- 1Center for Oral Health Research, College of Dentistry, University of Kentucky, Lexington, KY, United States
- 2Department of Biomedical Sciences, School of Dental Medicine, University of Nevada Las Vegas, Las Vegas, NV, United States
Introduction: Periodontitis is delineated by a dysbiotic microbiome at sites of lesions accompanied by a dysregulated persistent inflammatory response that undermines the integrity of the periodontium. The interplay of the altered microbial ecology and warning signals from host cells would be a critical feature for maintaining or re-establishing homeostasis in these tissues.
Methods: This study used a nonhuman primate model (Macaca mulatta) with naturally-occurring periodontitis (n = 34) and experimental ligature-induced periodontitis (n = 36) to describe the features of gene expression for an array of damage-associate molecular patterns (DAMPs) or alarmins within the gingival tissues. The animals were age stratified into: ≤3 years (Young), 7–12 years (Adolescent), 12–15 years (Adult) and 17–23 years (Aged). Gingival tissue biopsies were examined via microarray. The analysis focused on 51 genes representative of the DAMPs/alarmins family of host cell warning factors and 18 genes associated with tissue destructive processed in the gingival tissues. Bacterial plaque samples were collected by curette sampling and 16S rRNA gene sequences used to describe the oral microbiome.
Results: A subset of DAMPs/alarmins were expressed in healthy and naturally-occurring periodontitis tissues in the animals and suggested local effects on gingival tissues leading to altered levels of DAMPs/alarmins related to age and disease. Significant differences from adult healthy levels were most frequently observed in the young and adolescent animals with few representatives in this gene array altered in the healthy aged gingival tissues. Of the 51 target genes, only approximately ⅓ were altered by ≥1.5-fold in any of the age groups of animals during disease, with those increases observed during disease initiation. Distinctive positive and negative correlations were noted with the DAMP/alarmin gene levels and comparative expression changes of tissue destructive molecules during disease across the age groups. Finally, specific correlations of DAMP/alarmin genes and relative abundance of particular microbes were observed in health and resolution samples in younger animals, while increased correlations during disease in the older groups were noted.
Conclusions: Thus, using this human-like preclinical model of induced periodontitis, we demonstrated the dynamics of the activation of the DAMP/alarmin warning system in the gingival tissues that showed some specific differences based on age.
Introduction
Gingival tissues are constantly subjected to a range of stressors in the oral environment, many derived from the continuous challenge of the complex oral microbial ecology existing as biofilms juxtaposed to the tissues, including proteases (1–7), exotoxins (8–11), and endotoxins (12–19) among many other bacterial molecules. Moreover, these stimuli create a level of inflammation with associated inflammatory molecules derived from both resident cells and inflammatory cells that have emigrated from the vasculature in response to the local challenge (20–26). These activities often create a microenvironment for the cells that is increased in temperature (27–30), and pH (31–33), show altered electrolyte levels (34, 35), and elevated concentrations of reactive oxygen and nitrogen species (36–39) that can affect the physiology of the gingival tissue cells. Chronicity of this type of environment leads to destruction of both the soft tissues and alveolar bone, which result in the clinical measures of attachment loss and radiographic evidence of bone resorption that describe periodontitis.
It is also well recognized that the incidence and severity of periodontitis is increased with aging, leading to a “paradigm” of periodontitis as a frequent disease in the population reflecting long-term continuous noxious challenge to the tissues, which finally succumb to the destructive nature of the chronic stimuli (40–44). However, evidence provided over the last few decades has emphasized that the tissue destruction in periodontitis most likely is a manifestation of the chronic inflammatory response of the host to the complex microbial challenge that increases in quantity, as well as enabling the emergence of more pathogenic species to stimulate elevated levels of tissue destructive molecules (25, 45, 46). This view is also supported by ongoing genomic studies suggesting linkages between alterations in inflammatory gene expression and enhanced risk for development of periodontitis (47–52). Recent investigations have begun to emphasize that this chronic inflammation with tissue destruction is not a unidirectional process. Molecular studies have identified anti-inflammatory cytokines (53–59), pathways of inflammation resolving molecules (60–64), and molecules recognized as danger-associated molecular patterns (DAMPs) or alarmins (65–68) recognized by host cells to help minimize tissue damage and help to control destructive processes.
This report describes our studies using gingival tissues, as a representative mucosal tissue, derived from nonhuman primates (Macaca mulatta). We evaluated the expression of the DAMPs/alarmins that accompany healthy aging of these tissues. Finally, alterations in the expression of the DAMP/alarmin gene distribution and levels that reflect naturally-occurring and experimental periodontal disease across the lifespan and related to features of the oral microbiome in health and disease were delineated.
Methods
Nonhuman primate model and oral clinical evaluation
A population of rhesus monkeys (Macaca mulatta) housed at the Caribbean Primate Research Center (CPRC) at Sabana Seca, Puerto Rico, were used in this report and the previously published studies (69–72). “A protocol approved by the Institutional Animal Care and Use Committee (IACUC) of the University of Puerto Rico, enabled anesthetized animals to be examined for clinical measures of periodontal parameters including probing pocket depth (PPD), and bleeding on probing (BOP) as we have described previously (73). The nonhuman primates are typically fed a 20% protein, 5% fat, and 10% fiber commercial monkey diet (diet 8773, Teklad NIB primate diet modified: Harlan Teklad). The diet is supplemented with fruits and vegetables, and water is provided ad libitum in an enclosed corral setting” (74).
“For the naturally-occurring periodontitis model 34 animals were used (14 females and 20 males) reported in (75). Healthy animals (5–7/group) were distributed by age in 4 groups as follows: ≤3 years (young), 7–12 years (adolescent), 12–15 years (adult), and 18–22 years (aged). Only adult (n = 5) and aged animals (n = 6) with naturally-occurring periodontitis were used, since periodontitis does not occur in young animals. For this cohort, a clinical examination included probing pocket depth (PD) and bleeding on probing (BOP; 0–3 scale) (73). Clinical characterization for each group included: young and adolescent animals (mean PD < 2.0 mm, mean BOP < 0.5); adult healthy group (mean PD < 2.0 mm, mean BOP < 0.3); aged healthy group (mean PD < 3.0 mm, mean BOP < 1); adult periodontitis group (mean PD > 3.4 mm, mean BOP > 1.0); mean BOP < 1.0); and aged periodontitis group (mean PD > 3.4 mm, mean BOP > 1.5) described in (76).”
“For the longitudinal experimental ligature study, healthy animals were distributed by age into four groups of 9 animals/group (n = 36; 19 females and 17 males): ≤ 3 years (Young), 7–12 years (Adolescent), 12–15 years (Adult) and 17–23 years (Aged). A full mouth examination, excluding 3rd molars and canines, by a single investigator using a Maryland probe on the facial aspect of the teeth, e.g., 2 interproximal sites/tooth (mesio- and disto-buccal) was conducted. The healthy animals classified by a mean BOP of ≤1.0 and mean PPD of ≤3.0 were included. The animals then participated in a standard experimental ligature-induced periodontitis study (69, 72). Baseline (healthy) gingival tissues were biopsied from each animal from a single site prior to the experimental periodontitis procedure. Ligature-induced periodontal disease was then initiated whereby 2nd premolar and 1st and 2nd molar teeth in all 4 quadrants were ligated by tying 3–0 non-resorbable silk sutures around the cementoenamel junction of each tooth and using a periodontal probe to position the ligatures below the gingival margin. The methods were carried out in accordance with all relevant regulations for the use of nonhuman primates following ARRIVE guidelines (https://arriveguidelines.org/resources/author-checklists).”
“Further, clinical evaluation of ligated sites and gingival tissue samples were obtained at 0.5 (initiation), 1 (early progression), and 3 months (late progression). Determination of periodontal disease at the sampled site was documented by assessment of the presence of BOP and probing pocket depth of >4 mm, as we have described previously (69, 77). Then, ligatures were removed after sampling at 3 months and samples taken 2 months later (resolution) (69, 77). Since the removal of the ligature eliminates the local noxious mechanical challenge and decreases the microbial burden accumulating on the tooth, this process is similar to nonsurgical periodontal therapy in humans. Our previous reports have documented the significant increase in inflammation in all age groups of animals within the first two weeks of ligature placement identified as disease initiation. Additionally, we have shown that adult and aged animals demonstrated significantly greater destructive disease compared to young and adolescent animals in this experimental periodontitis model (78). These methods have been reported previously in (79).”
Tissue sampling and gene expression microarray analysis
“A buccal gingival sample from either healthy or periodontitis-affected tissue from the premolar/molar maxillary region of each animal was taken at each time point using a standard gingivectomy technique (80), and maintained frozen in RNAlater solution. Total RNA was isolated from each gingival tissue using a standard procedure as we have described and tissue RNA samples submitted to the microarray core to assess RNA quality analyze the transcriptome using the GeneChip® Rhesus Macaque Genome Array (Affymetrix) (76, 81). Each animal provided 1 gingival biopsy for gene expression analysis at each time point. Thus, individual samples were used for the analytics. The genes explored in this study focused on 48 genes representative of this family of host biomolecules (Table 1). It must be recognized that the genes selected for the analysis represent those described in the literature as related to this biological warning process across the gingival cell population, and do not specifically address the cell types displaying these changes within the complex of the gingival tissues (74).”
We have also published previously regarding qPCR validation of the microarray results for 57 genes with altered expression related to age and/or disease, including SAA, S100A8, FN1, and IL1A from this targeted dataset (72, 75, 82–90). Generally, across this range of genes, the findings were consistent both directionally and in magnitude of differential expression.
Microbiome and data analysis
“Bacterial plaque samples were collected by curette sampling from the subgingival pocket juxtaposed to the site providing the tissue sample at each time point. Only one sample was obtained at a time point from each animal, and the site was only sampled a single time. The plaque was suspended in sterile PBS and centrifuged for 10 min at 14,000 g to pellet the bacterial cells. DNA was extracted from the pelleted cells using a MagNA Pure LC DNA isolation kit III (Roche Applied Science) in MagNA Pure LC automated nucleic acid purification system (Roche Applied Science). DNA was quantified using a Nanodrop (ThermoFisher). The range of DNA obtained from the microbiome samples was 100 ng to 2.1 µg, with generally lower concentrations in baseline (healthy) samples. 5 ng of DNA was used in PCR and subsequently equimolar concentrations used for sequencing all samples as reported previously (74).”
