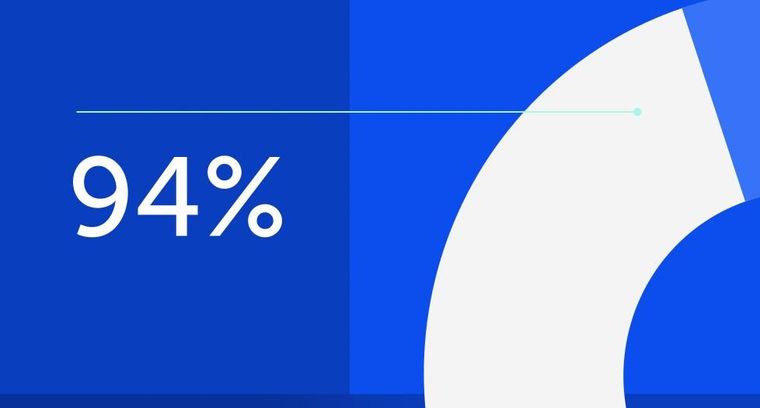
94% of researchers rate our articles as excellent or good
Learn more about the work of our research integrity team to safeguard the quality of each article we publish.
Find out more
MINI REVIEW article
Front. Oncol., 09 April 2025
Sec. Cancer Immunity and Immunotherapy
Volume 15 - 2025 | https://doi.org/10.3389/fonc.2025.1583250
This article is part of the Research TopicCommunity Series in Immunological Precision Therapeutics: Integrating Multi-Omics Technologies and Comprehensive Approaches for Personalized Immune Intervention: Volume IIView all 6 articles
Diffuse large B-cell lymphoma (DLBCL) is a highly heterogeneous malignancy with challenges in treatment resistance and relapse. Single-cell RNA sequencing (scRNA-seq) has provided important insights into tumor heterogeneity, microenvironment interactions, resistance mechanisms, and prognostic biomarkers. This review summarizes key findings from scRNA-seq studies, which have deepened our understanding of DLBCL and contributed to the development of precision therapeutic strategies. Integrating scRNA-seq with spatial transcriptomics and single-cell multi-omics may further elucidate disease mechanisms and identify novel therapeutic targets, supporting the advancement of precision medicine in DLBCL.
Diffuse large B-cell lymphoma (DLBCL), the most prevalent hematologic malignancy in adults, constitutes approximately 35% of non-Hodgkin lymphoma cases (1, 2). Epidemiologic data from the U.S. Cancer Registry reveal an age-standardized incidence rate of 7.2 per 100,000 individuals, with a pronounced male predominance and a clear correlation between advancing age and disease occurrence (3). A significant rise in cases has been noted in areas with historically low incidence rates of DLBCL, despite the fact that the general incidence has remained largely unchanged.
The cornerstone of first-line treatment continues to be the R-CHOP regimen, which consists of vincristine, doxorubicin, cyclophosphamide, prednisone, and rituximab. Although it has significantly improved survival outcomes, approximately 50% of patients experience refractory or relapsed disease following treatment (4–6). The prognosis for patients with refractory DLBCL is poor, with overall survival rates around 20% (7, 8). Chimeric antigen receptor T-cell (CAR-T) therapy has become a viable therapeutic strategy in recent years (9). However, the persistent problems of resistance and recurrence underscore the urgent need for a deeper comprehension of immune escape mechanisms and tumor biology (10, 11).
Single-cell RNA sequencing (ScRNA-seq) is a transformative high-throughput technology that enables high-resolution analysis of gene expression, epigenetic modifications, and intercellular interactions at the single-cell level, offering significant advantages over traditional bulk sequencing (12–16).
In cancer research, scRNA-seq has been widely used to characterize tumor heterogeneity (17–19), identify key gene expression signatures (20–22), and elucidate their roles in tumor progression, metastasis, and drug resistance (17–19, 23). It also enables precise mapping of the tumor immune microenvironment (24, 25), revealing immune cell composition, functional states, and tumor-immune interactions, thereby advancing immunotherapy strategies (26–28). Beyond oncology, scRNA-seq provides critical molecular insights into cardiovascular diseases, muscle development, autoimmune disorders, and tissue regeneration. It has delineated the transcriptional landscape of hypertrophic cardiomyopathy (29), uncovered alternative splicing dynamics in muscle cells (30), and contributed to the study of autoimmune diseases, skin wound healing, and animal physiology (31–34). Moreover, iMLGAM integrates scRNA-seq with machine learning to predict ICB outcomes, offering significant potential for advancing precision medicine (35). Overall, scRNA-seq has significantly contributed to biomedical research, providing a valuable tool for understanding disease mechanisms and supporting precision medicine advancements.
The scRNA-seq enables high-resolution analysis of cellular heterogeneity by isolating individual cells, capturing RNA, and sequencing transcriptomes to generate gene expression profiles (36). Recent advances, such as droplet-based systems (e.g., 10x Genomics) and combinatorial indexing, have enhanced scalability and cost-efficiency, enabling large-scale studies. However, challenges remain, including low capture efficiency, amplification bias, data sparsity, and batch effects, which limit the analysis of low-abundance transcripts. Additionally, scRNA-seq fails to preserve spatial relationships and microenvironmental context, which spatial transcriptomics can partially address (37).
