger connectivity between the ACC and thalamus is associated with HIV distal neuropathic pain.
In the setting of experimental heat pain, significant interaction has been found within the right anterior insula during expectation of experimental pain offset in that a group with HIV distal neuropathic pain compared group without HIV distal neuropathic pain exhibited increased insula activation in the feet (with painful neuropathic pain) compared to the hand (without neuropathic pain) (209). These findings are consistent with abnormal processing of expectation of experimental pain offset or abnormal pain relief mechanisms potentially due to increased negative expectation regarding the experience of chronic endogenous neuropathic pain.
Imaging Biomarkers for Chemotherapy Peripheral Neuropathic Pain
Anterior cingulate cerebral perfusion and gray matter density correlate with chemotherapy-induced peripheral neuropathy symptoms including pain (210). Patients with chemotherapy-induced peripheral neuropathy symptoms (including pain) demonstrated greater activation during painful stimulation in the precuneus compared to healthy controls and exhibited hypo-activation of the right superior frontal gyrus compared to healthy controls. Painful stimuli delivered chemotherapy-induced peripheral neuropathy symptoms patients evoke differential activation of distinct cortical regions, reflecting a unique pattern of central pain processing compared with healthy controls providing a tool for monitoring cerebral changes during anti-cancer and analgesic treatment (211).
Small-Fiber Peripheral Neuropathy Brain Changes
A population of mixed small-fiber peripheral neuropathy (metabolic, inflammatory, chemo, idiopathic) was used to investigate how dysfunction of skin nerves led to abnormal recruitment of pain-related brain regions, suggesting that the brain may be affected in SFN. Greater volume reduction in pain-processing regions, particularly the bilateral anterior cingulate cortices was associated with greater depletion of intraepidermal nerve fibers. There was significant reduction in functional connectivity from the anterior cingulate cortex to the insula pain-processing cortex that is linearly correlated with the severity of intraepidermal nerve fiber depletion (212). Similarly, another population of mixed small-fiber peripheral neuropathy (metabolic, inflammatory, chemo, idiopathic) the degree of skin nerve degeneration was associated with the reduction of connectivity between the thalamus and pain-related areas. Despite altered white matter connectivity, there was no change in white matter integrity assessed with fractional anisotropy. These findings indicate that alterations in structural connectivity may serve as a biomarker of maladaptive brain plasticity that contributes to neuropathic pain after peripheral nerve degeneration (213).
Imaging Biomarkers for Other Peripheral Neuropathies and Pain
A population of Charcot-Marie-Tooth patients had abnormal diffusion tensor imaging findings indicative of significant cerebral white matter abnormalities. Diffusion tensor imaging abnormalities were correlated with clinical disability, suggesting that there is comorbidity of central nervous system damage with peripheral neuropathy in Charcot-Marie-Tooth patients (214). A population of patients with hereditary neuropathy with liability to pressure palsies were compared to a population of normal controls and the fractional anisotropy values of the patients were significantly lower in bilateral frontal, orbitofrontal, and temporal areas of white matter (215). Patient populations of paresthesia-dominant and pain-dominant patient groups were compared and contralesional cortical thickness were correlated with pain severity (216). Acquired and hereditary peripheral neuropathies are associated with increased functional connectivity of the left precuneus/posterior cingulate cortex in the default mode network. This increased connectivity in the default mode network is correlated with duration of peripheral neuropathy and severity of clinical total neuropathy score (217).
Composite Pain Biomarkers
As discussed in the introduction, if used in combination, biomarkers related to pain mechanisms offer the possibility to develop objective pain-related indicators that may help diagnosis, treatment, and understanding of pain pathophysiology (8, 10). One possible application of such an approach might be to determine if a patient who is not communicative is experiencing pain. Another example may be to help guide selection of treatment for neuropathy, such as whether transcranial magnetic stimulation may alter network activity among those with neuropathy.
Modeling pain brain mechanisms can be achieved using multi-modal brain imaging including functional magnetic resonance imaging, structural magnetic resonance imaging, diffusion tensor magnetic resonance imaging, electroencephalography, EMG, and PET (10, 13, 14, 218). As we have reviewed here, in addition to using imaging biomarkers, composite pain biomarkers can be investigated using a multitude of non-imaging biomarkers.
Multiple analytic approaches have been used to investigate composite pain biomarkers: (i) composite algorithms have been investigated (219), (ii) unsupervised and supervised multivariate analyses have been used to distinguish pain groups and non-pain groups (220), (iii) supervised pattern recognition have been used to cluster diagnostic groups for different pain conditions (221), (iv) mechanism-based pharmacokinetic-pharmacodynamic modeling has been used to identify biomarkers that help diagnose pain and predict pain treatment (16), (v) principal component analysis has been applied to biochemical markers to create distinct pain profiles (222), (vi) patterns of inflammatory blood cytokines and chemokines have been used to differentiate pain and non-pain groups (223), (vii) multivariable data analysis using simultaneous analysis of 92 inflammation-related proteins with pain intensity and pain thresholds were used to identify protein patterns which distinguish pain and non-pain groups (223), (viii) metabolomics have been applied to chronic pain (224).
Discussion
Methods to Find Composite Pain Biomarkers
As detailed above, chronic pain and neuropathic pain impact multiple organ systems. Advancing the value of pain biomarkers depends on (1) selection of measurements and metrics that are the most mechanistically valid and informative, and (2) combining the selected measurements such that they mechanistically and statistically maximize accurate classification. Advancement of measurement accuracy is vital and the subsequent steps of the approach are entirely contingent upon the success of this step. This literature for the domains discussed in this manuscript is too voluminous for a single review. In the above reviewed literature, we attempted principally to focus on which biological systems and which biomarkers should be the focus of measurement. For effective application of measurements of these domains it is important to discuss approaches for measurement selection.
In Figure 2, we provide a significantly abbreviated schematic of key available statistical approaches to handling multimodal datasets in building composite biomarkers. We have highlighted four general areas of statistics/machine learning: (1) feature reduction (225), (2) classification (226, 227), (3) regression (228), and (4) clustering (229). Feature reduction can occur during or prior to classification, regression, or clustering. Feature reduction primarily focuses on two primary approaches: (1) integration of measurements toward creation of a composite variable to simplify and enhance model performance, and (2) effective feature reduction through variable selection to use optimal variables. Thus, feature reduction can represent the effective combining of strong measurements to a meaningful and robust latent variable or elimination of unnecessary, or statistically weak, measurements. Some methods, such as random forest, has built in feature reduction (230). Classification methods are often utilized to build toward categorical variables, however methods like neural networks are also designed for predicting continuous variables (231). Regression models are often used for the prediction of continuous measures or in the case of canonical approaches this can be with multiple dependent variables predicted simultaneously (232). Finally, in the case where there is no existent or optimal category or variable that the biomarkers seek to predict unsupervised approaches can be useful. With all these approaches variables can either be approached as linear or non-linear, although transformations and feature reduction approaches can mitigate these differences. It is important, regardless of approach, to understand the biological mechanisms being modeled by defining a model that best reflects the underlying systems to optimize prediction.
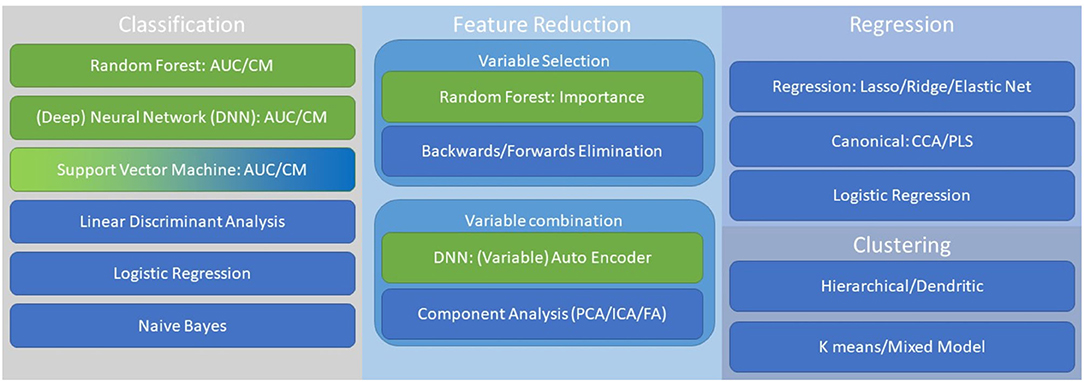
Figure 2. This is a non-comprehensive guide to important approaches when considering multimodal biomarkers. Key approaches include, classification, feature reduction, regression, and clustering. Linear (blue) and non-linear (green) approaches are highlighted, although ranking order and other transformations can be adapted across methodology.
Two key methods for statistical reduction of variables are (1) selecting top ranking variables and (2) creation of composite variables by factor or component-based analysis. Random Forest, as depicted in Figure 2, can be utilized to determine importance scores by evaluating the hierarchical functionality of a given variable as a bifurcator for optimizing classification (226). Random Forest is not alone in its utility to provide variable importance ranking but provides a nice mechanism for this analysis. The statistical creation of composite variables can be done through principal component analysis (or independent component analysis among other methods) such that novel values are calculated for a set of variables that account for large swaths of variance with a single value vector (233, 234). This can substantially increase the efficiency of a model and serve to highlight a robust latent feature.