“A Dual index paired-end sequencing approach for sequencing (91) using a MiSeq sequencer instrument was employed to determine the total composition of the oral microbiota from each sample as we have described previously (70, 92). First DNA from each sample was amplified using 16S universal primers, which amplifies 254 bp from V4 region of the 16S rRNA gene. Each primer consisted of the appropriate Illumina adapter, an 8-nt index sequence to identify each sample, a 10-nt pad sequence, 2-nt linker and the gene specific 16S forward or 16S reverse primer sequence. The resulting 254 bp 16S rRNA gene amplicons generated from each sample was purified and pooled in equimolar concentrations. These amplicon libraries were mixed with Illumina-generated PhiX control libraries and used for shotgun library construction using Nextera XT genomic library construction protocol. Sequencing of each DNA fragment was done using two sequence reads and two index reads by three sequence primers. The overall process of cluster generation, sequencing, image processing, de-multiplexing and quality score calculation was performed on the MiSeq instrument (Illumina).
Barcoded sequences from the MiSeq run were trimmed and aligned to similar 16S rRNA sequences from the SILVA version4 rRNA database (https://www.arb-silva.de/) using MOTHUR (v.1.38.0) pipeline (93). Artificial erroneous reads were corrected using the pre.cluster mothur command, and chimeric sequences were removed using the UCHIME (94) command in MOTHUR pipeline, followed by removal of non-archaeal/bacterial sequence removal. Sequences were clustered into phylotypes based on their sequence similarity and these binned phylotypes were assigned to their respective taxonomic classification using the Human Oral Microbiome Database (HOMD V13) (https://www.homd.org/ftp/16S_rRNA_refseq/HOMD_16S_rRNA_RefSeq/). We used the HOMD database to classify the OTUs. Since the study classified bacteria in the oral microbiome of rhesus monkeys by comparing to bacterial species present in humans (ie. HOMD) there were bacteria sequences identified at the genus level but unclassified by further species comparison since they are not sufficiently similar to existing entries present in the HOMD database. The cutoff percentage used in the MOTHUR software (classify.seqs command) for classifying sequences is 80%, which would leave some OTUs unclassified or classify only at the genus level. The raw data are deposited at BioProject ID PRJNA516659 through the NIH NCBI. The bacterial OTUs targeted in this report are provided in Table 2 and reported previously (70, 74).”
Statistical analysis
“The details of the gene expression intensities across the samples as reported previously (79) were estimated using the Robust Multi-array Average (RMA) algorithm with probe-level quintile normalization, as implemented in the Partek Genomics Suite software version 6.6 (Partek, St. Louis, MO). The data have been uploaded into GEO accession GSE180588 (https://www.ncbi.nlm.nih.gov/gds). Statistical differences of bacterial OTUs were determined with a t-test at least at p < 0.05. Correlation analyses were determined using a Pearson Correlation Coefficient with a p-value defined as p < 0.01 for the OTU-DAMP/alarmin gene comparisons.”
Results
DAMP/alarmin gene expression profiles in gingival tissues
The initial studies examined the levels of DAMPs/alarmins expressed in healthy and naturally-occurring periodontitis tissues in the animals (Figure 1). There results demonstrated some prominent genes expressed in healthy gingival tissues in all age groups. These included ACTG1, ANXA1, DCN, HMGB1, S100A8 and S100A9, APOE/ß2-GPI, IL33, and LTF. A number of these genes were also significantly increased in aging and periodontitis tissues (e.g., ANXA1, HMGB1, S100A8, S100A9, APOE/ß2-GPI, LTF, TSLP). Additionally, altered levels of DEF103B and IL1A were seen in younger healthy tissues. These results suggested local effects on gingival tissues leading to altered levels of DAMPs/alarmins related to age and naturally-occurring disease.
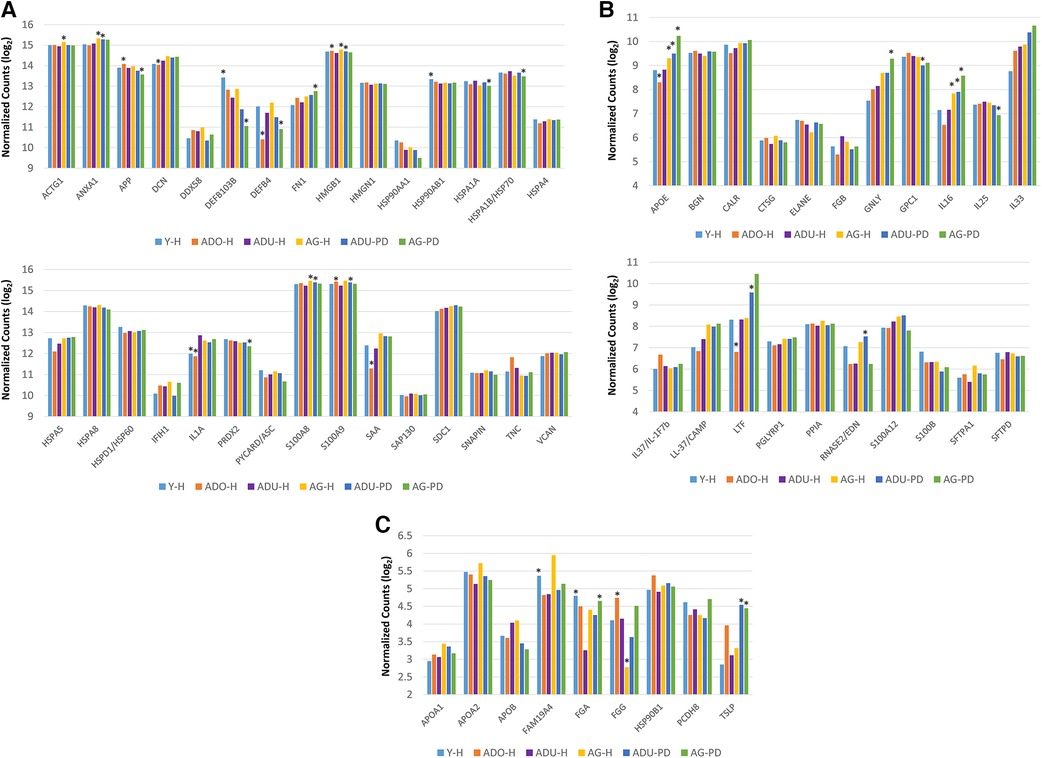
Figure 1. (A–C) normalized counts of DAMP/alarmin gene expression in gingival tissues from healthy animals of different ages (Y-H; ADO-H; ADU-H; AG-H) or with naturally-occurring periodontitis (ADU-PD; AG-PD). Asterisks (*) denote significantly different from ADU-H levels at p < 0.05.
Figure 2 provides a summary of the DAMP/alarmin gene expression patterns in healthy gingival tissues for a subsequent prospective longitudinal study of experimental periodontitis. Significant differences from adult healthy levels were most frequently observed in the young and adolescent animals (e.g., APP, PRDX2, TNC, BGN, HMGN1, FGA). Few representative in this gene array were altered in the healthy aged gingival tissues.
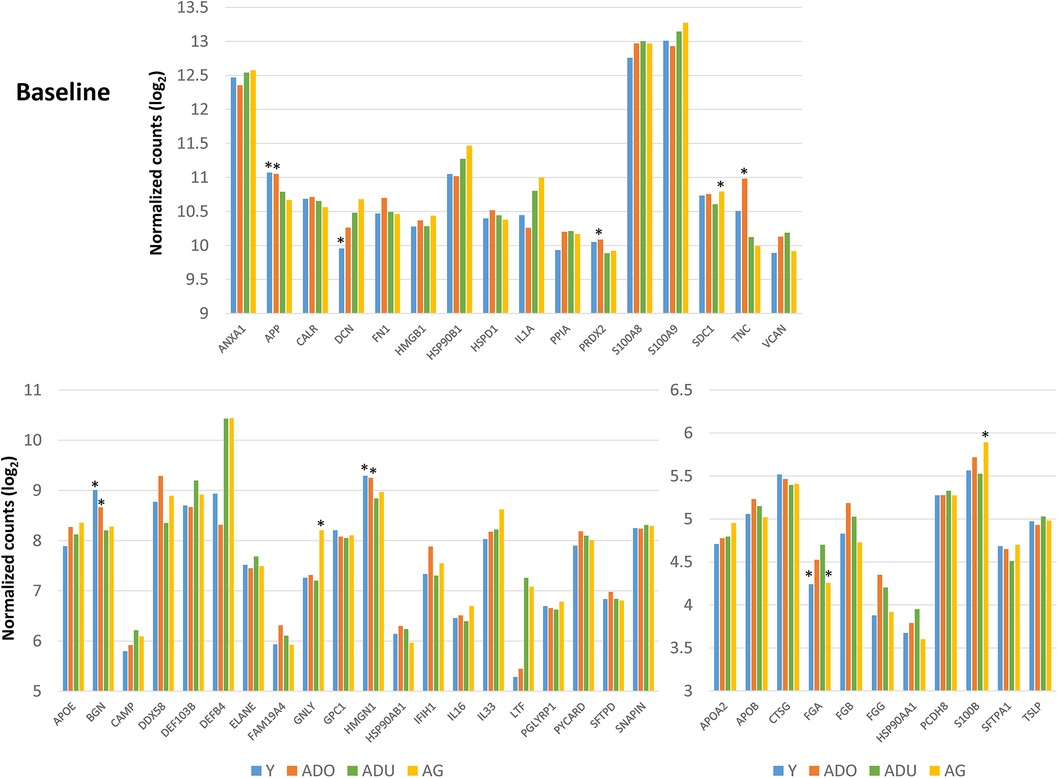
Figure 2. Normalized counts of DAMP/alarmin gene expression in healthy gingival tissues of different age groups at baseline of the experimental periodontitis model. Asterisks (*) denote significantly different from ADU-H levels at p < 0.05.
Figure 3 displays an overview of the changes in the gene expression during periodontitis and with resolution of the periodontal lesions. Of the 48 target genes, only 15 were altered by ≥1.5-fold in any of the age groups of animals. Generally, DEFB4, DEFB103B, DDX58 were decreased with disease, as was TNC in the young and adult animals. Increases in LTF, IL33, DCN, BGN, and FN1 were observed across the age groups, with increases observed during disease initiation (0.5 months) and maintaining these elevated levels throughout disease progression.