In DLBCL, scRNA-seq has identified key cell subpopulations (e.g., cancer stem cell-like B cells and exhausted T cells), elucidated microenvironment dynamics, and uncovered mechanisms of immune evasion and therapy resistance (38–41) (Figure 1). Despite challenges like technical biases and data integration, scRNA-seq holds immense potential for discovering prognostic biomarkers and therapeutic targets. Integrating scRNA-seq with multi-omics and spatial transcriptomics will further advance precision medicine, improving DLBCL diagnosis, treatment, and patient outcomes.
ScRNA-seq has played a pivotal role in uncovering the tumor heterogeneity of DLBCL. Fengling Liu et al. conducted a single-cell analysis of B-cell subpopulations in DLBCL and normal lymphoid tissues, revealing that the high infiltration of cancer stem cell-like B-cell subpopulations is significantly associated with poor patient prognosis. They discovered a critical transcription factor network controlled by HMGB3, SAP30, and E2F8, and confirmed the existence of this subpopulation through immunofluorescence assays (38). Shu Wang et al. examined the heterogeneity between primary central nervous system DLBCL and extracerebral DLBCL at both the transcriptomic and genomic levels, revealing that mutations in the TLR and BCR-NFκB pathways are critical drivers of malignant B-cell proliferation within the central nervous system (42).
In terms of immune cell functional heterogeneity, Qiqi Zhu focused on the exhaustion heterogeneity of CD8+ tumor-infiltrating lymphocytes (TILs) in DLBCL, revealing that exhausted CD8+ TILs comprise both precursor and terminal states. She also identified that the CD39/A2AR pathway may drive the exhaustion process of these TILs (43). By analyzing single-cell databases in DLBCL, Zhencang Zhou discovered a significantly higher proportion of exhausted T cells in DLBCL compared to normal tissues, along with a notable upregulation of the ID3 gene in these exhausted T cells (44). Furthermore, Anthony R. Colombo et al. compared the expression of immune checkpoint molecules, such as PD-L1, PD-1, and TIM-3, in Hodgkin lymphoma and DLBCL using imaging mass cytometry and single-cell spatial analysis. Potential therapeutic targets like TIM-3, CCR4, and CXCR3 were found via their study (45).
In terms of signal transduction, a study utilized phospho-specific flow cytometry to analyze the B-cell signaling profiles of non-Hodgkin lymphoma patients. The researchers found that lymphoma cells from DLBCL patients exhibited higher phosphorylation levels at multiple signaling nodes, a significant distinction from those observed in mantle cell lymphoma (46). These findings emphasize the potential of targeted therapies that tackle tumor cell heterogeneity. Future advancements in single-cell technologies may further refine treatment strategies and improve prognostic accuracy in DLBCL.
Tumor heterogeneity in DLBCL shapes a dynamic interplay between tumor cells and immune cells within the microenvironment. Single-cell analysis has revealed that diverse tumor subpopulations influence the microenvironment, contributing to immune evasion, tumor progression, and resistance to treatment. These subpopulations interact with immune cells and stromal components, creating a complex microenvironment that drives disease progression. ScRNA-seq has provided key insights into these intercellular interactions, emphasizing the importance of understanding tumor-immune cell dynamics for developing more effective therapeutic strategies in DLBCL.
Benoit Manfroi et al. found through single-cell sequencing that tumor-associated macrophages (TAMs) in DLBCL exhibit atypical genetic characteristics and express both M1 and M2 macrophage marker genes, which may explain the lack of significant influence of TAMs in DLBCL progression (47). Additionally, studies have shown that highly immunosuppressive activated tumor-infiltrating regulatory T cells (Tregs) are significantly enriched in DLBCL tissues, suggesting that they may play a crucial role in tumorigenesis, progression, and treatment response (39). By comparing scRNA-seq data from DLBCL patients and control samples, Xiaofei Ye et al. identified a CD70-CD27 interaction between malignant B cells and T cells. They also discovered that co-inhibitory signaling through TIM3 and TIGIT may be a major driver of T cell exhaustion and highlighted that HBV infection could influence DLBCL progression by promoting malignant cell survival or inducing immune escape (48). Together, these studies highlight the intricate interactions between immune and tumor cells within the DLBCL microenvironment, offering valuable insights for the design of therapies targeting the microenvironment.
ScRNA-seq technology has made significant progress in uncovering the mechanisms of resistance in DLBCL. Xiyue Xu et al. employed scRNA-seq to comprehensively analyze the genomic features of the CD58 in DLBCL patients. Their study revealed that CD58 deletion could increase PD-L1 and IDO expression by activating the LYN/CD22/SHP1 signaling pathway, thereby facilitating immune evasion and contributing to treatment resistance (40). In terms of T-cell function regulation, Tingting Zhang et al. proposed that targeting the PD-1/PD-L1/L2 pathway in combination with CD73/A2aR could reverse T-cell dysfunction, providing a new therapeutic strategy for DLBCL (49). Additionally, Zi-Xun Yan et al. explored the potential mechanisms of resistance to CAR-T therapy in DLBCL patients through scRNA-seq. They discovered that cholesterol efflux in M2 macrophages might induce CD8+ T-cell exhaustion, thereby impairing CAR-T cell anti-tumor responses. The study suggested that cholesterol-lowering drugs or antibodies targeting HAVCR2 or TIGIT, when combined with CAR-T therapy, might enhance therapeutic efficacy (50).