Summary of Possible Pain Biomarkers
A summary of pain biomarkers discussed in this review article are provided in Table 1. Non-imaging pain biomarkers include opioid pain biomarkers: Beta-endorphin, B-cell opioid receptors, composite genetic, Mu-opioid receptor A118G polymorphisms, migraine opioid PET, and endogenous opioid function. Inflammatory pain biomarkers include cytokines, sICAM-1, cytokines related to back pain, cytokines related to peripheral neuropathy, substance P, and neuropeptides. Endocannabinoid pain biomarkers include: AEA in CRPS, 2-AG in optic neuromyelitis, AEA and 2-AG in headaches, ECB elements in multiple non-neuropathic pain conditions, ECB elements in endogenous opioid function, and ECB elements in gut-brain interactions. There are pain biomarker genes related to neuropathic pain risk. MICRO-RNA dysregulation pain biomarkers are found in neuropathic pain, peripheral neuropathic pain, CRPS, migraine, and non-neuropathic pain conditions. Stress related pain biomarkers include allostatic load, Cortisol, DHEA(S), and allopregnanolone. Measuring saliva contains potentially particularly accessible pain biomarkers. Other pain biomarkers can be accessed via QST, skin conductance, pupil dilation, fatty acid pain biomarkers (ornithine and linoleic acid derivatives), neurotrophic factors, and serum neurotransmitters.
Brain imaging pain biomarkers for measuring pain can be evaluated using three different MRI brain methods: gray matter structural imaging, white matter diffusion tensor imaging, and functional brain activation. Brain circuits related to pain mechanisms include an ascending brain circuit, a descending pain modulation circuit, the default mode circuit, the executive network brain circuit, and finally the salience network. Pain mechanisms in the brain can be measured via modulation in brain circuits: acute pain machine learning measures of chronic pain, pain rumination, pain mind wandering, placebo mechanisms, pain traits and states, and resilience. HIV peripheral neuropathy changes in the brain include reduced total cortical gray matter and reduced posterior cingulate cortex volume in particular, white matter degeneration, altered resting state networks, and aberrant expectation of pain relief.
By focusing on a broad array of mechanisms and biomarkers, we can uncover important mechanistic connections and interactions across systems. Neuropathic pain is a debilitating condition that has primary, and cascading affects across body systems. Assessment and understanding in an appropriately comprehensive approach are challenging due to the vast and diverse literature and the complexity measurement. This review aims to facilitate navigation of this literature and the appropriate selection of biomarkers for future research.
Author Contributions
All authors have contributed to the writing and scientific direction of this manuscript. All authors contributed to the article and approved the submitted version.
Conflict of Interest
The authors declare that the research was conducted in the absence of any commercial or financial relationships that could be construed as a potential conflict of interest.
Publisher's Note
All claims expressed in this article are solely those of the authors and do not necessarily represent those of their affiliated organizations, or those of the publisher, the editors and the reviewers. Any product that may be evaluated in this article, or claim that may be made by its manufacturer, is not guaranteed or endorsed by the publisher.
Abbreviations
2-AG, 2-arachidonoylglycerol; AEA, anandamide; CBR, G protein-coupled ECB receptor; CSF, cerebral spinal fluid; CRPS, complex regional pain syndrome; CRP, c reactive protein; DMN, default mode network; DHEA, dehydroepiandrosterone; ECB, endocannabinoid; FAAH, fatty acid amide hydrolase; MAGL, cytosolic monoacylglycerol lipase; MPF, medial prefrontal cortex; NA, nucleus accumbens; QST, quantitative sensory testing; SFN, small fiber neuropathy; sICAM-1, soluble intercellular adhesion molecule-1; TNF, tumor necrosis factor; WBC, white blood cell.
References
1. Costigan M, Scholz J, Woolf CJ. Neuropathic pain: a maladaptive response of the nervous system to damage. Annu Rev Neurosci. (2009) 32:1–32. doi: 10.1146/annurev.neuro.051508.135531
2. Woodhams SG, Chapman V, Finn DP, Hohmann AG, Neugebauer V. The cannabinoid system and pain. Neuropharmacology. (2017) 124:105–20. doi: 10.1016/j.neuropharm.2017.06.015
3. Interagency Pain Research Coordinating Committee. National Pain Strategy: A Comprehensive Population Health-Level Strategy for Pain. Washington, DC: US Department of Health and Human Services, National Institutes of Health, (2016) 36.
4. Gaskin DJ, Richard P. The economic costs of pain in the United States. J Pain. (2012) 13:715–24. doi: 10.1016/j.jpain.2012.03.009
5. Bouhassira D, Lanteri-Minet M, Attal N, Laurent B, Touboul C. Prevalence of chronic pain with neuropathic characteristics in the general population. Pain. (2008) 136:380–7. doi: 10.1016/j.pain.2007.08.013
6. Bond M. Pain education issues in developing countries and responses to them by the international association for the study of pain. Pain Res Manag. (2011) 16:404–6. doi: 10.1155/2011/654746
7. Finnerup NB, Haroutounian S, Kamerman P, Baron R, Bennett DLH, Bouhassira D, et al. Neuropathic pain: an updated grading system for research and clinical practice. Pain. (2016) 157:1599–606. doi: 10.1097/j.pain.0000000000000492
8. Tracey I, Woolf CJ, Andrews NA. Composite pain biomarker signatures for objective assessment and effective treatment. Neuron. (2019) 101:783–800. doi: 10.1016/j.neuron.2019.02.019
9. Sisignano M, Lotsch J, Parnham MJ, Geisslinger G. Potential biomarkers for persistent and neuropathic pain therapy. Pharmacol Ther. (2019) 199:16–29. doi: 10.1016/j.pharmthera.2019.02.004
10. Davis KD. Imaging vs quantitative sensory testing to predict chronic pain treatment outcomes. Pain. (2019) 160 (Suppl. 1):S59–65. doi: 10.1097/j.pain.0000000000001479
11. Doshi TL, Nixdorf DR, Campbell CM, Raja SN. Biomarkers in temporomandibular disorder and trigeminal neuralgia: a conceptual framework for understanding chronic pain. Can J Pain. (2020) 4:1–18. doi: 10.1080/24740527.2019.1709163
12. Mackey S, Greely HT, Martucci KT. Neuroimaging-based pain biomarkers: definitions, clinical and research applications, and evaluation frameworks to achieve personalized pain medicine. Pain Rep. (2019) 4:e762. doi: 10.1097/PR9.0000000000000762
13. Wager TD, Atlas LY, Lindquist MA, Roy M, Woo CW, Kross E. An fMRI-based neurologic signature of physical pain. N Engl J Med. (2013) 368:1388–97. doi: 10.1056/NEJMoa1204471
14. van der Miesen MM, Lindquist MA, Wager TD. Neuroimaging-based biomarkers for pain: state of the field and current directions. Pain Rep. (2019) 4:e751. doi: 10.1097/PR9.0000000000000751
15. Mouraux A, Iannetti GD. The search for pain biomarkers in the human brain. Brain. (2018) 141:3290–307. doi: 10.1093/brain/awy281
16. Goulooze SC, Krekels EHJ, van Dijk M, Tibboel D, van der Graaf PH, Hankemeier T, et al. Towards personalized treatment of pain using a quantitative systems pharmacology approach. Eur J Pharm Sci. (2017) 109S:S32–8. doi: 10.1016/j.ejps.2017.05.027
17. Holden JE, Jeong Y, Forrest JM. The endogenous opioid system and clinical pain management. AACN Clin Issues. (2005) 16:291–301. doi: 10.1097/00044067-200507000-00003
18. Navratilova E, Xie JY, Meske D, Qu C, Morimura K, Okun A, et al. Endogenous opioid activity in the anterior cingulate cortex is required for relief of pain. J Neurosci. (2015) 35:7264–71. doi: 10.1523/JNEUROSCI.3862-14.2015
19. LaGraize SC, Borzan J, Peng YB, Fuchs PN. Selective regulation of pain affect following activation of the opioid anterior cingulate cortex system. Exp Neurol. (2006) 197:22–30. doi: 10.1016/j.expneurol.2005.05.008
20. Navratilova E, Xie JY, Okun A, Qu C, Eyde N, Ci S, et al. Pain relief produces negative reinforcement through activation of mesolimbic reward-valuation circuitry. Proc Natl Acad Sci USA. (2012) 109:20709–13. doi: 10.1073/pnas.1214605109
21. Stevens CW. Bioinformatics and evolution of vertebrate nociceptin and opioid receptors. Vitam Horm. (2015) 97:57–94. doi: 10.1016/bs.vh.2014.10.002
22. Law P-Y, Reggio PH, Loh HH. Opioid receptors: toward separation of analgesic from undesirable effects. Trends Biochem Sci. (2013) 38:275–82. doi: 10.1016/j.tibs.2013.03.003
23. Stein C, Machelska H. Modulation of peripheral sensory neurons by the immune system: implications for pain therapy. Pharmacol Rev. (2011) 63:860–81. doi: 10.1124/pr.110.003145
24. Schumacher M, Basbaum A, Naidu R. Opioid agonists & antagonists. In: Katzung B, Trevor A, editors. Basic & Clinical Pharmacology & Toxicology. 13th edition. McGraw Hill (2015). p. 531.