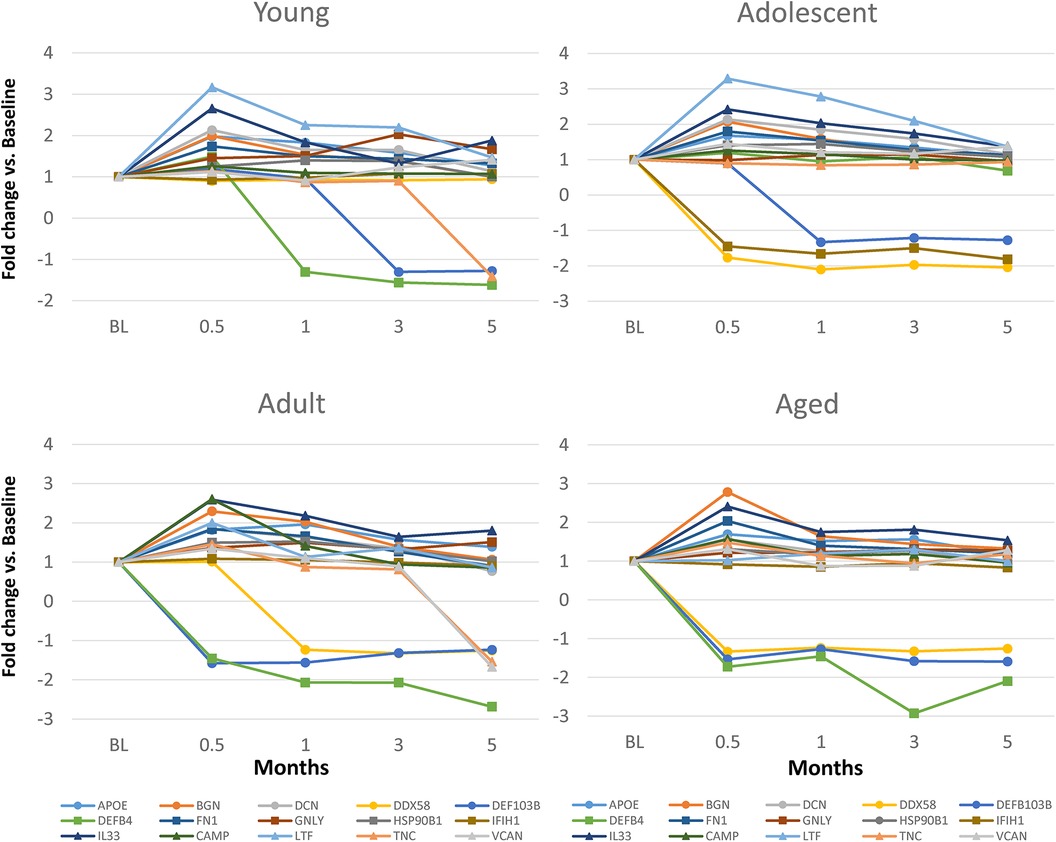
Figure 3. Fold changes from baseline (health) in selected DAMP/alarmin genes that showed a ≥1.5-fold difference at one or more time points in any of the age groups.
Relationship of DAMP/alarmin expression to tissue destructive gene expression patterns
Changes in DAMP/alarmin levels in the gingival tissues can represent a component of the tissue alteration/destruction that it occurring during the formation of periodontal lesions. As such, Figure 4 summarizes the findings from a targeted set of genes that would be related to these tissue changes in disease. Figure 4A identifies increases in MMP1, MMP3, MMP9, CTSK, RANKL, NFATc1, and c-FOS with naturally-occurring disease, accompanied by decreases in IL18, CSPG4, and STAT1. Similar alterations were observed in the longitudinal experimental periodontitis model across all age groups with elevated MMP1, MMP3, MMP9, and ALPL. STAT1 was similarly decreased, albeit the levels of c-FOS differed between these disease models.
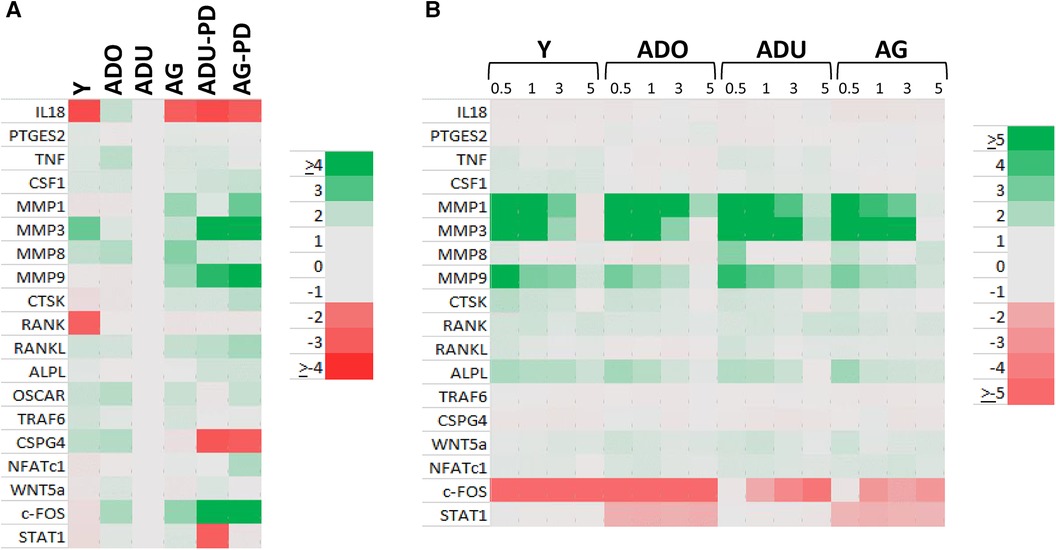
Figure 4. Heatmap of fold differences in normalized counts in (A) naturally-occurring disease model across age groups. Fold changes compared to ADU-H tissues. (B) Experimental periodontitis model across age groups and time points of disease (0.5, 1, 3) and resolution (5). Fold changes compared to baseline (healthy) tissues for each age group.
The data analysis then focused in identifying patterns of the DAMP/alarmins that were related to potential tissue destructive changes (Figure 5). Figure 5A displays significant correlations of the DAMP/alarmin gene expression with the tissue destructive gene patterns in health compared with naturally-occurring disease. The results showed similar frequencies of positive and negative correlations in the young/adolescent samples. In contrast, in the adult/aged healthy samples nearly 5 times as many DAMP/alarmin genes were positively correlated with the tissue destructive gene levels. A similar skewed distribution was noted in the disease samples with approximately 50% more DAMP/alarmin genes positively correlated. Of interest was the distribution of DAMP/alarmin correlations across the samples with DDX58, FN1, IFIH1, IL16, VCAN, and HSPA4 showing a predilection for these relationships.
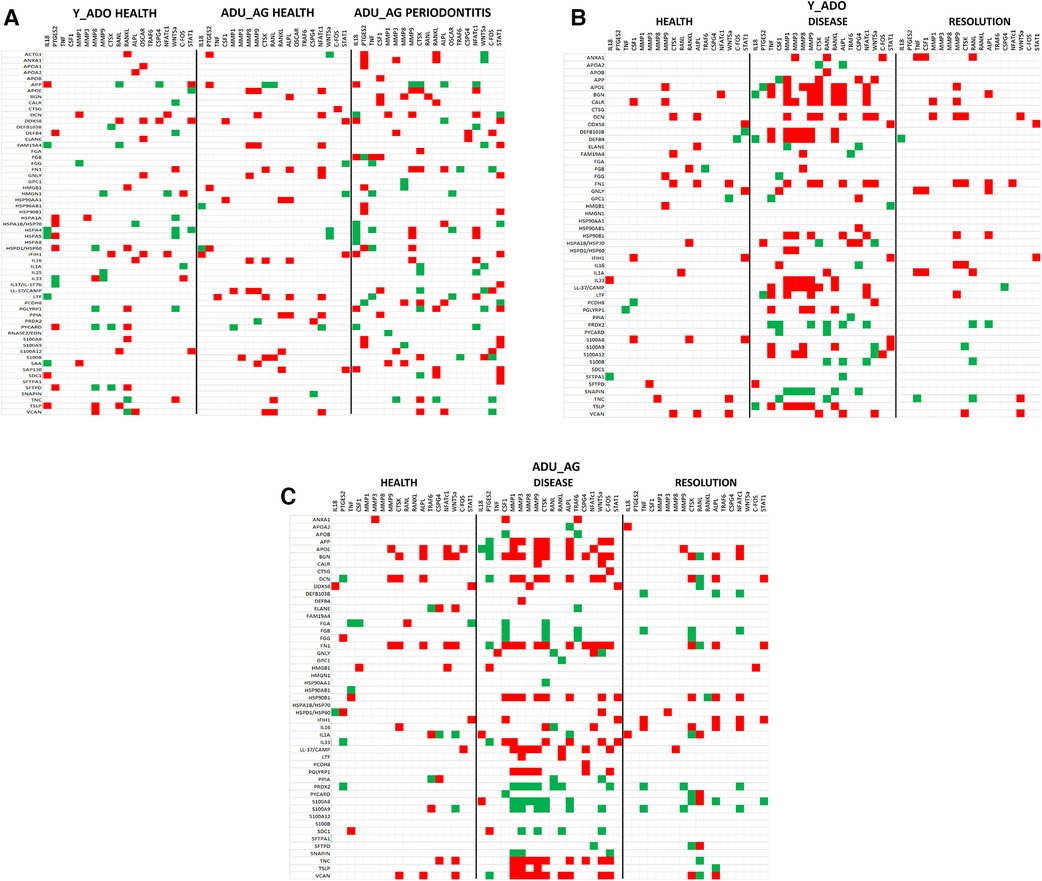
Figure 5. Summary of significant correlations between normalized counts of DAMP/alarmin genes and genes associated with inflammatory tissue destruction outcomes. Red denotes significant positive correlation and green denotes significant negative correlation at p < 0.01. (A) naturally-occurring disease model; (B) experimental periodontitis model in combined data from younger animals (young, adolescent); and (C) experimental periodontitis model in combined data from older animals (adult, aged).
Figure 5B shows a similar analysis for the longitudinal experimental periodontitis model. With the young and adolescent animals, significant positive correlations dominated the relationships in both healthy and resolution samples. DCN and FN1 tended to show a higher frequency of these correlations in the two types of samples. Disease in this younger group of animals demonstrated a broad array of significant correlations with the genes related to tissue destructive activities. In this analysis, distinct patterns of positive correlations were specifically noted with soft tissue destruction processes, e.g., MMP, CTSK, as well as with bone biology related genes (e.g., RANKL, ALPL). Also of interest was that the DAMP/alarmins related to the correlations were also somewhat distinctive with APOE, BGN, CALR, DCN, DEFB103B, DEFB4, FN1, IL33, CAMP and TSLP. More limited negative correlations were observed, with PRDX2 and SNAPIN skewed towards this comparison.
Figure 5C provides a summary of similar outcomes for the adult and aged groups of animals. As with the younger groups, in health the positive correlations predominated with APOE, BGN, and FN1 showing more frequent correlations. With resolution in the older groups, the distribution of positive and negative correlations were more similar with rather limited patterns for any particular DAMP/alarmin gene. However, with disease there was a dramatic increase in significant correlations, including both positive and negative correlations. Similar to the younger groups, APP, APOE, BGN, FN1, CAMP, PGLYRP1, TNC, TSLP, and VCAN were all significantly related to MMPs and ALPL related to bone biology. Additionally, there was a large array of positive correlations with the various transcription factors. In contrast, PRDX2, S100A8 and S100A9 were negatively correlated with the MMPs and bone biology genes.