Epigenetic regulation has also provided new insights for overcoming resistance in DLBCL. Oliver H. Krämer and Günter Schneider reviewed the potential of combining c-FOS and HDAC inhibitors (HDACi) in DLBCL, noting that this combined strategy might enhance anti-tumor efficacy through the modulation of epigenetic modifications (51). Further studies revealed that the potent HDACi LAQ824 could effectively kill DLBCL cells, and when used in combination with a c-Fos inhibitor, it significantly enhanced anti-tumor activity, offering a new direction for HDACi combination therapy (52).
In targeted therapy, Syahru Agung Setiawan et al. found that the BTK inhibitor zanubrutinib, in combination with the BCL-2 inhibitor navitoclax, synergistically suppressed double-hit lymphoma by inducing apoptosis and ferroptosis, providing a new combination therapy strategy to overcome DLBCL resistance (53). Additionally, the mechanism of bendamustine-rituximab (BR) therapy in DLBCL partially relied on apoptosis and enhanced immune responses induced by the cGAS-STING pathway, providing a conceptual framework for the clinical use of BR therapy (54).
In conclusion, these studies have identified key resistance mechanisms in DLBCL, including immune evasion, epigenetic alterations, and dysregulated signaling pathways, which provide critical insights for the development of combination therapies. By integrating scRNA-seq with functional assays such as CRISPR screens, a deeper understanding of these mechanisms is achieved, enabling the identification and validation of potential therapeutic targets (55). ScRNA-seq uncovers gene mutations and pathway changes, while CRISPR screens facilitate the functional validation of these targets, offering a promising strategy for therapeutic advancement.
ScRNA-seq technology has advanced significantly in the investigation of DLBCL prognostic indicators, revealing a close relationship between various cell types and molecular features with patient prognosis.
In terms of B lymphocytes, Zijun Y. Xu-Monette et al. found that the prognosis of DLBCL patients was substantially correlated with the abundance of tumor-infiltrating B lymphocytes (TIL-B), with patients with high TIL-B abundance showing larger proportions of memory B cells and naive CD4 T cells (56).
In T cells, Sylvia Zöphel et al. showed that CD16+ T cell populations had a protective role, with higher CD16+ T cell counts correlating with better prognosis, suggesting their potentialas markers for progression-free survival in aggressive B-NHL,including DLBCL (41). T-cell dysfunction was identified as a core mechanism of immune evasion and CAR-T therapy failure in DLBCL. Jinrong Zhao pointed out that intrinsic defects in CAR-T cells were one of the reasons for the variation in treatment efficacy (57). However, while these markers offer promising insights, their clinical validation in larger cohorts is still needed. Jin Jin et al., through scRNA-seq, discovered that elevated levels of Ki67, CD57, and TIM3, along with decreased CD69 levels in T cells, were associated with poor prognosis (58). Yao Wang et al. further found that a high proportion of CD8+ CAR-T cells and enhanced activation of CD8+ stem cell-like memory T cell populations were key to prolonging clinical efficacy, while the absence of CCR7 gene expression might explain the variability in CAR-T therapy outcomes (59). Qiqi Zhu et al., through scRNA-seq, revealed that the TIM3/Galectin-9 pathway induces exhaustion in CD8+ tumor-infiltrating lymphocytes, which is associated with immune suppression, poor prognosis, and a reduced response to CHOP chemotherapy (60). Nianping Liu et al., analyzing the tumor microenvironment of primary central nervous system DLBCL (PCNS DLBCL), found that a plasmablast-like program was linked to worse prognosis, with a higher score of exhausted CD8 T cells possibly contributing to poor outcomes (61).
In macrophages, their polarization status was closely associated with prognosis in DLBCL. Baoping Guo developed a prognostic model based on M2 macrophage-related genes (MS4A4A, CCL13, LTB, etc.), finding that patients in the high-risk group had poorer prognosis but were more sensitive to chemotherapy drugs and immune checkpoint inhibitors (62). Guangcan Gao et al. discovered that high expression of C1qC M2 macrophages predicted poor prognosis, with C1qC expression positively correlating with immune checkpoint molecules (63). Min Liu et al., through comprehensive transcriptomic analysis of macrophages in reactive lymphoid tissue (RLT) and different spatial regions of DLBCL, revealed transcriptomic differences in macrophages between the two, and established six macrophage signature profiles (MacroSigs) from distinct spatial sources. They found that specific MacroSigs were closely associated with the cellular subtypes of DLBCL and patient survival rates (64). These findings suggest potential therapeutic implications, but their clinical significance in guiding treatment strategies still requires robust validation.
In monocytes, Juliette Ferrant et al. identified S100A9^high monocytes as potential biomarkers for DLBCL, suggesting their role in tumor progression (65). Additionally, elevated expression of S100A8 was associated with poor prognosis in DLBCL, and inhibiting S100A8 was found to promote apoptosis and suppress tumor growth (66).
The role of other immune-related biomarkers in DLBCL prognosis has also attracted significant attention. Other immune-related biomarkers, such as CD161 monoclonal antibodies identified by Francesca Alvarez Calderon, have also shown potential for improving prognosis (67), but their clinical application is yet to be established. Martin A. Rivas et al. demonstrated that the cohesin complex played a key role in lymphoma development, and reduced expression of its subunits was linked to poorer prognosis in DLBCL patients (68). Jing Tang et al. developed a prognostic feature model based on exocytosis-related molecules, including SNRPB and CEP290, and confirmed the predictive capability of this model through immunohistochemistry (69). Additionally, Jurriaan Brouwer-Visser et al. found that CD20 expression loss in patients with relapsed/refractory B-cell non-Hodgkin lymphoma treated with Odronextamab could be a potential mechanism of resistance (70). In relapsed/refractory (R/R) DLBCL, scRNA-seq analysis of peripheral blood mononuclear cell samples identified 12 biomarkers (CD82, CD55, CD36, CD63, CD59, IKZF1, CD69, CD163, CD14, CD226, CD84, and CD31) that were notably upregulated. These markers correlate with patient prognosis and may offer potential new targets for therapy in R/R DLBCL (71). These biomarkers, while promising, require validation in larger, diverse patient populations to determine their clinical relevance in guiding treatment decisions.