25. Stein C. Opioid receptors. Ann Rev Med. (2016) 67:433–51. doi: 10.1146/annurev-med-062613-093100
26. Benarroch EE. Endogenous opioid systems: current concepts and clinical correlations. Neurology. (2012) 79:807–14. doi: 10.1212/WNL.0b013e3182662098
27. Riley JL, 3rd Cruz-Almeida Y, Dasilva Ribeiro MC, Simon CB, Eckert NR, Aguirre M et al. Age differences in the time course and magnitude of changes in circulating neuropeptides after pain evocation in humans. J Pain. (2017) 18:1078–86. doi: 10.1016/j.jpain.2017.04.006
28. Backryd E, Ghafouri B, Larsson B, Gerdle B. Do low levels of beta-endorphin in the cerebrospinal fluid indicate defective top-down inhibition in patients with chronic neuropathic pain? A cross-sectional, comparative study. Pain Med. (2014) 15:111–9. doi: 10.1111/pme.12248
29. Qin ZL, Yang LQ, Li N, Yue JN, Wu BS, Tang YZ, et al. Clinical study of cerebrospinal fluid neuropeptides in patients with primary trigeminal neuralgia. Clin Neurol Neurosurg. (2016) 143:111–5. doi: 10.1016/j.clineuro.2016.02.012
30. Choi HY, Lee CH. Can beta-endorphin be used as a biomarker for chronic low back pain? A meta-analysis of randomized controlled trials. Pain Med. (2019) 20:28–36. doi: 10.1093/pm/pny186
31. Raffaeli W, Malafoglia V, Bonci A, Tenti M, Ilari S, Gremigni P, et al. Identification of MOR-positive B cell as possible innovative biomarker (mu lympho-marker) for chronic pain diagnosis in patients with fibromyalgia and osteoarthritis diseases. Int J Mol Sci. (2020) 21:1499. doi: 10.3390/ijms21041499
32. Kringel D, Ultsch A, Zimmermann M, Jansen JP, Ilias W, Freynhagen R, et al. Emergent biomarker derived from next-generation sequencing to identify pain patients requiring uncommonly high opioid doses. Pharmacogenomics J. (2017) 17:419–26. doi: 10.1038/tpj.2016.28
33. Kallman TF, Ghafouri B, Backryd E. Salivary beta-endorphin and substance P are not biomarkers of neuropathic chronic pain propensity. Heliyon. (2018) 4:e00718. doi: 10.1016/j.heliyon.2018.e00718
34. Wei SY, Chen LF, Lin MW, Li WC, Low I, Yang CJ, et al. The OPRM1 A118G polymorphism modulates the descending pain modulatory system for individual pain experience in young women with primary dysmenorrhea. Sci Rep. (2017) 7:39906. doi: 10.1038/srep39906
35. DaSilva AF, Zubieta JK, DosSantos MF. Positron emission tomography imaging of endogenous mu-opioid mechanisms during pain and migraine. Pain Rep. (2019) 4:e769. doi: 10.1097/PR9.0000000000000769
36. Woolf CJ. Central sensitization: implications for the diagnosis and treatment of pain. Pain. (2011) 152 (3 Suppl):S2–15. doi: 10.1016/j.pain.2010.09.030
37. Calvo M, Dawes JM, Bennett DL. The role of the immune system in the generation of neuropathic pain. Lancet Neurol. (2012) 11:629–42. doi: 10.1016/S1474-4422(12)70134-5
38. Ellis A, Bennett DL. Neuroinflammation and the generation of neuropathic pain. Br J Anaesth. (2013) 111:26–37. doi: 10.1093/bja/aet128
39. Klewer J, Goyen M, Froese G, Wilhelmsen E, Mertens M. [Anxiety immediately before lumbar myelography and possibilities for recognizing anxious patients in routine clinical practice]. Rontgenpraxis. (1998) 51:16–22.
40. Taves S, Berta T, Chen G, Ji RR. Microglia and spinal cord synaptic plasticity in persistent pain. Neural Plast. (2013) 2013:753656. doi: 10.1155/2013/753656
41. Ji RR, Xu ZZ, Gao YJ. Emerging targets in neuroinflammation-driven chronic pain. Nat Rev Drug Discov. (2014) 13:533–48. doi: 10.1038/nrd4334
42. Gilron I, Watson CP, Cahill CM, Moulin DE. Neuropathic pain: a practical guide for the clinician. CMAJ. (2006) 175:265–75. doi: 10.1503/cmaj.060146
43. Ji RR, Suter MR. p38 MAPK, microglial signaling, and neuropathic pain. Mol Pain. (2007) 3:33. doi: 10.1186/1744-8069-3-33
44. Liefner M, Siebert H, Sachse T, Michel U, Kollias G, Bruck W. The role of TNF-alpha during wallerian degeneration. J Neuroimmunol. (2000) 108:147–52. doi: 10.1016/S0165-5728(00)00262-9
45. Taneja A, Della Pasqua O, Danhof M. Challenges in translational drug research in neuropathic and inflammatory pain: the prerequisites for a new paradigm. Eur J Clin Pharmacol. (2017) 73:1219–36. doi: 10.1007/s00228-017-2301-8
46. Fernandes V, Sharma D, Vaidya S, Shantanu PA, Guan Y, Kalia K, et al. Cellular and molecular mechanisms driving neuropathic pain: recent advancements and challenges. Expert Opin Ther Targets. (2018) 22:131–42. doi: 10.1080/14728222.2018.1420781
47. Xu Q, Yaksh TL. A brief comparison of the pathophysiology of inflammatory versus neuropathic pain. Curr Opin Anaesthesiol. (2011) 24:400–7. doi: 10.1097/ACO.0b013e32834871df
48. Echeverry S, Shi XQ, Rivest S, Zhang J. Peripheral nerve injury alters blood–spinal cord barrier functional and molecular integrity through a selective inflammatory pathway. J Neurosci. (2011) 31:10819–28. doi: 10.1523/JNEUROSCI.1642-11.2011
49. Basbaum AI, Bautista DM, Scherrer G, Julius D. Cellular and molecular mechanisms of pain. Cell. (2009) 139:267–84. doi: 10.1016/j.cell.2009.09.028
50. Ji R-R, Berta T, Nedergaard M. Glia and pain: is chronic pain a gliopathy? Pain. (2013) 154 (Suppl 1):S10–28. doi: 10.1016/j.pain.2013.06.022
51. Tremblay M-E, Stevens B, Sierra A, Wake H, Bessis A, Nimmerjahn A. The role of microglia in the healthy brain. J Neurosci. (2011) 31:16064–9. doi: 10.1523/JNEUROSCI.4158-11.2011
52. Guan Z, Kuhn JA, Wang X, Colquitt B, Solorzano C, Vaman S, et al. Injured sensory neuron-derived CSF1 induces microglial proliferation and DAP12-dependent pain. Nat Neurosci. (2016) 19:94–101. doi: 10.1038/nn.4189
53. Bjurstrom MF, Giron SE, Griffis CA. Cerebrospinal fluid cytokines and neurotrophic factors in human chronic pain populations: a comprehensive review. Pain Pract. (2016) 16:183–203. doi: 10.1111/papr.12252
54. DeVon HA, Piano MR, Rosenfeld AG, Hoppensteadt DA. The association of pain with protein inflammatory biomarkers: a review of the literature. Nurs Res. (2014) 63:51–62. doi: 10.1097/NNR.0000000000000013
55. Luchting B, Hinske LC, Rachinger-Adam B, Celi LA, Kreth S, Azad SC. Soluble intercellular adhesion molecule-1: a potential biomarker for pain intensity in chronic pain patients. Biomark Med. (2017) 11:265–76. doi: 10.2217/bmm-2016-0246
56. Uceyler N, Riediger N, Kafke W, Sommer C. Differential gene expression of cytokines and neurotrophic factors in nerve and skin of patients with peripheral neuropathies. J Neurol. (2015) 262:203–12. doi: 10.1007/s00415-014-7556-8
57. Doupis J, Lyons TE, Wu S, Gnardellis C, Dinh T, Veves A. Microvascular reactivity and inflammatory cytokines in painful and painless peripheral diabetic neuropathy. J Clin Endocrinol Metab. (2009) 94:2157–63. doi: 10.1210/jc.2008-2385
58. Mu ZP, Wang YG, Li CQ, Lv WS, Wang B, Jing ZH, et al. Association between tumor necrosis factor-alpha and diabetic peripheral neuropathy in patients with type 2 diabetes: a meta-analysis. Mol Neurobiol. (2017) 54:983–96. doi: 10.1007/s12035-016-9702-z
59. Sun C, Zhang J, Chen L, Liu T, Xu G, Li C, et al. IL-17 contributed to the neuropathic pain following peripheral nerve injury by promoting astrocyte proliferation and secretion of proinflammatory cytokines. Mol Med Rep. (2017) 15:89–96. doi: 10.3892/mmr.2016.6018
60. Nadeau S, Filali M, Zhang J, Kerr BJ, Rivest S, Soulet D, et al. Functional recovery after peripheral nerve injury is dependent on the pro-inflammatory cytokines IL-1beta and TNF: implications for neuropathic pain. J Neurosci. (2011) 31:12533–42. doi: 10.1523/JNEUROSCI.2840-11.2011
61. Douglas SD. Substance P and sickle cell disease-a marker for pain and novel therapeutic approaches. Br J Haematol. (2016) 175:187–8. doi: 10.1111/bjh.14299
62. Albrecht DS, Granziera C, Hooker JM, Loggia ML. In vivo imaging of human neuroinflammation. ACS Chem Neurosci. (2016) 7:470–83. doi: 10.1021/acschemneuro.6b00056
63. Albrecht DS, Granziera C, Hooker JM, Loggia ML. Correction to in vivo imaging of human neuroinflammation. ACS Chem Neurosci. (2018) 9:1515. doi: 10.1021/acschemneuro.8b00188