Relationship of DAMP/alarmin expression to oral microbiome members
In Figure 6A, we evaluated the relationship between members of the oral microbiome in samples from sites in the experimental periodontitis model in health, progression periodontal lesions, and with resolution related to the expression of DAMP/alarmin genes. Forty-one OTUs represented the primary microbiome members in the young and adolescent samples. In healthy samples, the array of DAMP/alarmin genes showed significant correlations with the various OTUs particularly evident with ANXA1, FGA, HSP90AB1, HSPD1, IL1A, PPIA, PYCARD/ASC, S100B, and SDC1 in the healthy samples. The frequency of correlations decreased with disease, with only FN1 remaining highly correlated with various OTUs. Finally, in the resolution samples the microbial correlations of the DAMP/alarmin genes was limited with only DEFB103B, HSP90AB1, IL16, PYCARD/ASC, and S100A12 demonstrating an elevated frequency of correlations.
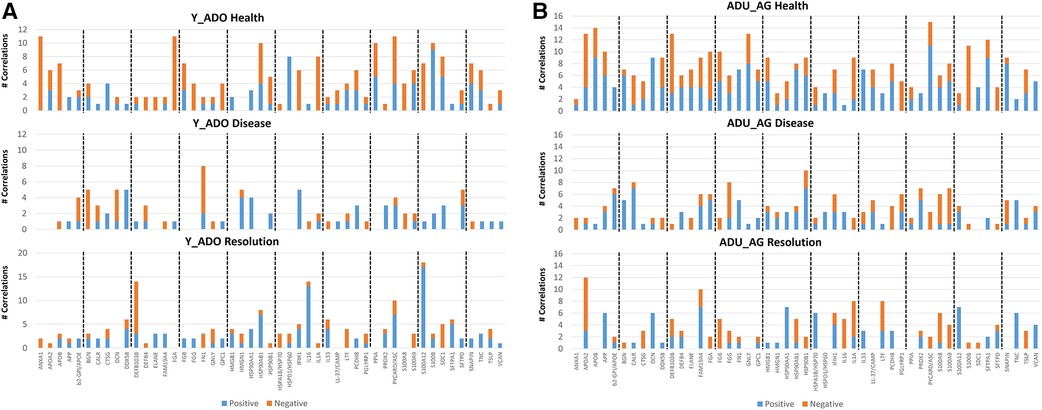
Figure 6. Comparison of the frequency of significant (p < 0.01) correlations between the normalized counts of DAMP/alarmin genes and the relative abundance of the overall taxa in the oral microbiome of (A) younger (young, adolescent) and (B) older (adult, aged) animals. Frequency of both significant positive and negative correlations are presented.
As in healthy samples from the younger animals, Figure 6B shows a greater frequency of correlations with the OTUs in healthy gingival tissues from the older groups of animals. These correlations were seen with APOA2, APOB, APP, DCN, DDX58, DEFB103B, FAM19A4, FGA, FGB, GNLY, HMGB1, HSP90AB1, HSP90B1, IL1A, PCDH8, PYCARD/ASC, S100B, SFTPA1, and SNAPIN. The frequency of correlations decreased in the disease samples with only CALR, FGG and HSP90B1 showing an elevated frequency. As in the young group, the resolution samples showed a low frequency of correlations, limited to APOA2, FAM19A4, IL1A, and LTF showing these correlations.
The data was then evaluated to determine which member of the oral microbiome were most closely related to alterations in the DAMP/alarmin gene expression profiles in health, disease and resolution specimens. Figure 7A displays the frequency of significant correlations between an individual OTU and the array of 51 DAMP/alarmin genes. In the younger groups, SR1 HMT345, Prevotella intermedia HMT643, Prevotella_unclassified, Fusobacterium sp. HMT203, Leptotrichia_unclassified, S. parasanguinis HMT411, Haemophilus sp. HMT035, Selenomonas_unclassified, T. denticola HMT584, and V. parvula HMT161 were all highly correlated with the range of DAMP/alarmin genes. Generally, in this group of animals, the correlations were in the healthy and resolution samples, except with Fretibacterium sp. HMT361 and V. parvula HMT161, whereby the prevalence of correlations were in disease samples.
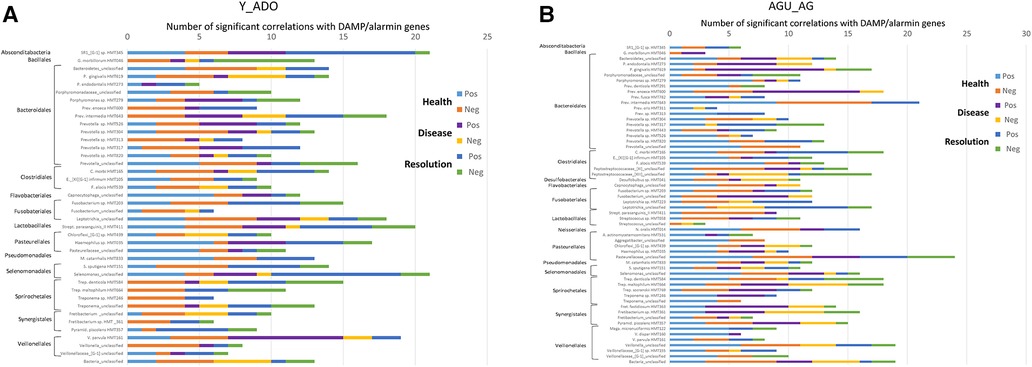
Figure 7. Frequency of significant (p < 0.01) correlations of the relative abundance of individual microbial taxa organized by order with the normalized counts of the array of DAMP/alarmin genes. Stacked bars summarize the number of positive and negative correlations in health, disease, and resolution samples from (A) younger (young, adolescent) and (B) older (adult, aged) animals.
Figure 7B summarizes similar results from the adult and aged specimens. In these samples, P. gingivalis HMT619, P. enoeca HMT600, P. intermedia HMT643, C. morbi HMT165, Peptostreptococcaceae [XI]/[XII]_unclassified, Leptotrichia_unclassified, N. oralis HMT014, Pasteurellaceae_unclassified, Selenmonas_unclassified, T. denticola HMT584, T. maltophilum HMT664, Fretibacterium sp. HMT361, P. piscolens HMT357, and Veillonella_unclassified all showed a predominant frequency of correlations. Of these, P. gingivalis, P. enoeca, Peptostreptococcaceae [XII]_unclassified, T. maltophilum, Fretibacterium, and P. piscolens correlations were skewed towards disease samples.
Discussion
At sites of local tissue destruction resulting from chronic inflammatory lesions, various triggers occur that presage the inflammatory responses. In periodontitis, the triggers include microbial stimulants derived from the complex oral bacterial biofilms transitioning from a symbiotic and homeostatic microbiome to a disease associated dystiotic community (45, 95). Additional stimulants are derived from disruption of host cell integrity and functions releasing an array of damage-associated molecular pattern biomolecules (DAMPs) that are considered alarmins for triggering protective/reparative host responses (65, 66, 68, 96–98). We have reported extensively on the methods for the use of this human-like disease model of naturally-occurring and experimental ligature-induced periodontitis (72, 76, 82, 87, 92). The model provided a large quantity of transcriptomic data from mucosal gingival tissues in health and disease. Querying the transcriptome provides the opportunity to target cellular biologic pathways that comprise the complex milieu of the periodontium and in this case, exploring features of these DAMPs and alarmin host molecules in the gingival tissues.
The study also evaluated the effect of age of the animals on the attributes of this host response system in health and disease. Across the array of DAMPs/alarmins, there were a limited number showing age effects and differences from healthy adult tissue levels. These were most frequently affected in the younger animals or in the aged and diseased samples. Also, in the longitudinal experimental periodontitis study, the distinctive DAMP/alarmin genes were commonly increased or decreased with disease initiation across the age groups. The characteristics of the decreased gene expression were targeted toward antimicrobial peptide production (DEPB4, DEFB103B), related to innate immune recognition processes especially viral related (DDX58/RIGI), and an extracellular matrix protein (TNC) important in guidance of cell migration. Those genes that were substantially increased following the initiation of disease featured antimicrobial molecules (LTF, CAMP), a chemoattractant for T cell maturation (IL33), and contributing to innate and adaptive immune responses in regulating immune suppressive activities (APOE). Additionally, the elevated gene expression profiles included genes related to fundamental cell functions that could trigger an alarmin response including DCN as a stimulator of autophagy and inflammation, BGN related to collagen fiber assembly and inflammation and innate immune responses, and FN1 focused on normal cell adhesion and migration capabilities.
The study was also designed to examine the expression of these DAMPs/alarmins as the linkage between bacterial triggers of inflammation and potential downstream host modulators of tissue integrity. Thus, we identified specific DAMP/alarmin genes that significantly correlated with an array of the bacteria detected in the microbiome samples from the juxtaposed healthy, disease, or resolution sites. The vice versa analysis also identified a set of the oral bacteria that appeared to be most related to altered expression of these DAMP/alarmin genes. A notable similarity occurred between samples from younger vs. older animals, with respect to the most frequent correlations were with the genes in healthy samples from the groups. A decreased frequency was observed across the samples during disease, and the lowest correlation prevalence was detected in the resolution samples. Here the differences showed in the younger animals that the correlations were predominantly in the healthy and resolution samples, while in the older animals the correlations were skewed towards disease samples.
The literature is replete with a description of innate immune system components specifically designed and expressed to detect that vast array of bacterial, viral, fungal, and other noxious stimuli by host cells (99–108). These detectors include both cell surface and intracellular components that evolved to combat specific components on extracellular and intracellular pathogens. In addition, many of these are directly involved in more physiologic functions to help maintain homeostasis with the autochthonous microbiota colonizing surfaces throughout the body. However, recognized as part of inflammation and innate immunity is the production/release of biomolecules that are specific warning signals to the host immune apparatus that cells in a particular location are undergoing stress and or mortal outcomes. These DAMPs or alarmins comprise a wide array of cell surface and intracellular components that reflect disruption of the integrity of cells. The host has developed a variety of detectors for these molecules and evolved to respond to reestablish homeostasis and stimulate resolution and healing (67, 96, 98, 100, 109, 110–112). Also clear is that these biomolecules are elevated in chronic inflammatory diseases, including periodontitis (##). These findings are generally derived from cross-sectional observational human studies, often comparing levels in tissues or fluids from healthy sites compared to established periodontal lesions or in vitro oral cell culture models.
