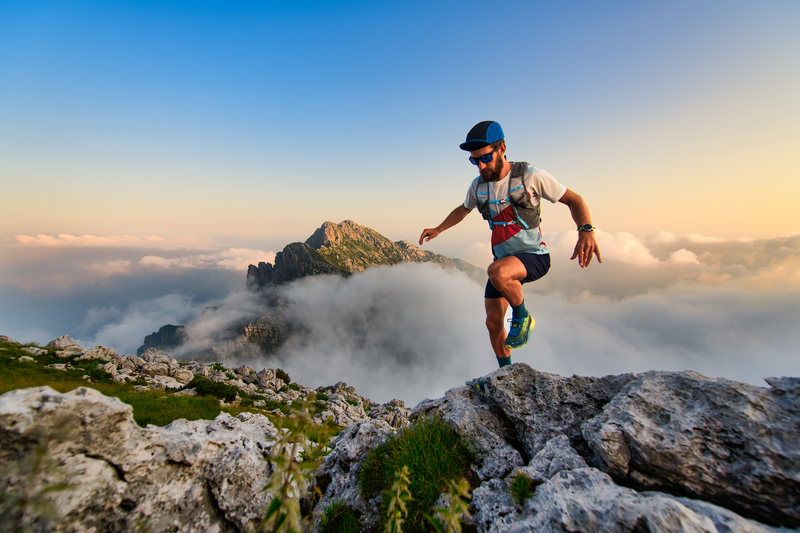
95% of researchers rate our articles as excellent or good
Learn more about the work of our research integrity team to safeguard the quality of each article we publish.
Find out more
ORIGINAL RESEARCH article
Front. Oncol.
Sec. Radiation Oncology
Volume 15 - 2025 | doi: 10.3389/fonc.2025.1585125
This article is part of the Research Topic AI-Based Prognosis Prediction and Dose Optimization Strategy in Radiotherapy for Malignant Tumors View all articles
The final, formatted version of the article will be published soon.
You have multiple emails registered with Frontiers:
Please enter your email address:
If you already have an account, please login
You don't have a Frontiers account ? You can register here
This study develops a machine learning-based predictive model to evaluate the survival outcomes of elderly patients with unresectable hepatocellular carcinoma (HCC) undergoing radiotherapy.The 2377 patients from SEER database were divided into training and internal validation cohorts. Additionally, 99 patients from our hospital were used for an external validation cohort. In the training cohort, 101 machine learning-based radiomics models were developed, and the optimal model's performance was subsequently evaluated in both the internal and external validation cohorts.The StepCox + GBM model demonstrated the highest C-index of 0.7 in the training cohort. The model was further evaluated using area under the receiver operating characteristic (AUC-ROC) curves, with AUC values 2 ranging from 0.736 to 0.783, indicating strong predictive performance. Furthermore, the calibration curve and decision curves confirmed that the model had good predictive performance.The StepCox + GBM model could help optimize the use of radiotherapy for elderly HCC patients, improving survival outcomes and guiding personalized treatment strategies.
Keywords: Hepatocellular Carcinoma, Radiotherapy, survival prognosis, machine learning, survial
Received: 28 Feb 2025; Accepted: 31 Mar 2025.
Copyright: © 2025 Liu and Shi. This is an open-access article distributed under the terms of the Creative Commons Attribution License (CC BY). The use, distribution or reproduction in other forums is permitted, provided the original author(s) or licensor are credited and that the original publication in this journal is cited, in accordance with accepted academic practice. No use, distribution or reproduction is permitted which does not comply with these terms.
* Correspondence:
Xianguo Liu, AVIC 363 Hospital, Chengdu, China
Disclaimer: All claims expressed in this article are solely those of the authors and do not necessarily represent those of their affiliated organizations, or those of the publisher, the editors and the reviewers. Any product that may be evaluated in this article or claim that may be made by its manufacturer is not guaranteed or endorsed by the publisher.
Research integrity at Frontiers
Learn more about the work of our research integrity team to safeguard the quality of each article we publish.