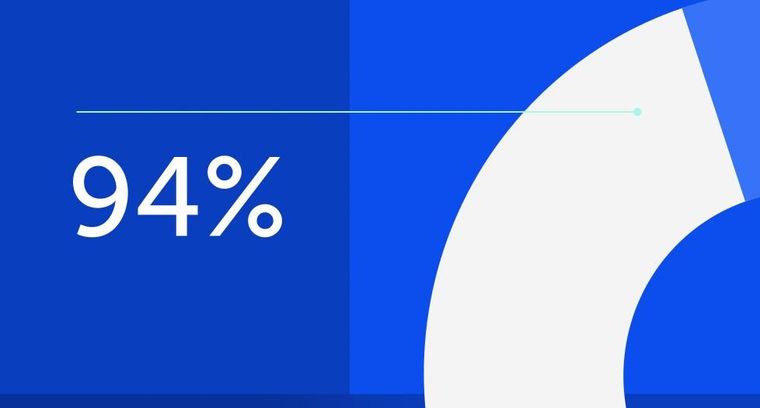
94% of researchers rate our articles as excellent or good
Learn more about the work of our research integrity team to safeguard the quality of each article we publish.
Find out more
ORIGINAL RESEARCH article
Front. Oncol.
Sec. Head and Neck Cancer
Volume 15 - 2025 | doi: 10.3389/fonc.2025.1577198
This article is part of the Research TopicNext-Generation Technologies and Multidisciplinary Integration for Oral Cancer Diagnosis and TreatmentView all articles
The final, formatted version of the article will be published soon.
Select one of your emails
You have multiple emails registered with Frontiers:
Notify me on publication
Please enter your email address:
If you already have an account, please login
You don't have a Frontiers account ? You can register here
Objectives: Oral cavity-derived cancer pathological images (OPI) are crucial for diagnosing oral squamous cell carcinoma (OSCC), but existing deep learning methods for OPI segmentation rely heavily on large, accurately labeled datasets, which are labor-and resource-intensive to obtain. This paper presents a semi-supervised segmentation method for OPI to mitigate the limitations of scarce labeled data by leveraging both labeled and unlabeled data. Materials and Methods: We use the Hematoxylin and Eosin (H&E)-stained oral cavity-derived cancer dataset (OCDC), which consists of 451 images with tumor regions annotated and verified by pathologists. Our method combines transformation uncertainty and multi-scale contrastive learning. The transformation uncertainty estimation evaluates the model's confidence on data transformed via different methods, reducing discrepancies between the teacher and student models. Multi-scale contrastive learning enhances class similarity and separability while reducing teacher-student model similarity, encouraging diverse feature representations. Additionally, a boundary-aware enhanced U-Net is proposed to capture boundary information and improve segmentation accuracy. Results: Experimental results on the OCDC dataset demonstrate that our method outperforms both fully supervised and existing semi-supervised approaches, achieving superior segmentation performance. Conclusions: Our semi-supervised method, integrating transformation uncertainty, multi-scale contrastive learning, and a boundary-aware enhanced U-Net, effectively addresses data scarcity and improves segmentation accuracy. This approach reduces the dependency on large labeled datasets, promoting the application of AI in OSCC detection and improving the efficiency and accuracy of clinical diagnoses for OSCC.
Keywords: Pathological image segmentation, Semi-Supervised Learning, Oral cavity-derived cancer, Contrastive learning, Uncertainty Estimation
Received: 15 Feb 2025; Accepted: 14 Apr 2025.
Copyright: © 2025 Wang, Lyu and Yu. This is an open-access article distributed under the terms of the Creative Commons Attribution License (CC BY). The use, distribution or reproduction in other forums is permitted, provided the original author(s) or licensor are credited and that the original publication in this journal is cited, in accordance with accepted academic practice. No use, distribution or reproduction is permitted which does not comply with these terms.
* Correspondence:
Chengqi Lyu, Department of Stomatology, Shanghai Sixth People's Hospital Affiliated to Shanghai Jiao Tong University School of Medicine, Shanghai, China
Lvfeng Yu, Department of Stomatology, Shanghai Sixth People's Hospital Affiliated to Shanghai Jiao Tong University School of Medicine, Shanghai, China
Disclaimer: All claims expressed in this article are solely those of the authors and do not necessarily represent those of their affiliated organizations, or those of the publisher, the editors and the reviewers. Any product that may be evaluated in this article or claim that may be made by its manufacturer is not guaranteed or endorsed by the publisher.
Supplementary Material
Research integrity at Frontiers
Learn more about the work of our research integrity team to safeguard the quality of each article we publish.