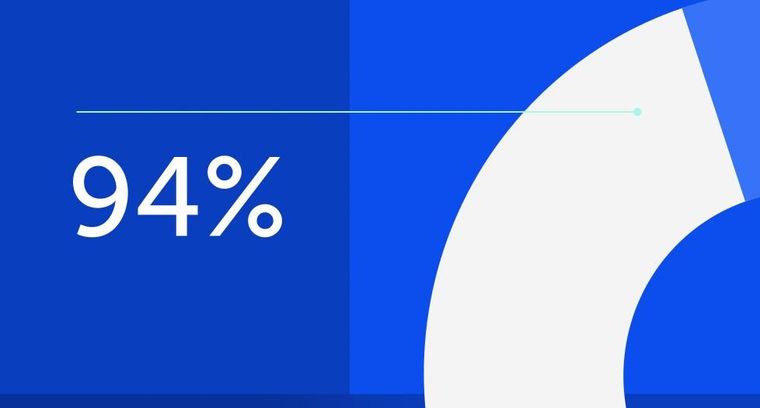
94% of researchers rate our articles as excellent or good
Learn more about the work of our research integrity team to safeguard the quality of each article we publish.
Find out more
ORIGINAL RESEARCH article
Front. Oncol.
Sec. Cancer Imaging and Image-directed Interventions
Volume 15 - 2025 | doi: 10.3389/fonc.2025.1572720
This article is part of the Research TopicAdvances in Oncological Imaging TechniquesView all 4 articles
The final, formatted version of the article will be published soon.
Select one of your emails
You have multiple emails registered with Frontiers:
Notify me on publication
Please enter your email address:
If you already have an account, please login
You don't have a Frontiers account ? You can register here
Purpose The goal of this paper is to compare the effectiveness of three deep learning models (2D, 3D, and 2.5D), three radiomics models (INTRA, Peri2mm, and Fusion2mm), and a combined model in predicting the spread through air spaces (STAS) in non-small cell lung cancer (NSCLC) to identify the optimal model for clinical surgery planning.We included 480 patients who underwent surgery at four centers between January 2019 and August 2024, dividing them into a training cohort, an internal test cohort, and an external validation cohort. We extracted deep learning features using the ResNet50 algorithm. Least absolute shrinkage selection operator(Lasso) and spearman rank correlation were utilized to choose features. Extreme Gradient Boosting (XGboost) was used to execute deep learning and radiomics. Then, a combination model was developed, integrating both sources of data. Result The combined model showed outstanding performance, with an area under the receiver operating characteristic curve (AUC) of 0.927 (95% CI 0.870 -0.984) in the test set and 0.867 (95% CI 0.819 -0.915) in the validation set. This model significantly distinguished between high-risk and low-risk patients and demonstrated significant advantages in clinical application. Conclusion: The combined model is adequate for preoperative prediction of STAS in patients with stage T1 NSCLC, outperforming the other six models in predicting STAS risk.
Keywords: deep learning, Radiomics, Lung Adenocarcinoma, artificial intelligence, STAS
Received: 07 Feb 2025; Accepted: 16 Apr 2025.
Copyright: © 2025 Xu, Yu, Xing, Zhang, Hu, Li, Jia, Zhi and Xiuhua. This is an open-access article distributed under the terms of the Creative Commons Attribution License (CC BY). The use, distribution or reproduction in other forums is permitted, provided the original author(s) or licensor are credited and that the original publication in this journal is cited, in accordance with accepted academic practice. No use, distribution or reproduction is permitted which does not comply with these terms.
* Correspondence:
Pengliang Xu, The First People's Hospital of Huzhou, Huzhou, China
Peng Xiuhua, The First People's Hospital of Huzhou, Huzhou, China
Disclaimer: All claims expressed in this article are solely those of the authors and do not necessarily represent those of their affiliated organizations, or those of the publisher, the editors and the reviewers. Any product that may be evaluated in this article or claim that may be made by its manufacturer is not guaranteed or endorsed by the publisher.
Supplementary Material
Research integrity at Frontiers
Learn more about the work of our research integrity team to safeguard the quality of each article we publish.