64. Loggia ML, Chonde DB, Akeju O, Arabasz G, Catana C, Edwards RR, et al. Evidence for brain glial activation in chronic pain patients. Brain. (2015) 138 (Pt. 3):604–15. doi: 10.1093/brain/awu377
65. Pascual D, Sanchez-Robles EM, Garcia MM, Goicoechea C. Chronic pain and cannabinoids. Great expectations or a christmas carol. Biochem Pharmacol. (2018) 157:33–42. doi: 10.1016/j.bcp.2018.07.033
66. Paulsen RT, Burrell BD. Comparative studies of endocannabinoid modulation of pain. Philos Trans R Soc Lond B Biol Sci. (2019) 374:20190279. doi: 10.1098/rstb.2019.0279
67. Donvito G, Nass SR, Wilkerson JL, Curry ZA, Schurman LD, Kinsey SG, et al. The endogenous cannabinoid system: a budding source of targets for treating inflammatory and neuropathic pain. Neuropsychopharmacology. (2018) 43:52–79. doi: 10.1038/npp.2017.204
68. Vuckovic S, Srebro D, Vujovic KS, Vucetic C, Prostran M. Cannabinoids and pain: new insights from old molecules. Front Pharmacol. (2018) 9:1259. doi: 10.3389/fphar.2018.01259
69. Greco R, Demartini C, Zanaboni AM, Piomelli D, Tassorelli C. Endocannabinoid system and migraine pain: an update. Front Neurosci. (2018) 12:172. doi: 10.3389/fnins.2018.00172
70. Lotsch J, Weyer-Menkhoff I, Tegeder I. Current evidence of cannabinoid-based analgesia obtained in preclinical and human experimental settings. Eur J Pain. (2018) 22:471–84. doi: 10.1002/ejp.1148
71. Maldonado R, Banos JE, Cabanero D. The endocannabinoid system and neuropathic pain. Pain. (2016) 157 (Suppl. 1):S23–32. doi: 10.1097/j.pain.0000000000000428
72. Baron EP. Medicinal properties of cannabinoids, terpenes, and flavonoids in cannabis, and benefits in migraine, headache, and pain: an update on current evidence and cannabis science. Headache. (2018) 58:1139–86. doi: 10.1111/head.13345
73. Zou S, Kumar U. Cannabinoid receptors and the endocannabinoid system: signaling and function in the central nervous system. Int J Mol Sci. (2018) 19:833. doi: 10.3390/ijms19030833
74. Tassorelli C, Greco R, Silberstein SD. The endocannabinoid system in migraine: from bench to pharmacy and back. Curr Opin Neurol. (2019) 32:405–12. doi: 10.1097/WCO.0000000000000688
75. Pellati F, Borgonetti V, Brighenti V, Biagi M, Benvenuti S, Corsi L. Cannabis sativa L. and nonpsychoactive cannabinoids: their chemistry and role against oxidative stress, inflammation, and cancer. Biomed Res Int. (2018) 2018:1691428. doi: 10.1155/2018/1691428
76. Costiniuk CT, Jenabian MA. Cannabinoids and inflammation: implications for people living with HIV. AIDS. (2019) 33:2273–88. doi: 10.1097/QAD.0000000000002345
77. Hillard CJ. Circulating endocannabinoids: from whence do they come and where are they going? Neuropsychopharmacology. (2018) 43:155–72. doi: 10.1038/npp.2017.130
78. Kaufmann I, Hauer D, Huge V, Vogeser M, Campolongo P, Chouker A, et al. Enhanced anandamide plasma levels in patients with complex regional pain syndrome following traumatic injury: a preliminary report. Eur Surg Res. (2009) 43:325–9. doi: 10.1159/000235870
79. Pellkofer HL, Havla J, Hauer D, Schelling G, Azad SC, Kuempfel T, et al. The major brain endocannabinoid 2-AG controls neuropathic pain and mechanical hyperalgesia in patients with neuromyelitis optica. PLoS ONE. (2013) 8:e71500. doi: 10.1371/journal.pone.0071500
80. Ramsden CE, Zamora D, Makriyannis A, Wood JT, Mann JD, Faurot KR, et al. Diet-induced changes in n-3- and n-6-derived endocannabinoids and reductions in headache pain and psychological distress. J Pain. (2015) 16:707–16. doi: 10.1016/j.jpain.2015.04.007
81. Sultana S, Berger G, Lehmann C. Components of the endogenous cannabinoid system as potential biomarkers for interstitial cystitis/bladder pain syndrome. Diagnostics. (2021) 12:19. doi: 10.3390/diagnostics12010019
82. Stensson N, Ghafouri N, Ernberg M, Mannerkorpi K, Kosek E, Gerdle B, et al. The relationship of endocannabinoidome lipid mediators with pain and psychological stress in women with fibromyalgia: a case-control study. J Pain. (2018) 19:1318–28. doi: 10.1016/j.jpain.2018.05.008
83. Cajanus K, Holmstrom EJ, Wessman M, Anttila V, Kaunisto MA, Kalso E. Effect of endocannabinoid degradation on pain: role of FAAH polymorphisms in experimental and postoperative pain in women treated for breast cancer. Pain. (2016) 157:361–9. doi: 10.1097/j.pain.0000000000000398
84. La Porta C, Bura SA, Llorente-Onaindia J, Pastor A, Navarrete F, Garcia-Gutierrez MS, et al. Role of the endocannabinoid system in the emotional manifestations of osteoarthritis pain. Pain. (2015) 156:2001–12. doi: 10.1097/j.pain.0000000000000260
85. Azim S, Nicholson J, Rebecchi MJ, Galbavy W, Feng T, Reinsel R, et al. Endocannabinoids and acute pain after total knee arthroplasty. Pain. (2015) 156:341–7. doi: 10.1097/01.j.pain.0000460315.80981.59
86. Ramesh D, D'Agata A, Starkweather AR, Young EE. Contribution of endocannabinoid gene expression and genotype on low back pain susceptibility and chronicity. Clin J Pain. (2018) 34:8–14. doi: 10.1097/AJP.0000000000000508
87. Russo EB. Clinical endocannabinoid deficiency reconsidered: current research supports the theory in migraine, fibromyalgia, irritable bowel, and other treatment-resistant syndromes. Cannabis Cannabinoid Res. (2016) 1:154–65. doi: 10.1089/can.2016.0009
88. Lu HC, Mackie K. An introduction to the endogenous cannabinoid system. Biol Psychiatry. (2016) 79:516–25. doi: 10.1016/j.biopsych.2015.07.028
89. Buisseret B, Alhouayek M, Guillemot-Legris O, Muccioli GG. Endocannabinoid and prostanoid crosstalk in pain. Trends Mol Med. (2019) 25:882–96. doi: 10.1016/j.molmed.2019.04.009
90. Di Marzo V. New approaches and challenges to targeting the endocannabinoid system. Nat Rev Drug Discov. (2018) 17:623–39. doi: 10.1038/nrd.2018.115
91. De Gregorio D, McLaughlin RJ, Posa L, Ochoa-Sanchez R, Enns J, Lopez-Canul M, et al. Cannabidiol modulates serotonergic transmission and reverses both allodynia and anxiety-like behavior in a model of neuropathic pain. Pain. (2019) 160:136–50. doi: 10.1097/j.pain.0000000000001386
92. Noreen N, Muhammad F, Akhtar B, Azam F, Anwar MI. Is cannabidiol a promising substance for new drug development? A review of its potential therapeutic applications. Crit Rev Eukaryot Gene Expr. (2018) 28:73–86. doi: 10.1615/CritRevEukaryotGeneExpr.2018021528
93. Romero-Sandoval EA, Asbill S, Paige CA, Byrd-Glover K. Peripherally restricted cannabinoids for the treatment of pain. Pharmacotherapy. (2015) 35:917–25. doi: 10.1002/phar.1642
94. Vanegas H, Vazquez E, Tortorici V. NSAIDs, opioids, cannabinoids and the control of pain by the central nervous system. Pharmaceuticals. (2010) 3:1335–47. doi: 10.3390/ph3051335
95. Bruehl S, Burns JW, Gupta R, Buvanendran A, Chont M, Kinner E, et al. Endogenous opioid function mediates the association between laboratory-evoked pain sensitivity and morphine analgesic responses. Pain. (2013) 154:1856–64. doi: 10.1016/j.pain.2013.06.002
96. Koltyn KF, Brellenthin AG, Cook DB, Sehgal N, Hillard C. Mechanisms of exercise-induced hypoalgesia. J Pain. (2014) 15:1294–304. doi: 10.1016/j.jpain.2014.09.006
97. Crombie KM, Brellenthin AG, Hillard CJ, Koltyn KF. Endocannabinoid and opioid system interactions in exercise-induced hypoalgesia. Pain Med. (2018) 19:118–23. doi: 10.1093/pm/pnx058
98. Kaczocha M, Azim S, Nicholson J, Rebecchi MJ, Lu Y, Feng T, et al. Intrathecal morphine administration reduces postoperative pain and peripheral endocannabinoid levels in total knee arthroplasty patients: a randomized clinical trial. BMC Anesthesiol. (2018) 18:27. doi: 10.1186/s12871-018-0489-5
99. Bruehl S, Burns JW, Morgan A, Koltyn K, Gupta R, Buvanendran A, et al. The association between endogenous opioid function and morphine responsiveness: a moderating role for endocannabinoids. Pain. (2019) 160:676–87. doi: 10.1097/j.pain.0000000000001447
100. Ellis RJ, Heaton RK, Gianella S, Rahman G, Knight R. reduced gut microbiome diversity in people with HIV who have distal neuropathic pain. J Pain. (2022) 23:318–25. doi: 10.1016/j.jpain.2021.08.006