Necroptosis is a method of programmed cell death that causes the release of DAMPs into the extracellular environment and thus transmit danger signals for inflammatory responses. This process can be induced by various periodontal pathogens and may play a role in this chronic inflammatory disease (113). Molecules contained in necrotic cell supernatants have been shown to stimulate inflammatory responses in both gingival epithelial cells and fibroblasts by activation of TLR3 (105). The presence and molecular mechanisms of release and detection of PAMPs and DAMPs has also been proposed to underpin the relationship of periodontitis and atherosclerosis, as well as presenting as potential therapeutic targets (65). Additional studies have presented the role of TLR and DAMP detection from damaged cells related to periodontal disease (114).
Information on the features of inflammasome activation that can result in pyroptosis and release of DAMPs in periodontitis is rather limited. However, this model of disease and engagement of the breadth of the host innate immune and inflammatory responses is likely important in this disease (115). More directly, DAMPs have been described in the oral cavity in periodontal disease, oral candidiasis, and Sjogren's syndrome. Not only do they likely intersect with exogenous signaling via pathogen-associated molecular patterns (PAMPs) but may aid in resolution of the oral fungal infection (66).
Various DAMPs/alarmins were found to be altered in the nonhuman primate tissues. IL-33, a member of the IL-1 family of cytokines, is a nuclear protein that is released from damaged cells as an alarmin (67). The cytokine was also elevated in GCF and plasma in generalized aggressive periodontitis patients compared to periodontally healthy or chronic periodontitis (116). Both IL-33 and IL-1α as alarmins were elevated in GCF and/or plasma in generalized aggressive periodontitis and in chronic periodontitis compared to healthy subjects (117). At the cellular level, IL-33 is overexpressed in gingival epithelial cells in chronic periodontitis and in a murine experimental periodontitis model with elevated expression of RANKL (118). Gingival fibroblasts have also been shown to release IL-33 induced by environmental TNFα levels (119).
HMGB1 is another major inflammatory mediator alarmin secreted by inflammatory cells. It interacts with TLRs to induce inflammatory cytokines. Paknejad et al. (111) showed that HMGB1 is released during inflammatory processes in periodontitis and likely promotes continued inflammation. Moreover, HMGB1 and the complement system provide important components of immune surveillance. Interplay between these pathways plays a role in chronic inflammatory diseases (120). This alarmin can be released by periodontal ligament cells and can modify macrophage differentiation and migration (121). There interplay between microbes and alarmins was suggested by a study showing that F. nucleatum was activates the NLRP3 inflammasome in gingival epithelial cells and causes the release of alarmins HMGB1 and ASC (PYCARD) from these cells (122).
Selected calcium-binding alarmins were also identified in this nonhuman primate disease model. S100A12, in the S100 subfamily of myeloid-related proteins acts as an alarmin to induce a pro-inflammatory innate immune response. It has been described to be elevated in various chronic inflammatory diseases. The molecule is produced by classical monocytes with cells from periodontitis patients producing higher levels compared to control subjects (123). More severe disease appeared to relate to the level of increase. S100A12 was also found to be increased in GCF and serum of CP patients and even more elevated in T2DM patients with periodontitis (124). S100A8, S100A9, S100A12, were all elevated in saliva from periodontitis and gingivitis patients compared to healthy controls (125) and salivary levels of S100A8, S100A9 and S100A12 were significantly related to clinical and radiographic signs of periodontitis (47). The S100A12 gene was a significant DEG in gingival tissues of periodontitis (126). Finally, GWAS studies of periodontitis found that S100A12 polymorphisms appear to influence periodontitis (127).
Finally, of the broad array of DAMP/alarmin molecules, SAA has been shown to be involved in the development of chronic inflammatory diseases. SAA is elevated in serum of periodontitis patients (128) and increased in inflamed gingival tissues where it triggers inflammatory cytokines via a TLR2 pathway (129). Obese patients with periodontitis were shown to have elevated levels of SAA in GCF (130). Additionally, in patients with coronary artery disease, inflamed gingival levels are correlated with systemic levels of SAA (Temelli 2018). Finally, Hirai et al. (110) showed SAA levels were positively associated with human periodontal inflammatory lesions. Mice lacking SAA also showed a decreased periodontal inflammatory infiltrate. The SAA levels appeared to be linked to TLR2/TLR4 functions in stimulating cellular responses.
Thus, differences in DAMP/alarmin levels have been linked to the clinical features of existing periodontal disease, albeit negligible data are available regarding the kinetics of the response related to disease process or the actual status of the particular affected site(s). Furthermore, minimal information is available regarding the biologic relationship between host factors involved in the tissue destructive events and the patterns of the DAMPs/alarmins in human lesions. Finally, while the alterations in more pathogenic oral microbiomes have been described, limited information is available concerning the potential linkage between changes in specific bacteria and the production of these biomarkers of cellular distress. Using this human-like preclinical model of induced periodontitis, we demonstrated the dynamics of the activation of the DAMP/alarmin warning system in the gingival tissues. The data indicated many similarities in the magnitude of these biomolecules across the lifespan, but did show some specific differences in young vs. older animals. The experimental design documented significant relationships between the elevated expression of DAMP/alarmin genes in the gingival tissues during the disease process and impacted by age. Further studies will be required to better understand how these relate to the biological triggers of the clinical disease features, as well as how they might be used as early harbingers of transition to disease, and/or therapeutic targets.
Data availability statement
The original contributions presented in the study are publicly available.The microbiological data can be found here: https://www.ncbi.nlm.nih.gov/, accession number PRJNA516659. The gene expression data have been uploaded into GEO accession GSE180588 (https://www.ncbi.nlm.nih.gov/gds).
Ethics statement
The animal study was approved by IACUC, University of Puerto Rico. The study was conducted in accordance with the local legislation and institutional requirements.
Author contributions
OG: Conceptualization, Data curation, Funding acquisition, Investigation, Methodology, Supervision, Writing – review & editing. SK: Data curation, Methodology, Writing – review & editing, Formal analysis. JE: Data curation, Formal analysis, Methodology, Writing – review & editing, Conceptualization, Funding acquisition, Investigation, Project administration, Resources, Supervision, Writing – original draft.
Funding
The author(s) declare financial support was received for the research, authorship, and/or publication of this article.
This work was supported by National Institute of Health grant P20GM103538. We express our gratitude to the Caribbean Primate Research Center (CPRC) supported by grant P40RR03640, and the Center for Oral Health Research in the College of Dentistry at the University of Kentucky.
Acknowledgments
We thank M. J. Novak (University of Kentucky) and L. Orraca (University of Puerto Rico) for their support in the clinical aspects of the protocol. We thank J. Gonzalez Martinez and A. G. Burgos Rodriguez from the Caribbean Primate Research Center for animal husbandry and sampling support. We also thank the Microarray Core Laboratory of University Kentucky for their invaluable technical and data management assistance and A. Stromberg for initial normalization of the data.
Conflict of interest
The authors declare that the research was conducted in the absence of any commercial or financial relationships that could be construed as a potential conflict of interest.
The author(s) declared that they were an editorial board member of Frontiers, at the time of submission. This had no impact on the peer review process and the final decision.
Publisher's note
All claims expressed in this article are solely those of the authors and do not necessarily represent those of their affiliated organizations, or those of the publisher, the editors and the reviewers. Any product that may be evaluated in this article, or claim that may be made by its manufacturer, is not guaranteed or endorsed by the publisher.
References
1. Zenobia C, Hajishengallis G. Porphyromonas gingivalis virulence factors involved in subversion of leukocytes and microbial dysbiosis. Virulence. (2015) 6:236–43. doi: 10.1080/21505594.2014.999567
2. Jusko M, Potempa J, Mizgalska D, Bielecka E, Ksiazek M, Riesbeck K, et al. A metalloproteinase mirolysin of Tannerella forsythia inhibits all pathways of the complement system. J Immunol. (2015) 195:2231–40. doi: 10.4049/jimmunol.1402892