The dual-protein expression lymphoma (DUEL) diagnostic method, based on the co-expression of BCL2 and MYC at the single-cell level, was validated through multiplex immunofluorescence and dual immunohistochemistry. The study demonstrated that DUEL was an independent adverse prognostic factor for DLBCL patients, providing a reliable basis for identifying high-risk patients and developing new therapeutic strategies (72). Liyuan Dai et al. combined single-cell and spatial transcriptomics technologies to reveal that the glycolytic metabolic activity in highly malignant DLBCL cells was significantly increased. High-glycolysis tissues exhibited abundant IFN_TAMs and reduced CD8+ T cells, and glycolysis gene expression was positively correlated with tumor malignancy. Immunohistochemical analysis validated the prognostic potential of glycolytic biomarkers such as STMN1, ENO1, PKM, and CDK1 (73). Additionally, radiomics parameters showed potential in prognostic prediction for DLBCL. One study has found that high total metabolic tumor volume (TMTV) and low tumor-infiltrating (TI) CD4+ cell levels are independently associated with poorer prognosis. Combining TMTV with TI cell analysis enhances the accuracy of prognostic prediction (74), but these findings need to be integrated with clinical data to validate their prognostic value.
ScRNA-seq technologies have demonstrated remarkable potential in DLBCL research, revealing key insights into tumor heterogeneity, microenvironment interactions, resistance mechanisms, and prognostic biomarkers. These studies have not only provided new perspectives for understanding the biological characteristics of DLBCL but also laid a solid foundation for developing precision therapeutic strategies. However, further validation of these findings in clinical settings is needed. Future research should explore how integrative approaches, such as spatial transcriptomics or single-cell multi-omics, can refine our understanding of DLBCL pathogenesis and help identify novel therapeutic targets. Overall, scRNA-seq could be instrumental in advancing early diagnosis, treatment monitoring, and personalized therapy, ultimately enhancing the field of precision medicine for DLBCL.
LL: Writing – original draft, Writing – review & editing. QL: Visualization, Writing – original draft. RN: Writing – original draft. WS: Writing – original draft, Writing – review & editing. HL: Supervision, Writing – original draft, Writing – review & editing.
The author(s) declare that no financial support was received for the research and/or publication of this article.
The Graphical Abstract was created using Figdraw (ID: UOAITf888c).
The authors declare that the research was conducted in the absence of any commercial or financial relationships that could be construed as a potential conflict of interest.
The author(s) declare that no Generative AI was used in the creation of this manuscript.
All claims expressed in this article are solely those of the authors and do not necessarily represent those of their affiliated organizations, or those of the publisher, the editors and the reviewers. Any product that may be evaluated in this article, or claim that may be made by its manufacturer, is not guaranteed or endorsed by the publisher.
1. Xu PP, Huo YJ, Zhao WL. All roads lead to targeted diffuse large B-cell lymphoma approaches. Cancer Cell. (2022) 40:131–3. doi: 10.1016/j.ccell.2022.01.013
2. Chapuy B, Stewart C, Dunford AJ, Kim J, Kamburov A, Redd RA, et al. Molecular subtypes of diffuse large B cell lymphoma are associated with distinct pathogenic mechanisms and outcomes. Nat Med. (2018) 24:679–90. doi: 10.1038/s41591-018-0016-8
3. Wang SS. Epidemiology and etiology of diffuse large B-cell lymphoma. Semin Hematol. (2023) 60:255–66. doi: 10.1053/j.seminhematol.2023.11.004
4. Matthews JM, Bhatt S, Patricelli MP, Nomanbhoy TK, Jiang X, Natkunam Y, et al. Pathophysiological significance and therapeutic targeting of germinal center kinase in diffuse large B-cell lymphoma. Blood. (2016) 128:239–48. doi: 10.1182/blood-2016-02-696856
5. Habermann TM, Weller EA, Morrison VA, Gascoyne RD, Cassileth PA, Cohn JB, et al. Rituximab-CHOP versus CHOP alone or with maintenance rituximab in older patients with diffuse large B-cell lymphoma. J Clin Oncol. (2006) 24:3121–7. doi: 10.1200/JCO.2005.05.1003