101. Wilson NL, Peterson SN, Ellis RJ. Cannabis and the gut-brain axis communication in HIV infection. Cannabis Cannabinoid Res. (2021) 6:92–104. doi: 10.1089/can.2020.0037
102. Ellis RJ, Wilson N, Peterson S. Cannabis and inflammation in HIV: a review of human and animal studies. Viruses. (2021) 13:1521. doi: 10.3390/v13081521
103. Veluchamy A, Hebert HL, Meng W, Palmer CNA, Smith BH. Systematic review and meta-analysis of genetic risk factors for neuropathic pain. Pain. (2018) 159:825–48. doi: 10.1097/j.pain.0000000000001164
104. Hebert HL, Veluchamy A, Torrance N, Smith BH. Risk factors for neuropathic pain in diabetes mellitus. Pain. (2017) 158:560–8. doi: 10.1097/j.pain.0000000000000785
105. Calvo M, Davies AJ, Hebert HL, Weir GA, Chesler EJ, Finnerup NB, et al. The genetics of neuropathic pain from model organisms to clinical application. Neuron. (2019) 104:637–53. doi: 10.1016/j.neuron.2019.09.018
106. Ramanathan S, Shenoda BB, Ajit SK. Overview of microRNA modulation in analgesic research. Curr Protoc Pharmacol. (2017) 79:9.25.1–10. doi: 10.1002/cpph.29
107. Gazerani P. Current evidence on potential uses of MicroRNA biomarkers for migraine: from diagnosis to treatment. Mol Diagn Ther. (2019) 23:681–94. doi: 10.1007/s40291-019-00428-8
109. Birklein F, Ajit SK, Goebel A, Perez R, Sommer C. Complex regional pain syndrome - phenotypic characteristics and potential biomarkers. Nat Rev Neurol. (2018) 14:272–84. doi: 10.1038/nrneurol.2018.20
110. McDonald MK, Ajit SK. MicroRNA biology and pain. Prog Mol Biol Transl Sci. (2015) 131:215–49. doi: 10.1016/bs.pmbts.2014.11.015
111. Gallelli L, Cione E, Peltrone F, Siviglia S, Verano A, Chirchiglia D, et al. Hsa-miR-34a-5p and hsa-miR-375 as biomarkers for monitoring the effects of drug treatment for migraine pain in children and adolescents: a pilot study. J Clin Med. (2019) 8:928. doi: 10.3390/jcm8070928
112. Ochs MJ, Steinhilber D, Suess B. MicroRNA involved in inflammation: control of eicosanoid pathway. Front Pharmacol. (2011) 2:39. doi: 10.3389/fphar.2011.00039
113. Ramanathan S, Ajit SK. MicroRNA-Based biomarkers in pain. Adv Pharmacol. (2016) 75:35–62. doi: 10.1016/bs.apha.2015.12.001
114. Reyes-Long S, Cortes-Altamirano JL, Clavijio-Cornejo D, Gutierrez M, Bertolazzi C, Bandala C, et al. Nociceptive related microRNAs and their role in rheumatoid arthritis. Mol Biol Rep. (2020) 47:7265–72. doi: 10.1007/s11033-020-05700-3
115. Tan PH, Pao YY, Cheng JK, Hung KC, Liu CC. MicroRNA-based therapy in pain medicine: current progress and future prospects. Acta Anaesthesiol Taiwan. (2013) 51:171–6. doi: 10.1016/j.aat.2013.11.001
116. De Guire V, Robitaille R, Tetreault N, Guerin R, Menard C, Bambace N, et al. Circulating miRNAs as sensitive and specific biomarkers for the diagnosis and monitoring of human diseases: promises and challenges. Clin Biochem. (2013) 46:846–60. doi: 10.1016/j.clinbiochem.2013.03.015
117. Lopez-Gonzalez MJ, Landry M, Favereaux A. MicroRNA and chronic pain: from mechanisms to therapeutic potential. Pharmacol Ther. (2017) 180:1–15. doi: 10.1016/j.pharmthera.2017.06.001
118. Andersen HH, Duroux M, Gazerani P. MicroRNAs as modulators and biomarkers of inflammatory and neuropathic pain conditions. Neurobiol Dis. (2014) 71:159–68. doi: 10.1016/j.nbd.2014.08.003
119. Leinders M, Uceyler N, Thomann A, Sommer C. Aberrant microRNA expression in patients with painful peripheral neuropathies. J Neurol Sci. (2017) 380:242–9. doi: 10.1016/j.jns.2017.07.041
120. Leinders M, Uceyler N, Pritchard RA, Sommer C, Sorkin LS. Increased miR-132-3p expression is associated with chronic neuropathic pain. Exp Neurol. (2016) 283 (Pt A):276–86. doi: 10.1016/j.expneurol.2016.06.025
121. Munjal A, Bapat S, Hubbard D, Hunter M, Kolhe R, Fulzele S. Advances in molecular biomarker for early diagnosis of osteoarthritis. Biomol Concepts. (2019) 10:111–9. doi: 10.1515/bmc-2019-0014
122. Gallelli L, Cione E, Caroleo MC, Carotenuto M, Lagana P, Siniscalchi A, et al. microRNAs to monitor pain-migraine and drug treatment. Microrna. (2017) 6:152–6. doi: 10.2174/2211536606666170913152821
123. D'Agnelli S, Gerra MC, Bignami E, Arendt-Nielsen L. Exosomes as a new pain biomarker opportunity. Mol Pain. (2020) 16:1744806920957800. doi: 10.1177/1744806920957800
124. Sibille KT, McBeth J, Smith D, Wilkie R. Allostatic load and pain severity in older adults: results from the english longitudinal study of ageing. Exp Gerontol. (2017) 88:51–8. doi: 10.1016/j.exger.2016.12.013
125. Bagnato G, Cordova F, Sciortino D, Miceli G, Bruno A, Ferrera A, et al. Association between cortisol levels and pain threshold in systemic sclerosis and major depression. Rheumatol Int. (2018) 38:433–41. doi: 10.1007/s00296-017-3866-3
126. Naylor JC, Kilts JD, Strauss JL, Szabo ST, Dunn CE, Wagner HR, et al. An exploratory pilot investigation of neurosteroids and self-reported pain in female Iraq/Afghanistan-era veterans. J Rehabil Res Dev. (2016) 53:499–510. doi: 10.1682/JRRD.2014.11.0294
127. Juster RP, Sindi S, Marin MF, Perna A, Hashemi A, Pruessner JC, et al. A clinical allostatic load index is associated with burnout symptoms and hypocortisolemic profiles in healthy workers. Psychoneuroendocrinology. (2011) 36:797–805. doi: 10.1016/j.psyneuen.2010.11.001
128. Juster RP, McEwen BS, Lupien SJ. Allostatic load biomarkers of chronic stress and impact on health and cognition. Neurosci Biobehav Rev. (2010) 35:2–16. doi: 10.1016/j.neubiorev.2009.10.002
129. Marinelli A, Prodi A, Pesel G, Ronchese F, Bovenzi M, Negro C, et al. Serum dehydroepiandrosterone sulphate, psychosocial factors and musculoskeletal pain in workers. Occup Med. (2017) 67:684–6. doi: 10.1093/occmed/kqx159
130. Grimby-Ekman A, Ghafouri B, Sanden H, Larsson B, Gerdle B. Different DHEA-S levels and response patterns in individuals with chronic neck pain, compared with a pain free group-a pilot study. Pain Med. (2017) 18:846–55. doi: 10.1093/pm/pnw162
131. Mukerji SS, Misra V, Lorenz DR, Chettimada S, Keller K, Letendre S, et al. Low neuroactive steroids identifies a biological subtype of depression in adults with human immunodeficiency virus on suppressive antiretroviral therapy. J Infect Dis. (2021) 223:1601–11. doi: 10.1093/infdis/jiaa104
132. Kilts JD, Tupler LA, Keefe FJ, Payne VM, Hamer RM, Naylor JC, et al. Neurosteroids and self-reported pain in veterans who served in the U.S. Military after September 11, 2001. Pain Med. (2010) 11:1469–76. doi: 10.1111/j.1526-4637.2010.00927.x
133. Mechlin B, Morrow AL, Maixner W, Girdler SS. The relationship of allopregnanolone immunoreactivity and HPA-axis measures to experimental pain sensitivity: evidence for ethnic differences. Pain. (2007) 131:142–52. doi: 10.1016/j.pain.2006.12.027
134. Naylor JC, Kilts JD, Szabo ST, Dunn CE, Keefe FJ, Tupler LA, et al. Allopregnanolone levels are inversely associated with self-reported pain symptoms in U.S. Iraq and Afghanistan-Era veterans: implications for biomarkers and therapeutics. Pain Med. (2016) 17:25–32. doi: 10.1111/pme.12860
135. Jasim H, Carlsson A, Hedenberg-Magnusson B, Ghafouri B, Ernberg M. Saliva as a medium to detect and measure biomarkers related to pain. Sci Rep. (2018) 8:3220. doi: 10.1038/s41598-018-21131-4
136. Choi JC, Chung MI, Lee YD. Modulation of pain sensation by stress-related testosterone and cortisol. Anaesthesia. (2012) 67:1146–51. doi: 10.1111/j.1365-2044.2012.07267.x
137. Goodin BR, Quinn NB, King CD, Page GG, Haythornthwaite JA, Edwards RR, et al. Salivary cortisol and soluble tumor necrosis factor-alpha receptor II responses to multiple experimental modalities of acute pain. Psychophysiology. (2012) 49:118–27. doi: 10.1111/j.1469-8986.2011.01280.x
138. McLean SA, Williams DA, Harris RE, Kop WJ, Groner KH, Ambrose K, et al. Momentary relationship between cortisol secretion and symptoms in patients with fibromyalgia. Arthritis Rheum. (2005) 52:3660–9. doi: 10.1002/art.21372