3. Sano Y, Okamoto-Shibayama K, Tanaka K, Ito R, Shintani S, Yakushiji M, et al. Dentilisin involvement in coaggregation between Treponema denticola and Tannerella forsythia. Anaerobe. (2014) 30:45–50. doi: 10.1016/j.anaerobe.2014.08.008
4. Chu L, Dong Z, Xu X, Cochran DL, Ebersole JL. Role of glutathione metabolism of Treponema denticola in bacterial growth and virulence expression. Infect Immun. (2002) 70:1113–20. doi: 10.1128/IAI.70.3.1113-1120.2002
5. Chioma O, Aruni AW, Milford TA, Fletcher HM. Filifactor alocis collagenase can modulate apoptosis of normal oral keratinocytes. Mol Oral Microbiol. (2017) 32:166–77. doi: 10.1111/omi.12163
6. Doron L, Coppenhagen-Glazer S, Ibrahim Y, Eini A, Naor R, Rosen G, et al. Identification and characterization of fusolisin, the Fusobacterium nucleatum autotransporter serine protease. PLoS One. (2014) 9:e111329. doi: 10.1371/journal.pone.0111329
7. Jie Bao G, Kari K, Tervahartiala T, Sorsa T, Meurman JH. Proteolytic activities of oral bacteria on ProMMP-9 and the effect of synthetic proteinase inhibitors. Open Dent J. (2008) 2:96–102. doi: 10.2174/1874210600802010096
8. Kachlany SC. Aggregatibacter actinomycetemcomitans leukotoxin: from threat to therapy. J Dent Res. (2010) 89:561–70. doi: 10.1177/0022034510363682
9. Belibasakis GN, Bostanci N. Inflammatory and bone remodeling responses to the cytolethal distending toxins. Cells. (2014) 3:236–46. doi: 10.3390/cells3020236
10. LaGier MJ, Threadgill DS. Identification of novel genes in the oral pathogen Campylobacter rectus. Oral Microbiol Immunol. (2008) 23:406–12. doi: 10.1111/j.1399-302X.2008.00443.x
11. Henderson B, Ward JM, Ready D. Aggregatibacter (Actinobacillus) actinomycetemcomitans: a triple A* periodontopathogen? Periodontol 2000. (2010) 54:78–105. doi: 10.1111/j.1600-0757.2009.00331.x
12. Yousefi L, Leylabadlo HE, Pourlak T, Eslami H, Taghizadeh S, Ganbarov K, et al. Oral spirochetes: pathogenic mechanisms in periodontal disease. Microb Pathog. (2020) 144:104193. doi: 10.1016/j.micpath.2020.104193
13. Li YY, Cai Q, Li BS, Qiao SW, Jiang JY, Wang D, et al. The effect of Porphyromonas gingivalis lipopolysaccharide on the pyroptosis of gingival fibroblasts. Inflammation. (2021) 44:846–58. doi: 10.1007/s10753-020-01379-7
14. Kantrong N, To TT, Darveau RP. Gingival epithelial cell recognition of lipopolysaccharide. Adv Exp Med Biol. (2019) 1197:55–67. doi: 10.1007/978-3-030-28524-1_5
15. Liu J, Zeng J, Wang X, Zheng M, Luan Q. P53 mediates lipopolysaccharide-induced inflammation in human gingival fibroblasts. J Periodontol. (2018) 89:1142–51. doi: 10.1002/JPER.18-0026
16. Liu J, Wang X, Zheng M, Luan Q. Lipopolysaccharide from Porphyromonas gingivalis promotes autophagy of human gingival fibroblasts through the PI3K/Akt/mTOR signaling pathway. Life Sci. (2018) 211:133–9. doi: 10.1016/j.lfs.2018.09.023
17. Zhang Y, Li X. Lipopolysaccharide-regulated production of bone sialoprotein and interleukin-8 in human periodontal ligament fibroblasts: the role of toll-like receptors 2 and 4 and the MAPK pathway. J Periodontal Res. (2015) 50:141–51. doi: 10.1111/jre.12193
18. Jian C, Li C, Ren Y, He Y, Li Y, Feng X, et al. Hypoxia augments lipopolysaccharide-induced cytokine expression in periodontal ligament cells. Inflammation. (2014) 37:1413–23. doi: 10.1007/s10753-014-9865-6
19. Sun Y, Li H, Yang MF, Shu W, Sun MJ, Xu Y. Effects of aging on endotoxin tolerance induced by lipopolysaccharides derived from Porphyromonas gingivalis and Escherichia coli. PLoS One. (2012) 7:e39224. doi: 10.1371/journal.pone.0039224
20. Uemura Y, Hiroshima Y, Tada A, Murakami K, Yoshida K, Inagaki Y, et al. Porphyromonas gingivalis outer membrane vesicles stimulate gingival epithelial cells to induce pro-inflammatory cytokines via the MAPK and STING pathways. Biomedicines. (2022) 10:2643. doi: 10.3390/biomedicines10102643
21. Gonzalez OA, Kirakodu S, Nguyen LM, Orraca L, Novak MJ, Gonzalez-Martinez J, et al. Comparative analysis of gene expression patterns for oral epithelial cell functions in periodontitis. Front Oral Health. (2022) 3:863231. doi: 10.3389/froh.2022.863231
22. Zhang S, Yu N, Arce RM. Periodontal inflammation: integrating genes and dysbiosis. Periodontol 2000. (2020) 82:129–42. doi: 10.1111/prd.12267
23. Bhuyan R, Bhuyan SK, Mohanty JN, Das S, Juliana N, Juliana IF. Periodontitis and its inflammatory changes linked to various systemic diseases: a review of its underlying mechanisms. Biomedicines. (2022) 10:2659. doi: 10.3390/biomedicines10102659
24. Williams DW, Greenwell-Wild T, Brenchley L, Dutzan N, Overmiller A, Sawaya AP, et al. Human oral mucosa cell atlas reveals a stromal-neutrophil axis regulating tissue immunity. Cell. (2021) 184:4090–4104.e15. doi: 10.1016/j.cell.2021.05.013
25. Lamont RJ, Koo H, Hajishengallis G. The oral microbiota: dynamic communities and host interactions. Nat Rev Microbiol. (2018) 16:745–59. doi: 10.1038/s41579-018-0089-x
26. Hajishengallis G. The inflammophilic character of the periodontitis-associated microbiota. Mol Oral Microbiol. (2014) 29:248–57. doi: 10.1111/omi.12065
27. Mukherjee S. The temperature of the periodontal pockets. J Clin Periodontol. (1981) 8:17–20. doi: 10.1111/j.1600-051X.1981.tb02020.x
28. Meyerov RH, Lemmer J, Cleaton-Jones PE, Volchansky A. Temperature gradients in periodontal pockets. J Periodontol. (1991) 62:95–9. doi: 10.1902/jop.1991.62.2.95
29. Ko TJ, Byrd KM, Kim SA. The chairside periodontal diagnostic toolkit: past, present, and future. Diagnostics (Basel). (2021) 11:932. doi: 10.3390/diagnostics11060932
30. Goodson JM. Diagnosis of periodontitis by physical measurement: interpretation from episodic disease hypothesis. J Periodontol. (1992) 63(Suppl 4S):373–82. doi: 10.1902/jop.1992.63.4s.373
31. Watanabe T, Soeda W, Kobayashi K, Nagao M. The pH value changes in the periodontal pockets. Bull Tokyo Med Dent Univ. (1996) 43:67–73.9031618
32. Galgut PN. The relevance of pH to gingivitis and periodontitis. J Int Acad Periodontol. (2001) 3:61–7.12666943
33. Eggert FM, Drewell L, Bigelow JA, Speck JE, Goldner M. The pH of gingival crevices and periodontal pockets in children, teenagers and adults. Arch Oral Biol. (1991) 36:233–8. doi: 10.1016/0003-9969(91)90091-8
34. Bang J, Cimasoni G, Rosenbusch C, Duckert A. Sodium, potassium and calcium contents of crevicular exudate: their relations to gingivitis and periodontitis. J Periodontol. (1973) 44:770–4. doi: 10.1902/jop.1973.44.12.770
35. Koregol AC, More SP, Nainegali S, Kalburgi N, Verma S. Analysis of inorganic ions in gingival crevicular fluid as indicators of periodontal disease activity: a clinico-biochemical study. Contemp Clin Dent. (2011) 2:278–82. doi: 10.4103/0976-237X.91788
36. Chapple IL, Matthews JB. The role of reactive oxygen and antioxidant species in periodontal tissue destruction. Periodontol 2000. (2007) 43:160–232. doi: 10.1111/j.1600-0757.2006.00178.x
37. Yin Q, Liu C, Bao H, Li S, Huang Z, Gu D, et al. Estimation of gingival crevicular fluid oxidative stress markers in school-aged children and teenagers with insufficient sleep. BMC oral Health. (2022) 22:616. doi: 10.1186/s12903-022-02642-z
38. Pradeep AR, Ramchandraprasad MV, Bajaj P, Rao NS, Agarwal E. Protein carbonyl: an oxidative stress marker in gingival crevicular fluid in healthy, gingivitis, and chronic periodontitis subjects. Contemp Clin Dent. (2013) 4:27–31. doi: 10.4103/0976-237X.111589
39. Manjeu J, Babu S, Kala CN, Paul GT, Soorya KV, Gandhimadhi D. Evaluation of the reactive oxygen metabolite levels in plasma, gingival crevicular fluid, and saliva in generalized chronic periodontitis patients before and after nonsurgical periodontal therapy: a case-control and interventional study. J Indian Soc Periodontol. (2022) 26:37–43. doi: 10.4103/jisp.jisp_519_20
40. Kapila YL. Oral health’s inextricable connection to systemic health: special populations bring to bear multimodal relationships and factors connecting periodontal disease to systemic diseases and conditions. Periodontol 2000. (2021) 87:11–6. doi: 10.1111/prd.12398
41. Ebersole JL, Dawson DA 3rd, Huja P Emecen, Pandruvada S, Basu A, Nguyen L, et al. Age and periodontal health—immunological view. Curr Oral Health Rep. (2018) 5:229–41. doi: 10.1007/s40496-018-0202-2
42. Papapanou PN, Susin C. Periodontitis epidemiology: is periodontitis under-recognized, over-diagnosed, or both? Periodontol 2000. (2017) 75:45–51. doi: 10.1111/prd.12200
43. Lamster IB, Asadourian L, Del Carmen T, Friedman PK. The aging mouth: differentiating normal aging from disease. Periodontol 2000. (2016) 72:96–107. doi: 10.1111/prd.12131
44. Lamster IB. Geriatric periodontology: how the need to care for the aging population can influence the future of the dental profession. Periodontol 2000. (2016) 72:7–12. doi: 10.1111/prd.12157
45. Curtis MA, Diaz PI, Van Dyke TE. The role of the microbiota in periodontal disease. Periodontol 2000. (2020) 83:14–25. doi: 10.1111/prd.12296
46. Sudhakara P, Gupta A, Bhardwaj A, Wilson A. Oral dysbiotic communities and their implications in systemic diseases. Dent J (Basel). (2018) 6:10. doi: 10.3390/dj6020010
47. Salminen A, Pietiainen M, Paju S, Sorsa T, Mantyla P, Buhlin K, et al. Common complement factor H polymorphisms are linked with periodontitis in elderly patients. J Periodontol. (2022) 93:1626–34. doi: 10.1002/JPER.22-0005
48. Liukkonen A, He Q, Gursoy UK, Pussinen PJ, Grondahl-Yli-Hannuksela K, Liukkonen J, et al. Mannose-binding lectin gene polymorphism in relation to periodontal infection. J Periodontal Res. (2016) 52:540–5. doi: 10.1111/jre.12420