6. Coiffier B, Lepage E, Briere J, Herbrecht R, Tilly H, Bouabdallah R, et al. CHOP chemotherapy plus rituximab compared with CHOP alone in elderly patients with diffuse large-B-cell lymphoma. N Engl J Med. (2002) 346:235–42. doi: 10.1056/NEJMoa011795
7. Sehn LH, Salles G. Diffuse large B-cell lymphoma. N Engl J Med. (2021) 384:842–58. doi: 10.1056/NEJMra2027612
8. Crump M, Neelapu SS, Farooq U, Van Den Neste E, Kuruvilla J, Westin J, et al. Outcomes in refractory diffuse large B-cell lymphoma: results from the international SCHOLAR-1 study. Blood. (2017) 130:1800–8. doi: 10.1182/blood-2017-03-769620
9. Safarzadeh KP, Safarzadeh KP, Rahbarizadeh F. Novel antigens of CAR T cell therapy: New roads; old destination. Transl Oncol. (2021) 14:101079. doi: 10.1016/j.tranon.2021.101079
10. Chong EA, Ruella M, Schuster SJ. Five-year outcomes for refractory B-cell lymphomas with CAR T-cell therapy. N Engl J Med. (2021) 384:673–4. doi: 10.1056/NEJMc2030164
11. Spiegel JY, Dahiya S, Jain MD, Tamaresis J, Nastoupil LJ, Jacobs MT, et al. Outcomes of patients with large B-cell lymphoma progressing after axicabtagene ciloleucel therapy. Blood. (2021) 137:1832–5. doi: 10.1182/blood.2020006245
12. Hou M, Zhao Z, Li S, Zhang Z, Li X, Zhang Y, et al. Single-cell analysis unveils cell subtypes of acral melanoma cells at the early and late differentiation stages. J Cancer. (2025) 16:898–916. doi: 10.7150/jca.102045
13. Li H, Bian Y, Xiahou Z, Zhao Z, Zhao F, Zhang Q. The cellular signaling crosstalk between memory B cells and tumor cells in nasopharyngeal carcinoma cannot be overlooked: Their involvement in tumor progression and treatment strategy is significant. J Cancer. (2025) 16:288–314. doi: 10.7150/jca.101420
14. Lin L, Zou J, Pei S, Huang W, Zhang Y, Zhao Z, et al. Germinal center B-cell subgroups in the tumor microenvironment cannot be overlooked: Their involvement in prognosis, immunotherapy response, and treatment resistance in head and neck squamous carcinoma. Heliyon. (2024) 10:e37726. doi: 10.1016/j.heliyon.2024.e37726
15. Zhang Y, Zhao Z, Huang W, Kim BS, Lin L, Li X, et al. Pan-cancer single-cell analysis revealing the heterogeneity of cancer-associated fibroblasts in skin tumors. Curr Gene Ther. (2024). doi: 10.2174/0115665232331353240911080642
16. Nie W, Zhao Z, Liu Y, Wang Y, Zhang J, Hu Y, et al. Integrative single-cell analysis of cardiomyopathy identifies differences in cell stemness and transcriptional regulatory networks among fibroblast subpopulations. Cardiol Res Pract. (2024) 2024:3131633. doi: 10.1155/2024/3131633
17. Jin W, Zhang Y, Zhao Z, Gao M. Developing targeted therapies for neuroblastoma by dissecting the effects of metabolic reprogramming on tumor microenvironments and progression. Theranostics. (2024) 14:3439–69. doi: 10.7150/thno.93962
18. Ge Q, Zhao Z, Li X, Yang F, Zhang M, Hao Z, et al. Deciphering the suppressive immune microenvironment of prostate cancer based on CD4+ regulatory T cells: Implications for prognosis and therapy prediction. Clin Transl Med. (2024) 14:e1552. doi: 10.1002/ctm2.1552
19. Ding Y, Zhao Z, Cai H, Zhou Y, Chen H, Bai Y, et al. Single-cell sequencing analysis related to sphingolipid metabolism guides immunotherapy and prognosis of skin cutaneous melanoma. Front Immunol. (2023) 14:1304466. doi: 10.3389/fimmu.2023.1304466
20. Lin Z, Wang F, Yin R, Li S, Bai Y, Zhang B, et al. Single-cell RNA sequencing and immune microenvironment analysis reveal PLOD2-driven Malignant transformation in cervical cancer. Front Immunol. (2024) 15:1522655. doi: 10.3389/fimmu.2024.1522655
21. Shao W, Lin Z, Xiahou Z, Zhao F, Xu J, Liu X, et al. Single-cell RNA sequencing reveals that MYBL2 in Malignant epithelial cells is involved in the development and progression of ovarian cancer. Front Immunol. (2024) 15:1438198. doi: 10.3389/fimmu.2024.1438198
22. Zhao F, Hong J, Zhou G, Huang T, Lin Z, Zhang Y, et al. Elucidating the role of tumor-associated ALOX5+ mast cells with transformative function in cervical cancer progression via single-cell RNA sequencing. Front Immunol. (2024) 15:1434450. doi: 10.3389/fimmu.2024.1434450
23. Lei Y, Tang R, Xu J, Wang W, Zhang B, Liu J, et al. Applications of single-cell sequencing in cancer research: progress and perspectives. J Hematol Oncol. (2021) 14:91. doi: 10.1186/s13045-021-01105-2
24. Huang W, Kim BS, Zhang Y, Lin L, Chai G, Zhao Z. Regulatory T cells subgroups in the tumor microenvironment cannot be overlooked: Their involvement in prognosis and treatment strategy in melanoma. Environ Toxicol. (2024) 39:4512–30. doi: 10.1002/tox.24247