139. Canton-Habas V, Carrera-Gonzalez MDP, Moreno-Casbas MT, Quesada-Gomez JM, Rich-Ruiz M. Correlation between biomarkers of pain in saliva and PAINAD scale in elderly people with cognitive impairment and inability to communicate: descriptive study protocol. BMJ Open. (2019) 9:e032927. doi: 10.1136/bmjopen-2019-032927
140. Sobas EM, Reinoso R, Cuadrado-Asensio R, Fernandez I, Maldonado MJ, Pastor JC. Reliability of potential pain biomarkers in the saliva of healthy subjects: inter-individual differences and intersession variability. PLoS ONE. (2016) 11:e0166976. doi: 10.1371/journal.pone.0166976
141. Smith SM, Dworkin RH, Turk DC, Baron R, Polydefkis M, Tracey I, et al. The potential role of sensory testing, skin biopsy, and functional brain imaging as biomarkers in chronic pain clinical trials: IMMPACT considerations. J Pain. (2017) 18:757–77. doi: 10.1016/j.jpain.2017.02.429
142. Sloan G, Shillo P, Selvarajah D, Wu J, Wilkinson ID, Tracey I, et al. A new look at painful diabetic neuropathy. Diabetes Res Clin Pract. (2018) 144:177–91. doi: 10.1016/j.diabres.2018.08.020
143. Evans MC, Wade C, Hohenschurz-Schmidt D, Lally P, Ugwudike A, Shah K, et al. Magnetic resonance imaging as a biomarker in diabetic and HIV-associated peripheral neuropathy: a systematic review-based narrative. Front Neurosci. (2021) 15:727311. doi: 10.3389/fnins.2021.727311
144. Matthewson GM, Woo CW, Reddan MC, Wager TD. Cognitive self-regulation influences pain-related physiology. Pain. (2019) 160:2338–49. doi: 10.1097/j.pain.0000000000001621
145. Eisenach JC, Curry R, Aschenbrenner CA, Coghill RC, Houle TT. Pupil responses and pain ratings to heat stimuli: reliability and effects of expectations and a conditioning pain stimulus. J Neurosci Methods. (2017) 279:52–9. doi: 10.1016/j.jneumeth.2017.01.005
146. Mantyselka P, Ali-Sisto T, Kautiainen H, Niskanen L, Viinamaki H, Velagapudi V, et al. The association between musculoskeletal pain and circulating ornithine: a population-based study. Pain Med. (2017) 18:1145–51. doi: 10.1093/pm/pnw285
147. Hellstrom F, Gouveia-Figueira S, Nording ML, Bjorklund M, Fowler CJ. Association between plasma concentrations of linoleic acid-derived oxylipins and the perceived pain scores in an exploratory study in women with chronic neck pain. BMC Musculoskelet Disord. (2016) 17:103. doi: 10.1186/s12891-016-0951-9
148. Sowa GA, Perera S, Bechara B, Agarwal V, Boardman J, Huang W, et al. Associations between serum biomarkers and pain and pain-related function in older adults with low back pain: a pilot study. J Am Geriatr Soc. (2014) 62:2047–55. doi: 10.1111/jgs.13102
149. Diz JBM, de Souza Moreira B, Felicio DC, Teixeira LF, de Jesus-Moraleida FR, de Queiroz BZ, et al. Brain-derived neurotrophic factor plasma levels are increased in older women after an acute episode of low back pain. Arch Gerontol Geriatr. (2017) 71:75–82. doi: 10.1016/j.archger.2017.03.005
150. Goode AP, Schwartz TA, Kraus VB, Huebner JL, George SZ, Cleveland RJ, et al. Inflammatory, structural, and pain biochemical biomarkers may reflect radiographic disc space narrowing: the Johnston county osteoarthritis project. J Orthop Res. (2020) 38:1027–37. doi: 10.1002/jor.24534
151. Dawson A, Stensson N, Ghafouri B, Gerdle B, List T, Svensson P, et al. Dopamine in plasma - a biomarker for myofascial TMD pain? J Headache Pain. (2016) 17:65. doi: 10.1186/s10194-016-0656-3
152. Ferland CE, Teles AR, Ingelmo P, Saran N, Marchand S, Ouellet JA. Blood monoamines as potential biomarkers for conditioned pain modulation efficacy: an exploratory study in paediatrics. Eur J Pain. (2019) 23:327–40. doi: 10.1002/ejp.1307
153. Hammers A, Allom R, Koepp MJ, Free SL, Myers R, Lemieux L, et al. Three-dimensional maximum probability atlas of the human brain, with particular reference to the temporal lobe. Hum Brain Mapp. (2003) 19:224–47. doi: 10.1002/hbm.10123
154. Fox MD, Snyder AZ, Vincent JL, Corbetta M, Van Essen DC, Raichle ME. The human brain is intrinsically organized into dynamic, anticorrelated functional networks. Proc Natl Acad Sci USA. (2005) 102:9673–8. doi: 10.1073/pnas.0504136102
155. Raichle ME. The restless brain: how intrinsic activity organizes brain function. Philos Trans R Soc Lond B Biol Sci. (2015) 370, 20140172. doi: 10.1098/rstb.2014.0172
156. Heine L, Soddu A, Gomez F, Vanhaudenhuyse A, Tshibanda L, Thonnard M, et al. Resting state networks and consciousness: alterations of multiple resting state network connectivity in physiological, pharmacological, and pathological consciousness States. Front Psychol. (2012) 3:295. doi: 10.3389/fpsyg.2012.00295
157. Kucyi A, Davis KD. The dynamic pain connectome. Trends Neurosci. (2015) 38:86–95. doi: 10.1016/j.tins.2014.11.006
158. Fields HL. Understanding how opioids contribute to reward and analgesia. Reg Anesth Pain Med. (2007) 32:242–6. doi: 10.1016/j.rapm.2007.01.001
159. Kucyi A, Davis KD. The neural code for pain: from single-cell electrophysiology to the dynamic pain connectome. Neuroscientist. (2017) 23:397–414. doi: 10.1177/1073858416667716
160. Apkarian AV, Sosa Y, Sonty S, Levy RM, Harden RN, Parrish TB, et al. Chronic back pain is associated with decreased prefrontal and thalamic gray matter density. J Neurosci. (2004) 24:10410–5. doi: 10.1523/JNEUROSCI.2541-04.2004
161. Baliki MN, Schnitzer TJ, Bauer WR, Apkarian AV. Brain morphological signatures for chronic pain. PLoS ONE. (2011) 6:e26010. doi: 10.1371/journal.pone.0026010
162. May A. Structural brain imaging: a window into chronic pain. Neuroscientist. (2011) 17:209–20. doi: 10.1177/1073858410396220
163. Smallwood RF, Laird AR, Ramage AE, Parkinson AL, Lewis J, Clauw DJ, et al. Structural brain anomalies and chronic pain: a quantitative meta-analysis of gray matter volume. J Pain. (2013) 14:663–75. doi: 10.1016/j.jpain.2013.03.001
164. Rodriguez-Raecke R, Niemeier A, Ihle K, Ruether W, May A. Brain gray matter decrease in chronic pain is the consequence and not the cause of pain. J Neurosci. (2009) 29:13746–50. doi: 10.1523/JNEUROSCI.3687-09.2009
165. Borsook D. Neurological diseases and pain. Brain. (2012) 135 (Pt. 2):320–44. doi: 10.1093/brain/awr271
166. Baliki MN, Mansour AR, Baria AT, Apkarian AV. Functional reorganization of the default mode network across chronic pain conditions. PLoS ONE. (2014) 9:e106133. doi: 10.1371/journal.pone.0106133
167. Ichesco E, Quintero A, Clauw DJ, Peltier S, Sundgren PM, Gerstner GE, et al. Altered functional connectivity between the insula and the cingulate cortex in patients with temporomandibular disorder: a pilot study. Headache. (2012) 52:441–54. doi: 10.1111/j.1526-4610.2011.01998.x
168. Loggia ML, Kim J, Gollub RL, Vangel MG, Kirsch I, Kong J, et al. Default mode network connectivity encodes clinical pain: an arterial spin labeling study. Pain. (2013) 154:24–33. doi: 10.1016/j.pain.2012.07.029
169. Napadow V, LaCount L, Park K, As-Sanie S, Clauw DJ, Harris RE. Intrinsic brain connectivity in fibromyalgia is associated with chronic pain intensity. Arthritis Rheum. (2010) 62:2545–55. doi: 10.1002/art.27497
170. Tagliazucchi E, Balenzuela P, Fraiman D, Chialvo DR. Brain resting state is disrupted in chronic back pain patients. Neurosci Lett. (2010) 485:26–31. doi: 10.1016/j.neulet.2010.08.053
171. Cauda F, Sacco K, Duca S, Cocito D, D'Agata F, Geminiani GC, et al. Altered resting state in diabetic neuropathic pain. PLoS ONE. (2009) 4:e4542. doi: 10.1371/journal.pone.0004542
172. Kucyi A, Moayedi M, Weissman-Fogel I, Goldberg MB, Freeman BV, Tenenbaum HC, et al. Enhanced medial prefrontal-default mode network functional connectivity in chronic pain and its association with pain rumination. J Neurosci. (2014) 34:3969–75. doi: 10.1523/JNEUROSCI.5055-13.2014
173. Kucyi A, Salomons TV, Davis KD. Mind wandering away from pain dynamically engages antinociceptive and default mode brain networks. Proc Natl Acad Sci USA. (2013) 110:18692–7. doi: 10.1073/pnas.1312902110