49. Rhodin K, Divaris K, North KE, Barros SP, Moss K, Beck JD, et al. Chronic periodontitis genome-wide association studies: gene-centric and gene set enrichment analyses. J Dent Res. (2014) 93:882–90. doi: 10.1177/0022034514544506
50. Correa JD, Madeira MF, Resende RG, Correia-Silva Jde F, Gomez RS, de Souza Dda G, et al. Association between polymorphisms in interleukin-17A and -17F genes and chronic periodontal disease. Mediators Inflamm. (2012) 2012:846052. doi: 10.1155/2012/846052
51. Loo WT, Wang M, Jin LJ, Cheung MN, Li GR. Association of matrix metalloproteinase (MMP-1, MMP-3 and MMP-9) and cyclooxygenase-2 gene polymorphisms and their proteins with chronic periodontitis. Arch Oral Biol. (2011) 56:1081–90. doi: 10.1016/j.archoralbio.2011.03.011
52. Loos BG, Van Dyke TE. The role of inflammation and genetics in periodontal disease. Periodontol 2000. (2020) 83:26–39. doi: 10.1111/prd.12297
53. Ren B, Lu J, Li M, Zou X, Liu Y, Wang C, et al. Anti-inflammatory effect of IL-1ra-loaded dextran/PLGA microspheres on Porphyromonas gingivalis lipopolysaccharide-stimulated macrophages in vitro and in vivo in a rat model of periodontitis. Biomed Pharmacother. (2021) 134:111171. doi: 10.1016/j.biopha.2020.111171
54. Miranda TS, Heluy SL, Cruz DF, da Silva HDP, Feres M, Figueiredo LC, et al. The ratios of pro-inflammatory to anti-inflammatory cytokines in the serum of chronic periodontitis patients with and without type 2 diabetes and/or smoking habit. Clin Oral Investig. (2018) 23:640–51. doi: 10.1007/s00784-018-2471-5
55. Striz I, Brabcova E, Kolesar L, Sekerkova A. Cytokine networking of innate immunity cells: a potential target of therapy. Clin Sci. (2014) 126:593–612. doi: 10.1042/CS20130497
56. MacManus CF, Campbell EL, Keely S, Burgess A, Kominsky DJ, Colgan SP. Anti-inflammatory actions of adrenomedullin through fine tuning of HIF stabilization. FASEB J. (2011) 25:1856–64. doi: 10.1096/fj.10-170316
57. Garlet GP. Destructive and protective roles of cytokines in periodontitis: a re-appraisal from host defense and tissue destruction viewpoints. J Dent Res. (2010) 89:1349–63. doi: 10.1177/0022034510376402
58. Ramadan DE, Hariyani N, Indrawati R, Ridwan RD, Diyatri I. Cytokines and chemokines in periodontitis. Eur J Dent. (2020) 14:483–95. doi: 10.1055/s-0040-1712718
59. Zhang Z, Zheng Y, Li X. Interleukin-10 gene polymorphisms and chronic periodontitis susceptibility: evidence based on 33 studies. J Periodontal Res. (2019) 54:95–105. doi: 10.1111/jre.12612
60. Gao L, Faibish D, Fredman G, Herrera BS, Chiang N, Serhan CN, et al. Resolvin E1 and chemokine-like receptor 1 mediate bone preservation. J Immunol. (2013) 190:689–94. doi: 10.4049/jimmunol.1103688
61. Freire MO, Van Dyke TE. Natural resolution of inflammation. Periodontol 2000. (2013) 63:149–64. doi: 10.1111/prd.12034
62. Eltay EG, Van Dyke T. Resolution of inflammation in oral diseases. Pharmacol Ther. (2023) 247:108453. doi: 10.1016/j.pharmthera.2023.108453
63. Abdalla HB, Alvarez C, Wu YC, Rojas P, Hammock BD, Maddipati KR, et al. Soluble epoxide hydrolase inhibition enhances production of specialized pro-resolving lipid mediator and promotes macrophage plasticity. Br J Pharmacol. (2023) 180:1597–615. doi: 10.1111/bph.16009
64. Hasturk H, Kantarci A, Ohira T, Arita M, Ebrahimi N, Chiang N, et al. Rve1 protects from local inflammation and osteoclast- mediated bone destruction in periodontitis. Faseb J. (2006) 20:401–3. doi: 10.1096/fj.05-4724fje
65. Zhu X, Huang H, Zhao L. PAMPs and DAMPs as the bridge between periodontitis and atherosclerosis: the potential therapeutic targets. Front Cell Dev Biol. (2022) 10:856118. doi: 10.3389/fcell.2022.856118
66. Kay JG, Kramer JM, Visser MB. Danger signals in oral cavity-related diseases. J Leukocyte Biol. (2019) 106:193–200. doi: 10.1002/JLB.4MIR1118-439R
67. Papathanasiou E, Conti P, Carinci F, Lauritano D, Theoharides TC. IL-1 superfamily members and periodontal diseases. J Dent Res. (2020) 99:1425–34. doi: 10.1177/0022034520945209
68. Yang D, Han Z, Oppenheim JJ. Alarmins and immunity. Immunol Rev. (2017) 280:41–56. doi: 10.1111/imr.12577
69. Ebersole JL, Nagarajan R, Kirakodu S, Gonzalez OA. Transcriptomic phases of periodontitis lesions using the nonhuman primate model. Sci Rep. (2021) 11:9282. doi: 10.1038/s41598-021-88803-6
70. Kirakodu S, Chen J, Gonzalez Martinez J, Gonzalez OA, Ebersole J. Microbiome profiles of ligature-induced periodontitis in nonhuman primates across the life span. Infect Immun. (2019) 87:e00067–19. doi: 10.1128/IAI.00067-19
71. Ebersole JL, Orraca L, Kensler TB, Gonzalez-Martinez J, Maldonado E, Gonzalez OA. Periodontal disease susceptible matrilines in the cayo Santiago Macaca mulatta macaques. J Periodontal Res. (2019) 54:134–42. doi: 10.1111/jre.12610
72. Ebersole JL, Kirakodu S, Novak MJ, Stromberg AJ, Shen S, Orraca L, et al. Cytokine gene expression profiles during initiation, progression and resolution of periodontitis. J Clin Periodontol. (2014) 41:853–61. doi: 10.1111/jcpe.12286
73. Ebersole JL, Steffen MJ, Gonzalez-Martinez J, Novak MJ. Effects of age and oral disease on systemic inflammatory and immune parameters in nonhuman primates. Clin Vaccine Immunol. (2008) 15:1067–75. doi: 10.1128/CVI.00258-07
74. Gonzalez OA, Kirakodu S, Nguyen L, Ebersole JL. Macrophage-related gingival transcriptomic patterns and microbiome alterations in experimental periodontitis in nonhuman primates. J Periodontal Res. (2023) 58:1148–70. doi: 10.1111/jre.13156
75. Gonzalez OA, Novak MJ, Kirakodu S, Stromberg A, Nagarajan R, Huang CB, et al. Differential gene expression profiles reflecting macrophage polarization in aging and periodontitis gingival tissues. Immunol Investig. (2015) 44:643–64. doi: 10.3109/08820139.2015.1070269
76. Gonzalez OA, Stromberg AJ, Huggins PM, Gonzalez-Martinez J, Novak MJ, Ebersole JL. Apoptotic genes are differentially expressed in aged gingival tissue. J Dent Res. (2011) 90:880–6. doi: 10.1177/0022034511403744
77. Ebersole JL, Kirakodu S, Novak MJ, Stromberg AJ, Shen S, Orraca L, et al. Cytokine gene expression profiles during initiation, progression and resolution of periodontitis. J Clin Periodontol. (2014) 41:853–61. doi: 10.1111/jcpe.12286
78. Ebersole JL, Orraca L, Novak MJ, Kirakodu S, Gonzalez-Martinez J, Gonzalez OA. Comparative analysis of gene expression patterns for oral epithelium-related functions with aging. Adv Exp Med Biol. (2019) 1197:143–63. doi: 10.1007/978-3-030-28524-1_11
79. Gonzalez OA, Kirakodu SS, Nguyen LM, Ebersole JL. Gingival transcriptomic patterns of macrophage polarization during initiation, progression, and resolution of periodontitis. Clin Exp Immunol. (2023) 211:248–68. doi: 10.1093/cei/uxac122
80. Ebersole JL, Kirakodu SS, Gonzalez OA. Oral microbiome interactions with gingival gene expression patterns for apoptosis, autophagy and hypoxia pathways in progressing periodontitis. Immunology. (2021) 162:405–17. doi: 10.1111/imm.13292
81. Meka A, Bakthavatchalu V, Sathishkumar S, Lopez MC, Verma RK, Wallet SM, et al. Porphyromonas gingivalis infection-induced tissue and bone transcriptional profiles. Mol Oral Microbiol. (2010) 25:61–74. doi: 10.1111/j.2041-1014.2009.00555.x
82. Gonzalez OA, Nagarajan R, Novak MJ, Orraca L, Gonzalez-Martinez JA, Kirakodu SS, et al. Immune system transcriptome in gingival tissues of young nonhuman primates. J Periodontal Res. (2016) 51:152–63. doi: 10.1111/jre.12293
83. Al-Attar A, Alimova Y, Kirakodu S, Kozal A, Novak MJ, Stromberg AJ, et al. Activation of notch-1 in oral epithelial cells by P. gingivalis triggers the expression of the antimicrobial protein PLA2-IIA. Mucosal Immunol. (2018) 11:1047–59. doi: 10.1038/s41385-018-0014-7
84. Gonzalez OA, Kirakodu S, Novak MJ, Stromberg AJ, Orraca L, Gonzalez-Martinez J, et al. Comparative analysis of microbial sensing molecules in mucosal tissues with aging. Immunobiology. (2018) 223:279–87. doi: 10.1016/j.imbio.2017.10.034
85. Gonzalez OA, Novak MJ, Kirakodu S, Orraca L, Chen KC, Stromberg A, et al. Comparative analysis of gingival tissue antigen presentation pathways in ageing and periodontitis. J Clin Periodontol. (2014) 41:327–39. doi: 10.1111/jcpe.12212
86. Ebersole JL, Novak MJ, Orraca L, Martinez-Gonzalez J, Kirakodu S, Chen KC, et al. Hypoxia-inducible transcription factors, HIF1A and HIF2A, increase in aging mucosal tissues. Immunology. (2018) 154:452–64. doi: 10.1111/imm.12894
87. Ebersole JL, Kirakodu S, Novak MJ, Exposto CR, Stromberg AJ, Shen S, et al. Effects of aging in the expression of NOD-like receptors and inflammasome-related genes in oral mucosa. Mol Oral Microbiol. (2016) 31:18–32. doi: 10.1111/omi.12121
88. Ferrin J, Kirakodu S, Jensen D, Al-Attar A, Peyyala R, Novak MJ, et al. Gene expression analysis of neuropeptides in oral mucosa during periodontal disease in non-human primates. J Periodontol. (2018) 89:858–66. doi: 10.1002/JPER.17-0521
89. Pandruvada SN, Gonzalez OA, Kirakodu S, Gudhimella S, Stromberg AJ, Ebersole JL, et al. Bone biology-related gingival transcriptome in ageing and periodontitis in non-human primates. J Clin Periodontol. (2016) 43:408–17. doi: 10.1111/jcpe.12528
90. Ebersole JL, Gonzalez OA. Mucosal circadian rhythm pathway genes altered by aging and periodontitis. PLoS One. (2022) 17:e0275199. doi: 10.1371/journal.pone.0275199
91. Kozich JJ, Westcott SL, Baxter NT, Highlander SK, Schloss PD. Development of a dual-index sequencing strategy and curation pipeline for analyzing amplicon sequence data on the MiSeq illumina sequencing platform. Appl Environ Microbiol. (2013) 79:5112–20. doi: 10.1128/AEM.01043-13
