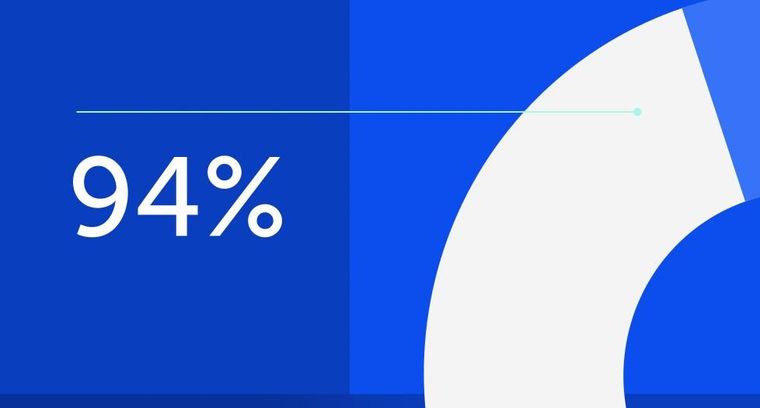
94% of researchers rate our articles as excellent or good
Learn more about the work of our research integrity team to safeguard the quality of each article we publish.
Find out more
ORIGINAL RESEARCH article
Front. Oncol.
Sec. Surgical Oncology
Volume 15 - 2025 | doi: 10.3389/fonc.2025.1590448
This article is part of the Research TopicArtificial Intelligence in Clinical Oncology: Enhancements in Tumor ManagementView all articles
The final, formatted version of the article will be published soon.
Select one of your emails
You have multiple emails registered with Frontiers:
Notify me on publication
Please enter your email address:
If you already have an account, please login
You don't have a Frontiers account ? You can register here
Background and Aims: Esophageal cancer (EC) patients may achieve pathological complete response (pCR) after receiving total neoadjuvant therapy (TNT), which allows them to avoid surgery and preserve organs. We aimed to benchmark the performance of existing artificial intelligence (AI) methods and develop a more accurate model for evaluating EC patients' response after TNT.: We built the Beijing-EC-TNT dataset, consisting of 7,359 images from 300 EC patients who underwent TNT at Beijing Cancer Hospital. The dataset was divided into Cohort1 (4,561 images, 209 patients) for cross-validation and Cohort 2 (2,798 images, 91 patients) for external evaluation. Patients and endoscopic images were labeled as either pCR or non-pCR based on postoperative pathology results. We systematically evaluated mainstream AI models and proposed EC-HAENet, a hybridarchitecture ensembled deep learning model.In image-level classification, EC-HAENet achieved an area under the curve of 0.98 in Cohort 1 and 0.99 in Cohort 2. In patient-level classification, the accuracy of EC-HAENet was significantly higher than that of endoscopic biopsy in both Cohorts 1 and 2 (accuracy, 0.93 vs. 0.78, P<0.0001 and 0.93 vs. 0.71, P<0.0001).EC-HAENet can assist endoscopists in accurately evaluating the response of EC patients after TNT.
Keywords: esophageal cancer, Endoscopy, Total neoadjuvant therapy, Pathological complete response, deep learning
Received: 09 Mar 2025; Accepted: 14 Apr 2025.
Copyright: © 2025 Yuan, Liu, He, Dai, Wu, Chen, Wu and Lu. This is an open-access article distributed under the terms of the Creative Commons Attribution License (CC BY). The use, distribution or reproduction in other forums is permitted, provided the original author(s) or licensor are credited and that the original publication in this journal is cited, in accordance with accepted academic practice. No use, distribution or reproduction is permitted which does not comply with these terms.
* Correspondence:
Ke-Neng Chen, Beijing Cancer Hospital, Peking University, Beijing, 100142, Beijing Municipality, China
Qi Wu, Beijing Cancer Hospital, Peking University, Beijing, 100142, Beijing Municipality, China
Yanye Lu, Peking University, Beijing, China
Disclaimer: All claims expressed in this article are solely those of the authors and do not necessarily represent those of their affiliated organizations, or those of the publisher, the editors and the reviewers. Any product that may be evaluated in this article or claim that may be made by its manufacturer is not guaranteed or endorsed by the publisher.
Supplementary Material
Research integrity at Frontiers
Learn more about the work of our research integrity team to safeguard the quality of each article we publish.