174. Baliki MN, Petre B, Torbey S, Herrmann KM, Huang L, Schnitzer TJ, et al. Corticostriatal functional connectivity predicts transition to chronic back pain. Nat Neurosci. (2012) 15:1117–9. doi: 10.1038/nn.3153
175. Apkarian AV, Baliki MN, Farmer MA. Predicting transition to chronic pain. Curr Opin Neurol. (2013) 26:360–7. doi: 10.1097/WCO.0b013e32836336ad
176. Vachon-Presseau E, Tetreault P, Petre B, Huang L, Berger SE, Torbey S, et al. Corticolimbic anatomical characteristics predetermine risk for chronic pain. Brain. (2016) 139 (Pt. 7):1958–70. doi: 10.1093/brain/aww100
177. Schafer SM, Geuter S, Wager TD. Mechanisms of placebo analgesia: a dual-process model informed by insights from cross-species comparisons. Prog Neurobiol. (2018) 160:101–22. doi: 10.1016/j.pneurobio.2017.10.008
178. Tetreault P, Baliki MN, Baria AT, Bauer WR, Schnitzer TJ, Apkarian AV. Inferring distinct mechanisms in the absence of subjective differences: placebo and centrally acting analgesic underlie unique brain adaptations. Hum Brain Mapp. (2018) 39:2210–23. doi: 10.1002/hbm.23999
179. Vachon-Presseau E, Berger SE, Abdullah TB, Huang L, Cecchi GA, Griffith JW, et al. Brain and psychological determinants of placebo pill response in chronic pain patients. Nat Commun. (2018) 9:3397. doi: 10.1038/s41467-018-05859-1
180. Crawford LS, Mills EP, Hanson T, Macey PM, Glarin R, Macefield VG, et al. Brainstem mechanisms of pain modulation: a within-subjects 7T fMRI study of placebo analgesic and nocebo hyperalgesic responses. J Neurosci. (2021) 41:9794–806. doi: 10.1523/JNEUROSCI.0806-21.2021
181. Davis KD, Cheng JC. Differentiating trait pain from state pain: a window into brain mechanisms underlying how we experience and cope with pain. Pain Rep. (2019) 4:e735. doi: 10.1097/PR9.0000000000000735
182. Hemington KS, Rogachov A, Cheng JC, Bosma RL, Kim JA, Osborne NR, et al. Patients with chronic pain exhibit a complex relationship triad between pain, resilience, and within- and cross-network functional connectivity of the default mode network. Pain. (2018) 159:1621–30. doi: 10.1097/j.pain.0000000000001252
183. Rogachov A, Cheng JC, Erpelding N, Hemington KS, Crawley AP, Davis KD. Regional brain signal variability: a novel indicator of pain sensitivity and coping. Pain. (2016) 157:2483–92. doi: 10.1097/j.pain.0000000000000665
184. Tesfaye S, Selvarajah D, Gandhi R, Greig M, Shillo P, Fang F, et al. Diabetic peripheral neuropathy may not be as its name suggests: evidence from magnetic resonance imaging. Pain. (2016) 157 (Suppl. 1):S72–80. doi: 10.1097/j.pain.0000000000000465
185. Feldman EL, Nave KA, Jensen TS, Bennett DL. New horizons in diabetic neuropathy: mechanisms, bioenergetics, and pain. Neuron. (2017) 93:1296–313. doi: 10.1016/j.neuron.2017.02.005
186. Selvarajah D, Wilkinson ID, Emery CJ, Shaw PJ, Griffiths PD, Gandhi R, et al. Thalamic neuronal dysfunction and chronic sensorimotor distal symmetrical polyneuropathy in patients with type 1 diabetes mellitus. Diabetologia. (2008) 51:2088–92. doi: 10.1007/s00125-008-1139-0
187. Sorensen L, Siddall PJ, Trenell MI, Yue DK. Differences in metabolites in pain-processing brain regions in patients with diabetes and painful neuropathy. Diabetes Care. (2008) 31:980–1. doi: 10.2337/dc07-2088
188. Selvarajah D, Wilkinson ID, Gandhi R, Griffiths PD, Tesfaye S. Microvascular perfusion abnormalities of the thalamus in painful but not painless diabetic polyneuropathy: a clue to the pathogenesis of pain in type 1 diabetes. Diabetes Care. (2011) 34:718–20. doi: 10.2337/dc10-1550
189. Eaton SE, Harris ND, Rajbhandari SM, Greenwood P, Wilkinson ID, Ward JD, et al. Spinal-cord involvement in diabetic peripheral neuropathy. Lancet. (2001) 358:35–6. doi: 10.1016/S0140-6736(00)05268-5
190. Selvarajah D, Wilkinson ID, Emery CJ, Harris ND, Shaw PJ, Witte DR, et al. Early involvement of the spinal cord in diabetic peripheral neuropathy. Diabetes Care. (2006) 29:2664–9. doi: 10.2337/dc06-0650
191. Casseb RF, de Paiva JL, Branco LM, Martinez AR, Reis F, de Lima-Junior JC, et al. Spinal cord diffusion tensor imaging in patients with sensory neuronopathy. Neuroradiology. (2016) 58:1103–8. doi: 10.1007/s00234-016-1738-2
192. Selvarajah D, Wilkinson ID, Maxwell M, Davies J, Sankar A, Boland E, et al. Magnetic resonance neuroimaging study of brain structural differences in diabetic peripheral neuropathy. Diabetes Care. (2014) 37:1681–8. doi: 10.2337/dc13-2610
193. Manor B, Newton E, Abduljalil A, Novak V. The relationship between brain volume and walking outcomes in older adults with and without diabetic peripheral neuropathy. Diabetes Care. (2012) 35:1907–12. doi: 10.2337/dc11-2463
194. Cauda F, Sacco K, D'Agata F, Duca S, Cocito D, Geminiani G, et al. Low-frequency BOLD fluctuations demonstrate altered thalamocortical connectivity in diabetic neuropathic pain. BMC Neurosci. (2009) 10:138. doi: 10.1186/1471-2202-10-138
195. Cauda F, D'Agata F, Sacco K, Duca S, Cocito D, Paolasso I, et al. Altered resting state attentional networks in diabetic neuropathic pain. J Neurol Neurosurg Psychiatry. (2010) 81:806–11. doi: 10.1136/jnnp.2009.188631
196. Tseng MT, Chiang MC, Chao CC, Tseng WY, Hsieh ST. fMRI evidence of degeneration-induced neuropathic pain in diabetes: enhanced limbic and striatal activations. Hum Brain Mapp. (2013) 34:2733–46. doi: 10.1002/hbm.22105
197. Teh K, Wilkinson ID, Heiberg-Gibbons F, Awadh M, Kelsall A, Pallai S, et al. Somatosensory network functional connectivity differentiates clinical pain phenotypes in diabetic neuropathy. Diabetologia. (2021) 64:1412–21. doi: 10.1007/s00125-021-05416-4
198. Chao CC, Tseng MT, Hsieh PC, Lin CJ, Huang SL, Hsieh ST, et al. Brain mechanisms of pain and dysautonomia in diabetic neuropathy: connectivity changes in thalamus and hypothalamus. J Clin Endocrinol Metab. (2022) 107:e1167–80. doi: 10.1210/clinem/dgab754
199. Zhang Y, Qu M, Yi X, Zhuo P, Tang J, Chen X, et al. Sensorimotor and pain-related alterations of the gray matter and white matter in Type 2 diabetic patients with peripheral neuropathy. Hum Brain Mapp. (2020) 41:710–25. doi: 10.1002/hbm.24834
200. Selvarajah D, Wilkinson ID, Fang F, Sankar A, Davies J, Boland E, et al. Structural and functional abnormalities of the primary somatosensory cortex in diabetic peripheral neuropathy: a multimodal MRI study. Diabetes. (2019) 68:796–806. doi: 10.2337/db18-0509
201. Watanabe K, Hirano S, Kojima K, Nagashima K, Mukai H, Sato T, et al. Altered cerebral blood flow in the anterior cingulate cortex is associated with neuropathic pain. J Neurol Neurosurg Psychiatry. (2018) 89:1082–7. doi: 10.1136/jnnp-2017-316601
202. Segerdahl AR, Themistocleous AC, Fido D, Bennett DL, Tracey I. A brain-based pain facilitation mechanism contributes to painful diabetic polyneuropathy. Brain. (2018) 141:357–64. doi: 10.1093/brain/awx337
203. Keltner JR, Fennema-Notestine C, Vaida F, Wang D, Franklin DR, Dworkin RH, et al. HIV-associated distal neuropathic pain is associated with smaller total cerebral cortical gray matter. J Neurovirol. (2014) 20:209–18. doi: 10.1007/s13365-014-0236-8
204. Keltner JR, Connolly CG, Vaida F, Jenkinson M, Fennema-Notestine C, Archibald S, et al. HIV distal neuropathic pain is associated with smaller ventral posterior cingulate cortex. Pain Med. (2017) 18:428–40. doi: 10.1093/pm/pnw180
205. Zahr NM, Pohl KM, Pfefferbaum A, Sullivan EV. Dissociable contributions of precuneus and cerebellum to subjective and objective neuropathy in HIV. J Neuroimmune Pharmacol. (2019) 14:436–47. doi: 10.1007/s11481-019-09837-2
206. Keltner JR, Tong A, Visser E, Jenkinson M, Connolly CG, Dasca A, et al. Evidence for a novel subcortical mechanism for posterior cingulate cortex atrophy in HIV peripheral neuropathy. J Neurovirol. (2020) 26:530–43. doi: 10.1007/s13365-020-00850-3
207. Timtim S, Simmons A, Keltner JR. HIV peripheral neuropathy-related degeneration of white matter tracts to sensorimotor cortex. Neurovirology. (2022).