25. Zhao Z, Ding Y, Tran LJ, Chai G, Lin L. Innovative breakthroughs facilitated by single-cell multi-omics: manipulating natural killer cell functionality correlates with a novel subcategory of melanoma cells. Front Immunol. (2023) 14:1196892. doi: 10.3389/fimmu.2023.1196892
26. Sun L, Shao W, Lin Z, Lin J, Zhao F, Yu J. Single-cell RNA sequencing explored potential therapeutic targets by revealing the tumor microenvironment of neuroblastoma and its expression in cell death. Discovery Oncol. (2024) 15:409. doi: 10.1007/s12672-024-01286-5
27. Li S, Yao J, Zhang S, Zhou X, Zhao X, Di N, et al. Prognostic value of tumor-microenvironment-associated genes in ovarian cancer. Bio Integr. (2023) 4. doi: 10.15212/bioi-2022-0008
28. Wang Z, Dai Z, Zhang H, Zhang N, Liang X, Peng L, et al. Comprehensive analysis of pyroptosis-related gene signatures for glioblastoma immune microenvironment and target therapy. Cell Prolif. (2023) 56. doi: 10.1111/cpr.13376
29. Lu J, Ren J, Liu J, Lu M, Cui Y, Liao Y, et al. High-resolution single-cell transcriptomic survey of cardiomyocytes from patients with hypertrophic cardiomyopathy. Cell Prolif. (2024) 57. doi: 10.1111/cpr.13557
30. Chen G, Chen J, Qi L, Yin Y, Lin Z, Wen H, et al. Bulk and single-cell alternative splicing analyses reveal roles ofTRA2Bin myogenic differentiation. Cell Prolif. (2024) 57. doi: 10.1111/cpr.13545
31. Luo S, Wang L, Xiao Y, Cao C, Liu Q, Zhou Y. Single-cell RNA-sequencing integration analysis revealed immune cell heterogeneity in five human autoimmune diseases. Bio Integr. (2023) 4. doi: 10.15212/bioi-2023-0012
32. Cheng XC, Tong WZ, Rui W, Feng Z, Shuai H, Zhe W. Single-cell sequencing technology in skin wound healing. Burns Trauma. (2024) 12:tkae043. doi: 10.1093/burnst/tkae043
33. Xia Y, Wang Y, Shan M, Hao Y, Liang Z. Decoding the molecular landscape of keloids: new insights from single-cell transcriptomics. Burns Trauma. (2023) 11:tkad017. doi: 10.1093/burnst/tkad017
34. Wu JJ, Zhu S, Tang YF, Gu F, Valencak TG, Liu JX, et al. Age- and microbiota-dependent cell stemness plasticity revealed by cattle cell landscape. Res (Wash D C). (2023) 6:25. doi: 10.34133/research.0025
35. Ye B, Fan J, Xue L, Zhuang Y, Luo P, Jiang A, et al. iMLGAM: Integrated Machine Learning and Genetic Algorithm-driven Multiomics analysis for pan-cancer immunotherapy response prediction. Imeta. (2025) 4(2025):e70011. doi: 10.1002/imt2.70011
36. Grun D, van Oudenaarden A. Design and analysis of single-cell sequencing experiments. Cell. (2015) 163:799–810. doi: 10.1016/j.cell.2015.10.039
37. Llorens-Rico V, Simcock JA, Huys G, Raes J. Single-cell approaches in human microbiome research. Cell. (2022) 185:2725–38. doi: 10.1016/j.cell.2022.06.040
38. Liu F, Zheng J, Yang G, Pan L, Xie Y, Chen S, et al. Unraveling the enigma of B cells in diffuse large B-cell lymphoma: unveiling cancer stem cell-like B cell subpopulation at single-cell resolution. Front Immunol. (2023) 14:1310292. doi: 10.3389/fimmu.2023.1310292
39. Spasevska I, Sharma A, Steen CB, Josefsson SE, Blaker YN, Kolstad A, et al. Diversity of intratumoral regulatory T cells in B-cell non-Hodgkin lymphoma. Blood Adv. (2023) 7:7216–30. doi: 10.1182/bloodadvances.2023010158
40. Xu X, Zhang Y, Lu Y, Zhang X, Zhao C, Wang J, et al. CD58 alterations govern antitumor immune responses by inducing PDL1 and IDO in diffuse large B-cell lymphoma. Cancer Res. (2024) 84:2123–40. doi: 10.1158/0008-5472.CAN-23-2874
41. Zophel S, Kuchler N, Jansky J, Hoxha C, Schafer G, Weise JJ, et al. CD16+ as predictive marker for early relapse in aggressive B-NHL/DLBCL patients. Mol Cancer. (2024) 23:210. doi: 10.1186/s12943-024-02123-7
42. Wang S, Chen H, Dai B, Zheng K, Zheng J, Zhu Y, et al. Comparison of differences in transcriptional and genetic profiles between intra-central nervous system and extra-central nervous system large B-cell lymphoma. Neoplasia. (2025) 60:101119. doi: 10.1016/j.neo.2024.101119
43. Zhu Q, Yang Y, Zeng Y, Chen K, Zhang Q, Wang L, et al. The significance of CD8(+) tumor-infiltrating lymphocytes exhaustion heterogeneity and its underlying mechanism in diffuse large B-cell lymphoma. Int Immunopharmacol. (2024) 137:112447. doi: 10.1016/j.intimp.2024.112447
44. Zhou Z, Zhu P, Ge J, Li Q, Li H, Zhe N, et al. Gene regulatory mechanisms of T cell exhaustion in diffuse large B cell lymphoma based on single-cell transcriptome data. Leuk Res. (2024) 146:107588. doi: 10.1016/j.leukres.2024.107588