92. Ebersole J, Kirakodu S, Chen J, Nagarajan R, Gonzalez OA. Oral microbiome and gingival transcriptome profiles of ligature-induced periodontitis. J Dent Res. (2020) 99:746–57. doi: 10.1177/0022034520906138
93. Schloss PD, Westcott SL, Ryabin T, Hall JR, Hartmann M, Hollister EB, et al. Introducing mothur: open-source, platform-independent, community-supported software for describing and comparing microbial communities. Appl Environ Microbiol. (2009) 75:7537–41. doi: 10.1128/AEM.01541-09
94. Edgar RC, Haas BJ, Clemente JC, Quince C, Knight R. UCHIME Improves sensitivity and speed of chimera detection. Bioinformatics. (2011) 27:2194–200. doi: 10.1093/bioinformatics/btr381
95. Joseph S, Curtis MA. Microbial transitions from health to disease. Periodontol 2000. (2021) 86:201–9. doi: 10.1111/prd.12377
96. Gong T, Liu L, Jiang W, Zhou R. DAMP-sensing receptors in sterile inflammation and inflammatory diseases. Nat Rev Immunol. (2020) 20:95–112. doi: 10.1038/s41577-019-0215-7
97. Patel S. Danger-Associated molecular patterns (DAMPs): the derivatives and triggers of inflammation. Curr Allergy Asthma Rep. (2018) 18:63. doi: 10.1007/s11882-018-0817-3
98. Jounai N, Kobiyama K, Takeshita F, Ishii KJ. Recognition of damage-associated molecular patterns related to nucleic acids during inflammation and vaccination. Front Cell Infect Microbiol. (2012) 2:168. doi: 10.3389/fcimb.2012.00168
99. Gu Y, Han X. Toll-Like receptor signaling and immune regulatory lymphocytes in periodontal disease. Int J Mol Sci. (2020) 21:3329. doi: 10.3390/ijms21093329
100. Rajaee A, Barnett R, Cheadle WG. Pathogen- and danger-associated molecular patterns and the cytokine response in sepsis. Surg Infect (Larchmt). (2018) 19:107–16. doi: 10.1089/sur.2017.264
101. Walsh D, McCarthy J, O'Driscoll C, Melgar S. Pattern recognition receptors–molecular orchestrators of inflammation in inflammatory bowel disease. Cytokine Growth Factor Rev. (2013) 24:91–104. doi: 10.1016/j.cytogfr.2012.09.003
102. Qian C, Cao X. Regulation of toll-like receptor signaling pathways in innate immune responses. Ann N Y Acad Sci. (2013) 1283:67–74. doi: 10.1111/j.1749-6632.2012.06786.x
103. Kumar S, Ingle H, Prasad DV, Kumar H. Recognition of bacterial infection by innate immune sensors. Crit Rev Microbiol. (2013) 39:229–46. doi: 10.3109/1040841X.2012.706249
104. Yu X, Lin J, Yu Q, Kawai T, Taubman MA, Han X. Activation of toll-like receptor 9 inhibits lipopolysaccharide-induced receptor activator of nuclear factor kappa- B ligand expression in rat B lymphocytes. Microbiol Immunol. (2014) 58:51–60. doi: 10.1111/1348-0421.12115
105. Mori K, Yanagita M, Hasegawa S, Kubota M, Yamashita M, Yamada S, et al. Necrosis-induced TLR3 activation promotes TLR2 expression in gingival cells. J Dent Res. (2015) 94:1149–57. doi: 10.1177/0022034515589289
106. Qian F, Wang X, Zhang L, Chen S, Piecychna M, Allore H, et al. Age-associated elevation in TLR5 leads to increased inflammatory responses in the elderly. Aging Cell. (2012) 11:104–10. doi: 10.1111/j.1474-9726.2011.00759.x
107. Dominguez-Martinez DA, Nunez-Avellaneda D, Castanon-Sanchez CA, Salazar MI. NOD2: activation during bacterial and viral infections, polymorphisms and potential as therapeutic target. Rev Invest Clin. (2018) 70:18–28. doi: 10.24875/RIC.17002327
108. Marchesan J, Jiao YZ, Schaff RA, Hao J, Morelli T, Kinney JS, et al. TLR4, NOD1 and NOD2 mediate immune recognition of putative newly identified periodontal pathogens. Mol Oral Microbiol. (2016) 31:243–58. doi: 10.1111/omi.12116
109. Escamilla-Tilch M, Filio-Rodriguez G, Garcia-Rocha R, Mancilla-Herrera I, Mitchison NA, Ruiz-Pacheco JA, et al. The interplay between pathogen-associated and danger-associated molecular patterns: an inflammatory code in cancer? Immunol Cell Biol. (2013) 91:601–10. doi: 10.1038/icb.2013.58
110. Hirai K, Furusho H, Kawashima N, Xu S, de Beer MC, Battaglino R, et al. Serum amyloid A contributes to chronic apical periodontitis via TLR2 and TLR4. J Dent Res. (2019) 98:117–25. doi: 10.1177/0022034518796456
111. Paknejad M, Sattari M, Roozbahani Z, Ershadi M, Mehrfard A. Relationships between high-mobility group protein B1 and triggering receptor expressed on myeloid cells concentrations in gingival crevicular fluid and chronic periodontitis. Iran J Allergy Asthma Immunol. (2016) 15:381–5.27917624
112. Shin S, Brodsky IE. The inflammasome: learning from bacterial evasion strategies. Semin Immunol. (2015) 27:102–10. doi: 10.1016/j.smim.2015.03.006
113. Li J, Ke X, Yan F, Lei L, Li H. Necroptosis in the periodontal homeostasis: signals emanating from dying cells. Oral Dis. (2018) 24:900–7. doi: 10.1111/odi.12722
114. Wallet SM, Puri V, Gibson FC. Linkage of infection to adverse systemic complications: periodontal disease, toll-like receptors, and other pattern recognition systems. Vaccines (Basel). (2018) 6:21. doi: 10.3390/vaccines6020021
115. Bullon P, Pavillard LE, de la Torre-Torres R. Inflammasome and oral diseases. Exp Suppl. (2018) 108:153–76. doi: 10.1007/978-3-319-89390-7_7
116. Ballambettu SP, Pradeep AR, Purushottam M, Sen S. Higher interleukin-33 levels in aggressive periodontitis cases. J Indian Soc Periodontol. (2019) 23:424–9. doi: 10.4103/jisp.jisp_217_19
117. Pai BS, Pradeep AR. Correlations between interleukin-33 and -1alpha levels in gingival crevicular fluid and plasma in patients with chronic or aggressive periodontitis and disease-free subjects. Bull Tokyo Dent Coll. (2019) 60:279–89. doi: 10.2209/tdcpublication.2019-0002
118. Laperine O, Cloitre A, Caillon J, Huck O, Bugueno IM, Pilet P, et al. Interleukin-33 and RANK-L interplay in the alveolar bone loss associated to periodontitis. PLoS One. (2016) 11:e0168080. doi: 10.1371/journal.pone.0168080
119. Beklen A, Tsaous Memet G. Interleukin-1 superfamily member, interleukin-33, in periodontal diseases. Biotechn Histochem. (2014) 89:209–14. doi: 10.3109/10520295.2013.832800
120. Gaboriaud C, Lorvellec M, Rossi V, Dumestre-Perard C, Thielens NM. Complement system and alarmin HMGB1 crosstalk: for better or worse. Front Immunol. (2022) 13:869720. doi: 10.3389/fimmu.2022.869720
121. Wolf M, Lossdorfer S, Craveiro R, Jager A. High-mobility group box protein-1 released by human-periodontal ligament cells modulates macrophage migration and activity in vitro. Innate Immun. (2014) 20:688–96. doi: 10.1177/1753425913505121
122. Bui FQ, Johnson L, Roberts J, Hung SC, Lee J, Atanasova KR, et al. Fusobacterium nucleatum infection of gingival epithelial cells leads to NLRP3 inflammasome-dependent secretion of IL-1beta and the danger signals ASC and HMGB1. Cell Microbiol. (2016) 18:970–81. doi: 10.1111/cmi.12560
123. Lira-Junior R, Holmstrom SB, Clark R, Zwicker S, Majster M, Johannsen G, et al. S100a12 expression is modulated during monocyte differentiation and reflects periodontitis severity. Front Immunol. (2020) 11:86. doi: 10.3389/fimmu.2020.00086
124. Pradeep AR, Martande SS, Singh SP, Suke DK, Raju AP, Naik SB. Correlation of human S100A12 (EN-RAGE) and high-sensitivity C-reactive protein as gingival crevicular fluid and serum markers of inflammation in chronic periodontitis and type 2 diabetes. Inflamm Res. (2014) 63:317–23. doi: 10.1007/s00011-013-0703-3
125. Lira-Junior R, Bissett SM, Preshaw PM, Taylor JJ, Bostrom EA. Levels of myeloid-related proteins in saliva for screening and monitoring of periodontal disease. J Clin Periodontol. (2021) 48:1430–40. doi: 10.1111/jcpe.13534
126. Jin Y, Wang Y, Lin X. Identification of key gene targets for periodontitis treatment by bioinformatics analysis. BioMed Res Int. (2022) 2022:7992981. doi: 10.1155/2022/7992981
127. Li W, Zheng Q, Meng H, Chen D. Integration of genome-wide association study and expression quantitative trait loci data identifies AIM2 as a risk gene of periodontitis. J Clin Periodontol. (2020) 47:583–93. doi: 10.1111/jcpe.13268
128. Ardila CM, Guzman IC. Comparison of serum amyloid A protein and C-reactive protein levels as inflammatory markers in periodontitis. J Periodontal Implant Sci. (2015) 45:14–22. doi: 10.5051/jpis.2015.45.1.14
129. Song LT, Lai W, Li JS, Mu YZ, Li CY, Jiang SY. The interaction between serum amyloid A and toll-like receptor 2 pathway regulates inflammatory cytokine secretion in human gingival fibroblasts. J Periodontol. (2020) 91:129–37. doi: 10.1002/JPER.19-0050
Keywords: periodontitis, damage-associated molecular patterns, alarmins, microbiome, host response
Citation: Gonzalez OA, Kirakodu SS and Ebersole JL (2023) DAMPs and alarmin gene expression patterns in aging healthy and diseased mucosal tissues. Front. Oral. Health 4:1320083. doi: 10.3389/froh.2023.1320083
Received: 11 October 2023; Accepted: 15 November 2023;
Published: 30 November 2023.
Edited by:
Hatice Hasturk, The Forsyth Institute, United StatesReviewed by:
Tomoki Maekawa, Niigata University, JapanSabine Groeger, University of Giessen, Germany
© 2023 Gonzalez, Kirakodu and Ebersole. This is an open-access article distributed under the terms of the Creative Commons Attribution License (CC BY). The use, distribution or reproduction in other forums is permitted, provided the original author(s) and the copyright owner(s) are credited and that the original publication in this journal is cited, in accordance with accepted academic practice. No use, distribution or reproduction is permitted which does not comply with these terms.
*Correspondence: J. L. Ebersole amVmZnJleS5lYmVyc29sZUB1bmx2LmVkdQ==