208. Hays C, Simmons A, Keltner JR. Decreased default mode functional connectivity and increased salience network connectivity associated with HIV distal nueuropathic pain. Currently under review. (2022).
209. Strigo IA, Keltner JR, Ellis RJ, Simmons AN. Association of painful human immunodeficiency virus distal sensory polyneuropathy with aberrant expectation of pain relief: functional magnetic resonance imaging evidence. Brain Commun. (2021) 3:fcab260. doi: 10.1093/braincomms/fcab260
210. Nudelman KN, McDonald BC, Wang Y, Smith DJ, West JD, O'Neill DP, et al. Cerebral perfusion and gray matter changes associated with chemotherapy-induced peripheral neuropathy. J Clin Oncol. (2016) 34:677–83. doi: 10.1200/JCO.2015.62.1276
211. Boland EG, Selvarajah D, Hunter M, Ezaydi Y, Tesfaye S, Ahmedzai SH, et al. Central pain processing in chronic chemotherapy-induced peripheral neuropathy: a functional magnetic resonance imaging study. PLoS ONE. (2014) 9:e96474. doi: 10.1371/journal.pone.0096474
212. Hsieh PC, Tseng MT, Chao CC, Lin YH, Tseng WY, Liu KH, et al. Imaging signatures of altered brain responses in small-fiber neuropathy: reduced functional connectivity of the limbic system after peripheral nerve degeneration. Pain. (2015) 156:904–16. doi: 10.1097/j.pain.0000000000000128
213. Chao CC, Tseng MT, Lin YH, Hsieh PC, Lin CJ, Huang SL, et al. Brain imaging signature of neuropathic pain phenotypes in small-fiber neuropathy: altered thalamic connectome and its associations with skin nerve degeneration. Pain. (2021) 162:1387–99. doi: 10.1097/j.pain.0000000000002155
214. Lee M, Park CH, Chung HK, Kim HJ, Choi Y, Yoo JH, et al. Cerebral white matter abnormalities in patients with charcot-marie-tooth disease. Ann Neurol. (2017) 81:147–51. doi: 10.1002/ana.24824
215. Wang WW, Song CL, Huang L, Song QW, Liang ZH, Wei Q, et al. DTI study of cerebral normal-appearing white matter in hereditary neuropathy with liability to pressure palsies (HNPP). Medicine (Baltimore). (2015) 94:e1909. doi: 10.1097/MD.0000000000001909
216. Maeda Y, Kettner N, Kim J, Kim H, Cina S, Malatesta C, et al. Primary somatosensory/motor cortical thickness distinguishes paresthesia-dominant from pain-dominant carpal tunnel syndrome. Pain. (2016) 157:1085–93. doi: 10.1097/j.pain.0000000000000486
217. Rocca MA, Valsasina P, Fazio R, Previtali SC, Messina R, Falini A, et al. Brain connectivity abnormalities extend beyond the sensorimotor network in peripheral neuropathy. Hum Brain Mapp. (2014) 35:513–26. doi: 10.1002/hbm.22198
218. Reddan MC, Wager TD. Modeling pain using fMRI: from regions to biomarkers. Neurosci Bull. (2018) 34:208–15. doi: 10.1007/s12264-017-0150-1
219. Cowen R, Stasiowska MK, Laycock H, Bantel C. Assessing pain objectively: the use of physiological markers. Anaesthesia. (2015) 70:828–47. doi: 10.1111/anae.13018
220. Malatji BG, Meyer H, Mason S, Engelke UFH, Wevers RA, van Reenen M, et al. A diagnostic biomarker profile for fibromyalgia syndrome based on an NMR metabolomics study of selected patients and controls. BMC Neurol. (2017) 17:88. doi: 10.1186/s12883-017-0863-9
221. Hackshaw KV, Aykas DP, Sigurdson GT, Plans M, Madiai F, Yu L, et al. Metabolic fingerprinting for diagnosis of fibromyalgia and other rheumatologic disorders. J Biol Chem. (2019) 294:2555–68. doi: 10.1074/jbc.RA118.005816
222. Egsgaard LL, Eskehave TN, Bay-Jensen AC, Hoeck HC, Arendt-Nielsen L. Identifying specific profiles in patients with different degrees of painful knee osteoarthritis based on serological biochemical and mechanistic pain biomarkers: a diagnostic approach based on cluster analysis. Pain. (2015) 156:96–107. doi: 10.1016/j.pain.0000000000000011
223. Gerdle B, Ghafouri B, Ghafouri N, Backryd E, Gordh T. Signs of ongoing inflammation in female patients with chronic widespread pain: a multivariate, explorative, cross-sectional study of blood samples. Medicine. (2017) 96:e6130. doi: 10.1097/MD.0000000000006130
224. Teckchandani S, Nagana Gowda GA, Raftery D, Curatolo M. Metabolomics in chronic pain research. Euro J Pain. (2021) 25:313–26. doi: 10.1002/ejp.1677
225. Saeys Y, Inza I, Larranaga P. A review of feature selection techniques in bioinformatics. Bioinformatics. (2007) 23:2507–17. doi: 10.1093/bioinformatics/btm344
227. LeCun Y, Bengio Y, Hinton G. Deep learning. Nature. (2015) 521:436–44. doi: 10.1038/nature14539
228. Zou H. Regularization and variable selection via the elastic net. J R Stat Soc Ser B. (2005) 67:301–20. doi: 10.1111/j.1467-9868.2005.00503.x
229. Jain A. Data clustering: a review. ACM Comput Surv. (1999) 31:264–323. doi: 10.1145/331499.331504
230. Li J. Feature selection: a data perspective. ACM Comput Surv. (2017) 50:1–45. doi: 10.1145/3136625
231. Hinton GE, Salakhutdinov RR. Reducing the dimensionality of data with neural networks. Science. (2006) 313:504–7. doi: 10.1126/science.1127647
232. Hardoon D. Canonical correlation analysis: an overview with application to learning methods. Neural Comput. (2004) 16:2639–64. doi: 10.1162/0899766042321814
233. Wold S. Principal component analysis. Chemometr Intell Lab Syst. (1987) 2:37–52. doi: 10.1016/0169-7439(87)80084-9
Keywords: pain, biomarker, neuropathic, endocannabinoid, inflammation, opiate
Citation: Diaz MM, Caylor J, Strigo I, Lerman I, Henry B, Lopez E, Wallace MS, Ellis RJ, Simmons AN and Keltner JR (2022) Toward Composite Pain Biomarkers of Neuropathic Pain—Focus on Peripheral Neuropathic Pain. Front. Pain Res. 3:869215. doi: 10.3389/fpain.2022.869215
Received: 04 February 2022; Accepted: 21 April 2022;
Published: 11 May 2022.
Edited by:
Sandra Carvalho, University of Aveiro, PortugalReviewed by:
Paul Jonathon Austin, The University of Sydney, AustraliaXu-Yun Hua, Shanghai University of Traditional Chinese Medicine, China
Copyright © 2022 Diaz, Caylor, Strigo, Lerman, Henry, Lopez, Wallace, Ellis, Simmons and Keltner. This is an open-access article distributed under the terms of the Creative Commons Attribution License (CC BY). The use, distribution or reproduction in other forums is permitted, provided the original author(s) and the copyright owner(s) are credited and that the original publication in this journal is cited, in accordance with accepted academic practice. No use, distribution or reproduction is permitted which does not comply with these terms.
*Correspondence: Monica M. Diaz, bW9uaWNhLmRpYXpAbmV1cm9sb2d5LnVuYy5lZHU=