45. Colombo AR, Hav M, Singh M, Xu A, Gamboa A, Lemos T, et al. Single-cell spatial analysis of tumor immune architecture in diffuse large B-cell lymphoma. Blood Adv. (2022) 6:4675–90. doi: 10.1182/bloodadvances.2022007493
46. Myklebust JH, Brody J, Kohrt HE, Kolstad A, Czerwinski DK, Walchli S, et al. Distinct patterns of B-cell receptor signaling in non-Hodgkin lymphomas identified by single-cell profiling. Blood. (2017) 129:759–70. doi: 10.1182/blood-2016-05-718494
47. Manfroi B, De Grandis M, Moreaux J, Tabruyn S, Mayol JF, Quintero M, et al. The microenvironment of DLBCL is characterized by noncanonical macrophages recruited by tumor-derived CCL5. Blood Adv. (2021) 5:4338–51. doi: 10.1182/bloodadvances.2021004203
48. Ye X, Wang L, Nie M, Wang Y, Dong S, Ren W, et al. A single-cell atlas of diffuse large B cell lymphoma. Cell Rep. (2022) 39:110713. doi: 10.1016/j.celrep.2022.110713
49. Zhang T, Liu H, Jiao L, Zhang Z, He J, Li L, et al. Genetic characteristics involving the PD-1/PD-L1/L2 and CD73/A2aR axes and the immunosuppressive microenvironment in DLBCL. J Immunother Cancer. (2022) 10. doi: 10.1136/jitc-2021-004114
50. Yan ZX, Dong Y, Qiao N, Zhang YL, Wu W, Zhu Y, et al. Cholesterol efflux from C1QB-expressing macrophages is associated with resistance to chimeric antigen receptor T cell therapy in primary refractory diffuse large B cell lymphoma. Nat Commun. (2024) 15:5183. doi: 10.1038/s41467-024-49495-4
51. Kramer OH, Schneider G. Single-cell profiling guided combination therapy of c-Fos and histone deacetylase inhibitors in diffuse large B-cell lymphoma. Clin Transl Med. (2022) 12:e858. doi: 10.1002/ctm2.858
52. Wang L, Wu Z, Xia Y, Lu X, Li J, Fan L, et al. Single-cell profiling-guided combination therapy of c-Fos and histone deacetylase inhibitors in diffuse large B-cell lymphoma. Clin Transl Med. (2022) 12:e798. doi: 10.1002/ctm2.798
53. Setiawan SA, Liu WZ, Weng PW, Lee CH, Yadav VK, Hardianti MS, et al. Synergistic disruption of BTK and BCL-2 causes apoptosis while inducing ferroptosis in double-hit lymphoma. Eur J Pharmacol. (2023) 943:175526. doi: 10.1016/j.ejphar.2023.175526
54. Xiao R, Zhao W, Lin W, Xiao Y, Ren J, Zhou Y, et al. Bendamustine-rituximab elicits dual tumoricidal and immunomodulatory responses via cGAS-STING activation in diffuse large B-cell lymphoma. J Immunother Cancer. (2024) 12. doi: 10.1136/jitc-2024-009212
55. Replogle JM, Saunders RA, Pogson AN, Hussmann JA, Lenail A, Guna A, et al. Mapping information-rich genotype-phenotype landscapes with genome-scale Perturb-seq. Cell. (2022) 185:2559–75. doi: 10.1016/j.cell.2022.05.013
56. Xu-Monette ZY, Li Y, Snyder T, Yu T, Lu T, Tzankov A, et al. Tumor-infiltrating normal B cells revealed by immunoglobulin repertoire clonotype analysis are highly prognostic and crucial for antitumor immune responses in DLBCL. Clin Cancer Res. (2023) 29:4808–21. doi: 10.1158/1078-0432.CCR-23-1554
57. Zhao J, Wei C, Wang S, Zhang Y, Wang W, Zhao D, et al. The intrinsic defects of T cells impact the efficacy of CAR-T therapy in patients with diffuse large B-cell lymphoma. Blood Cancer J. (2023) 13:186. doi: 10.1038/s41408-023-00958-9
58. Jin J, Lin L, Meng J, Jiang L, Zhang M, Fang Y, et al. High-multiplex single-cell imaging analysis reveals tumor immune contexture associated with clinical outcomes after CAR T cell therapy. Mol Ther. (2024) 32:1252–65. doi: 10.1016/j.ymthe.2024.03.023
59. Wang Y, Tong C, Lu Y, Wu Z, Guo Y, Liu Y, et al. Characteristics of premanufacture CD8(+)T cells determine CAR-T efficacy in patients with diffuse large B-cell lymphoma. Signal Transduct Target Ther. (2023) 8:409. doi: 10.1038/s41392-023-01659-2
60. Zhu Q, Yang Y, Chen K, Zhang Q, Huang Y, Jian S. Diffuse large B-cell lymphoma: the significance of CD8(+) tumor-infiltrating lymphocytes exhaustion mediated by TIM3/Galectin-9 pathway. J Transl Med. (2024) 22:174. doi: 10.1186/s12967-024-05002-3
61. Liu N, Jiang C, Yao X, Fang M, Qiao X, Zhu L, et al. Single-cell landscape of primary central nervous system diffuse large B-cell lymphoma. Cell Discovery. (2023) 9:55. doi: 10.1038/s41421-023-00559-7
62. Guo B, Duan Y, Cen H. Prognostic model based on M2 macrophage-related signatures for predicting outcomes, enhancing risk stratification, and providing therapeutic insights in diffuse large B-cell lymphoma. Heliyon. (2024) 10:e41007. doi: 10.1016/j.heliyon.2024.e41007
63. Gao G, Sun N, Zhang Y, Li J, Jiang Y, Chen N, et al. Single-cell sequencing in diffuse large B-cell lymphoma: C1qC is a potential tumor-promoting factor. Int Immunopharmacol. (2024) 143:113319. doi: 10.1016/j.intimp.2024.113319
64. Liu M, Bertolazzi G, Sridhar S, Lee RX, Jaynes P, Mulder K, et al. Spatially-resolved transcriptomics reveal macrophage heterogeneity and prognostic significance in diffuse large B-cell lymphoma. Nat Commun. (2024) 15:2113. doi: 10.1038/s41467-024-46220-z
65. Ferrant J, Lhomme F, Le Gallou S, Irish JM, Roussel M. Circulating myeloid regulatory cells: promising biomarkers in B-cell lymphomas. Front Immunol. (2020) 11:623993. doi: 10.3389/fimmu.2020.623993
66. Lin Q, Su J, Fang Y, Zhong Z, Chen J, Zhang C. S100A8 is a prognostic signature and associated with immune response in diffuse large B-cell lymphoma. Front Oncol. (2024) 14:1344669. doi: 10.3389/fonc.2024.1344669
67. Alvarez CF, Kang BH, Kyrysyuk O, Zheng S, Wang H, Mathewson ND, et al. Targeting of the CD161 inhibitory receptor enhances T-cell-mediated immunity against hematological Malignancies. Blood. (2024) 143:1124–38. doi: 10.1182/blood.2023022882
68. Rivas MA, Durmaz C, Kloetgen A, Chin CR, Chen Z, Bhinder B, et al. Cohesin core complex gene dosage contributes to germinal center derived lymphoma phenotypes and outcomes. Front Immunol. (2021) 12:688493. doi: 10.3389/fimmu.2021.688493
69. Tang J, Lu B, Bin T, Xu XJ, Lin C, Wang Y, et al. SNRPB and CEP290, predicting the prognosis of diffuse large B cell lymphoma and associated with tumour immune microenvironment. Ann Med. (2024) 56:2425065. doi: 10.1080/07853890.2024.2425065
70. Brouwer-Visser J, Fiaschi N, Deering RP, Cygan KJ, Scott D, Jeong S, et al. Molecular assessment of intratumoral immune cell subsets and potential mechanisms of resistance to odronextamab, a CD20xCD3 bispecific antibody, in patients with relapsed/refractory B-cell non-Hodgkin lymphoma. J Immunother Cancer. (2024) 12. doi: 10.1136/jitc-2023-008338
71. Shi Y, Ding W, Gu W, Shen Y, Li H, Zheng Z, et al. Single-cell phenotypic profiling to identify a set of immune cell protein biomarkers for relapsed and refractory diffuse large B cell lymphoma: A single-center study. J Leukoc Biol. (2022) 112:1633–48. doi: 10.1002/JLB.6MA0822-720RR
72. Roh J, Yoon DH, Lee YK, Pak HK, Kim SY, Han JH, et al. Significance of single-cell level dual expression of BCL2 and MYC determined with multiplex immunohistochemistry in diffuse large B-cell lymphoma. Am J Surg Pathol. (2022) 46:289–99. doi: 10.1097/PAS.0000000000001830
73. Dai L, Fan G, Xie T, Li L, Tang L, Chen H, et al. Single-cell and spatial transcriptomics reveal a high glycolysis B cell and tumor-associated macrophages cluster correlated with poor prognosis and exhausted immune microenvironment in diffuse large B-cell lymphoma. biomark Res. (2024) 12:58. doi: 10.1186/s40364-024-00605-w
Keywords: single-cell RNA sequencing, diffuse large B-cell lymphoma (DLBCL), tumor heterogeneity, microenvironment, prognostic biomarkers
Citation: Li L, Li Q, Niu R, Sun W and Liang H (2025) Single-cell RNA sequencing in diffuse large B-cell lymphoma: tumor heterogeneity, microenvironment, resistance, and prognostic markers. Front. Oncol. 15:1583250. doi: 10.3389/fonc.2025.1583250
Received: 25 February 2025; Accepted: 26 March 2025;
Published: 09 April 2025.
Edited by:
Zhiheng Lin, Shanghai University of Traditional Chinese Medicine, ChinaReviewed by:
Wei Wang, Nanjing Medical University, ChinaCopyright © 2025 Li, Li, Niu, Sun and Liang. This is an open-access article distributed under the terms of the Creative Commons Attribution License (CC BY). The use, distribution or reproduction in other forums is permitted, provided the original author(s) and the copyright owner(s) are credited and that the original publication in this journal is cited, in accordance with accepted academic practice. No use, distribution or reproduction is permitted which does not comply with these terms.
*Correspondence: Hua Liang, aGxqdWNtX2xpYW5naHVhQDEyNi5jb20=
Disclaimer: All claims expressed in this article are solely those of the authors and do not necessarily represent those of their affiliated organizations, or those of the publisher, the editors and the reviewers. Any product that may be evaluated in this article or claim that may be made by its manufacturer is not guaranteed or endorsed by the publisher.
Research integrity at Frontiers
Learn more about the work of our research integrity team to safeguard the quality of each article we publish.